Key Points
-
Electronic health record (EHR) systems are increasingly being implemented all over the world, but represent a vast, underused data resource for biomedical research.
-
Structured EHR data, such as encoded diagnosis and medication information, are the easiest data sources to process, but advances in text-mining methods has made it possible to also use the narrative parts of patient records.
-
Statistical studies of the distribution and co-occurrence of clinical features in large collections of patient records enables identification of correlations between, for example, diseases (comorbidities) or between medications and adverse drug reactions.
-
Knowledge-discovery and machine-learning methods can be used both for discovering novel patterns in patient data and for classification and predictive purposes, such as outcome or risk assessment. This has the potential to extend current EHR decision support systems, which integrate available patient data with clinical guidelines to provide assistance to the physician at the point of care.
-
Research platforms built on EHR data, alone or coupled to genotype data, provide an inexpensive and timely way to sample relevant case and control cohorts based on relevant clinical features. As EHR and DNA databases become increasingly interlinked, genotype–phenotype association studies may be designed and conducted by re-using existing data.
-
The growing political focus on the adoption of EHR systems must be accompanied by funding and strategic research into data standards, interoperability and security. Legal matters such as data ownership, privacy and consent need to be addressed to find the right balance between public demands for autonomy and privacy, and manageable procedures for researchers to access data.
-
Fulfilling the full potential of electronic health data for scientific discovery and improved public health will require collaboration across stakeholders and research groups.
Abstract
Clinical data describing the phenotypes and treatment of patients represents an underused data source that has much greater research potential than is currently realized. Mining of electronic health records (EHRs) has the potential for establishing new patient-stratification principles and for revealing unknown disease correlations. Integrating EHR data with genetic data will also give a finer understanding of genotype–phenotype relationships. However, a broad range of ethical, legal and technical reasons currently hinder the systematic deposition of these data in EHRs and their mining. Here, we consider the potential for furthering medical research and clinical care using EHR data and the challenges that must be overcome before this is a reality.
This is a preview of subscription content, access via your institution
Access options
Subscribe to this journal
Receive 12 print issues and online access
$189.00 per year
only $15.75 per issue
Buy this article
- Purchase on Springer Link
- Instant access to full article PDF
Prices may be subject to local taxes which are calculated during checkout
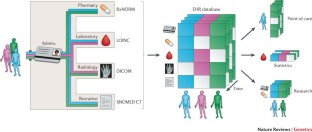

Similar content being viewed by others
References
Stewart, W. F., Shah, N. R., Selna, M. J., Paulus, R. A. & Walker, J. M. Bridging the inferential gap: the electronic health record and clinical evidence. Health Aff. 26, w181–w191 (2007).
Hillestad, R. et al. Can electronic medical record systems transform health care? Potential health benefits, savings, and costs. Health Aff. 24, 1103–1117 (2005).
Prokosch, H.-U. & Ganslandt, T. Perspectives for medical informatics. Reusing the electronic medical record for clinical research. Methods Inf. Med. 1, 38–44 (2009).
Kohane, I. S. Using electronic health records to drive discovery in disease genomics. Nature Rev. Genet. 12, 417–428 (2011).
Kush, R. D., Helton, E., Rockhold, F. W. & Hardison, C. D. Electronic health records, medical research, and the Tower of Babel. N. Eng. J. Med. 358, 1738–1740 (2008).
Taylor, P. When consent gets in the way. Nature 456, 32–33 (2008).
Himmelstein, D. U., Wright, A. & Woolhandler, S. Hospital computing and the costs and quality of care: a national study. Am. J. Med. 123, 40–46 (2010).
Buntin, M. B., Burke, M. F., Hoaglin, M. C. & Blumenthal, D. The benefits of health information technology: a review of the recent literature shows predominantly positive results. Health Aff. 30, 464–471 (2011).
Sarkar, I. N. Biomedical informatics and translational medicine. J. Transl. Med. 8, 22 (2010).
Blumenthal, D. Launching HITECH. N. Eng. J. Med. 362, 382–385 (2010).
Hunter, J. The Innovative Medicines Initiative: a pre-competitive initiative to enhance the biomedical science base of Europe to expedite the development of new medicines for patients. Drug Discov. Today 13, 371–373 (2008).
Coiera, E. Building a National Health IT System from the middle out. J. Am. Med. Inform. Assoc. 16, 271–273 (2009).
Morrison, Z., Robertson, A., Cresswell, K., Crowe, S. & Sheikh, A. Understanding contrasting approaches to nationwide implementations of electronic health record systems: England, the USA and Australia. J. Healthc. Engin. 2, 25–41 (2010).
Jha, A. K., DesRoches, C. M., Kralovec, P. D. & Joshi, M. S. A progress report on electronic health records in US hospitals. Health Aff. 29, 1951–1957 (2010).
Serdén, L., Lindqvist, R. & Rosén, M. Have DRG-based prospective payment systems influenced the number of secondary diagnoses in health care administrative data? Health Policy 65, 101–107 (2003).
Thygesen, L. C., Daasnes, C., Thaulow, I. & Bronnum-Hansen, H. Introduction to Danish (nationwide) registers on health and social issues: structure, access, legislation, and archiving. Scand. J. Public Health 39, 12–16 (2011). An overview of Danish health and socio-economic registries and research possibilities as an example of extensive population-wide registration.
Frank, L. When an entire country is a cohort. Science 287, 2398–2399 (2000).
Øyen, N. et al. Recurrence of congenital heart defects in families. Circulation 120, 295–301 (2009).
Masutani, Y., MacMahon, H. & Doi, K. Computerized detection of pulmonary embolism in spiral CT angiography based on volumetric image analysis. IEEE Trans. Med. Imaging. 21, 1517–1523 (2002).
Hoffman, M. The genome-enabled electronic medical record. J. Biomed. Inform. 40, 44–46 (2007).
Sax, U. & Schmidt, S. Integration of genomic data in Electronic Health Records — opportunities and dilemmas. Methods Inform. Med. 44, 546–550 (2005).
Meystre, S. M., Savova, G. K., Kipper-Schuler, K. C. & Hurdle, J. F. Extracting information from textual documents in the electronic health record: a review of recent research. Yearb. Med. Inform. 2008, 128–144 (2008). An introduction to NLP and information extraction in the challenging clinical context, which also reviews the relevant research in the field.
Rosenbloom, S. T. et al. Data from clinical notes: a perspective on the tension between structure and flexible documentation. J. Am. Med. Inform. Assoc. 8, 181–186 (2011). A summary of the conflicting views on structured and narrative health data in the context of how to produce valuable and reusable data.
Johnson, S. B. et al. An electronic health record based on structured narrative. J. Am. Med. Inform. Assoc. 15, 54–65 (2008).
The International Health Terminology Standards Development Organisation. Systematized Nomenclature of Medicine-Clinical Terms (SNOMED CT). [online]
Bodenreider, O. The Unified Medical Language System (UMLS): integrating biomedical terminology. Nucleic Acids Res. 32, D267–D270 (2004).
Savova, G. K. et al. Mayo clinical Text Analysis and Knowledge Extraction System (cTAKES): architecture, component evaluation and applications. J. Am. Med. Inform. Assoc. 17, 507–513 (2010).
Zeng, Q. T. et al. Extracting principal diagnosis, co-morbidity and smoking status for asthma research: evaluation of a natural language processing system. BMC Med. Inform. Decis. Mak. 6, 30 (2006).
Friedman, C., Alderson, P. O., Austin, J. H. M., Cimino, J. J. & Johnson, S. B. A general natural-language text processor for clinical radiology. J. Am. Med. Inform. Assoc. 1, 161–174 (1994).
Friedman, C., Shagina, L., Lussier, Y. & Hripcsak, G. Automated encoding of clinical documents based on natural language processing. J. Am. Med. Inform. Assoc. 11, 392–402 (2004).
Xu, H. et al. MedEx: a medication information extraction system for clinical narratives. J. Am. Med. Inform. Assoc. 17, 19–24 (2010).
Ohno-Machado, L. Realizing the full potential of electronic health records: the role of natural language processing. J. Am. Med. Inform. Assoc. 18, 539 (2011).
Evans, R. S. et al. A computer-assisted management program for antibiotics and other antiinfective agents. N. Eng. J. Med. 338, 232–238 (1998).
Demner-Fushman, D., Chapman, W. W. & McDonald, C. J. What can natural language processing do for clinical decision support? J. Biomed. Inform. 42, 760–772 (2009).
Bellazzi, R. & Zupan, B. Predictive data mining in clinical medicine: current issues and guidelines. Int. J. Med. Inform. 77, 81–97 (2008). A review of the use of predictive methods in medicine with a special focus on temporal data.
Bellazzi, R., Ferrazzi, F. & Sacchi, L. Predictive data mining in clinical medicine: a focus on selected methods and applications. WIREs Data Mining Knowl. Discov. 1, 416–430 (2011).
Lavrac, N. Selected techniques for data mining in medicine. Artif. Intell. Med. 16, 3–23 (1999).
Degroot, V., Beckerman, H., Lankhorst, G. & Bouter, L. How to measure comorbidity. A critical review of available methods. J. Clin. Epidemiol. 56, 221–229 (2003).
Hanauer, D., Rhodes, D. R. & Chinnaiyan, A. M. Exploring clinical associations using “-omics” based enrichment analyses. PLoS ONE 4, e5203 (2009).
Roque, F. S. et al. Using electronic patient records to discover disease correlations and stratify patient cohorts. PLoS Comput. Biol. 7, e1002141 (2011). Patient stratification and discovery of disease comorbidities and their causes at the molecular level using structured data and text mining on a psychiatric cohort.
Holmes, A. B. et al. Discovering disease associations by integrating electronic clinical data and medical literature. PLoS ONE 6, e21132 (2011).
Hidalgo, C., Blumm, N., Barabási, A.-L. & Christakis, N. A dynamic network approach for the study of human phenotypes. PLoS Comput. Biol. 5, e1000353 (2009).
Gibbons, R. D. et al. Post-approval drug safety surveillance. Annu. Rev. Public Health 2010, 419–437 (2010).
Lopez-Gonzalez, E., Herdeiro, M. T. & Figueiras, A. Determinants of under-reporting of adverse drug reactions: a systematic review. Drug Saf. 32, 19–31 (2009).
Wang, X., Hripcsak, G., Markatou, M. & Friedman, C. Active computerized pharmacovigilance using natural language processing, statistics, and electronic health records: a feasibility study. J. Am. Med. Inform. Assoc. 16, 328–337 (2009). An example of how text mining of bulk EHR data can be used to uncover statistical correlations between clinical concepts, specifically between medications and ADEs.
Gini, R., Herings, R., Coloma, P. M., Schuemie, M. J. & Trifiro, G. Combining electronic healthcare databases in Europe to allow for large-scale drug safety monitoring : the EU-ADR Project. Pharmacoepidemiol. Drug Saf. 20, 1–11 (2011).
Yao, L., Zhang, Y., Li, Y., Sanseau, P. & Agarwal, P. Electronic health records: implications for drug discovery. Drug Discov. Today 16, 594–599 (2011).
Mullins, I. M. et al. Data mining and clinical data repositories: Insights from a 667,000 patient data set. Comput. Biol. Med. 36, 1351–1377 (2006).
Wright, A., Chen, E. S. & Maloney, F. L. An automated technique for identifying associations between medications, laboratory results and problems. J. Biomed. Inform. 43, 891–901 (2010).
Harpaz, R., Chase, H. S. & Friedman, C. Mining multi-item drug adverse effect associations in spontaneous reporting systems. BMC Bioinformatics 11 (Suppl. 9), S7 (2010).
Swanson, D. R. Fish oil, Raynaud's syndrome, and undiscovered public knowledge. Perspect. Biol. Med. 30, 7–18 (1986).
Tsuruoka, Y., Miwa, M., Hamamoto, K., Tsujii, J. & Ananiadou, S. Discovering and visualizing indirect associations between biomedical concepts. Bioinformatics 27, 111–119 (2011).
Oztekin, A., Delen, D. & Kong, Z. J. Predicting the graft survival for heart-lung transplantation patients: an integrated data mining methodology. Int. J. Med. Inform. 78, e84–e96 (2009).
Delen, D., Walker, G. & Kadam, A. Predicting breast cancer survivability: a comparison of three data mining methods. Artif. Intell. Med. 34, 113–127 (2005).
Kurt, I., Ture, M. & Kurum, A. T. Comparing performances of logistic regression, classification and regression tree, and neural networks for predicting coronary artery disease. Expert Syst. Appl. 34, 366–374 (2008).
Valentino-Devries, J. May the best algorithm win. The Wall Street Journal [online], (2011).
Ohlsson, M., Peterson, C. & Dictor, M. Using hidden Markov models to characterize disease trajectories. Proc. Neural Networks and Expert Systems in Medicine and Healthcare Conference 2001, 324–326 (2001).
Chen, L. L., Blumm, N., Christakis, N. A., Barabási, A.-L. & Deisboeck, T. S. Cancer metastasis networks and the prediction of progression patterns. Br. J. Cancer 101, 749–758 (2009).
Fu, T.-C. A review on time series data mining. Eng. Appl. Artif. Intell. 24, 164–181 (2011).
Cao, H., Melton, G. B., Markatou, M. & Hripcsak, G. Use abstracted patient-specific features to assist an information-theoretic measurement to assess similarity between medical cases. J. Biomed. Inform. 41, 882–888 (2008).
Melton, G. B. et al. Inter-patient distance metrics using SNOMED CT defining relationships. J. Biomed. Inform. 39, 697–705 (2006).
Murphy, S. et al. Instrumenting the health care enterprise for discovery research in the genomic era. Genome Res. 19, 1675–1681 (2009).
Murphy, S. N. et al. Serving the enterprise and beyond with informatics for integrating biology and the bedside (i2b2). J. Am. Med. Inform. Assoc. 17, 124–130 (2010). A thorough description of the architecture and capabilities of the i2b2 research platform for biomedical research based on EHR data.
McCarty, C. A. et al. The eMERGE Network: A consortium of biorepositories linked to electronic medical records data for conducting genomic studies. BMC Med. Genomics 4, 13 (2011).
Kho, A. N. et al. Electronic medical records for genetic research: results of the eMERGE consortium. Science Transl. Med. 3, 79re1 (2011).
Schildcrout, J. S. et al. An analytical approach to characterize morbidity profile dissimilarity between distinct cohorts using electronic medical records. J. Biomed. Inform. 43, 914–923 (2010).
Kurreeman, F. et al. Genetic basis of autoantibody positive and negative rheumatoid arthritis risk in a multi-ethnic cohort derived from electronic health records. Am. J. Hum. Genet. 88, 57–69 (2011). The i2b2 platform put to use for case–control generation and study design based on EHR and DNA data in a rheumatoid arthritis project.
Kullo, I. J. et al. Complement receptor 1 gene variants are associated with erythrocyte sedimentation rate. Am. J. Hum. Genet. 89, 131–138 (2011).
Kullo, I. J., Ding, K., Jouni, H., Smith, C. Y. & Chute, C. G. A genome-wide association study of red blood cell traits using the electronic medical record. PLoS ONE 5, 9 (2010).
Denny, J. C. et al. Variants near FOXE1 are associated with hypothyroidism and other thyroid conditions: using electronic medical records for genome- and phenome-wide studies. Am. J. Hum. Genet. 89, 529–542 (2011).
Ritchie, M. D. et al. Robust replication of genotype-phenotype associations across multiple diseases in an electronic medical record. Am. J. Hum. Genet. 86, 560–572 (2010).
Perlis, R. H. et al. Using electronic medical records to enable large-scale studies in psychiatry: treatment resistant depression as a model. Psychol. Med. 42, 41–50 (2012).
Kho, A. N. et al. Use of diverse electronic medical record systems to identify genetic risk for type 2 diabetes within a genome-wide association study. J. Am. Med. Inform. Assoc. 19, 212–218 (2011).
Himes, B. E., Dai, Y., Kohane, I. S., Weiss, S. T. & Ramoni, M. F. Prediction of chronic obstructive pulmonary disease (COPD) in asthma patients using electronic medical records. J. Am. Med. Inform. Assoc. 16, 371–379 (2009).
Roden, D. M. et al. Development of a large-scale de-identified DNA biobank to enable personalized medicine. Clin. Pharmacol. Ther. 84, 362–369 (2008). A description of the technical, scientific and legal aspects of the development of an EHR–DNA linked research database with an opt-out consent model.
Denny, J. C. et al. PheWAS: demonstrating the feasibility of a phenome-wide scan to discover gene-disease associations. Bioinformatics 26, 1205–1210 (2010). A demonstration of how EHR data linked with DNA data can be used in a reversal of the normal GWAS approach to search for disease phenotypes associated with SNPs.
Wilke, R. et al. The emerging role of electronic medical records in pharmacogenomics. Clin. Pharmacol. Ther. 89, 379–386 (2011).
Al Mallah, A., Guelpa, P., Marsh, S. & van Rooij, T. Integrating genomic-based clinical decision support into electronic health records. Personalized Med. 7, 163–170 (2010).
McCarty, C. A. & Wilke, R. A. Biobanking and pharmacogenomics. Pharmacogenomics 11, 637–641 (2010).
Schwarz, U. I. et al. Genetic determinants of response to warfarin during initial anticoagulation. N. Eng. J. Med. 358, 999–1008 (2008).
Onitilo, A. et al. Estrogen receptor genotype is associated with risk of venous thromboembolism during tamoxifen therapy. Breast Cancer Res. Treat. 115, 643–650 (2009).
Lage, K. et al. Dissecting spatio-temporal protein networks driving human heart development and related disorders. Mol. Syst. Biol. 6, 1–9 (2010).
Greenblum, S., Turnbaugh, P. J. & Borenstein, E. Metagenomic systems biology of the human gut microbiome reveals topological shifts associated with obesity and inflammatory bowel disease. Proc. Natl Acad. Sci. USA. 109, 594–599 (2012).
Rzhetsky, A., Wajngurt, D., Park, N. & Zheng, T. Probing genetic overlap among complex human phenotypes. Proc. Natl Acad. Sci. USA. 104, 11694–11699 (2007).
Goh, K.-I. et al. The human disease network. Proc. Natl Acad. Sci. USA. 104, 8685–8690 (2007).
Park, J., Lee, D.-S., Christakis, N. A. & Barabási, A.-L. The impact of cellular networks on disease comorbidity. Mol. Syst. Biol. 5, 262 (2009).
Ashley, E. A. et al. Clinical assessment incorporating a personal genome. Lancet 375, 1525–1535 (2010).
Hood, L., Heath, J. R., Phelps, M. E. & Lin, B. Systems biology and new technologies enable predictive and preventative medicine. Science 306, 640–643 (2004).
Galas, D. J. & Hood, L. Systems biology and emerging technologies will catalyze the transition from reactive medicine to predictive, personalized, preventive and participatory (P4) medicine. Interdisciplinary Bio Central 1, 6 (2009).
Hall, M. A. Property, privacy, and the pursuit of interconnected electronic medical records. Iowa Law Review 2010, 631–663 (2010).
Noble, S. et al. Feasibility and cost of obtaining informed consent for essential review of medical records in large-scale health services research. J. Health Serv. Res. Policy 14, 77–81 (2009).
Kho, M. E., Duffett, M., Willison, D. J., Cook, D. J. & Brouwers, M. C. Written informed consent and selection bias in observational studies using medical records: systematic review. BMJ 338, 1–8 (2009).
Hoffman, S. Balancing privacy, autonomy, and scientific needs in electronic health records research. Case Research Paper Series in Legal Studies [online], (2011). An extensive summary of legal and ethical issues encountered in health research and their potential consequences for conducting scientific research.
Meystre, S. M., Friedlin, F. J., South, B. R., Shen, S. & Samore, M. H. Automatic de-identification of textual documents in the electronic health record: a review of recent research. BMC Med. Res. Methodol. 10, 1–16 (2010).
Benitez, K. & Malin, B. Evaluating re-identification risks with respect to the HIPAA privacy rule. J. Am. Med. Inform. Assoc. 17, 169–177 (2010).
Heeney, C., Hawkins, N., de Vries, J., Boddington, P. & Kaye, J. Assessing the privacy risks of data sharing in genomics. Public Health Genomics 14, 17–25 (2011).
Homer, N. et al. Resolving individuals contributing trace amounts of DNA to highly complex mixtures using high-density SNP genotyping microarrays. PLoS Genet. 4, e1000167 (2008).
Malin, B. & Sweeney, L. Re-identification of DNA through an automated linkage process. Proc. AMIA Symp. 2001, 423–427 (2001).
Arumugam, M. et al. Enterotypes of the human gut microbiome. Nature 473, 174–180 (2011).
Rothstein, M. A. Is deidentification sufficient to protect health privacy in research? Am. J. Bioeth. 10, 3–11 (2010).
Begoyan, A. An overview of interoperability standards for electronic health records. In Integrated Design and Process Technology (IDPT-2007) (2007).
Goossen, W., Goossen-Baremans, A. & van der Zel, M. Detailed clinical models: a review. Healthc. Inform. Res. 16, 201–214 (2010). An introduction to modelling and representation of clinical concepts and meaning, which is important for data interoperability.
Knaup, P., Bott, O., Kohl, C., Lovis, C. & Garde, S. Electronic patient records: moving from islands and bridges towards electronic health records for continuity of care. Yearb. Med. Inform. 2007, 34–46 (2007).
Garde, S., Knaup, P., Hovenga, E. & Heard, S. Towards semantic interoperability for electronic health records. Methods Inf. Med. 46, 332–343 (2007).
Wicks, P., Vaughan, T. E., Massagli, M. P. & Heywood, J. Accelerated clinical discovery using self-reported patient data collected online and a patient-matching algorithm. Nature Biotech. 29, 411–414 (2011).
Aronson, R. Effective mapping of biomedical text to the UMLS Metathesaurus: the MetaMap program. Proc. AMIA Symp. 2001, 17–21 (2001).
Uzuner, O., Goldstein, I., Luo, Y. & Kohane, I. Identifying patient smoking status from medical discharge records. J. Am. Med. Inform. Assoc. 15, 14–24 (2008).
Uzuner, O. Recognizing obesity and comorbidities in sparse data. J. Am. Med. Inform. Assoc. 16, 561–570 (2009).
Uzuner, O., Solti, I. & Cadag, E. Extracting medication information from clinical text. J. Am. Med. Inform. Assoc. 17, 514–518 (2010).
Uzuner, O., South, B. R., Shen, S. & Duvall, S. L. 2010 i2b2/VA challenge on concepts, assertions, and relations in clinical text. J. Am. Med. Inform. Assoc. 18, 552–557 (2011).
Fung, K. W., McDonald, C. & Bray, B. E. RxTerms - a drug interface terminology derived from RxNorm. Proc. AMIA Symp. 2008, 227–231 (2008).
Steindel, S. J. International classification of diseases, 10th edition, clinical modification and procedure coding system: descriptive overview of the next generation HIPAA code sets. J. Am. Med. Inform. Assoc. 17, 274–282 (2010).
Acknowledgements
We thank U. Buddrus for kindly providing unpublished data on the adoption of EHR systems in Europe. Any errors in communicating these insights are the sole responsibility of the authors. The authors were supported in part by the Villum Kann Rasmussen Foundation, the Novo Nordisk Foundation and the Danish Research Council for Strategic Research.
Author information
Authors and Affiliations
Corresponding author
Ethics declarations
Competing interests
The authors declare no competing financial interests.
Glossary
- Clinical decision support
-
(CDS). Software systems providing support for decision making to physicians through the application of health knowledge and logical rules to patient data.
- Biobanks
-
Central repositories of biological material that are mainly used for research. They facilitate the re-use of collected samples in different research projects.
- Electronic health records
-
(EHRs). In this review we do not distinguish between EHRs, Electronic Patient Records (EPRs) and Electronic Medical Records (EMRs).
- HITECH act
-
Part of the American Recovery and Reinvestment act from 2009. The Health Information Technology for Economic and Clinical Health (HITECH) act allocates funding and attention to HIT infrastructure and electronic health record adoption and research in the United States.
- ICD
-
The International Classification of Diseases (ICD) published by the World Health Organization. It has been translated into numerous languages.
- Medicare
-
A US government health insurance programme primarily covering people aged 65 yearsand older.
- Charlson index
-
A measure of the accumulated disease burden for a patient. It is calculated as a weighted sum of 22 selected medical conditions that are assigned scores depending on the severity of the condition.
- Pharmacovigilance
-
Monitoring of adverse drug events during clinical trials and after marketing in order to prevent harm to patients. It is typically based on statistical pattern-finding in databases of reported adverse events.
- Adverse drug event
-
(ADE). Used in pharmacology to describe any unexpected or harmful event associated with a given medication.
- Feature vector
-
The representation of objects (patients) as vectors in the space of all relevant features. Each dimension of the vector specifies the association of a patient with a certain feature.
- Clustering
-
A common task in statistical data exploration using measures of similarity between data points, network topology or other methods to group data points with similar characteristics together in clusters.
- Semantic similarity
-
A measure of the similarity of two concepts in terms of their meaning or semantic content. Often quantified using topological measures of distance in an ontology of concepts, such as WordNet or Systematized Nomenclature of Medicine — Clinical Terms (SNOMED CT).
- Electronic Medical Records and Genomics Network
-
(eMERGE Network). An institutional network that is exploring the potential of electronic health record data in genetic and medical research. Participating institutions are: GroupHealth, Geisinger, Marshfield Clinic, Mayo Clinic, Mount Sinai School of Medicine, Northwestern University and Vanderbilt University.
- Pharmacogenomics
-
The study of how genetic variants influence the effects of drugs on, for example, drug metabolism, efficacy and toxicity, with the goal of improving and personalizing drug therapy.
- Million Veteran Program
-
A research project initiated by the Veterans Affairs Office of Research and Development that is aimed at establishing a database with DNA and health record data from one million people. Participation is opt-in.
- Kaiser RPGEH
-
The Kaiser Permanente Research Project on Genes, Environment and Health (Kaiser RPGEH). This project aims to establish a research database with genetic data, environment data and health record data from 500,000 people. Participation is opt-in.
Rights and permissions
About this article
Cite this article
Jensen, P., Jensen, L. & Brunak, S. Mining electronic health records: towards better research applications and clinical care. Nat Rev Genet 13, 395–405 (2012). https://doi.org/10.1038/nrg3208
Published:
Issue Date:
DOI: https://doi.org/10.1038/nrg3208
This article is cited by
-
Prediction of Sjögren’s disease diagnosis using matched electronic dental-health record data
BMC Medical Informatics and Decision Making (2024)
-
Cluster analysis and visualisation of electronic health records data to identify undiagnosed patients with rare genetic diseases
Scientific Reports (2024)
-
FIT calculator: a multi-risk prediction framework for medical outcomes using cardiorespiratory fitness data
Scientific Reports (2024)
-
Systematic Review of Machine Learning Applied to the Secondary Prevention of Ischemic Stroke
Journal of Medical Systems (2024)
-
Knowledge Graph Embeddings for ICU readmission prediction
BMC Medical Informatics and Decision Making (2023)