Abstract
This study aims at identifying risk-related patterns of left ventricular contraction dynamics via novel volume transient characterization. A multicenter cohort of AMI survivors (n = 1021) who underwent Cardiac Magnetic Resonance (CMR) after infarction was considered for the study. The clinical endpoint was the 12-month rate of major adverse cardiac events (MACE, n = 73), consisting of all-cause death, reinfarction, and new congestive heart failure. Cardiac function was characterized from CMR in 3 potential directions: by (1) volume temporal transients (i.e. contraction dynamics); (2) feature tracking strain analysis (i.e. bulk tissue peak contraction); and (3) 3D shape analysis (i.e. 3D contraction morphology). A fully automated pipeline was developed to extract conventional and novel artificial-intelligence-derived metrics of cardiac contraction, and their relationship with MACE was investigated. Any of the 3 proposed directions demonstrated its additional prognostic value on top of established CMR indexes, myocardial injury markers, basic characteristics, and cardiovascular risk factors (P < 0.001). The combination of these 3 directions of enhancement towards a final CMR risk model improved MACE prediction by 13% compared to clinical baseline (0.774 (0.771—0.777) vs. 0.683 (0.681—0.685) cross-validated AUC, P < 0.001). The study evidences the contribution of the novel contraction characterization, enabled by a fully automated pipeline, to post-infarction assessment.
Similar content being viewed by others
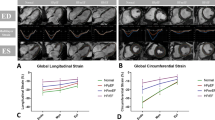
Introduction
Cardiovascular diseases are the worldwide leading cause of death, with a toll further climbing each decade1. Acute myocardial infarction (AMI) plays a major role among them, owing to its prevalence, counted in millions, yearly, and its high case-fatality2. Despite significant advances in medical treatment towards personalized and preventive medicine2,3, the mortality of AMI survivors within 6 months after infarct sits at 12%4. Early and improved risk assessment is crucial to reduce this burden.
Left ventricular (LV) remodeling is central to AMI early prognosis prediction5. Consequently, LV macro-function, quantified as LV ejection fraction (LVEF), is the preferred image biomarker for therapeutic decision‐making and clinical risk stratification, according to AMI guidelines4,6. Nonetheless, LVEF fails to capture contraction dynamics as well as functional and anatomical regional abnormalities7,8. Moreover, LVEF is mainly preserved or only moderately reduced in most AMI survivors and therefore recurrent adverse events often occur in patients at a theoretical low risk, which further evidences the need of stratification improvements9,10.
Cardiac Magnetic Resonance (CMR) imaging has proven uniquely suitable to assess morphological and functional myocardial alterations and provides prognostic information over and above LVEF11,12,13. Despite its cost and acquisition length limitations, the level of precision of CMR has established it as gold standard for quantitative assessment of the heart. Furthermore, CMR uses no ionizing radiation unlike computed tomography imaging14. LV micro-myocardial injury, assessed by late gadolinium enhancement (LGE) CMR and usually quantified as infarct size (IS) and microvascular obstruction (MVO), has emerged as a robust outcome measure in AMI risk assessment13,15. Estimation of local LV remodeling using CMR has been proposed to improve prognostic information16,17. Likewise, shape analysis of the LV to identify 3D patterns related to risk has demonstrated its additional contribution to risk assessment18. CMR myocardial feature tracking (CMR-FT) has been successfully applied for quantification of LV deformation indexes and mechanical uniformity alterations7,19, associated with hemodynamic alterations, adverse LV remodeling, and clinical outcomes9,20. In particular, the prognostic value of global longitudinal strain (GLS) by CRM-FT has proven superior and incremental to LVEF and other CMR markers of infarct severity21. Recent advances in deep learning enable the extraction of CMR-derived volume temporal transients, which have proven to be a robust and useful biomarker to characterize cardiac function22.
Building on these advances, and further automating and enhancing the descriptors of contraction, this work focuses on the ability to detect novel signatures that predict post-infarction risks from the temporal changes observed at chamber level (i.e. LV volume transients). The work additionally explores the complementary value of tissue level dynamics (i.e. strains) and, also, the static 3D detail that we have recently demonstrated to bring prognostic value in our previous work18, an analysis that is limited to the end-systolic (ES) and end-diastolic (ED) instances. Here we expand on the work in17 and complete it by applying related ideas to the characterization of CMR-FT strains and the novel use of LV volume transients for post-infarction risk assessment. We assess the prognostic contribution of this novel cardiac contraction characterization using all three image-based components (strains, transients and 3D LV patterns) on a large multicenter study, including ST‐segment–elevation myocardial infarction (STEMI) and non‐STEMI (NSTEMI) patients (see Fig. 1). The study demonstrates the potential of the herein proposed fully automated analysis for AMI risk management and identifies novel risk-related contraction patterns, expanding the understanding of post-infarction remodeling.
Comprehensive Characterization of Cardiac Contraction for Improved Post-Infarction Risk Assessment. The 3 proposed directions of enhancement of cardiac contraction characterization from CMR, enabled by a fully automated pipeline, for improved post-infarction management beyond left ventricular ejection fraction (LVEF), the established marker.
Methods
Study population
A total of 1235 AMI survivors from the AIDA-STEMI and TATORT-NSTEMI multicenter randomized trials were considered for the study23,24,25. The trials were registered with ClinicalTrials.gov (Registration ID and date: AIDA-STEMI: NCT00712101, 03/07/2008; TATORT-NSTEMI: NCT01612312, 01/06/2012) and their experimental protocols were approved by the lead ethical committee at the University of Leipzig and by all the local ethics and licensing committees of the participating sites (Zentralklinik Bad Berka, Unfallkrankenhaus Berlin, Klinikum Frankfurt/Oder, University of Saarland, Institut für Herzinfarktforschung, University of Tübingen, Herz- und Gefäß-KLinik Bad Neustadt, Herz und Diabeteszentrum Bad Oeynhausen, Klinikum Links der Weser Bremen, Klinikum Coburg, Carl-von-Basedow-Klinikum Merseburg, Klinikum Pirna, Krankenhaus der Barmherzigen Brüder, Jochen Wöhrle and Klinikum der Stadt Villingen-Schwenningen). This size of the study is justified in Supplemental Material VIII, based on26. Reperfusion therapy with primary percutaneous coronary intervention and postinfarction medical treatment were supplied according to state-of-the-art guideline recommendations4. The study was conducted according to the Declaration of Helsinki and written informed consent was obtained from all subjects and/or their legal guardian for study participation. The data that supports the findings is available upon reasonable request.
CMR imaging protocol
The patients underwent CMR imaging on 1.5T (Siemens models: Aera, Avanto, Espree, Sonata, SonataVision and Symphony; Philips models: Achieva and Intera; GE models: Signa excite) or 3T (Siemens Verio) clinical scanners within 10 days after infarct following a standardized protocol6,23,24, that includes ECG-gated balanced steady-state free precession sequences (TR = 3.573 ms; TE median of 1.786 (1.649—1.786) ms; Flip angle = 60°) and T1-weighted LGE images. All sequences were acquired in horizontal and vertical long-axis views as well as continuous short-axis (SAx) stacks capturing the whole LV (Pixel size: 1.25 (1.25—1.48) mm; Spacing between SAx: 8.00 (8.00–8.00) mm). Ventricular volumes and infarct characteristics were determined in sequential SAx by blinded clinicians6,24, via dedicated software cmr4227. Standard thresholding techniques were applied to assess IS and MVO, as explained in6,24.
Study endpoints
The predefined clinical endpoint of the study was the 12-month rate of major adverse cardiac events (MACE), consisting of new congestive heart failure, reinfarction and all-cause death, as detailed in23,24,25. In case of multiple MACE only one contribution per patient to the composite of endpoints was considered (death > reinfarction > congestive heart failure). The events were adjudicated by a blinded clinical committee based on the data collected in the study sites.
CMR-FT strain analysis (strain)
CMR-FT was conducted at the University Medical Centre Göttingen core laboratory28, using certified software 2D Cardiac Performance Analysis MR29. Final values were based on the average of 3 independent analyses30. Circumferential and radial strains were determined at basal, midventricular, and apical locations, as described in9,20. Longitudinal strains were derived at both 2- and 4-chamber long-axis views. Global longitudinal, circumferential, and radial strains (GLS, GCS and GRS, respectively) as well as circumferential and radial uniformity ratio estimates (CURE and RURE), were reported31. CURE and RURE range between 0, complete nonuniform contraction, and 1, perfect uniformity, that is, equal strain across the myocardium at any given time point. Calculation details can be found in9.
LV fully automated volume transients analysis
The process, illustrated in Fig. 2, is fully automated and consist of the following steps: (1) LV myocardium segmentation from SAx images and LV volume temporal transient reconstruction from the cavity volume integration along the cardiac cycle; (2) Computation of conventional metrics from LV volume transient; (3) Definition of Artificial Intelligence (AI) based metrics of LV volume transients, by unsupervised construction of statistical models and the subsequent selection of the features with best prognostic value.
Automated volume transient analysis. LV fully automated volume transient analysis pipeline (top-left), along with patient S692 sample case, consisting of the following steps: (1.I.) the SAx stack (image I) is pre-processed normalizing for intensity, resolution and orientation and cropped to the region of interest, following the prior detection of the heart; (1.II.) the LV myocardium is fine segmented in the SAx stack (image II); (1.III) a volumetric segmentation is synthesized (image III) and the LV volume transient derived; (2) the conventional metrics of the volume transient are estimated (top-right); and (3) PCA analysis is applied on the transients to facilitate the supervise learning of MACE occurrence related features (AI-derived transient metrics). The red circle landmarks on the representative volume transient (top-right) correspond to: a, end-diastole; b, systolic peak velocity; c, end-systole; d, passive filling peak velocity; e, beginning of diastasis; f, mid-diastasis; g, end-diastasis; and h, active filling peak velocity. This figure is available in video format (see Supplementary Video 1).
LV segmentation and volume transients estimation
A 2-step deep learning approach was applied to segment the LV cavity, based on a UNet architecture with enhanced pre-processing32,33, that reached the best performance in the 2019 LV Full Quantification Challenge33,34. Implementation details are available in Supplemental Material I. Resulting segmentations were arranged in binary volumetric images, and the LV cavity volume was derived for each frame via trapezoidal integration.
Volume transient conventional metrics (Vt)
The duration, average and peak velocity of the cardiac phases shown in Fig. 2 were derived22. The transients were resampled using splines to increase resolution and better capture the slope-sensitive metrics. Diastasis was estimated as the mid-diastolic region with a velocity inferior to 80% stroke volume (SV) per second (empirical threshold). The time to diastasis, measured from end-systole and mid-diastasis, was set as the boundary between the passive and active filling contributions. Passive and active filling metrics were not reported in those cases where diastasis was not reliably found (diastasis = 0s , n = 212). The average RR-interval and its variability, measured as highest minus lowest values, as well as the frame trigger times, were directly retrieved from the patient scans metadata.
Volume transient AI metrics (VtAI)
Principal Component Analysis (PCA) is an unsupervised machine learning technique that inspects the data to find the directions of change that maximize the variance observed in the population. The main advantages of the PCA strategy are: (I) the ability to extract interpretations from the linear space of features; (II) the unsupervised definition of features, that are unbiased to outcome prediction; (III) the ability to minimize the impact of noise and the reduced risk of overfitting by working with the principal modes of variation. Independently from the above calculations on raw volume transients, PCA was applied to the volume transients (Formulation details and a more detailed explanation is available in Supplemental Material III), minmax normalized to standardize in ventricle size and SV (see implications in Supplemental Material II). The resulting directions are the so called ‘PCA modes’, and represent patterns of change with respect to the mean volume transients (e.g. RR-interval length, passive vs. active contribution, etc.—See Fig. 3, panel headings representing mode interpretation). Thus, the volume transient of each subject can be represented by the average transient of the population plus a weighted sum of these modes of variation. The weights used are, therefore, continuous variables which have a unique value for each patient and become potential biomarkers to predict MACE. Among them, those with highest prognostic value were identified by a stepwise multivariable Fisher linear discriminant analysis (LDA)35, as detailed below, on the modes that reconstruct 95% of the total variance of the population.
AI-derived volume transient metrics. AI-derived volume transient features most relevant to MACE occurrence prediction, resulting from unsupervised learning (VtAI1, VtAI2, VtAI3 and VtAI5). The MACE (red, class 1) and No-MACE (blue, class 0) traces shown correspond to the 10th and 90th percentiles. This allows to visualize the pattern of change encoded by each of the unsupervised variables (RR-interval, passive vs active filling, etc.), as well as to describe how a representative MACE and No-MACE normalized volume transient would theoretically look like according to each of these four unsupervised variables. The P-value, re-substitution and leave-one-out AUCs are presented along each mode as MACE and No-MACE distributions, further stratified into infarct aetiology (STEMI and NSTEMI). This figure is available in video format, where the evolution from the MACE to the No MACE extremes is shown to better appreciate the pattern of change encoded in each of the VTAI contraction modes (see Supplementary Video 2).
LV fully automated 3D shape analysis (LV3D)
The analysis is explained in18. In brief, an automated pipeline was developed to automatically obtain the personalized 3D LV mesh at ES and ED; and a similar AI methodology to the explained above is applied to identify the MACE-related ES shape and 3D contraction patterns. The contraction was approximated as ED to ES displacements. Table 1 summarizes the three ES shape and three contraction descriptors found to be prognostic, along with their interpretation18. These 3D LV patterns are illustrated in Fig. SIV.1.
Prognostic value assessment
The additional prognostic contribution of each of the 3 proposed directions of enhancement, CMR-FT strains (strains), volume temporal transients (conventional and AI-derived—Vt and VtAI) and LV 3D patterns (LV3D) markers, was assessed considering only CMR biomarkers (CMR) and all the clinical variables (ALL) of the study. Table 1 lists the ‘CMR’ (e.g. MVO, IS, etc.) and ‘ALL’ (e.g. age, weight, etc.) baseline variables included in the analysis as predictor candidates. An additional analysis evaluated the prognostic value of the three proposed groups of contraction metrics in combination.
Comparisons were based on the prediction performance resulting from both a multivariable LDA (binary classification–MACE occurrence at 12 months) and a multivariable Cox analysis (time to MACE occurrence). A backward stepwise strategy (unbiased to univariable associations36) was followed in both analyses to find inter-variable synergies and address collinearity: all variables of interest (predictor candidates) were initially included, and the less significant in the model iteratively removed, until a 0.05 p‐value threshold was met by all of them. Significance in the LDA was approximated as significance in the generalized linear binomial sigmoid regression model.
The performance of the resulting configurations was assessed via area under the receiver-operator characteristic curve (AUC), for binary classifications, and concordance index (C-index)37, for time-dependent curves, to account for both specificity and sensitivity, given the MACE class imbalance. Performance in both resubstitution (apparent performance—learning and testing with the entire cohort) and cross-validation (optimism corrected performance—10 cross-fold-validation repeated for a hundred random data splits) is reported to ensure generality of findings.
Statistical analysis
The variables of the study are described according to MACE occurrence (Table 2). Continuous variables were not normally distributed in a Shapiro–Wilk test, except for the VtAI modes of variation (Gaussian distributed by definition), and are therefore presented as median and interquartile range (IQR). Distributions (variable stratifications, cross-validated AUC model results, etc.) were compared by the non-parametric Wilcoxon rank sum test. The resulting p-value of the MACE vs No MACE comparisons, as well as the univariate-LDA cross-validated performance, was reported for each variable. Univariate Cox regression analyses were likewise completed. Hazard ratios, 95% confidence intervals and the predictor significance are presented in the results. Correlation between variables was assessed via Pearson (rp) and Spearman (rs) coefficients. Patients with missing data in any of the model variables are excluded for the development of this particular model. All analyses were implemented in Matlab38, except for neural network training and inference; that were implemented in Keras with Tensorflow as backend39. The experiments and analyses were run on a standard laptop (RAM: 32Gb; GPU: 16Gb). The study follows the guidelines of transparent reporting of multivariable prediction model for individual prognosis or diagnosis (TRIPOD) as well as the Strengthening the Reporting of Observational Studies in Epidemiology (STROBE) Statement for case–control studies36,40. The relevant checklists are included in Supplemental Material VIII.
Results
Patients
In total, 1021 patients (STEMI: n = 723; NSTEMI: n = 298) of the 1235 cohort were included in the study (No CMR: n = 126; incomplete protocol: n = 86; no follow-up: n = 2). Out of them, 73 patients suffered from MACEs (congestive heart failure: n = 20; reinfarction: n = 21; death: n = 32) 23,24,25. The population was predominantly male (74.5%) with a median age of 63 (IQR: 52–72) years, LVEF of 50.5% (IQR: 43.3–57.3) and IS of 13.4% of LV mass (IQR: 5.4–21.8). Their CMR and baseline clinical characteristics have been reported and discussed previously 23,24,25 and are reproduced in Supplemental Material IV.
CMR-FT strain analysis (strain)
The CMR-FT strain results are described in Table 2. The MACE subgroup exhibited significantly lower global longitudinal, circumferential, and radial strains (P < 0.001) and a less uniform radial and circumferential contraction (P < 0.001).
Automated volume transient AI analysis
LV segmentation and volume calculation
The automated segmentation of the SAx resulted in a median Dice score of 0.971 (IQR 0.945–0.981) for the LV cavity and 0.975 (IQR 0.959–0.982) for the LV epicardium. The correlation, rs, between the volumes calculated from the automated and manual segmentations were 0.916 and 0.919, for end-systolic and end-diastolic volume (ESV and EDV), respectively.
Volume transient conventional metrics (Vt)
Results are detailed in Table 2. The MACE subpopulation was characterized by a significantly shorter and more variable RR-interval (P < 0.001). Their systolic (P = 0.038), diastolic (P = 0.025), diastasis (P = 0.037) and time to diastasis (P = 0.013) was accordingly reduced. Nevertheless, only diastasis was significantly smaller when normalizing in time (P = 0.032). The passive (P = 0.002) and active (P < 0.001) contributions to LV filling were significantly compromised in MACE patients, as ESV and EF were significant (P < 0.001—See Table SIV.1), but not when normalizing by SV. Regarding velocities, the MACE cohort presented significantly lower systolic peak (P = 0.029), and active filling average (P = 0.008) and peak (P = 0.003) velocities. However, it was the passive filling average velocity the only one significant to MACE (P = 0.013) when normalizing by SV. Fig SIV.2 shows the average MACE and No MACE volume transients normalized by SV.
Volume transient AI ANALYSIS (VtAI)
95% of the variance was explained by the first 6 unsupervised modes of variation. Among them, modes 1, 2, 3 and 5 (VtAI1, VtAI2, VtAI3 and VtAI5) were determined the most relevant to MACE by the LDA stepwise analysis. These modes are interpreted as RR-interval (VtAI1), systolic velocity (VtAI2), passive vs active filling (VtAI3) and diastasis (VtAI5) (see Discussion). Figure 3 illustrates these modes and summarizes their significance, classification performance and distributions, stratified by MACE occurrence and infarct aetiology. The correlation between these AI metrics and the conventional transient ones is summarized in Fig. 4 (Pearson) and Supplemental Material VI (Spearman and R2).
LV fully automated 3D shape analysis (LV3D)
The ES shape and 3D contraction descriptors were significant to MACE (P < 0.001) and proved superior to their corresponding stand‐alone versions ESV and LVEF18. Their statistics were reported in18, and are reproduced in Table SIV.1.
Prognostic contributions
The results of the augmented prognostic contribution of proposed biomarkers to characterize LV contraction are shown in Table 3. Both LDA and Cox multivariable analysis converged to a nearly identical selection of variables for each of the models.
Prediction of MACE occurrence via conventional and AI automated volume transient metrics, strains and 3D LV markers proved significantly superior (P < 0.001) to LVEF using either CMR-derived (CMR: 2.49%; CMR + Vt: 3.66%; CMR + VtAI: 6.3%; CMR + Strain: 5.86%; CMR + LV3D: 8.05% AUC improvement) or ALL clinical features (ALL: 6.73%; ALL + Vt: 7.91%; ALL + VtAI: 9.22%; ALL + Strain: 10.1%; ALL + LV3D: 9.37% AUC improvement), as illustrated in Fig. 5A. Similar results were obtained in Cox analyses. The additional prognostic value was larger (P < 0.001) when using AI-derived metrics vs conventional transient ones (Table 3 and Fig. 5A).
MACE prediction results and interpretation of the AUC differences. (A): MACE prediction performance results and prognostic contribution of the 3 proposed directions of enhancement. The performance of LVEF, the most established marker for AMI prognosis, is included as reference. Selection of significant variables is based on LDA multivariable stepwise analysis, and results are expressed in AUC resubstitution (rs, blue) and AUC 10-cross-fold validation (k, orange), repeated for a hundred random data splits (black distributions). All differences in performance were significant (P < 0.001). Note the remarkable improvement of the final configuration, combining the 3 proposed directions of enhancement. (B): Interpretation and implications of the AUC differences in ROC prediction curves, illustrated by the comparison between LVEF and our multivariable model, combining the 3 directions of enhancement. Given a recommended operating point of high sensitivity (80%) in the LVEF curve (point A), our model is able to predict MACE at the same sensitivity but reducing the false positive rate a 40% (point B), i. e., removing 379 false positive MACE predictions. Likewise, when operating at a similar false positive rate than LVEF (point C), our method improves sensitivity by 20%, detecting 96% of the MACE cases. Alternatively, the operating point D of our model could be chosen for MACE prediction, where both sensitivity and specificity are improved with respect to LVEF. This illustrates the potential for risk management improvement associated to superior AUC scores.
The combination of the 3 directions of enhancement led to a major improvement compared to ALL baseline, and to the selection of one variable from each of these directions (GLS, C16 and VtAI5 – and VtAI3, in LDA). In comparison to LVEF, the AUC improvement was superior to 13% in both LDA and Cox analyses (See Fig. 5A and Table 3).
At a univariate level (see Table 2), GLS was the most prognostic variable (AUCk = 0.710), followed by GCS, active filling contribution, and RR-interval. The AI-derived volume transient metrics were not among the most predictive variables, but ended up being the most complementary in multivariable settings.
Discussion
This is the first large-sized multicenter CMR study to date that comprehensively characterizes the LV cardiac pump function after AMI using (I) LV volume temporal transient; and that combines it with (II) CMR-FT strain and (III) 3D shape analyses for improved risk assessment. We have successfully (a) developed a fully automated AI pipeline, available and easy to run, that segments the CMR SAx stacks and identifies the AMI acute contraction signatures associated to risk; (b) demonstrated the additional prognostic value of cardiac characterization via any of the 3 proposed directions of enhancement; and (c) combined these markers with the established CMR indexes, cardiovascular risk factors and patient characteristics towards a final CMR prediction model, that outdoes LVEF in a 13% (LDA, cross-validated AUC).
Impact and clinical translation
While multiple trials have shown the incremental prognostic information of CMR-based risk models in AMI management, these models have not found their role in clinical practice6,9,15. The more common availability of echocardiography, the length of the CMR acquisitions, the requirement for manual interaction, and the increasing complexity of CMR multi-parameter models have been among the barriers for adoption4,9. Nevertheless, CMR post-infarction protocols have been shortened and CMR availability has significantly increased in recent years. Moreover, complex CMR prognostic predictors can be now synthetized into simple risk scores models9, and fully automated as shown in this study. Our work further facilitates the adoption of CMR post-infarction risk management by contributing to two of the pillars of healthcare systems: efficacy and efficiency3. That is, it leads to an improved prognostic assessment while reducing the analysis time.
Our automated pipeline for volume temporal transients runs on a standard laptop: it receives a CMR scan, segments the LV cavity, calculates the volume transient and summarizes the patient contraction dynamics into 4 biomarkers (VtAI), that are used in the multivariable models to predict risk. The same applies to the automated shape analysis pipeline presented in18. This is done in the order of seconds, removing the burden of manual segmentations, and drastically reducing the analysis time. Moreover, the method is deterministic (i.e. given a scan, it always returns the same outputs) which, in turn, eliminates intra- and interobserver variability towards a more objective, standardized, and quantitative diagnosis. Conventional metrics extracted from volume transients often rely on rather qualitative detection landmarks (e.g. diastasis), which may be problematic especially in pathological cases. Importantly, our proposed VtAI markers are robust to outliers and abnormal cases. Furthermore, the strong correlation between the automated and manual volumes, along with the high values and small variance of the segmentations dice scores (see Results), supports the accuracy and precision of the automated pipeline, which additionally outdoes commercially available software in performance41.
LV contraction dynamics are conventionally based on global function metrics evaluated at two single time points (ES and ED, or their ratio, LVEF). The tree proposed approaches to unveil further prognostic value complement each other in the final multi-variate regression model: first, the temporal resolution is increased by the volume transient metrics (from two points to the whole cycle); second, the bulk tissue contraction is assessed by the strain patterns (CMR-FT—from global function to quantification in longitudinal, radial and circumferential directions); and third, the 3D anatomical detail is studied by statistical shape models18. The relevance of looking at AMI management from all these different angles is evidenced by the results of the final risk-assessment model that combines global function descriptors (ESV, EDV), 3D contraction patterns (C16), strains (GLS), contraction dynamics indexes (VtAI3, VtAI5) and patient characteristics (age).
Our model provides a remarkable boost in MACE prediction: 13% AUC improvement, when comparing against LVEF (Fig. 5A and Table 3), the reference marker according to AMI guidelines4,6. The implications of these AUC improvements, in terms of specificity and sensitivity, are illustrated in Fig. 5B. The large size of the cohort of this study, the small gap between resubstitution and cross-validation metrics, and the narrow variance when using 100 random data splits (see Fig. 5A and Table 3) suggest the robustness of our method for MACE prediction.
The prognostic contribution of the proposed enhanced characterization of cardiac contraction is in line with recent publications exploring alternative characterizations to LVEF42,43. For example, Dong et al. demonstrated the contribution of CURE and RURE to AMI risk stratification in a cohort of 450 STEMI patients42; and Lange et al. proved the incremental prognostic value of an improved myocardial deformation assessment above LVEF (a c-index increase from 0.7 to 0.74, p = 0.03) in a trial including 566 STEMI patients43. Our work incorporates most of the prognostic parameters discussed in these publications; considers a larger cohort of patients, including both STEMI and NSTEMI aetiologies; and results in a superior prognostic contribution in comparison to LVEF (a c-index increase from 0.67 to 0.76, p < 0.001—See Table 3).
As a result of the automated analysis, we have built a reference LV volume transient atlas from 1000 + AMI subjects that captures the average post-infarct contraction behavior along with the main variations (PCA modes). The atlas and the associated methods to calculate the herein proposed VtAI markers and the resulting risk models, made publicly available (doi.org/https://doi.org/10.6084/m9.figshare.16735300), will not only allow the validation of the presented findings but also further AMI studies, computer simulations, and synthetic patient data generation for training algorithms or educational use, among others. Moreover, while the AI decomposition methodology has been deployed using CMR volume transients, its application to echocardiography is straightforward. The segmentation algorithm, extendable to different protocols and scanners to the ones used in this study as well as to other cardiac diseases44, is likewise available.
Interpretability: unravelling the AMI contraction
While infarct severity is associated with functional and morphological alterations, and certain acute changes have proven significant to AMI prognosis, their interplay in modulating risk remains unsolved6,13,45,46. In this regard, we have identified detailed contraction traits, combining strains, LV shape and volume transient, significantly relevant to MACE occurrence.
Conventional descriptors of the contraction dynamics have identified the RR-interval length and the active and passive contributions to filling, in mL, as particularly related to MACE occurrence (see Table 2). Since patients at risk exhibit a decreased LVEF4,6, their passive and active contributions are accordingly reduced and, in consequence, their RR-interval shortened to increase cardiac output47. This, in turn, leads to a smaller preload and a less effective contraction, with the corresponding drop in LVEF (negative feedback loop).
RR variability was also found to be highly prognostic. It can be hypothesized that this beat-to-beat variation could be related to atrial fibrillation, as evidenced in48, especially given the mentioned fast heart rates, related to ventricular tachycardia and fibrillation risk according to the MADIT-ICD score49, that characterize our MACE population. Nevertheless, and oppositely, the RR variability has been shown beneficial in other atrial fibrillation disease models50.
One of the advantages of our AI methodology is that the resulting VtAI variables are not obscure features for prediction improvement, but they rather encode explainable contraction patterns, as shown in Fig. 3. Thus, VtAI1 can be primarily interpreted as RR-interval length (rp = -0.84); VtAI2, as average systolic velocity (rp = 0.60); VtAI3, as a balance between the active and passive contributions (passive filling: rp = 0.67); and VtAI5, as diastasis once corrected for RR-interval length (diastasis: rp = -0.32; rs = -0.43. See Supplemental Material VI). Nevertheless, these contraction variations contain more information than just a single conventional metric, reason why they are also correlated to other variables (i.e. in a contraction with reduced RR-interval the diastolic and diastasis times will be likewise compromised; therefore, VtAI1, correlated to RR-interval, is also correlated to these indexes) and reason why they led to greater prognostic contributions.
In this context, the minmax normalization prior to the AI analysis, standardizing the transients by removing ventricle size and SV information, should be considered when visually interpreting the results (See Supplemental Material II). This normalization decreases the univariate predictive power of the VtAI markers to the benefit of removing cofounding factors and facilitating their contribution to multivariable settings. This is evidenced by their significant contribution in any of the considered multivariable approaches (Table 3), unlike, for instance, the CMR tissue markers (MVO and IS), which are predictive according to the univariate analysis but do not significantly add value to the multivariable models. VtAI3 and VtAI5, both interpreted as related to diastolic function, are the AI-derived transient markers that contribute the most towards additional prognosis in the multivariable settings (Table 3). While mechanistic explanations are to be further explored, a plausible interpretation is that, in patients at risk, RR-interval shortening compromises ventricular relaxation. This may come at the expense of diastasis (VtAI5), and/or faster dynamics with a swift in the relative filling contributions (%SV) towards the passive phase (VtAI3). This in turn explains the consequent significant increase in normalized passive filling velocity (note that the actual passive filling velocities, in mL/s, without normalization, are smaller for the MACE population, due to a smaller LVEF—see Table 2). This is particularly relevant for the STEMI population (see Fig. 3).
Likewise, the 3D LV patterns, already reported and interpreted in18, demonstrated being consistent with ESV, LFEF and ES myocardial thickness45,46 and highlighted the additional prognostic value of a lateral and a basal contraction impairment. The interplay between these myocardial 3D shape changes and micro-damage, in the form of IS and MVO, was also evidenced.
Strain has been proven an important parameter to evaluate risk after an infarct, as discussed in the introduction section. This is evidenced in this work, where all the CMR-FT strain metrics reported were highly significant to MACE occurrence (Table 2). The compromised GLS, GRS and GCS that the MACE population exhibits are in harmony with the LVEF decrease associated to risk4,6. Besides, the observed relationship between risk and impaired contraction uniformity (CURE and RURE) is widely discussed in9. The prognostic value of GLS, superior to LVEF and incremental to the baseline markers, is in accordance with21, that argues for its adoption in clinical practice.
The proposed risk models have superior prognostic value for patients with decreased LV function (LVEF < 35%), identified as high-risk group, in accordance with the literature4,10 (Fig. SV.2). With respect to infarct aetiology, the risk models that include CMR-FT strain or 3D LV patterns are more predictive for the NSTEMI population, despite the study being biased to STEMI patients (see Fig. SV.3 and Results—Patients). The final model, that combines strains, AI transient metrics and 3D LV metrics, reports a similar performance for STEMI (AUC = 0.795) and NTEMI (AUC = 0.799), and, thus, clinical validity for both infarct types could be claimed. If analyzed separately, both systolic and diastolic components significantly contribute to the multivariable risk assessment models (see Supplemental Material VII). However, as expected, the combination of systolic and diastolic function, that is, the entire transient, is more prognostic than each of the components on its own, especially in the systolic scenario (in line with the fact that the modes that provide more additional prognostic value, VtAI3 and VtAI5, are related to diastolic function, as mentioned above).
To sum up, patients at risk of MACE occurrence have a compromised cardiac function, which contributes to an impaired global contraction (decreased LVEF and GLS) and, therefore, to a reduced RR-interval, in order to maintain the cardiac output. This, in turn, leads to diastolic impairment, exhibited in the form of compromised diastasis and active filling.
Limitations
Patients were imaged within 10 days after infarction in the absence of optimal post-infarction CMR imaging time recommendations9. The effect of this post-infarction imaging time on the proposed AI-derived metrics has not been assessed. It is however hypothesized that we are capturing acute injury, whose extent and phenotype can predict outcome, and therefore long-term LV adaptions. The main objective of this work was to evaluate this hypothesis in a clinical cohort with the proposed enhanced characterization of cardiac contraction, and not to optimize MACE prediction. This motivates the herein explained identification of metrics based on linear models that eases interpretability. Future research could explore the use of advanced nonlinear classifiers, as illustrated in51, to improve performance in MACE prediction at the cost of losing the ability to interpret findings. CMR long-axis views were excluded for simplicity, but ideally could be incorporated to correct for SAx misalignment and improve the cavity volume calculations. Likewise, while only CMR-FT global strains and uniformity ratio estimates have proved prognostic in the literature7,9,13,19,20, future work could consider a more detailed strain analysis, in time and by regions, especially given the outstanding prognostic power of GLS. Similar methodologies to the PCA analysis formulated in this study could be considered for this. The resolution of the volume transients herein derived is limited to the resolution of the CMR scan. Given the limited number of MACE events (class imbalance), splitting the cohort into an independent testing dataset would be rather noisy. The combination of cross-fold validation, repeated for a hundred data-splits, is therefore preferred as it ensures fair performance and robust conclusions reporting, as argued in36,40. The study population is biased towards male sex. Finally, the results are limited to AMI patients that can undergo a CMR scan. This is an active field of research and future investigations are required to integrate these measures into standard clinical practice.
Conclusion
This large multicenter study evidences the prognostic value of a comprehensive characterization of cardiac contraction by the novel use of LV volume transients, combined with metrics of strain and 3D shape, in post-infarction management. Moreover, it further contributes towards the adoption of CMR multivariable models in clinical practice by demonstrating the feasibility of fully automated analysis and the relevance of these models in AMI stratification.
Data availability
The data that supports the findings is available upon reasonable request to the corresponding author of this manuscript.
Abbreviations
- 2D:
-
Two-dimensional
- 3D:
-
Three-dimensional
- AI:
-
Artificial intelligence
- AMI:
-
Acute myocardial infarction
- AUC:
-
Area under the receiver-operator characteristic curve
- C-index:
-
Concordance index
- CMR:
-
Cardiac magnetic resonance
- CMR-FT:
-
CMR myocardial feature tracking
- CURE:
-
Circumferential uniformity ratio estimate
- ED:
-
End-diastole
- EDV:
-
End-diastolic volume
- ES:
-
End-systole
- ESV:
-
End-systolic volume
- FT:
-
Feature tracking
- GCS:
-
Global circumferential strain
- GLS:
-
Global longitudinal strain
- GRS:
-
Global radial strain
- IQR:
-
Interquartile range
- IS:
-
Infarct size
- LDA:
-
Linear discriminant analysis
- LGE:
-
Late gadolinium enhancement
- LV:
-
Left ventricle
- LV3D :
-
LV 3D patterns markers
- LVEF:
-
Left ventricular ejection fraction
- MACE:
-
Major adverse cardiac events
- MVO:
-
Microvascular obstruction
- NSTEMI:
-
Non-ST‐segment-elevation myocardial infarction
- PCA:
-
Principal component analysis
- rp :
-
Pearson correlation coefficient
- rs :
-
Spearman correlation coefficient
- RURE:
-
Radial uniformity ratio estimate
- SAx:
-
Short-axis stack
- STEMI:
-
ST‐segment-elevation myocardial infarction
- SV:
-
Stroke volume
- Vt:
-
Conventional volume temporal transients
- VtAI :
-
AI-derived volume temporal transients
References
Virani, S. S. et al. Heart disease and stroke statistics-2020 update: A report from the American heart association. Circulation 141(9), e139-596 (2020).
James, S. L. et al. Global, regional, and national incidence, prevalence, and years lived with disability for 354 diseases and injuries for 195 countries and territories, 1990–2017: A systematic analysis for the global burden of disease study 2017. Lancet 392(10159), 1789–1858 (2018).
Corral Acero, J. et al. The ‘Digital Twin’ to enable the vision of precision cardiology. Eur. Heart J. 41, 4556–4564 (2020).
Ibanez, B. et al. 2017 ESC guidelines for the management of acute myocardial infarction in patients presenting with ST-segment elevation: The task force for the management of acute myocardial infarction in patients presenting with ST-segment elevation of the European society of cardiology (ESC). Eur. Heart J. 39(2), 119–177 (2017).
Anderson, J. L. & Morrow, D. A. Acute myocardial infarction. N. Engl. J. Med. 376(21), 2053–2064 (2017).
Eitel, I. et al. Comprehensive prognosis assessment by CMR imaging after ST-segment elevation myocardial infarction. J. Am. Coll. Cardiol. 64(12), 1217–1226 (2014).
Amzulescu, M. S. et al. Myocardial strain imaging: Review of general principles, validation, and sources of discrepancies. Eur. Heart J. Cardiovasc. Imaging 20(6), 605–619 (2019).
Smiseth, O. A., Torp, H., Opdahl, A., Haugaa, K. H. & Urheim, S. Myocardial strain imaging: How useful is it in clinical decision making?. Eur. Heart J. 37(15), 1196–1207 (2015).
Stiermaier, T. et al. Cardiac magnetic resonance left ventricular mechanical uniformity alterations for risk assessment after acute myocardial infarction. J. Am. Heart Assoc. 8(16), e011576 (2019).
Dagres, N. & Hindricks, G. Risk stratification after myocardial infarction: Is left ventricular ejection fraction enough to prevent sudden cardiac death?. Eur. Heart J. 34(26), 1964–1971 (2013).
Bulluck, H., Dharmakumar, R., Arai, A. E., Berry, C. & Hausenloy, D. J. Cardiovascular magnetic resonance in acute ST-segment–elevation myocardial infarction. Circulation 137(18), 1949–1964 (2018).
Dall’Armellina, E. From recognized to novel quantitative CMR biomarkers of LV recovery. JACC Cardiovasc. Imaging 10(9), 1000–1002 (2017).
Reindl, M., Eitel, I. & Reinstadler, S. J. Role of cardiac magnetic resonance to improve risk prediction following acute ST-elevation myocardial infarction. J. Clin. Med. 9(4), 1041 (2020).
Stokes, M. B. & Roberts-Thomson, R. The role of cardiac imaging in clinical practice. Aust. Prescr. 40(4), 151–155 (2017).
Stiermaier, T. et al. Optimized prognosis assessment in ST-segment-elevation myocardial infarction using a cardiac magnetic resonance imaging risk score. Circ. Cardiovasc. Imaging 10(11), e006774 (2017).
Masci, P. G. et al. Relationship between location and size of myocardial infarction and their reciprocal influences on post-infarction left ventricular remodelling. Eur. Heart J. 32(13), 1640–1648 (2011).
Ortiz-Pérez, J. T. et al. Correspondence between the 17-segment model and coronary arterial anatomy using contrast-enhanced cardiac magnetic resonance imaging. JACC Cardiovasc. Imaging 1(3), 282–293 (2008).
Corral Acero, J. et al. Understanding and improving risk assessment after infarction using automated left ventricular shape analysis. JACC Cardiovasc. Imaging 15, 1563–1574 (2022).
Mangion, K., McComb, C., Auger, D. A., Epstein, F. H. & Berry, C. Magnetic resonance imaging of myocardial strain after acute ST-segment-elevation myocardial infarction a systematic review. Circ. Cardiovasc. Imaging 10(8), e006498 (2017).
Eitel, I. et al. Cardiac magnetic resonance myocardial feature tracking for optimized prediction of cardiovascular events following myocardial infarction. JACC Cardiovasc. Imaging 11(10), 1433–1444 (2018).
Reindl, M. et al. Prognostic implications of global longitudinal strain by feature-tracking cardiac magnetic resonance in ST-elevation myocardial infarction. Circ. Cardiovasc. Imaging 12(11), e009404 (2019).
Ruijsink, B. et al. Fully automated, quality-controlled cardiac analysis from CMR: Validation and large-scale application to characterize cardiac function. JACC Cardiovasc. Imaging 13(3), 684–695 (2020).
Thiele, H. et al. Effect of aspiration thrombectomy on microvascular obstruction in NSTEMI patients: The TATORT-NSTEMI trial. J. Am. Coll. Cardiol. 64(11), 1117–1124 (2014).
Eitel, I. et al. Intracoronary compared with intravenous bolus abciximab application during primary percutaneous coronary intervention in ST-segment elevation myocardial infarction: Cardiac magnetic resonance substudy of the AIDA STEMI trial. J. Am. Coll. Cardiol. 61(13), 1447–1454 (2013).
Thiele, H. et al. Intracoronary versus intravenous bolus abciximab during primary percutaneous coronary intervention in patients with acute ST-elevation myocardial infarction: A randomised trial. Lancet 379(9819), 923–931 (2012).
Riley, R. D. et al. Calculating the sample size required for developing a clinical prediction model. BMJ 368, m441 (2020).
Circle Cardiovascular Imaging Inc. cvi42 [Computer Software]. Version: v5.14.3. https://www.circlecvi.com/cvi42 (03 Mar. 2024).
Backhaus, S. J. et al. Head-to-head comparison of cardiovascular MR feature tracking cine versus acquisition-based deformation strain imaging using myocardial tagging and strain encoding. Magn. Reson. Med. 85(1), 357–368 (2021).
TomTec Imaging Systems. 2D Cardiac Performance Analysis MR [Computer Software]. Version: v1.1.2. https://www.tomtec.de/products/application-finder/2d-cpa# (03 Mar. 2024).
Gertz, R. J. et al. Inter-vendor reproducibility of left and right ventricular cardiovascular magnetic resonance myocardial feature-tracking. PLoS One 13(3), e0193746 (2018).
Kowallick, J. T. et al. Quantitative assessment of left ventricular mechanical dyssynchrony using cine cardiovascular magnetic resonance imaging: Inter-study reproducibility. JRSM Cardiovasc. Dis. 6(1), 8 (2017).
Corral-Acero, J. et al. SMOD-Data Augmentation Based on Statistical Models of Deformation to Enhance Segmentation in 2D Cine Cardiac MRI. In FIMH 2019. LNCS Vol. 11504 (eds Coudière, Y. et al.) 361–369 (Springer, 2019).
Corral Acero, J. et al. Left Ventricle Quantification with Cardiac MRI: Deep Learning Meets Statistical Models of Deformation. In STACOM 2019 LNCS Vol. 12009 (eds Pop, M. et al.) 384–394 (Springer, 2019).
Yang G, Hua T, Xue W, Shuo L. Left Ventricle Full Quantification Challenge MICCAI 2019. https://lvquan19.github.io/ (29 Sept. 2021).
Belhumeur, P. N., Hespanha, J. P. & Kriegman, D. J. Eigenfaces vs. fisherfaces: Recognition using class specific linear projection. IEEE Trans. Pattern Anal. Mach. Intell. 19(7), 711–720 (1997).
Moons, K. G. M. et al. Transparent reporting of a multivariable prediction model for individual prognosis or diagnosis (TRIPOD): Explanation and elaboration. Ann. Intern. Med. 162(1), W1–W73 (2015).
Harrell, F. E., Califf, R. M., Pryor, D. B., Lee, K. L. & Rosati, R. A. Evaluating the yield of medical tests. J. Am. Med. Assoc. 247(18), 2543–2546 (1982).
The MathWorks Inc. MATLAB [Computer Software]. Version: R2019b. https://uk.mathworks.com/products/matlab.html (03 Mar. 2024).
ONEIROS. Keras: The high-level API for TensorFlow [Computer Software]. Version: Keras 2.3.0. https://www.tensorflow.org/guide/keras (03 Mar. 2024).
Vandenbroucke, J. P. et al. Strengthening the reporting of observational studies in epidemiology (STROBE): Explanation and elaboration. PLoS Med. 4(10), e297 (2007).
Schuster, A. et al. Fully automated cardiac assessment for diagnostic and prognostic stratification following myocardial infarction. J. Am. Heart Assoc. 9(18), e016612 (2020).
Dong, J. X. et al. MR uniformity ratio estimates to evaluate ventricular mechanical dyssynchrony and prognosis after ST-segment elevation myocardial infarction. J. Magn. Reson. Imaging 59, 1820–1831 (2023).
Lange, T. et al. Impact of myocardial deformation on risk prediction in patients following acute myocardial infarction. Front. Cardiovasc. Med. 10, 1199936 (2023).
Corral Acero, J., Sundaresan, V., Dinsdale, N., Grau, V. & Jenkinson, M. A 2-Step Deep Learning Method with Domain Adaptation for Multi-Centre Multi-Vendor and Multi-Disease Cardiac Magnetic Resonance Segmentation. In STACOM 2020 LNCS Vol. 12592 (eds Puyol, E. et al.) 196–207 (Springer, 2021).
Cohn, J. N., Ferrari, R. & Sharpe, N. Cardiac remodeling-concepts and clinical implications: A consensus paper from an international forum on cardiac remodeling. J. Am. Coll. Cardiol. 35(3), 569–582 (2000).
Hassell, M. E. et al. Long-term left ventricular remodelling after revascularisation for ST-segment elevation myocardial infarction as assessed by cardiac magnetic resonance imaging. Open Heart 4(1), e000569 (2017).
Jabre, P. et al. Resting heart rate in first year survivors of myocardial infarction and long-term mortality: A community study. Mayo Clin. Proc. 89(12), 1655–1663 (2014).
Lyon, A. et al. Both beat-to-beat changes in RR-interval and left ventricular filling time determine ventricular function during atrial fibrillation. EP Europace 23, i21–i28 (2021).
Younis, A. et al. Predicted benefit of an implantable cardioverter-defibrillator: The MADIT-ICD benefit score. Eur. Heart J. 42(17), 1676–1684 (2021).
Brateanu, A. Heart rate variability after myocardial infarction: What we know and what we still need to find out. Curr. Med. Res. Opin. 31(10), 1855–1860 (2015).
Arano-Martinez, J. A., Martínez-González, C. L., Salazar, M. I. & Torres-Torres, C. A framework for biosensors assisted by multiphoton effects and machine learning. Biosensors 12(9), 710 (2022).
Acknowledgements
This work was supported by the EU’s Horizon 2020 research and innovation program under the Marie Sklodowska-Curie (g.a. 764738), the DZHK (German Center for Cardiovascular Research), the British Heart Foundation (PG/16/75/32383, FS/17/22/32644), and the Wellcome Trust (209450/Z/17). For the purpose of Open Access, the authors have applied a CC BY public copyright license to any Author Accepted Manuscript (AAM) version arising from this submission.
Author information
Authors and Affiliations
Contributions
All the authors listed in the article contributed to the conception, design, analysis and interpretation of the data, as well as to the revision of the article. They all have read and approved this final version.
Corresponding authors
Ethics declarations
Competing interests
The authors declare no competing interests.
Additional information
Publisher's note
Springer Nature remains neutral with regard to jurisdictional claims in published maps and institutional affiliations.
Supplementary Information
Rights and permissions
Open Access This article is licensed under a Creative Commons Attribution 4.0 International License, which permits use, sharing, adaptation, distribution and reproduction in any medium or format, as long as you give appropriate credit to the original author(s) and the source, provide a link to the Creative Commons licence, and indicate if changes were made. The images or other third party material in this article are included in the article's Creative Commons licence, unless indicated otherwise in a credit line to the material. If material is not included in the article's Creative Commons licence and your intended use is not permitted by statutory regulation or exceeds the permitted use, you will need to obtain permission directly from the copyright holder. To view a copy of this licence, visit http://creativecommons.org/licenses/by/4.0/.
About this article
Cite this article
Corral Acero, J., Lamata, P., Eitel, I. et al. Comprehensive characterization of cardiac contraction for improved post-infarction risk assessment. Sci Rep 14, 8951 (2024). https://doi.org/10.1038/s41598-024-59114-3
Received:
Accepted:
Published:
DOI: https://doi.org/10.1038/s41598-024-59114-3
Comments
By submitting a comment you agree to abide by our Terms and Community Guidelines. If you find something abusive or that does not comply with our terms or guidelines please flag it as inappropriate.