Abstract
Biodiversity and ecosystem functions are highly threatened by global change. It has been proposed that geodiversity can be used as an easy-to-measure surrogate of biodiversity to guide conservation management. However, so far, there is mixed evidence to what extent geodiversity can predict biodiversity and ecosystem functions at the regional scale relevant for conservation planning. Here, we analyse how geodiversity computed as a compound index is suited to predict the diversity of four taxa and associated ecosystem functions in a tropical mountain hotspot of biodiversity and compare the results with the predictive power of environmental conditions and resources (climate, habitat, soil). We show that combinations of these environmental variables better explain species diversity and ecosystem functions than a geodiversity index and identified climate variables as more important predictors than habitat and soil variables, although the best predictors differ between taxa and functions. We conclude that a compound geodiversity index cannot be used as a single surrogate predictor for species diversity and ecosystem functions in tropical mountain rain forest ecosystems and is thus little suited to facilitate conservation management at the regional scale. Instead, both the selection and the combination of environmental variables are essential to guide conservation efforts to safeguard biodiversity and ecosystem functions.
Similar content being viewed by others
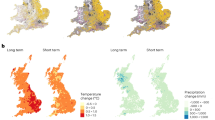
Introduction
Safeguarding biodiversity1,2,3 and ecosystem functions under global change4,5 is of key importance for the provision of essential services to human societies6. Geodiversity indices (i.e., the diversity of earth system's abiotic and structural features) are increasingly used to predict biodiversity and ecosystem functions1,2,7. A comprehensive review on how the term geodiversity evolved and diversified over time is given in ref.8. Early concepts of geodiversity proposed the conservation of a broad range of environments to maintain biological diversity9, in particular, considering geological features of a region (Supplementary Table S1). These concepts were later extended by a variety of geomorphological, hydrological and pedological features (Supplementary Table S1, see also ref.10). To describe geodiversity, simple feature numbers were initially used but later, inspired by biology, replaced by metrics such as the Shannon diversity index11,12,13.
Using geodiversity as a surrogate for biodiversity burgeoned around the new millennium13, mainly to identify areas for conservation or natural heritage or to manage the exploitation of ecosystem services12,14,15,16. This endeavour was based on the hypothesis that specific geo-sites should support unique biota so that high geodiversity is coupled with high biodiversity17,18,19. This concept has been particularly addressed by the Conserving Nature's Stage (CNS) approach which focusses on the use of geodiversity in conservation planning9,18,19. Because geodiversity was expected to be more resilient against environmental changes than biodiversity, it was claimed that preserving geodiversity should safeguard biodiversity and ecosystem functions and services20,21,22. Consequently, geodiversity data, in parts easily available through remote sensing, were proposed as suitable planning tools to predict biodiversity and ecosystem functions, particularly in the frequent case of biodiversity data unavailability23,24,25.
To date, different types of geodiversity indices are used to predict biodiversity pattern8. They range from simple feature-based to complex multivariate indices such as geodiversity indices that consider climate, hydrology, soil, topography and habitat, and their variability in space and time (Supplementary Table S1, see also ref.23). Our understanding of the concept of geodiversity is in line with the resource-based measure of geodiversity explained in ref.23 (also discussed in ref.8). In the following, we will refer to geodiversity as the spatial variation of environmental variables. The environmental variables (also elsewhere termed as abiotic conditions, abiotic variables or abiotic stages26) used in this study consider conditions and resources related to climate, habitat and soil which are requirements for the establishment and survival of organisms and have been used as meaningful predictors of species diversity and ecosystem functions (Supplementary Methods). These variables are here used as a direct (the value measured at a plot) or indirect (the diversity of multiple variables at a plot and its surrounding; hereafter the compound geodiversity index) surrogate for species diversity and ecosystem functions (see Methods and Supplementary Methods). Our view on using the environmental variables to calculate a geodiversity compound index is supported by a review on the CNS approach that recommends to consider a broader spectrum of environmental variables at a finer thematic resolution27. At the same time, a harmonization of geodiversity approaches has recently been claimed to reach intercomparability of studies focussing on the geodiversity-biodiversity relationship, and further test this relationship for underrepresented ecosystems (such as tropical mountain rain forests)7.
While biodiversity is defined by the wealth and variety of life, depending on the scale and the level of complexity, a range of measures can be applied to surrogate it, i.e., the diversity of ecosystems, communities, or taxa28,29. So far, most biodiversity studies used measures of species diversity such as species richness, or abundance-weighted diversity metrics, e.g. the Shannon index30. This is often due to the simplicity of these indices and the ease with which they can be compared within and across ecosystems. As taxonomic species diversity alone, however, misses much of the overall diversity of an ecosystem31, measures of ecosystem functions can be additionally addressed to describe the state of an ecosystem. Ecosystem functions are often defined as the biological, physical, and geochemical processes that occur within an ecosystem and consider the sizes of compartments (such as carbon storage) as well as the rates of their changes (such as rates of carbon sequestration)32. They can provide or regulate ecosystem services32 and are, therefore, an integral part of the biodiversity of ecosystems32.
Using the Shannon index for the calculation of geodiversity and species diversity could thus facilitate the direct comparisons of geodiversity and species diversity. But research on geodiversity-biodiversity relations showed different performance depending on selected ecosystem, taxa, and scale, where geodiversity predictors seemed to generally work better for intermediate to large scales33,34,35. CNS research activities, however, revealed best performance of geo-biodiversity at landscape to regional scales that are most important for conservation planning26. Also challenging is often the match of geo- and biodiversity scales. It is important that the environmental variables used for the calculation of a geodiversity compound index are drivers of the species diversity of the taxon under investigation. Since the strength of environmental variables as predictors differs between different taxa, it is unlikely that there is a geodiversity index which can be used as a surrogate of species diversity across multiple taxa. Therefore, the choice of environmental variables to calculate taxon-specific geodiversity indices is of great importance. Not only the environmental variables but also the scales used to calculate the geodiversity compound index should have ecological significance for the taxa and ecosystem functions studied35.
Here, we test the hypothesis that geodiversity compound indices are powerful predictors of species diversity and ecosystem functions in a tropical mountain rainforest hotspot for biodiversity. Specifically, we compare predictive models of species diversity and ecosystem functions based on a geodiversity index with those based on environmental variables. In this study we use the following definitions: (i) Environmental variables consider the conditions and resources at each plot. (ii) The geodiversity index is assembled as the sum of the spatial diversity of multiple environmental variables measured within each plot and its surrounding (see Methods and Supplementary Fig. S1). For both, (i) and (ii) environmental variables belong to the three groups climate, habitat, and soil, each comprising a set of different environmental conditions and resources available as spatial grids (Table 1). We consider one condition or resource from each group and subsequently use the selected three environmental variables as predictors of species diversity and ecosystem functions (Extended Data Table E3). For the geodiversity compound index, all spatial grids are classified, and the central pixel of each plot and its adjacent pixels are used to calculate their spatial diversity analogous to the calculation of Shannon diversity (Supplementary Fig. S1). The geodiversity index is subsequently calculated by summing up the diversities of the selected environmental variables indicating the degree of variation across space. Comparison of the geodiversity index models with models of the environmental variables has been conducted for the following taxa: trees, testate amoebae, ants and birds, and their associated ecosystem functions: carbon sequestration, decomposition, predation, and seed dispersal, along an elevational gradient ranging from 1000 to 3000 m a.s.l.
Results and discussion
Geodiversity and environmental variables
First, we compared the extent to which the geodiversity index and environmental variables were associated with species diversity and ecosystem functions using GAMs (generalized additive model, Fig. 1, see also Extended Data Figs. E1, E2, Extended Data Tables E3, E4, E5). Contrary to the expectation, we found that the geodiversity index explains only a very limited amount of the variation of species diversity and ecosystem functions along the elevational gradient. In contrast, models based on selected environmental conditions and resources explain most of the variation in species diversity and associated ecosystem functions (Fig. 1).
Explained deviance of species diversity (left) and ecosystem functions (right) by a geodiversity index as well as environmental variables. Models using environmental variables are based on three predictors accounting for conditions and resources of the three groups climate, habitat, and soil, while models using the geodiversity index consider the summed spatial diversities of the same three predictors. The predictor sets are selected individually for each response (depicted in Fig. 2) and are hold equal for both models based on the geodiversity index as well as environmental variables (see also Extended Data Figs. E1 and E2).
Our findings contradict the results of previous studies which successfully linked geo- and biodiversity35,36. On closer inspection however, only a few of the previous studies rely on geodiversity indices only (Supplementary Table S1). Most of them used mixed sets of predictors comprising both, geodiversity features and indices, and environmental variables considering different conditions and resources (e.g., climate, hydrology, soil, habitat, topography). For instance, ref.10 combined geodiversity indices with levels of potential radiation (climate) and NDVI (Normalized Difference Vegetation Index; habitat) to explain biodiversity. In many studies that used such a mixed set of predictors, environmental conditions and resources had higher predictive power than geodiversity indices to explain biodiversity across taxa and scales5,34,37.
A reason for the relatively poor performance of geodiversity indices might be the information loss by aggregating fine-grain resource data into lower resolution compound metrics. Different predictors with reversed patterns might cancel each other out in geodiversity compound indices1. Secondly, scale mismatches might impact the predictive performance of geodiversity. In general, the combination of environmental variables should match the targeted measurement scales at which the diversities of taxa and ecosystem functions have been sampled. In our study, these measurement scales varied depending on the different taxonomic groups studied and the associated ecosystem functions due to differences in activity ranges among the taxa (Methods). On the other hand, the used environmental data varied in their spatial resolution, mainly due to the fact that we used mostly freely available data at a certain spatial resolution (Table 1) which is a common practice in geo-biodiversity studies38. While there is only a small mismatch in the case of models using environmental variables (e.g., comparing tree Shannon diversity measured within an area of 400 m2 with the values of environmental predictors at 30 m spatial resolution), the mismatch increases when calculating spatial diversity measures from these environmental variables. In detail, the spatial scale of the environmental data in our study is in a resolution of 6–30 m and for the calculation of geodiversity the diversity measures are calculated in a 3 pixel × 3 pixel environment (320–8100 m2) which differ from the measurement scales of the investigated taxa and ecosystem functions (400–10,000 m2). In addition, this limitation resulted in geodiversity indices that in some cases combined the spatial variation of environmental variables calculated within different areas. This is a limitation of our study and needs to be addressed in future research as it is a common practice in geodiversity studies to combine remote sensing products such as digital elevation models at only moderate spatial resolution with local field data at finer resolution35,39. But as stated in ref.26 smaller mismatches between geo- and biodiversity can be tolerated and, furthermore, the knowledge that the plots have been chosen to be representative of their surrounding environment also counteracts this limitation.
Despite these limitations and recommendations, our findings suggest that geodiversity computed as a single compound index is not able to model species diversity and ecosystem functions in the here studied highly diverse tropical mountain ecosystem. This is in line with the findings in ref.38, that geodiversity indices better work as a surrogate for common than rare species, which often occur in biodiversity hotspot areas. By contrast, we showed that environmental variables are fundamental to conservation planning, as they imply that the availability of specific environmental conditions and resources, rather than surrogate geodiversity indices, are required to accurately predict species diversity and ecosystem functions.
Relationships of environmental variables with species diversity and ecosystem functions
The selected environmental predictors accounting for climate, habitat, and soil variables revealed broad-scale relationships with species diversity for most taxa (Fig. 2a–d, lower panels) and ecosystem functions (Fig. 2e–h, lower panels). In the univariate models, climate conditions explained the patterns in species diversity and ecosystem functions on average better than habitat and soil predictors. In addition, variation partitioning of multivariate models showed that the most important predictors considering climate, habitat and soil differed among taxa and ecosystem functions (Fig. 2, upper panels). Conditions of air temperature were the main driver of animal diversity and ecosystem functions, whereas habitat conditions gained in relevance for plants and soil-associated taxa and functions. While tree diversity was best explained by habitat but with little explanatory support, C-sequestration was strongly related to climate, but at high shared explained variance with soil and habitat predictors. The diversity of the least mobile taxon, testate amoebae, was best explained by habitat. An increase in the diversity of testate amoebae was positively related to an increasing forest coverage which is associated with lower climatic stress40. Less important predictors for the diversity of testate amoebae were soil P as a key food resource40, and humidity41. Litter decomposition was best explained by climate, and in particular an increase in mean annual temperature (MAT). Litter decomposition is known to be related to the soil microbiome42 whose activity is positively related to MAT43. An increase in ant diversity and ant predation was best explained by climate, i.e., a low seasonal variability in air temperature. Ant diversity is generally positively related to enhanced temperatures44,45. Because ants with specifically narrow optimum temperature ranges are mostly occurring in the tropics, they particularly profit from low temperature fluctuations46. Bird diversity and seed dispersal by birds were positively related to MAT. This relationship corroborates findings on the global scale47. The importance of MAT for seed dispersal is similar to the effect on bird diversity itself, which points to more dispersal of seeds under increased temperatures. Furthermore, bird diversity showed a hump-shaped relationship to habitat, i.e., a spatial texture metric of the NDVI, confirming that species diversity is positively related to the number of niches in heterogeneous habitats48. The relationships between species richness and ecosystem functions with environmental conditions and resources found in this study are largely consistent with the patterns found in other tropical mountain systems49,50 such as Kilimanjaro51.
Relative importance of the three environmental variables accounting for conditions and resources from climate, habitat, and soil in explaining species diversity of four taxonomic groups (a–d) and related ecosystem functions (e–h). Upper panels: We conducted variation partitioning (VP) based on generalized additive models (GAM). Venn diagrams show the pure proportions explained exclusively by each predictor, and their share of explained deviance (expl. dev.) due to overlapping effects of pairs of predictors or all three predictors. A negative value indicates that the explained deviance by the predictor overlap is less than that explained separately by the predictors62. Lower panels: Dots present the response of the three selected predictors (same as in the upper panel) against Shannon taxon diversity as a measure of species diversity and the associated ecosystem functions. Lines depict the respective univariate GAM smooth functions with the corresponding explained deviance indicated. NDVI: Normalized Difference Vegetation Index, sd: standard deviation of air temperature and air humidity over time. The reader may refer to the Methods section and the Supplementary Methods for the individual selection procedure of the three predictors for each response. The plant and animal images used in the figure were provided by Cordula Mann and Christiane Enderle.
Relevance of scale, identity, and complementarity of geodiversity for biodiversity and ecosystem functions
So far, most evidence for a general relationship between geodiversity, biodiversity and ecosystem functions is based on global analyses2 (but see also refs.34,37). Based on these studies, geodiversity has been proposed to be in principle a useful surrogate of biodiversity for conservation planning on the global scale4,37. Yet, our results revealed that species diversity and ecosystem functioning cannot be inferred from a geodiversity index on the regional spatial scale within a biodiversity hotspot. Instead, we found that the combination of individually selected environmental conditions and resources was much more indicative and thus, is needed to reveal associations between biodiversity and its environment on regional spatial scales. Geodiversity approaches that work well on a global scale are apparently of relatively little value for conservation management that must be implemented on the regional scale.
Our findings are analogous to those from biodiversity-ecosystem function (BEF) research where trait values are often more relevant than trait diversity to understand the role of biodiversity for ecosystem functioning at regional scales52,53,54. Similarly, a geodiversity index does not hold as an all-in-one solution to predict species diversity and ecosystem functioning. Instead, the individual environmental conditions and resources matter—and they differ between the different components of biodiversity and ecosystem functions. Both, trait identity and complementarity are needed to predict ecosystem functions from biodiversity52,53,54. This analogy suggests that research linking geo- with biodiversity and ecosystem functions might go through a similar progression of conceptual and methodological refinement as BEF research. Consequently, it must be also acknowledged, that from a conservation perspective, the here targeted species diversity is always a simplification of overall species composition. Species composition, and thus changes in species identity, are therefore often more informative and thus relevant to the conservation of biodiversity and functions55,56,57,58. Since the Shannon index is limited in surrogating the biotic and abiotic composition of ecosystems, we suggest that future studies should test geodiversity-biodiversity relationships focusing on the biotic and abiotic composition of environments instead of their diversity. This could be realised, i.e. using techniques of ordination to link the composition of taxa and their environment in a distance-based framework58,59,60,61.
Conclusion
Our study identifies four key research gaps that future geo-biodiversity approaches should address and test with comparative studies. First, geodiversity indices seem to be better predictors of biodiversity in simple than in complex ecosystems given the selection and availability of key conditions or resources could be more important than the composition of different conditions and resources for biodiversity and functionality in these systems34,37. Second, the relevance of spatial scale, i.e., at larger spatial and temporal scales geodiversity indices might be more relevant than the identity of specific environmental variables given that it provides higher flexibility depending on the components of biodiversity and functions that are studied63. Third, the need to consider species identity in geodiversity studies, e.g., by testing the relationship between species and environmental composition instead of diversity. Fourth, the tailored selection of environmental conditions and resources is important as these affect the extent to which biodiversity and ecosystem functions can be predicted34,37. Our study provides new insights into how combinations of environmental variables, rather than geodiversity indices, shape species diversity and ecosystem functions at regional scales. At such spatial scales, both the selection and the combination of environmental variables can increase our mechanistic understanding and support conservation management of biodiversity and ecosystem functions.
Methods
Study area
We exemplify our analysis on the mountain rainforest of south-eastern Ecuador that belongs to the biodiversity hotspot of the tropical Andes which is globally most important for conservation support64. We use data of a unique ecosystem, the mountain rainforest of the eastern Andean slopes65. This is essential to avoid confounding effects from multiple biogeographic units, as it is the case in most other studies on the relation of geo- and biodiversity. The field data were collected between 2007 and 2017 along an elevational gradient between 1000 and 3000 m a.s.l. (Fig. 3) with study sites equally distributed along the gradient66,67,68.
Location of the study area in the southern part of Ecuador (left) and its three main elevational levels from 3000 to 1000 m a.s.l. (white boxes from left to right). Map created in QGIS 3.14.16-Pi (https://qgis.org/). Background imagery (right): Landsat-8 true-color composite (in courtesy of USGS, https://earthexplorer.usgs.gov/).
Species diversity and ecosystem functions
We assessed the species diversity of four taxa and four associated ecosystem functions using standardized methods. The details of the sampling procedure of each taxon and function have mostly been described in their original publications. We sampled species diversity data of trees40,69, testate amoebae70,71, ants72 and birds73. For ants, species abundance data from two field campaigns were pooled and for birds, nine point count records per plot were pooled to obtain the bird species abundance of all species and individuals per plot. Species abundance for trees and testate amoebae was measured based on distinct samples. To compare the Shannon diversity of the four taxa in a standardized way, we used a rarefaction and extrapolation framework based on sample coverage, implemented in the R package iNEXT74,75. In this framework, it is statistically valid to extrapolate the observed Shannon diversity values to the maximal sample coverage (the asymptote of the rarefaction and extrapolation curve). We therefore set the extrapolation ratio for the calculation of the standardized species diversity values to one, corresponding to a complete sample coverage for all taxa76.
Four important ecosystem functions that are associated with the four taxa were measured in the study area. C-sequestration was observed as the aboveground net primary production77, deduced from annual wood production and annual fine litter production (tree diversity/C-sequestration, partial overlap of plots, Pearson r = 0.35, p < 0.01, n = 52). The decomposition of soil organic matter was measured using a Tea Bag experiment78 reflecting the weight loss of tea bags after 21 days (testate amoebae diversity/decomposition, distinct plots). Predation was assessed using the number of bite marks of ants on artificial caterpillars72 (ant diversity/predation, Pearson r = 0.40 p < 0.05, n = 27). Seed dispersal was measured as the number of observed seed removal events of frugivorous birds on fleshy fruited plants79 (bird diversity/seed dispersal, Pearson r = 0.62, p < 0.01, n = 15).
Environmental variables
In this study we investigated a set of 13 environmental variables based on conditions and resources within the three groups climate, habitat and soil (Table 1). We did not include geology which has often been used in other geodiversity research studies due to the geological homogeneity of our study area66. The predictors of the three groups were available as spatial datasets or were spatially predicted with varying spatial resolution (see Table 1). The following predictors were considered for the three groups:
Climate: We used climate stations in combination with a Landsat-8 image classification (recorded on 20/11/2016, see also ref.77) to derive gridded forest temperature and humidity data at a spatial resolution of 30 m following previous approaches80,81. We calculated four climatic predictors: the annual mean, maximum and standard deviation of mean monthly air temperatures over the year as well as the mean annual relative air humidity.
Habitat: Based on the pre-processed Landsat-8 scene, we calculated the Normalized Difference Vegetation Index (NDVI) and its textural metric ‘correlation’ using all directions in the ‘glcm’ package82 in R as well as the Red-Blue Difference Vegetation index (RBVI, see refs.77,83) and the forest cover per pixel in percentage. These habitat variables are known to be related to habitat structure and productivity in the study area77,83. In addition, we used the Sentinel-2 product Leaf Area Index (LAI) available at 10 m spatial resolution. To characterize topography, we calculated the Topographical Position Index (TPI) based on an airborne high-spatial-resolution digital elevation model from the SIGTIERRAS campaign83.
Soil: Due to the lack of spatial soil data products at a moderate spatial resolution, we modeled and spatially predicted a suite of soil variables sampled in the study area84. These soil variables were related to phosphorus and nitrogen availability and pH (in H2O) in the mineral soil horizon. We used multi-linear models with a stepwise predictor selection (forward and backward) using spatial predictors (Extended Data Table E1). Only three models explained more than 45% in organic layer depth, Phosphorus content in Ah-horizon and pH in Bv-horizon. The explained variance of their models ranged between 45 and 69% (Extended Data Table E2). Their spatial predictions were subsequently used as soil predictors.
Environmental variables and geodiversity indices
Our study targets to compare models of species diversity and ecosystem functions using combinations of environmental predictors grouped within climate, habitat, and soil variables with models using a single geodiversity compound index which is defined as the summed spatial diversity of the same selected environmental variables. For this purpose, from each group (climate, habitat, and soil) one condition or resource has been selected as predictor. The choice of a predictor within each group (e.g., temperature, rainfall etc. out of climate) was guided by the findings of prior studies (Extended Data Table E3, Supplementary Methods) and the resulting combination of environmental variables, thus, comprises the requirements that the corresponding organisms need for their establishment and survival. A second criterion was the collinearity present in the predictor space. We changed the predictor combination if the correlation between two or more predictors were greater than r = 0.6. Whenever present, different statistics such as mean, maximum, and standard deviation of the climate group have been compared and the environmental variables with the best performance concerning the Akaike information criterion of the corresponding model fit was chosen. For all response variables, the pixel values of the selected environmental variables were used that overlapped with either the point or shape geometries of the corresponding plots. The resulting predictor set, thus, assess the central tendency of three environmental factors at each plot.
The geodiversity index, in contrast, is computed as the summed spatial diversity of the same three environmental factors measured within each plot and its surrounding and follows the recent plea to consistently use diversity indices commonly applied in biodiversity studies7. For this purpose, we classified the spatial grids of the three selected predictors into five classes using intervals following the Fisher style85 in the ‘classInt’ R package86. After the classification, the central pixel of each plot and in addition all adjacent pixels were extracted. This resulted in nine pixels per plot. For these pixels, the frequency of all present classes was calculated. From an ecological perspective each class was used analogous to a present species at the corresponding plot and their frequency was used as their abundance. For the resulting abundance matrix of the three predictors, Shannon diversity was calculated using the diversity function of the ‘vegan’ package87,88. This results in three predictors addressing the spatial variation of the chosen environmental variables. As in some ecological studies where the diversity of multiple taxa has been summed or averaged to study multi-taxa diversity51, we here treated each of the three predictors like a single taxon. Thus, analogously to multi-taxa approaches, we calculated the geodiversity index by summing up the spatial diversity of the three environmental variables (for further explanation see also Supplementary Fig. S1).
Statistical analyses
We used generalized additive regression models (GAMs) to model species diversity, and ecosystem functions. To address the (non-)linear relationships between the index of geodiversity, species diversity and ecosystem functions, univariate GAMs were used. Multivariate GAMs were used to address the relationships to the three environmental variables considering conditions and resources of the three groups climate, habitat, and soil. For all models, GAMs were calibrated using a Gaussian error distribution in the model fitting based on the exploration of residuals and the generalized cross-validation score of the models (GCVS). Non-linear relationships were considered using cubic regression splines with a degree of freedom permitted to vary between one and three. GAMs however maintained the flexibility to model linear relationships wherever present. We performed all GAM analyses using the ‘mgcv’ package in R89. The model performance of combined environmental variables and the geodiversity index among all models was compared (Fig. 1).
For the combination of environmental variables, we further explored the driving conditions and resources within climate, habitat and soil variables considering their pure and shared proportions in explaining species diversity and ecosystem functions. For that purpose, we used Variance Partitioning (VP) implemented in the ‘modEvA’ R package90 in combination with GAMs. All environmental variable combinations from single to three predictors were considered, resulting in 7 different GAMs (3 models with single predictors, three models with two predictors and one model with three predictors). Model parameters have been fixed as in the model using all three climate, habitat, and soil predictors to enhance model comparability.
Extended data
See Extended Data Figs. E1, E2 and Tables E1, E2, E3, E4 and E5.
Effects of the geodiversity index on species diversity and ecosystem functions. The index of geodiversity is defined as the sum of Shannon diversity of three selected environmental predictors considering climate, habitat, and soil (see Supplementary Fig. S1). For each sampling plot, fitted (x-axis) versus observed (y-axis) values of species diversity of four taxa (trees, testate amoebae, ants, and birds; top) and related ecosystems functions (C-sequestration, decomposition, predation, and seed dispersal; bottom) are represented derived from generalized additive models (GAMs). The dashed line indicates the 1:1 line. The goodness of fit is depicted by the adjusted R2 and the explained deviance. Legend is shown in Fig. 2. The plant and animal images used in the figure were provided by Cordula Mann and Christiane Enderle.
Effects of three selected environmental variables on taxon Shannon diversity and ecosystem functions. The three predictors account for environmental conditions and resources of the three groups climate, habitat, and soil. Further explanation is given in Extended Data Fig. E1 and Fig. 2. The plant and animal images used in the figure were provided by Cordula Mann and Christiane Enderle.
Data availability
Data on species abundance of taxonomic groups and ecosystem functions will be made available in the data warehouse91 under www.TropicalMountainForest.org.
Code availability
The R code and the datasets generated and analysed within the current study are available from the corresponding authors on reasonable request.
References
Muellner-Riehl, A. N. et al. Origins of global mountain plant biodiversity: Testing the ‘mountain-geobiodiversity hypothesis’. J. Biogeogr. 46, 2826–2838 (2019).
Antonelli, A. et al. Geological and climatic influences on mountain biodiversity. Nat. Geosci. 11, 718–725 (2018).
Schrodt, F. et al. Opinion: To advance sustainable stewardship, we must document not only biodiversity but geodiversity. Proc. Natl. Acad. Sci. 116, 16155–16158 (2019).
Alahuhta, J. et al. The role of geodiversity in providing ecosystem services at broad scales. Ecol. Indic. 91, 47–56 (2018).
Read, Q. D. et al. Beyond counts and averages: Relating geodiversity to dimensions of biodiversity. Glob. Ecol. Biogeogr. 29, 696–710 (2020).
Díaz, S. et al. Pervasive human-driven decline of life on Earth points to the need for transformative change. Science 366, eaax3100 (2019).
Alahuhta, J., Toivanen, M. & Hjort, J. Geodiversity–biodiversity relationship needs more empirical evidence. Nat. Ecol. Evol. 4, 2–3 (2020).
Boothroyd, A. & McHenry, M. Old processes, new movements: the inclusion of geodiversity in biological and ecological discourse. Diversity 11, 216 (2019).
Hunter, M. L., Jacobson, G. L. & Webb, T. Paleoecology and the coarse-filter approach to maintaining biological diversity. Conserv. Biol. 2, 375–385 (1988).
Hjort, J. & Luoto, M. Can geodiversity be predicted from space?. Geomorphology 153–154, 74–80 (2012).
Benito-Calvo, A., Pérez-González, A., Magri, O. & Meza, P. Assessing regional geodiversity: the Iberian Peninsula. Earth Surf. Process. Landf. 34, 1433–1445 (2009).
dos Santos, F. M., de La Corte Bacci, D., Saad, A. R. & da Silva Ferreira, A. T. Geodiversity index weighted by multivariate statistical analysis. Appl. Geomat. 12, 361–370 (2020).
Crisp, J. R., Ellison, J. C. & Fischer, A. Current trends and future directions in quantitative geodiversity assessment. Prog. Phys. Geogr. Earth Environ. https://doi.org/10.1177/0309133320967219 (2020).
Pereira, D. I., Pereira, P., Brilha, J. & Santos, L. Geodiversity assessment of Paraná State (Brazil): An innovative approach. Environ. Manag. 52, 541–552 (2013).
Gray, M. Geodiversity and geoconservation: What, why, and how?. George Wright Forum 22, 4–12 (2005).
Ruban, D. A. Quantification of geodiversity and its loss. Proc. Geol. Assoc. 121, 326–333 (2010).
Hjort, J., Gordon, J. E., Gray, M. & Hunter, M. L. Why geodiversity matters in valuing nature’s stage: Why geodiversity matters. Conserv. Biol. 29, 630–639 (2015).
Beier, P. & Brost, B. Use of land facets to plan for climate change: Conserving the arenas, not the actors. Conserv. Biol. J. Soc. Conserv. Biol. 24, 701–710 (2010).
Anderson, M. G. & Ferree, C. E. Conserving the stage: Climate change and the geophysical underpinnings of species diversity. PLoS ONE 5, e11554 (2010).
Knudson, C., Kay, K. & Fisher, S. Appraising geodiversity and cultural diversity approaches to building resilience through conservation. Nat. Clim. Change 8, 678–685 (2018).
Turner, J. A. Geodiversity: The natural support system of ecosystems. In Landscape Planning with Ecosystem Services: Theories and Methods for Application in Europe 253–265 (eds von Haaren, C. et al.) (Springer, 2019). https://doi.org/10.1007/978-94-024-1681-7_16.
Fox, N., Graham, L. J., Eigenbrod, F., Bullock, J. M. & Parks, K. E. Incorporating geodiversity in ecosystem service decisions. Ecosyst. People 16, 151–159 (2020).
Parks, K. E. & Mulligan, M. On the relationship between a resource based measure of geodiversity and broad scale biodiversity patterns. Biodivers. Conserv. 19, 2751–2766 (2010).
Comer, P. J. et al. Incorporating geodiversity into conservation decisions: Geodiversity and conservation decisions. Conserv. Biol. 29, 692–701 (2015).
Chakraborty, A. & Gray, M. A call for mainstreaming geodiversity in nature conservation research and praxis. J. Nat. Conserv. 56, 125862 (2020).
Lawler, J. et al. The theory behind, and the challenges of, conserving nature’s stage in a time of rapid change. Conserv. Biol. 29, 618–629 (2015).
Beier, P. et al. A review of selection-based tests of abiotic surrogates for species representation. Conserv. Biol. J. Soc. Conserv. Biol. 29, 668–679 (2015).
Purvis, A. & Hector, A. Getting the Measure of Biodiversity. Nature 405, 212–219 (2000).
Moreno, C. et al. Measuring biodiversity in the Anthropocene: A simple guide to helpful methods. Biodivers. Conserv. 26, 2993–2998 (2017).
Roswell, M., Dushoff, J. & Winfree, R. A conceptual guide to measuring species diversity. Oikos 130, 321–338 (2021).
Chiarucci, A., Bacaro, G. & Scheiner, S. M. Old and new challenges in using species diversity for assessing biodiversity. Philos. Trans. R. Soc. B Biol. Sci. 366, 2426–2437 (2011).
Hooper, D. U. et al. Effects of biodiversity on ecosystem functioning: A consensus of current knowledge. Ecol. Monogr. 75, 3–35 (2005).
Hjort, J., Heikkinen, R. K. & Luoto, M. Inclusion of explicit measures of geodiversity improve biodiversity models in a boreal landscape. Biodivers. Conserv. 21, 3487–3506 (2012).
Bailey, J. J., Boyd, D. S., Hjort, J., Lavers, C. P. & Field, R. Modelling native and alien vascular plant species richness: At which scales is geodiversity most relevant?. Glob. Ecol. Biogeogr. 26, 763–776 (2017).
Zarnetske, P. L. et al. Towards connecting biodiversity and geodiversity across scales with satellite remote sensing. Glob. Ecol. Biogeogr. 28, 548–556 (2019).
Bétard, F. Patch-scale relationships between geodiversity and biodiversity in hard rock quarries: Case study from a disused quartzite quarry in NW France. Geoheritage 5, 59–71 (2013).
Tukiainen, H. et al. Spatial relationship between biodiversity and geodiversity across a gradient of land-use intensity in high-latitude landscapes. Landsc. Ecol. 32, 1049–1063 (2017).
Anderson, M. G. et al. Case studies of conservation plans that incorporate geodiversity. Conserv. Biol. 29, 680–691 (2015).
Ren, Y., Lü, Y., Hu, J. & Yin, L. Geodiversity underpins biodiversity but the relations can be complex: Implications from two biodiversity proxies. Glob. Ecol. Conserv. 31, e01830 (2021).
Homeier, J., Breckle, S.-W., Günter, S., Rollenbeck, R. T. & Leuschner, C. Tree diversity, forest structure and productivity along altitudinal and topographical gradients in a species-rich Ecuadorian montane rain forest: Ecuadorian Montane forest diversity and structure. Biotropica 42, 140–148 (2010).
Krashevska, V., Bonkowski, M., Maraun, M. & Scheu, S. Testate amoebae (protista) of an elevational gradient in the tropical mountain rain forest of Ecuador. Pedobiologia 51, 319–331 (2007).
Zhalnina, K. et al. Soil pH determines microbial diversity and composition in the park grass experiment. Microb. Ecol. 69, 395–406 (2015).
Fierer, N., Craine, J. M., McLauchlan, K. & Schimel, J. P. Litter quality and the temperature sensiticity of decomposition. Ecology 86, 320–326 (2005).
Gibb, H. et al. Climate mediates the effects of disturbance on ant assemblage structure. Proc. R. Soc. B Biol. Sci. 282, 20150418 (2015).
Sanders, N. J., Lessard, J.-P., Fitzpatrick, M. C. & Dunn, R. R. Temperature, but not productivity or geometry, predicts elevational diversity gradients in ants across spatial grains. Glob. Ecol. Biogeogr. 16, 640–649 (2007).
Paaijmans, K. P. et al. Temperature variation makes ectotherms more sensitive to climate change. Glob. Change Biol. 19, 2373–2380 (2013).
McCain, C. M. Global analysis of bird elevational diversity. Glob. Ecol. Biogeogr. 18, 346–360 (2009).
Tews, J. et al. Animal species diversity driven by habitat heterogeneity/diversity: The importance of keystone structures: Animal species diversity driven by habitat heterogeneity. J. Biogeogr. 31, 79–92 (2004).
Rahbek, C. et al. Humboldt’s enigma: What causes global patterns of mountain biodiversity?. Science 365, 1108–1113 (2019).
Hofhansl, F. et al. Climatic and edaphic controls over tropical forest diversity and vegetation carbon storage. Sci. Rep. 10, 5066 (2020).
Peters, M. K. et al. Predictors of elevational biodiversity gradients change from single taxa to the multi-taxa community level. Nat. Commun. 7, 13736 (2016).
Gagic, V. et al. Functional identity and diversity of animals predict ecosystem functioning better than species-based indices. Proc. R. Soc. B Biol. Sci. 282, 20142620 (2015).
Kraft, N. J. B., Godoy, O. & Levine, J. M. Plant functional traits and the multidimensional nature of species coexistence. Proc. Natl. Acad. Sci. 112, 797–802 (2015).
Cadotte, M. W. Functional traits explain ecosystem function through opposing mechanisms. Ecol. Lett. 20, 989–996 (2017).
Hillebrand, H. et al. Biodiversity change is uncoupled from species richness trends: Consequences for conservation and monitoring. J. Appl. Ecol. 55, 169–184 (2018).
Whittaker, R. H. Evolution and measurement of species diversity. Taxon 21, 213–251 (1972).
Socolar, J. B., Gilroy, J. J., Kunin, W. E. & Edwards, D. P. How should beta-diversity inform biodiversity conservation?. Trends Ecol. Evol. 31, 67–80 (2016).
Legendre, P. & De Cáceres, M. Beta diversity as the variance of community data: Dissimilarity coefficients and partitioning. Ecol. Lett. 16, 951–963 (2013).
Lichstein, J. W. Multiple regression on distance matrices: A multivariate spatial analysis tool. Plant Ecol. 188, 117–131 (2007).
Tuomisto, H. & Ruokolainen, K. Analyzing or explaining beta diversity? Understanding the targets of different methods of analysis. Ecology 87, 2697–2708 (2006).
Peres-Neto, P. R. & Jackson, D. A. How well do multivariate data sets match? The advantages of a Procrustean superimposition approach over the Mantel test. Oecologia 129, 169–178 (2001).
Peres-Neto, P. R., Legendre, P., Dray, S. & Borcard, D. Variation partitioning of species data matrices: Estimation and comparison of fractions. Ecology 87, 2614–2625 (2006).
Hillebrand, H. & Matthiessen, B. Biodiversity in a complex world: Consolidation and progress in functional biodiversity research: Consolidation and progress in BDEF research. Ecol. Lett. 12, 1405–1419 (2009).
Myers, N., Mittermeier, R. A., Mittermeier, C. G., da Fonseca, G. A. B. & Kent, J. Biodiversity hotspots for conservation priorities. Nature 403, 853–858 (2000).
Bendix, J. et al. A research framework for projecting ecosystem change in highly diverse tropical mountain ecosystems. Oecologia 195, 589–600 (2021).
Beck, E., Bendix, J., Kottke, I., Makeschin, F. & Mosandl, R. Gradients in a Tropical Mountain Ecosystem of Ecuador. ISBN: 978-3-540-73525-0 (Springer, 2008).
Landscape Restoration, Sustainable Use and Cross-Scale Monitoring of Biodiversity and Ecosystem Functions - A Science-directed Approach for South Ecuador (PAK823–825 Platform for Biodiversity and Ecosystem Monitoring and Research in South Ecuador, 2017).
Beck, E. et al. Ecosystem Services, Biodiversity and Environmental Change in a Tropical Mountain Ecosystem of South Ecuador. ISBN: 978-3-642-38136-2 (Springer, 2013).
Homeier, J. & Leuschner, C. Factors controlling the productivity of tropical Andean forests: Climate and soil are more important than tree diversity. Biogeosciences 18, 1525–1541 (2021).
Krashevska, V., Sandmann, D., Maraun, M. & Scheu, S. Consequences of exclusion of precipitation on microorganisms and microbial consumers in montane tropical rainforests. Oecologia 170, 1067–1076 (2012).
Krashevska, V., Sandmann, D., Maraun, M. & Scheu, S. Moderate changes in nutrient input alter tropical microbial and protist communities and belowground linkages. ISME J. 8, 1126–1134 (2014).
Tiede, Y. et al. Ants as indicators of environmental change and ecosystem processes. Ecol. Indic. 83, 527–537 (2017).
Santillán, V. et al. Spatio-temporal variation in bird assemblages is associated with fluctuations in temperature and precipitation along a tropical elevational gradient. PLoS ONE 13, e0196179 (2018).
Hsieh, T. C., Ma, K. H. & Chao, A. iNEXT: An R package for rarefaction and extrapolation of species diversity (Hill numbers). Methods Ecol. Evol. 7, 1451–1456 (2016).
Hsieh, T. C., Ma, K. H. & Chao, A. iNEXT: Interpolation and Extrapolation for Species Diversity. R package version 2.0.20, http://chao.stat.nthu.edu.tw/wordpress/software_download/ (2020).
Chao, A. et al. Rarefaction and extrapolation with Hill numbers: A framework for sampling and estimation in species diversity studies. Ecol. Monogr. 84, 45–67 (2014).
Wallis, C. I. B. et al. Modeling tropical montane forest biomass, productivity and canopy traits with multispectral remote sensing data. Remote Sens. Environ. 225, 77–92 (2019).
Keuskamp, J. A., Dingemans, B. J. J., Lehtinen, T., Sarneel, J. M. & Hefting, M. M. Tea Bag Index: A novel approach to collect uniform decomposition data across ecosystems. Methods Ecol. Evol. 4, 1070–1075 (2013).
Quitián, M. et al. Elevation-dependent effects of forest fragmentation on plant-bird interaction networks in the tropical Andes. Ecography 41, 1497–1506 (2018).
Fries, A. et al. Thermal structure of a megadiverse Andean mountain ecosystem in southern Ecuador and its regionalization. Erdkunde 63, 321–335 (2009).
Fries, A., Rollenbeck, R., Nauß, T., Peters, T. & Bendix, J. Near surface air humidity in a megadiverse Andean mountain ecosystem of southern Ecuador and its regionalization. Agric. For. Meteorol. 152, 17–30 (2012).
Zvoleff, A. glcm: calculate textures from grey-level co-occurrence matrices (GLCMs). R package version 1.6.1 (2016).
Wallis, C. I. B. et al. Remote sensing improves prediction of tropical montane species diversity but performance differs among taxa. Ecol. Indic. 83, 538–549 (2017).
Wolf, K., Veldkamp, E., Homeier, J. & Martinson, G. O. Nitrogen availability links forest productivity, soil nitrous oxide and nitric oxide fluxes of a tropical montane forest in southern Ecuador: N2 O + NO flux of tropical montane forests. Glob. Biogeochem. Cycles https://doi.org/10.1029/2010GB003876 (2011).
Fisher, W. D. On Grouping for Maximum Homogeneity. J. Am. Stat. Assoc. 53, 789–798 (1958).
Bivand, R. classInt: Choose Univariate Class Intervals (2020).
Oksanen, J. et al. vegan: Community Ecology Package (2020).
vegan: Community Ecology Package. https://CRAN.R-project.org/package=vegan.
Wood, S. N. Generalized Additive Models: An Introduction with R (Chapman and Hall/CRC, 2017).
Barbosa, A. M., Real, R., Munoz, A. R. & Brown, J. A. New measures for assessing model equilibrium and prediction mismatch in species distribution models. Divers. Distrib. 19, 1333–1338 (2013).
Lotz, T., Nieschulze, J., Bendix, J., Dobbermann, M. & König-Ries, B. Diverse or uniform? Intercomparison of two major German project databases for interdisciplinary collaborative functional biodiversity research. Ecol. Inform. 8, 10–19 (2012).
Göttlicher, D. et al. Land-cover classification in the Andes of southern Ecuador using Landsat ETM+ data as a basis for SVAT modelling. Int. J. Remote Sens. 30, 1867–1886 (2009).
Deng, Y., Wilson, J. P. & DEM Bauer, B. O. resolution dependencies of terrain attributes across a landscape. Int. J. Geogr. Inf. Sci. 21, 187–213 (2007).
Weiss, M. & Baret, F. S2ToolBox Level 2 products: LAI, FAPAR, FCOVER Version 1.1. in S2 Toolbox Level 2 Product algorithms v1.1 53.
Unger, M., Homeier, J. & Leuschner, C. Relationships among leaf area index, below-canopy light availability and tree diversity along a transect from tropical lowland to montane forests in NE Ecuador. Trop. Ecol. 54, 33–45 (2013).
Krashevska, V., Maraun, M. & Scheu, S. Micro- and macroscale changes in density and diversity of Testate amoebae of tropical montane rain forests of southern Ecuador. Acta Protozool. 49, 17–28 (2010).
Acknowledgements
We thank V. Krashevska, M. Quitián, V. Santillán, J. Schlautmann, N. Cumbicus and numerous interns for field sampling and Mark Vellend for constructive criticism on the manuscript. We also thank two anonymous reviewers for their thoughtful comments and efforts towards improving our manuscript. This work was funded by the German Research Foundation in the scope of the research units FOR402, 816 and 2730 as well as the knowledge transfer program PAK823-825. We thank the Ministerio del Ambiente y Aqua and the Instituto Nacional de Biodiversidad de Ecuador (INABIO) for granting research permits and the foundation “Nature and Culture International” for providing research facilities.
Funding
Open Access funding enabled and organized by Projekt DEAL.
Author information
Authors and Affiliations
Contributions
J.B., N.F., Y.T. and C.W. conceptualized the idea of this manuscript. J.H., K.M., Y.T., C.W. collected data. Y.T. and C.W. prepared the ecological data and C.W. prepared the environmental data and ran the models and analyses with notable contributions of Y.T., N.F. and J.B. J.B., N.F., Y.T. and C.W. wrote the first manuscript draft and finalized the manuscript. J.B., N.F., K.B.-G., R.B., D.D., A.F., J.H., C.L., M.M., K.M, E.L.N., S.S., M.S., B.T. made substantial contributions collecting the underlaying data. E.B., K.B.-G., R.B., J.H., E.L.N., C.L., M.S., S.S. contributed to the conceptual development of the work. C.E., D.I., J.S. and all other authors commented on the manuscript.
Corresponding author
Ethics declarations
Competing interests
The authors declare no competing interests.
Additional information
Publisher's note
Springer Nature remains neutral with regard to jurisdictional claims in published maps and institutional affiliations.
Supplementary Information
Rights and permissions
Open Access This article is licensed under a Creative Commons Attribution 4.0 International License, which permits use, sharing, adaptation, distribution and reproduction in any medium or format, as long as you give appropriate credit to the original author(s) and the source, provide a link to the Creative Commons licence, and indicate if changes were made. The images or other third party material in this article are included in the article's Creative Commons licence, unless indicated otherwise in a credit line to the material. If material is not included in the article's Creative Commons licence and your intended use is not permitted by statutory regulation or exceeds the permitted use, you will need to obtain permission directly from the copyright holder. To view a copy of this licence, visit http://creativecommons.org/licenses/by/4.0/.
About this article
Cite this article
Wallis, C.I.B., Tiede, Y.C., Beck, E. et al. Biodiversity and ecosystem functions depend on environmental conditions and resources rather than the geodiversity of a tropical biodiversity hotspot. Sci Rep 11, 24530 (2021). https://doi.org/10.1038/s41598-021-03488-1
Received:
Accepted:
Published:
DOI: https://doi.org/10.1038/s41598-021-03488-1
This article is cited by
-
Ecosystem type might mask the effect of ecosystem recovery on parasitoids’ biodiversity quality
Biodiversity and Conservation (2023)
Comments
By submitting a comment you agree to abide by our Terms and Community Guidelines. If you find something abusive or that does not comply with our terms or guidelines please flag it as inappropriate.