Abstract
Quantitative PCR (qPCR) is a widely used method to study gene expression changes following brain injury. The accuracy of this method depends on the tissue harvested, the time course analyzed and, in particular on the choice of appropriate internal controls, i.e., reference genes (RGs). In the present study we have developed and validated an algorithm for the accurate normalization of qPCR data using laser microdissected tissue from the mouse dentate gyrus after entorhinal denervation at 0, 1, 3, 7, 14 and 28 days postlesion. The expression stabilities of ten candidate RGs were evaluated in the denervated granule cell layer (gcl) and outer molecular layer (oml) of the dentate gyrus. Advanced software algorithms demonstrated differences in stability for single RGs in the two layers at several time points postlesion. In comparison, a normalization index of several stable RGs covered the entire post-lesional time course and showed high stability. Using these RGs, we validated our findings and quantified glial fibrillary acidic protein (Gfap) mRNA and allograft inflammatory factor 1 (Aif1/Iba1) mRNA in the denervated oml. We compared the use of single RGs for normalization with the normalization index and found that single RGs yield variable results. In contrast, the normalization index gave stable results. In sum, our study shows that qPCR can yield precise, reliable, and reproducible datasets even under such complex conditions as brain injury or denervation, provided appropriate RGs for the model are used. The algorithm reported here can easily be adapted and transferred to any other brain injury model.
Similar content being viewed by others
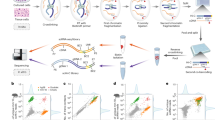
Introduction
Quantitative polymerase chain reaction (qPCR) has emerged as a standard for precise analysis of quantitative changes in gene expression, especially when only a few target genes are examined or when a small amount of tissue is used1,2,3,4. To ensure accurate and reproducible data, qPCR experiments comprise several aspects, including experimental design, sample preparation and data analysis5,6. The use of reference genes (RGs) is the most common strategy to normalize target gene expression of interest. However, their suitability must be experimentally validated7,8,9,10,11. Several studies demonstrated that expression levels of some commonly used RGs might vary considerably depending on the specific condition investigated, e.g., traumatic brain injury12,13,14,15,16. Unfortunately, there is no ideal RG for all experimental conditions and stability of RGs cannot be simply assumed. Under conditions of brain injury, the situation may be even more complex, because gene expression for multiple pathways of injury and repair vary with time following lesion17,18,19 as well as with age15,20,21,22. Furthermore, gene expression levels of neurons and glial cells depend on their distance from the lesion23 and, thus, studies using laser microdissected tissues may provide more precise, robust and reproducible results than studies using homogenized larger tissue blocks15,24.
To demonstrate the usefulness of this combined approach in a proof-of-principle experiment, we investigated the expression stability of candidate RGs in the dentate gyrus (DG) following entorhinal cortex lesion, a classical model for neural reorganization of the brain after injury20,25,26,27,28. In this model, transection of the perforant path results in the denervation of the outer molecular layer (oml) of the DG, accompanied by a strong glial reaction29,30. Candidate RGs were chosen based on earlier entorhinal lesion studies of the rodent brain16,31,32 and because of other relevant brain injury studies12,13,14,33,34,35,36. In contrast to previous studies using total hippocampal tissue following entorhinal lesion32, we used laser microdissection to harvest two hippocampal layers, i.e., the granule cell layers, where the denervated granule cells are located and the oml, i.e., the zone of denervation where strong glial reactions have been reported29,37,38. Expression stability of ten putative RGs, i.e., actin, beta (Actb), aminolevulinic acid synthase 1 (Alas1), beta-2 microglobulin (B2m), glyceraldehyd-3-phosphate dehydrogenase (Gapdh), hypoxanthine guanine phosphoribosyl transferase (Hprt), phosphoglycerate kinase I (Pgk1), peptidyl propyl isomerase A (Ppia), ribosomal protein L13A (Rpl13a), succinate dehydrogenase complex subunit A (Sdha) and transferrin receptor (Tfrc), were evaluated using commercially available software algorithms, i.e., NormFinder39 and geNorm40, and consensus ranking analysis41. To validate our findings, two prominent glial genes were chosen, i.e., glial fibrillary acidic protein (Gfap), a marker for reactive astrocytes, and allograft inflammatory factor 1 (Aif1)—better known as ionized calcium binding adaptor molecule 1 (Iba1)—a marker for activated microglia cells. Both genes are known to be upregulated after brain injuries and following entorhinal lesion29,42,43,44. Our study showed that single RGs were not sufficiently stable to study the time course of these genes over the entire postlesional time. In contrast, an index of several RGs yielded reliable, reproducible, and accurate results. This index should be used for normalization of qPCR data following entorhinal denervation and similar indexes should be identified using the algorithm described here for other lesion models to provide accurate qPCR data.
Results
Transection of the perforant path denervates the outer molecular layer of the dentate gyrus and induces strong and layer-specific astro- and microglial responses
The entorhino-hippocampal lesion model26,29 was used to examine the expression stability of putative RGs in the denervated adult mouse DG (Fig. 1). Transection of the perforant path leads to layer-specific denervation of the outer part of the dentate molecular layer, the oml (Fig. 1a). Quality and completeness of entorhinal denervation were demonstrated using Fluoro-Jade C staining (Fig. 2a,b). A strong glial reaction was observed in the DG ipsilateral to the lesion (ipsi) compared to the DG contralateral to the lesion (contra). Immunofluorescence labeling for GFAP, a classical marker for reactive astrocytes (Fig. 2c,d), as well as for IBA1, a widely used marker for activated microglia (Fig. 2e,f) revealed strongly increased reactivity for both markers in the denervated zone at 7 days postlesion.
Entorhino-hippocampal denervation model. (a) Schematic of a horizontal brain section illustrating the entorhino-hippocampal denervation model. The perforant path (green) originates from stellate neurons in the entorhinal cortex (EC) and terminates on distal parts of granule cell dendrites in the outer molecular layer (oml) of the dentate gyrus (DG). The transection site of the perforant path is indicated by a dotted line. (b) Representative brain section (horizontal plane, cresyl violet staining) after transection of the perforant path as indicated in (a). Note the distance of the dentate gyrus from the lesion site (arrowheads). CA1, CA3: cornu ammonis subregions, gcl: granule cell layer, h: hilus; Cx: cortex. Scale bar: 250 μm.
Entorhinal denervation leads to a layer-specific denervation and a layer-specific glial reaction in the outer molecular layer of the dentate gyrus. (a, b) Unilateral transection of the perforant path leads to layer-specific denervation in the dentate gyrus (DG) as shown by Fluoro-Jade C staining (green) at 7 days post lesion. The contralateral hippocampus is shown as control (a). Note that Fluoro-Jade C staining is seen in the outer molecular layer (oml) of the DG only in the ipsilateral hippocampus (b). Nuclei (blue) were counterstained with Hoechst 33342. (c–f) Layer-specific activation of glial cells in the DG oml following entorhinal lesion (7 days post lesion). Sections were immunolabeled against glial fibrillary acid protein (GFAP) (c, d) and ionized calcium-binding adapter molecule 1 (IBA1) (e, f), marker molecules for reactive astrocytes and activated microglia, respectively. The contralateral hippocampus is shown as control (GFAP (c); IBA1 (e)). Strong layer-specific activation of both glial cell types is seen in the denervated oml of the ipsilateral side (GFAP (d); IBA1 (f)). For counterstaining, NeuN (neuronal marker, green) was used. CA1, CA3: cornu ammonis subregions, slm: stratum lacunosum moleculare, gcl: granule cell layer. Scale bars: 250 μm.
Quantitative expression analysis of putative reference genes in the laser microdissected granule cell layer and outer molecular layer of the dentate gyrus
At first, expression levels of putative RGs were evaluated in the granule cell layer (gcl) and oml of the DG to prove for abundance as well as to examine the variability of selected RGs. Laser microdissection was used to dissect and harvest the two dentate layers (Fig. 3a). RNA integrity analysis confirmed the high quality of isolated RNA (RIN values > 8) from gcl and oml samples used for further qPCR experiments (Fig. 3b,c). Quantitative PCR amplification demonstrated absolute quantification cycle (Cq) values in the standard range from 20 to 32 for all putative RGs across the tissue samples (Fig. 4a). ΔCq values of replicates for the dentate gcl were below a cut-off of 0.5 and, thus, all RGs were included in our evaluation (Fig. 4b). In the dentate oml samples, however, three putative RGs, i.e., Alas1, Hprt, and Tfrc, had ΔCq values greater than 0.5 and, thus, these candidates had to be excluded (Fig. 4b).
Laser microdissection of dentate subregions. (a) Hippocampal section (coronal plane, dorsal part of the hippocampus, cresyl violet staining) before (inset) and after laser microdissection of the granule cell layer (gcl) and the outer molecular layer (oml). (b, c) RNA integrity analysis of total RNA isolated from the dissected gcl and oml demonstrating highly intact RNA (RIN-values: 8.15–8.3) as determined using the Agilent 2100 Bioanalyzer system. DG: dentate gyrus, CA1, CA3: cornu ammonis subregions). Scale bar: 200 μm.
Cq values of candidate reference genes in microdissected dentate gyrus regions. (a) Box plots represent absolute Cq values of ten putative reference genes in laser-microdissected granule cell layers and outer molecular layers of the dentate gyrus. Optimal range of Cq values is highlighted in grey. (b) ∆ Cq values of replicates (doublets) of candidate reference genes. Optimal range of ΔCq values is highlighted in grey. Cq, quantification cycle. N = 6 animals per layer.
Expression stability of putative reference genes in the granule cell layer of the dentate gyrus after entorhinal denervation
Ten putative RGs were tested for their expression stability in the dentate gcl at different time points following entorhinal denervation, i.e., 1, 3, 7, 14 and 28 days. RGs were ranked according to the stability values calculated by NormFinder and by averaged expression using geNorm. RG stability measurements calculated by these algorithms were then used for a consensus ranking analysis employing RankAggreg (Table 1). Furthermore, expression stability of candidate RGs was analyzed for the entire lesion period, i.e., from 1 to 28 dpl (Fig. 5a). Calculation of pairwise variation analysis based on the consensus ranking list for each time-point (see Table 1) and, in addition, for all time-points following lesion revealed a minimum number of at least three stable RGs required for normalization (Fig. 5b). NormFinder identified Hprt and Ppia as the best pair of RGs, whereas geNorm determined Gapdh, Pgk1 and Hprt as the most stable RGs for qPCR normalization. Summarized by RankAggreg, the consensus stability list of RGs for the entire lesion period reads as follows (most stable to least stable): Gapdh–Pgk1–Hprt–Ppia–Sdha–Rpl13a–Actb–Alas1–B2m–Tfrc.
Evaluation of consensus ranking and determination of the optimal number of reference genes by pairwise variation for the granule cell layer after entorhinal denervation. (a) Expression stability of candidate reference genes for all time-points after entorhinal denervation analyzed by NormFinder and geNorm. RankAggreg was used for consensus ranking analysis. (b) Determination of the optimal number of reference genes recommended for accurate normalization based on the analysis of pairwise variation (V) implemented in geNorm. Every bar represents the change in normalization accuracy by stepwise inclusion of an additional reference gene according to the ranking at specific time points (1, 3, 7, 14, 28 days post lesion, dpl) or for the entire lesion period (all dpl). A cut-off of 0.15 (dashed line) was used below the inclusion of an additional reference gene was not required for proper normalization. Pairwise variation analysis revealed a minimum number of three reference genes needed for accurate normalization.
Evaluation of reference gene expression stability in the outer molecular layer of the dentate gyrus after entorhinal denervation
Expression stability of seven putative RGs (Alas1, Hprt, and Tfrc were excluded, see above) was studied in the microdissected dentate oml after entorhinal denervation using the same approach as for the gcl, i.e., using geNorm, NormFinder and a consensus ranking analysis by RankAggreg (Table 2). In addition, expression stability of putative RGs was analyzed for the entire lesion period (all dpl) following denervation (Fig. 6a). The minimum number of RGs necessary for proper normalization was calculated by pairwise variation for all individual time points and for the entire period after denervation. As a result, three RGs were calculated to be sufficient for accurate qPCR normalization with the exception for 7 dpl, where the minimum number of four RGs was determined (Fig. 6b). NormFinder identified Sdha and Actb as the best RG pair, whereas geNorm calculated Rpl13a, Sdha and Gapdh as the most stable RGs suitable for accurate normalization for the entire lesion period. Summarized by RankAggreg, the consensus ranking list reads as follows (most stable to least stable): Sdha–Ppia–Gapdh–Rpl13a–Actb–Pgk1–B2m.
Evaluation of consensus ranking and determination of the optimal number of reference genes by pairwise variation for the outer molecular layer after entorhinal denervation. (a) Expression stability of candidate reference genes for all time-points after entorhinal denervation analyzed with NormFinder and geNorm. RankAggreg was used for consensus ranking analysis. (b) Determination of the optimal number of reference genes recommended for accurate normalization based on the analysis of pairwise variation (V) implemented in geNorm. Every bar represents the change in normalization accuracy by stepwise inclusion of an additional reference gene according to the ranking at specific time points (1, 3, 7, 14, 28 days post lesion, dpl) or for the entire lesion period (all dpl). A cut-off of 0.15 (dashed line) was set below the inclusion of additional reference genes was not required for normalization. Pairwise variation analysis revealed a minimum number of three reference genes for accurate normalization with the exception at 7 days post lesion, where a minimal number of four reference genes was determined.
Evaluation of validated reference genes for accurate quantification of gene expression changes following entorhinal denervation
Immunostaining for GFAP and IBA1 (see Fig. 2) shows a strong response of GFAP-positive astrocytes and IBA1-positive microglia cells in the denervated oml. To validate our normalization recommendations, we analyzed mRNA expression levels of Gfap and Aif1/Iba1 in the microdissected oml at 1, 3, 7, 14 and 28 dpl. To compare the different normalization strategies, target expression levels were normalized (1) against a single candidate RG, (2) against RG indices calculated by geNorm and NormFinder for each lesion time-point, and (3) using the recommended minimal number of RGs for normalization for the entire lesion period based on the consensus ranking analysis. Normalization against a single RG resulted in high variabilities of Gfap mRNA expression levels in the denervated oml (Fig. 7a,d). Of note, maximal upregulation of Gfap mRNA was found at 7 dpl for all RGs except for Pgk1 (Fig. 7a,d). Using an index of the most stable RGs according to NormFinder and geNorm, a very similar pattern and time course of Gfap mRNA expression following denervation were found for both algorithms (Fig. 7b,d). By using the best combination of RGs identified by NormFinder, a significant increase in Gfap mRNA expression at 3, 7 and 14 dpl (5.9-, 12.7- and 9.4-fold, respectively) was found. Likewise, using the minimal number of RGs for accurate normalization determined by geNorm, Gfap mRNA expression was also found to be significantly upregulated at 3, 7 and 14 dpl (7.4-, 17.37- and 9.4-fold, respectively) (Fig. 7b,d). Finally, using a consensus stability ranking taking the entire postlesion period and both software algorithms into account, an index of the three most stable RGs, i.e., Sdha, Ppia and Gapdh, was identified as appropriate for accurate normalization. As a result, a significant upregulation of Gfap mRNA at 3, 7 and 14 dpl with corresponding expression levels of 6.9-, 16.1 and 10.7-fold, respectively, were obtained (Fig. 7c,d). Likewise, the time course of Aif1 mRNA expression was analyzed in the dentate oml after entorhinal denervation. Aif1 mRNA was found to be upregulated at specific time points after denervation, however, to a much lower extent compared to Gfap mRNA expression. Normalization against a single candidate RG showed relatively variable Aif1 mRNA expression with maximal upregulation at 3 dpl, 7 dpl or 14 dpl, depending on the RG that was applied for normalization (Fig. 8a,d). Importantly, Aif mRNA expression was not significantly different compared to control, if Actb or B2m were used for normalization (Fig. 8a,d). Using RGs indices of both software algorithms, a significant upregulation of Aif1 mRNA was detected at 3 and 7 dpl (Fig. 8b,d). NormFinder identified Rpl13a and Actb at 3 dpl, and Actb and Ppia at 7 dpl as the best combination of RGs for normalization. Aif mRNA expression was found to be upregulated to 2.8- and 2.4-fold, respectively. Normalization with the minimal number of RGs as determined by geNorm revealed an upregulation of Aif1 mRNA of 3.6 and 3.3-fold at 3 dpl (Pgk1, Sdha and Rpl13a) and 7 dpl (Rpl13a, Sdha and Gapdh), respectively. Finally, using a consensus stability ranking taking all time points and both software algorithms into account, an index of the most stable RGs, i.e., Sdha, Ppia, Gapdh, was used for normalization. Here, a significant upregulation of Aif1 mRNA was found again at 3 dpl and 7 dpl as well as at 14 dpl with corresponding expression levels of 3.3-, 3.1- and 2.4-fold compared to control situation (Fig. 8c,d). In conclusion, a distinct time course of expression level changes for GFAP mRNA and for Aif mRNA could be identified in the dentate oml after entorhinal denervation by using indices of stable RGs for accurate qPCR normalization. Of note, maximal Aif1 mRNA upregulation was detected at 3 dpl, while Gfap mRNA level was highest at 7 dpl.
Influence of different normalization strategies for accurate quantification of Gfap mRNA expression levels in the dentate outer molecular layer after entorhinal denervation. (a, d) Normalization using a single reference gene resulted in different Gfap mRNA expression levels in the dentate outer molecular layer after entorhinal denervation. (b, d) In comparison, normalization using reference genes determined by geNorm or NormFinder for specific time-points after denervation showed a comparable time course and pattern of Gfap mRNA expression levels. (c, d) Results from both algorithms were combined using RankAggreg to obtain a consensus ranking for all time-points following denervation. dpl, days post lesion. Data are shown as mean ± SEM. N = 3 animals per time point. Statistics: One-way ANOVA, followed by Dunnett’s post-hoc test, with *p < 0.05.
Influence of different normalization strategies for accurate quantification of Aif1 mRNA expression levels in the dentate outer molecular layer after entorhinal denervation. (a, d) Normalization using a single reference gene resulted in different Aif1 mRNA expression pattern in the dentate outer molecular layer after entorhinal denervation. (b, d) Normalization using reference genes determined by geNorm or NormFinder showed comparable time courses and pattern of Aif1 mRNA expression levels. (c, d) Results from both algorithms were analyzed using RankAggreg to obtain a consensus ranking for the entire lesion period. dpl, days post lesion. Data are shown as mean ± SEM. N = 3 animals per time point. Statistics: One-way ANOVA, followed by Dunnett’s post-hoc test, with *p < 0.05.
To demonstrate the feasibility of our method also for neurons, we analyzed mRNA expression levels of Map2 in the microdissected gcl at 1, 3, 7, 14 and 28 dpl. In this layer, the somata of the denervated granule cells are densely packed and microdissection is an excellent tool to harvest tissue enriched in mRNA of these neurons. Although the response of granule cells to denervation is an order of magnitude weaker than the response of glial cells and barely detectable by conventional methods such as in situ-hybridization or northern blot45,46, we here report changes in Map2 mRNA in mouse granule cells following denervation (Supplementary Fig. 1). Gapdh, Pgk1 and Hprt were used as reference genes, as determined earlier using NormFinder and geNorm. The time course based on these three reference genes suggests an upregulation of Map2 mRNA to ~ 150% at 3 and 7 days after lesion, and a gradual decline thereafter (Supplementary Fig. 1).
Discussion
Quantitative PCR is a highly sensitive method of choice to analyze gene expression changes, e.g., after injury of the rodent brain16,29,37. In recent years, it has become more and more evident that accuracy of qPCR results depends on several critical steps, in particular on the choice of appropriate internal controls, i.e., reference genes5,47,48,49. Earlier studies postulated that a single RG might be sufficient in certain experimental instances35,50. However, recent studies have stressed the importance to select and validate a sufficient number of RGs suitable and specific for the experimental model used14,33,34,36,51,52,53. In studies focusing on brain injury, the situation may be even more complex, since the injured brain goes through a sequence of events with time and a RG suitable for one time point after injury may not be the best RG for other time points. In the present study, we have used a well-established brain injury model, i.e., the entorhinal denervation model, as an example. Using laser microdissection, we cut out with a very high degree of specificity cell layers of the dentate gyrus affected by denervation. Further, we collected tissue at several time points after denervation, thus covering most of the time during which denervation-induced reorganization occurs. This approach demonstrated for this model that it is crucial to select RGs suitable for all time points if time points are to be compared using qPCR. Furthermore, we provided an algorithm, which others can readily adapt and transfer to other brain injury models, making qPCR data in the field of brain injury and regeneration more precise and reproducible.
State-of-the-art software algorithms help to identify stable reference genes
Some of the most widely used RGs after entorhinal lesion are Actb, Gapdh and Hprt32,54,55. However, in those earlier studies mRNA levels were analyzed based on Cq-values relative to control without additional validation, e.g., by using advanced software algorithms like NormFinder or geNorm. These software algorithms are now state-of-the-art and changed profoundly the strategies to identify appropriate RGs39,40. Considering the importance of the design of qPCR experiments5,6, our study provides a useful algorithm to find suitable RGs for gene expression studies following brain injury or under other defined lesioning conditions. The two software algorithms used in the present study yielded similar results, with some notable differences: Because geNorm relies on pairwise comparison, it is more sensitive to co-regulated RGs. NormFinder, however, considers both the intra- and intergroup variation of RGs by calculating stability values. Thus, differences in ranking order can be explained by distinct mathematical calculations by the software tools. Therefore, we applied a consensus stability ranking analysis, which was used in previous RG validation studies56,57,58. In addition, the minimum number of RGs for accurate normalization was determined by using the recommended cut-off value of 0.1540. Earlier gene expression studies using brain injury models in the rodent, including the validation of suitable RGs, were mostly done in larger tissue samples, e.g., whole hippocampus13,14,16,32,59,60. However, RGs might be differentially expressed among distinct cell types because layers react differently to the injury. Therefore, we evaluated RGs in hippocampal layers by using the advantage of laser microdissection29,38.
Comparison of candidate reference genes
Initially, the expression of up to ten selected RGs was evaluated in two DG layers, i.e., the gcl and oml, to examine for abundance and variability of RGs. Of note, a few putative RGs had to be excluded from further validation analysis in the denervated oml, since they showed insufficient amplification because of low abundance expression, which could have influenced accurate qPCR quantification. Therefore, ten candidate RGs for the gcl and seven RGs for the oml were investigated for their expression stability after entorhinal denervation. Validation of RGs was performed at five individual time points after lesion, i.e., 1, 3, 7, 14 and 28 dpl, compared to control situation. Gapdh, which is one of the most used RGs in qPCR, has been discussed, on the one hand, as being suitable for proper normalization14,32,34,36,51,61, on the other hand, it was detected as being a less stable RG in other studies13,16,33,35,52,62. Based on our results, Gapdh belongs to the suitable RGs in the gcl as well as in the oml after entorhinal denervation, if the entire time-period after injury is considered. Also, Pgk1 was recognized as one of the most stable RGs in the gcl after entorhinal denervation. Similarly, it belongs to the most suitable RGs in the denervated oml, but except for 7 dpl, where it was found to be less stable compared to other RGs. In comparison, Actb was identified as least stable RG in the gcl at 1dpl and in the oml at 3 dpl, whereas at later lesion time points, it was recognized as one of the more suitable RGs in both layers. Previous studies on other brain injury models also observed an altered expression level of Actb, e.g., after traumatic brain injury31,36,59. Studying the controlled cortical impact model in mice, expression of Actb was increased at early time points after injury36. Our findings are supported by the study of Harris et al. (2009), which found that Actb was significantly upregulated at 2 dpl in the deafferented hippocampus, while at 7 and 15 dpl no apparent change of expression relative to control was detectable16. Interestingly, only Ppia (Cyclophilin A) demonstrated a relatively high expression stability at all lesion time points (2, 7 and 15 dpl) in this study. In our study, Ppia was recognized as a suitable RG in the denervated oml, too. In addition, various studies analyzed Ppia in other commonly used brain injury models and identified it as being one of the most stable RGs15,33,63,64. In contrast, B2m was recognized as a less stable RG in several studies12,14,34,36,51,59,60,63, but was postulated also as suitable RG in a few research papers31,35,65. In our study, B2m was identified as the least stable RG in the denervated oml at all time points analyzed following lesion. In contrary, Sdha was identified as the most stable RG in the oml throughout all time points after denervation. This is in accordance with previous brain injury studies, where Sdha was used as RG for accurate qPCR normalization14,64,65.
Accurate measurement of denervation-induced changes in Gfap and Aif1/Iba1 mRNA in the dentate gyrus using a normalization index
As proof-of-principle, we used the RGs found to be suitable for accurate qPCR normalization after denervation to analyze the expression of two mRNAs of astrocytes and microglia, i.e., Gfap and Aif1/Iba1, respectively. Both genes are upregulated in the denervated zone and reflect the strong glial response to entorhinal denervation26,66. Using immunofluorescence labeling for AIF1/IBA1 and GFAP, a major upregulation of these proteins in microglial cells as well as astrocytes, respectively, could also been seen at the protein level at 3 and 7 dpl in the denervated oml29. In previous studies, Gfap mRNA levels were measured in hippocampal samples using dot plot hybridization analysis44,67 or using laser microdissection in combination with qPCR using a single RG for qPCR normalization24. We now extended these earlier studies by analyzing the effect of different qPCR normalization strategies: target expression levels of Gfap and Aif1 mRNA were normalized in the denervated oml (1) against single candidate RGs, (2) against RG indices calculated by geNorm or NormFinder for each specific time-point following denervation, and (3) using the minimal number of RGs calculated for accurate qPCR normalization based on a consensus stability ranking for the entire lesion period and across the software algorithms used. Normalization against a single RG demonstrated a relatively high variation of mRNA expression levels for Gfap and Aif1 in the denervated oml. Significant upregulation of Gfap mRNA expression was found at 3, 7, 14 and 28 dpl depending on the RG that was used for normalization. Except for Pgk1, maximal upregulation of Gfap mRNA was detected at 7 dpl with expression levels varying considerably from 6.5- fold using B2m to 19.8- fold using Rpl13a. Similarly, a significant upregulation of Aif1 mRNA expression was found either at 3, 7 or 14 dpl depending on the RG used for qPCR normalization. Maximal upregulation of Aif1 mRNA was identified at 3 dpl using Gapdh, Pgk1, Rpl13a or Sdha. In contrast, normalization against Actb or B2m resulted in no significant expression changes of Aif1 mRNA. Of note, B2m was found to be the least stable RG in the oml at all time points analyzed following denervation. Similarly, Actb should also not be considered at earlier time points after denervation, but might be more suitable at later time points, which was also shown in the study of Harris and colleagues (2009). Using an index of suitable RGs according to the different software approaches by NormFinder and geNorm, a significant upregulation of Gfap mRNA at 3, 7 and 14 dpl and for Aif mRNA at 3 and 7 dpl was found. Importantly, a maximal upregulation of Aif1 mRNA was observed at 3 dpl, whereas maximal upregulation of Gfap mRNA upregulation was found at 7 dpl, which in both cases returned to lower levels at later time points after injury. Finally, qPCR normalization was performed using an index of the three most stable RGs, i.e., Sdha, Ppia and Gapdh, calculated based on a consensus stability ranking by including all lesion time-points and both software algorithms used. As a result, a significant upregulation of Aif1 mRNA and Gfap mRNA was found for both transcripts at 3, 7 and 14 dpl. Again, maximal upregulation of Aif mRNA was observed earlier (3 dpl) compared to Gfap mRNA (7 dpl). These results are in line with the early response of microglia cells, followed by the later reaction of astrocytes in rodents following entorhinal lesion42,66. Differences in the detailed time course of Gfap mRNA expression might be explained by anatomical species variations between mouse and rat or by distinct lesion techniques26. Our results demonstrate that normalization against non-validated or relatively non-stable RGs can lead to inconsistencies in qPCR expression analysis and, consequently, might lead to misinterpretation of real biological data. In contrast, normalization against an index of validated RGs determined by advanced software algorithms yields more accurate data. In addition, the precise harvesting of tissue, e.g., by using laser microdissection, helps to identify local changes after brain injury, which is of the essence to understand the sequence of events following damage. Based on our results, a normalization index with a minimal number of suitable RGs is sufficient for accurate qPCR quantification of target genes following entorhinal denervation. For a more precise analysis, we would recommend using time-point specific reference gene sets. However, the usage of a more broader normalization index of RGs calculated by consensus stability ranking for an entire lesion period is also highly appropriate and, thus, might be used in forthcoming studies, where more lesion time-points across different subregions are analyzed.
Changes in Map2 mRNA in denervated granule cells are revealed using laser microdissection and qPCR in combination with a normalization index
To demonstrate the usefulness of our algorithm for the detection of neuronal genes, we also analyzed changes in Map2 mRNA in granule cells. Microdissection of this neuronal layer is an excellent tool to harvest tissue enriched in granule cell mRNA, since granule cells are densely packed in this layer and greatly outnumber other neurons and glial cells. However, in contrast to glial cells, which react strongly to the degeneration of axon terminals and axons in the outer molecular layer, granule cells show only a very weak response. In fact, earlier studies performed in rats25,45,46 reported only very mild changes, which were an order of magnitude weaker than the changes found in glia and which were close or even under the threshold of detection using classical methods. Using laser microdissection and qPCR with a reference gene index established in the present study, we could, however, detect a mild upregulation of Map2 mRNA in mouse granule cells around day 3 to 7. This demonstrates that the algorithm proposed in this study also works with neurons and most likely any other cell type and allows for the identification of an index of stable and reliable reference genes.
In conclusion, we have shown here using the well-established and thoroughly studied entorhinal cortex lesion model, that tissue from different time points after lesion harvested with laser microdissection and analyzed with qPCR yields precise, reliable, and reproducible quantitative datasets. To achieve this precision, it was of the essence to identify an index of RGs that is (i) appropriate for the model and (ii) covers the entire time course postlesion. The algorithm reported here can easily be adapted and thus transferred to any other brain injury model, making qPCR data in the field of brain injury and regeneration more precise and reproducible.
Material and methods
Animals
For experimental analysis, adult male mice (3 to 4-month-old C57BL/6 J, Janvier) were used. Mice could survive 1, 3, 7, 14 or 28 days after entorhinal denervation. Experiments were carefully designed to examine control and lesioned animals of similar age.
Ethical approval
Animal care and experimental procedures were performed in agreement with the German law on the use of laboratory animals (animal welfare act; TierSchG) and approved by Regierungspräsidium Darmstadt (Hessen, Germany). The animal experiments described in this study were conducted in accordance with ARRIVE guidelines.
Entorhinal denervation and control of lesion quality
Unilateral transection of the perforant path was performed using a wire knife (David Kopf Instruments, USA) as described previously29. Correct placement of the wire knife cut was verified on horizontal brain sections (25 µm, Nissl staining) containing the lesion site and parts of the temporal dentate gyrus (DG). In addition, entorhinal denervation was verified on frontal hippocampal sections (25 µm) using Fluoro-Jade C (HistoChem Inc.) at early time points post lesion to monitor the appearance of degeneration products29,30,68 and counterstained with Hoechst 33242 (Invitrogen).
Immunofluorescence labeling
Mice were deeply anesthetized with an overdose of pentobarbital (300 mg/kg body weight) and transcardially perfused with 0.9% sodium chloride (NaCl) followed by 4% paraformaldehyde (PFA) in phosphate-buffered saline (pH 7.4). Brains were removed, post-fixed for 24 h in 4% PFA and sectioned in the coronal plane (40 µm) using a vibratome (VT1000 S, Leica Microsystems). Free-floating sections were incubated in a blocking buffer containing 0.5% Triton X-100 and 5% bovine serum albumin (BSA) in 0.05 M Tris-buffered saline (TBS) for 30 min at room temperature followed by incubation in the primary antibody (diluted in 0.1% Triton X-100 and 1% BSA in 0.05 M TBS) overnight at 4 °C. The following primary antibodies were used: rabbit anti-GFAP (immunogen: GFAP isolated from cow spinal cord; Z0334, Dako), mouse anti-NeuN (A60, immunogen: purified cell nuclei from mouse brain; MAB377, Chemicon) and anti-AIF1/IBA1 (1:100, Abcam, UK). After several washes, sections were incubated with the appropriate secondary Alexa-conjugated antibodies (1:2000, Invitrogen, USA) for several hours at room temperature and finally mounted in DAKO Fluorescent Mounting Medium (Dako).
Digital illustrations
Figures were prepared digitally using commercially available graphics software (Photoshop Adobe Inc.). Fluorescent images were acquired using a digital camera (Digital Sight DS-5Mc, Nikon, Germany) or confocal microscopy (Eclipse C1 Plus, Nikon). Single fluorescent images of the same section were digitally superimposed. The contrast, brightness and sharpness of images were adjusted as needed for each section. No additional image alteration was performed.
Laser microdissection
For layer-specific analysis of the granule cell layer (gcl) and outer molecular layer (oml) of the DG, prepared brains were embedded in tissue freezing medium and immediately flash-frozen in − 70 °C isopentane cooled by dry ice for 2 min. Until further processing, brains were transferred and stored at − 80 °C. For laser microdissection, 16 µm thin brain sections were cut using a cryostat (Leica Biosystems) and mounted on polyethylene naphthalene (PEN) membrane slides (Leica Microsystems). Sections were dried shortly at room temperature (RT), fixed in − 20 °C cold 75% and 100% ethanol (AppliChem) and stored at − 80 °C until further processing. Before laser microdissection, sections were thawed and stained quickly with 1% cresyl violet staining solution (Sigma-Aldrich) at RT and briefly dehydrated in 75% and 100% ethanol. Using a Leica LMD6500 system (Leica Microsystems), defined tissue portions of the gcl and oml of the DG from the same brain sections were collected separately in 50 µl lysis buffer (RNeasy Plus Micro Kit; Qiagen) with ß-mercaptoethanol. Tubes were refilled to 350 µl with lysis buffer, vortexed for 30 s and transferred immediately to − 80 °C until further processing. Total RNA was isolated using the RNeasy Plus Micro Kit (Qiagen) according to the manufacturer’s recommendations. RNA integrity was assessed using the Agilent 2100 Bioanalyzer system and Agilent RNA 6000 Pico Kit (Agilent Technologies). Only high RNA quality (RIN values > 8) samples were used for further processing.
Quantitative polymerase chain reaction
Total RNA from microdissected DG regions was reverse transcribed using HighCapacity cDNA Reverse Transcription Reagents Kit (Applied Biosystems) following the manufacturer’s recommendations. Quantitative PCR (qPCR) was performed using the StepOnePlus Real-Time PCR System (Applied Biosystems). qPCR conditions were carried out using EXPRESS SYBR GreenER qPCR SuperMix with Premixed Rox (Invitrogen) and a final primer concentration of 500 nM. Primers were designed to be intron-spanning to exclude amplification of genomic DNA. Ten potential reference genes (RGs) were selected for analysis: actin, beta (Actb), aminolevulinic acid synthase 1 (Alas1), beta-2 microglobulin (B2m), glyceraldehyd-3-phosphate dehydrogenase (Gapdh), hypoxanthine guanine phosphoribosyl transferase (Hprt), phosphoglycerate kinase I (Pgk1), peptidyl propyl isomerase A (Ppia), ribosomal protein L13A (Rpl13a), succinate dehydrogenase complex subunit A (Sdha) and transferrin receptor (Tfrc) (Table 3). Amplicon specificity was confirmed by melting curve analysis. In addition, PCR products were verified for product specificity and amplicon size with the Agilent 2100 Bioanalyzer system using Agilent DNA 1000 Chips (Agilent Technologies). Primer efficiencies and quantification cycle (Cq) values were calculated using LinRegPCR69,70.
Data analysis
To evaluate the gene expression stability of candidate RGs, two different software algorithms, i.e., NormFinder39 and geNorm40, were used according to the developer’s manuals. NormFinder calculates a stability value for each gene independently and matches combinations of gene pairs to compensate for variability, whereas geNorm calculates an average expression stability value (M), which is the average pairwise variation of a single gene to all other putative RGs and is the result of a stepwise exclusion of the least stable gene within the panel of RGs. In addition, to reach an unbiased consensus for both software algorithms, a comprehensive ranking analysis was performed using RankAggreg41. Minimum number of RGs for accurate normalization was calculated based on optimal gene rank lists by pairwise variation between two sequential normalization factors containing an increasing number of genes40. Based on this study, 0.15 was set as a cut-off, below the inclusion of an additional RG was not required for proper normalization. Statistical analysis of qPCR data was performed using GraphPad Prism 6 software. For one-way ANOVA, multiple comparisons between individual time-points and control were used in combination with Dunnett’s multiple comparisons test. P-values < 0.05 were considered statistically significant.
Data availability
The datasets used and/or analyzed during the current study available from the corresponding author on reasonable request.
References
Bustin, S. A., Benes, V., Nolan, T. & Pfaffl, M. W. Quantitative real-time RT-PCR–a perspective. J. Mol. Endocrinol. 34, 597–601. https://doi.org/10.1677/jme.1.01755 (2005).
Ginzinger, D. G. Gene quantification using real-time quantitative PCR: An emerging technology hits the mainstream. Exp. Hematol. 30, 503–512. https://doi.org/10.1016/s0301-472x(02)00806-8 (2002).
Kubista, M. et al. The real-time polymerase chain reaction. Mol. Asp. Med. 27, 95–125. https://doi.org/10.1016/j.mam.2005.12.007 (2006).
VanGuilder, H. D., Vrana, K. E. & Freeman, W. M. Twenty-five years of quantitative PCR for gene expression analysis. Biotechniques 44, 619–626. https://doi.org/10.2144/000112776 (2008).
Bustin, S. A. et al. The MIQE guidelines: Minimum information for publication of quantitative real-time PCR experiments. Clin. Chem. 55, 611–622. https://doi.org/10.1373/clinchem.2008.112797 (2009).
Derveaux, S., Vandesompele, J. & Hellemans, J. How to do successful gene expression analysis using real-time PCR. Methods 50, 227–230. https://doi.org/10.1016/j.ymeth.2009.11.001 (2010).
Chapman, J. R. & Waldenstrom, J. With reference to reference genes: A systematic review of endogenous controls in gene expression studies. PLoS ONE 10, e0141853. https://doi.org/10.1371/journal.pone.0141853 (2015).
Dheda, K. et al. Validation of housekeeping genes for normalizing RNA expression in real-time PCR. Biotechniques 37, 112–119. https://doi.org/10.2144/04371RR03 (2004).
Dheda, K. et al. The implications of using an inappropriate reference gene for real-time reverse transcription PCR data normalization. Anal. Biochem. 344, 141–143. https://doi.org/10.1016/j.ab.2005.05.022 (2005).
Huggett, J., Dheda, K., Bustin, S. & Zumla, A. Real-time RT-PCR normalisation; Strategies and considerations. Genes Immun. 6, 279–284. https://doi.org/10.1038/sj.gene.6364190 (2005).
Hellemans, J. & Vandesompele, J. Selection of reliable reference genes for RT-qPCR analysis. Methods Mol. Biol. 1160, 19–26. https://doi.org/10.1007/978-1-4939-0733-5_3 (2014).
Boda, E., Pini, A., Hoxha, E., Parolisi, R. & Tempia, F. Selection of reference genes for quantitative real-time RT-PCR studies in mouse brain. J. Mol. Neurosci. 37, 238–253. https://doi.org/10.1007/s12031-008-9128-9 (2009).
Bonefeld, B. E., Elfving, B. & Wegener, G. Reference genes for normalization: A study of rat brain tissue. Synapse 62, 302–309. https://doi.org/10.1002/syn.20496 (2008).
Cook, N. L., Vink, R., Donkin, J. J. & van den Heuvel, C. Validation of reference genes for normalization of real-time quantitative RT-PCR data in traumatic brain injury. J. Neurosci. Res. 87, 34–41. https://doi.org/10.1002/jnr.21846 (2009).
Zamani, A., Powell, K. L., May, A. & Semple, B. D. Validation of reference genes for gene expression analysis following experimental traumatic brain injury in a pediatric mouse model. Brain Res. Bull. 156, 43–49. https://doi.org/10.1016/j.brainresbull.2019.12.015 (2020).
Harris, J. L., Reeves, T. M. & Phillips, L. L. Injury modality, survival interval, and sample region are critical determinants of qRT-PCR reference gene selection during long-term recovery from brain trauma. J. Neurotrauma 26, 1669–1681. https://doi.org/10.1089/neu.2009-087510.1089/neu.2009.0875 (2009).
Caba, E. et al. Excitotoxic stimulation activates distinct pathogenic and protective expression signatures in the hippocampus. J Cell Mol. Med. 25, 9011–9027. https://doi.org/10.1111/jcmm.16864 (2021).
Kochanek, P. M. et al. Screening of biochemical and molecular mechanisms of secondary injury and repair in the brain after experimental blast-induced traumatic brain injury in rats. J. Neurotrauma 30, 920–937. https://doi.org/10.1089/neu.2013.2862 (2013).
Rickhag, M. et al. Comprehensive regional and temporal gene expression profiling of the rat brain during the first 24 h after experimental stroke identifies dynamic ischemia-induced gene expression patterns, and reveals a biphasic activation of genes in surviving tissue. J. Neurochem. 96, 14–29. https://doi.org/10.1111/j.1471-4159.2005.03508.x (2006).
Gall, C. & Lynch, G. Fiber architecture of the dentate gyrus following ablation of the entorhinal cortex in rats of different ages: Evidence for two forms of axon sprouting in the immature brain. Neuroscience 6, 903–910. https://doi.org/10.1016/0306-4522(81)90171-8 (1981).
Paul, M. H., Hildebrandt-Einfeldt, L., Beeg Moreno, V. J., Del Turco, D. & Deller, T. Maturation-dependent differences in the re-innervation of the denervated dentate gyrus by sprouting associational and commissural mossy cell axons in organotypic tissue cultures of entorhinal cortex and hippocampus. Front. Neuroanat. 15, 682383. https://doi.org/10.3389/fnana.2021.682383 (2021).
Ritzel, R. M. et al. Functional and transcriptional profiling of microglial activation during the chronic phase of TBI identifies an age-related driver of poor outcome in old mice. Geroscience 44, 1407–1440. https://doi.org/10.1007/s11357-022-00562-y (2022).
Burbach, G. J., Dehn, D., Nagel, B., Del Turco, D. & Deller, T. Laser microdissection of immunolabeled astrocytes allows quantification of astrocytic gene expression. J. Neurosci. Methods 138, 141–148. https://doi.org/10.1016/j.jneumeth.2004.03.022 (2004).
Burbach, G. J., Dehn, D., Del Turco, D., Staufenbiel, M. & Deller, T. Laser microdissection reveals regional and cellular differences in GFAP mRNA upregulation following brain injury, axonal denervation, and amyloid plaque deposition. Glia 48, 76–84. https://doi.org/10.1002/glia.20057 (2004).
Cotman, C., Gentry, C. & Steward, O. Synaptic replacement in the dentate gyrus after unilateral entorhinal lesion: Electron microscopic analysis of the extent of replacement of synapses by the remaining entorhinal cortex. J. Neurocytol. 6, 455–464. https://doi.org/10.1007/bf01178228 (1977).
Deller, T., Del Turco, D., Rappert, A. & Bechmann, I. Structural reorganization of the dentate gyrus following entorhinal denervation: Species differences between rat and mouse. Prog. Brain Res. 163, 501–528. https://doi.org/10.1016/S0079-6123(07)63027-1 (2007).
Deller, T. & Frotscher, M. Lesion-induced plasticity of central neurons: Sprouting of single fibres in the rat hippocampus after unilateral entorhinal cortex lesion. Prog. Neurobiol. 53, 687–727. https://doi.org/10.1016/s0301-0082(97)00044-0 (1997).
Steward, O. Lesion-induced synapse reorganization in the hippocampus of cats: Sprouting of entorhinal, commissural/associational, and mossy fiber projections after unilateral entorhinal cortex lesions, with comments on the normal organization of these pathways. Hippocampus 2, 247–268. https://doi.org/10.1002/hipo.450020305 (1992).
Del Turco, D., Schlaudraff, J., Bonin, M. & Deller, T. Upregulation of APP, ADAM10 and ADAM17 in the denervated mouse dentate gyrus. PLoS ONE 9, e84962. https://doi.org/10.1371/journal.pone.0084962 (2014).
Vuksic, M. et al. Unilateral entorhinal denervation leads to long-lasting dendritic alterations of mouse hippocampal granule cells. Exp. Neurol. 230, 176–185. https://doi.org/10.1016/j.expneurol.2011.04.011 (2011).
Swijsen, A., Nelissen, K., Janssen, D., Rigo, J. M. & Hoogland, G. Validation of reference genes for quantitative real-time PCR studies in the dentate gyrus after experimental febrile seizures. BMC Res. Notes 5, 685. https://doi.org/10.1186/1756-0500-5-685 (2012).
Meldgaard, M. et al. Validation of two reference genes for mRNA level studies of murine disease models in neurobiology. J. Neurosci. Methods 156, 101–110. https://doi.org/10.1016/j.jneumeth.2006.02.008 (2006).
Pernot, F., Dorandeu, F., Beaup, C. & Peinnequin, A. Selection of reference genes for real-time quantitative reverse transcription-polymerase chain reaction in hippocampal structure in a murine model of temporal lobe epilepsy with focal seizures. J. Neurosci. Res. 88, 1000–1008. https://doi.org/10.1002/jnr.22282 (2010).
Rhinn, H. et al. Housekeeping while brain’s storming Validation of normalizing factors for gene expression studies in a murine model of traumatic brain injury. BMC Mol. Biol. 9, 62. https://doi.org/10.1186/1471-2199-9-62 (2008).
Thal, S. C., Wyschkon, S., Pieter, D., Engelhard, K. & Werner, C. Selection of endogenous control genes for normalization of gene expression analysis after experimental brain trauma in mice. J. Neurotrauma 25, 785–794. https://doi.org/10.1089/neu.2007.0497 (2008).
Timaru-Kast, R., Herbig, E. L., Luh, C., Engelhard, K. & Thal, S. C. Influence of age on cerebral housekeeping gene expression for normalization of quantitative polymerase chain reaction after acute brain injury in mice. J. Neurotrauma 32, 1777–1788. https://doi.org/10.1089/neu.2014.3784 (2015).
Burbach, G. J., Dehn, D., Del Turco, D. & Deller, T. Quantification of layer-specific gene expression in the hippocampus: Effective use of laser microdissection in combination with quantitative RT-PCR. J. Neurosci. Methods 131, 83–91. https://doi.org/10.1016/s0165-0270(03)00232-2 (2003).
Del Turco, D. et al. Region-specific differences in amyloid precursor protein expression in the mouse hippocampus. Front. Mol. Neurosci. 9, 134. https://doi.org/10.3389/fnmol.2016.00134 (2016).
Andersen, C. L., Jensen, J. L. & Orntoft, T. F. Normalization of real-time quantitative reverse transcription-PCR data: A model-based variance estimation approach to identify genes suited for normalization, applied to bladder and colon cancer data sets. Cancer Res. 64, 5245–5250. https://doi.org/10.1158/0008-5472.CAN-04-0496 (2004).
Vandesompele, J. et al. Accurate normalization of real-time quantitative RT-PCR data by geometric averaging of multiple internal control genes. Genome Biol. 3, RESEARCH0034. https://doi.org/10.1186/gb-2002-3-7-research0034 (2002).
Pihur, V., Datta, S. & Datta, S. RankAggreg, an R package for weighted rank aggregation. BMC Bioinform. 10, 62. https://doi.org/10.1186/1471-2105-10-62 (2009).
Bechmann, I. & Nitsch, R. Astrocytes and microglial cells incorporate degenerating fibers following entorhinal lesion: A light, confocal, and electron microscopical study using a phagocytosis-dependent labeling technique. Glia 20, 145–154. https://doi.org/10.1002/(sici)1098-1136(199706)20:2%3c145::aid-glia6%3e3.0.co;2-8 (1997).
Hailer, N. P., Bechmann, I., Heizmann, S. & Nitsch, R. Adhesion molecule expression on phagocytic microglial cells following anterograde degeneration of perforant path axons. Hippocampus 7, 341–349. https://doi.org/10.1002/(SICI)1098-1063(1997)7:3%3c341::AID-HIPO8%3e3.0.CO;2-N (1997).
Steward, O., Torre, E. R., Phillips, L. L. & Trimmer, P. A. The process of reinnervation in the dentate gyrus of adult rats: Time course of increases in mRNA for glial fibrillary acidic protein. J. Neurosci. 10, 2373–2384 (1990).
Steward, O. Targeting of mRNAs to subsynaptic microdomains in dendrites. Curr. Opin. Neurobiol. 5, 55–61. https://doi.org/10.1016/0959-4388(95)80087-5 (1995).
Steward, O. & Wallace, C. S. mRNA distribution within dendrites: Relationship to afferent innervation. J. Neurobiol. 26, 447–449. https://doi.org/10.1002/neu.480260316 (1995).
Bustin, S. & Nolan, T. Talking the talk, but not walking the walk: RT-qPCR as a paradigm for the lack of reproducibility in molecular research. Eur. J. Clin. Invest 47, 756–774. https://doi.org/10.1111/eci.12801 (2017).
Bustin, S. A. Why the need for qPCR publication guidelines?–The case for MIQE. Methods 50, 217–226. https://doi.org/10.1016/j.ymeth.2009.12.006 (2010).
Kozera, B. & Rapacz, M. Reference genes in real-time PCR. J. Appl. Genet. 54, 391–406. https://doi.org/10.1007/s13353-013-0173-x (2013).
Ohl, F. et al. Gene expression studies in prostate cancer tissue: Which reference gene should be selected for normalization?. J. Mol. Med. (Berl) 83, 1014–1024. https://doi.org/10.1007/s00109-005-0703-z (2005).
Langnaese, K., John, R., Schweizer, H., Ebmeyer, U. & Keilhoff, G. Selection of reference genes for quantitative real-time PCR in a rat asphyxial cardiac arrest model. BMC Mol. Biol. 9, 53. https://doi.org/10.1186/1471-2199-9-53 (2008).
Nelissen, K., Smeets, K., Mulder, M., Hendriks, J. J. & Ameloot, M. Selection of reference genes for gene expression studies in rat oligodendrocytes using quantitative real time PCR. J. Neurosci. Methods 187, 78–83. https://doi.org/10.1016/j.jneumeth.2009.12.018 (2010).
Santos, A. R. & Duarte, C. B. Validation of internal control genes for expression studies: Effects of the neurotrophin BDNF on hippocampal neurons. J. Neurosci. Res. 86, 3684–3692. https://doi.org/10.1002/jnr.21796 (2008).
Jasmin, S. B. et al. Differential regulation of ABCA1 and ABCG1 gene expressions in the remodeling mouse hippocampus after entorhinal cortex lesion and liver-X receptor agonist treatment. Brain Res. 1562, 39–51. https://doi.org/10.1016/j.brainres.2014.03.016 (2014).
Rappert, A. et al. CXCR3-dependent microglial recruitment is essential for dendrite loss after brain lesion. J. Neurosci. 24, 8500–8509. https://doi.org/10.1523/JNEUROSCI.2451-04.2004 (2004).
Gholami, K., Loh, S. Y., Salleh, N., Lam, S. K. & Hoe, S. Z. Selection of suitable endogenous reference genes for qPCR in kidney and hypothalamus of rats under testosterone influence. PLoS ONE 12, e0176368. https://doi.org/10.1371/journal.pone.0176368 (2017).
Mallona, I., Lischewski, S., Weiss, J., Hause, B. & Egea-Cortines, M. Validation of reference genes for quantitative real-time PCR during leaf and flower development in Petunia hybrida. BMC Plant Biol. 10, 4. https://doi.org/10.1186/1471-2229-10-4 (2010).
Wang, J. J., Han, S., Yin, W., Xia, X. & Liu, C. Comparison of reliable reference genes following different hormone treatments by various algorithms for qRT-PCR analysis of metasequoia. Int. J. Mol. Sci. https://doi.org/10.3390/ijms20010034 (2018).
Marques, T. E. et al. Validation of suitable reference genes for expression studies in different pilocarpine-induced models of mesial temporal lobe epilepsy. PLoS ONE 8, e71892. https://doi.org/10.1371/journal.pone.0071892 (2013).
Sadangi, C., Rosenow, F. & Norwood, B. A. Validation of reference genes for quantitative gene expression analysis in experimental epilepsy. J. Neurosci. Res. 95, 2357–2366. https://doi.org/10.1002/jnr.24089 (2017).
Bangaru, M. L., Park, F., Hudmon, A., McCallum, J. B. & Hogan, Q. H. Quantification of gene expression after painful nerve injury: Validation of optimal reference genes. J. Mol. Neurosci. 46, 497–504. https://doi.org/10.1007/s12031-011-9628-x (2012).
Xu, D. et al. Identifying suitable reference genes for developing and injured mouse CNS tissues. Dev. Neurobiol. 78, 39–50. https://doi.org/10.1002/dneu.22558 (2018).
Otto, E. et al. Validation of reference genes for expression analysis in a murine trauma model combining traumatic brain injury and femoral fracture. Sci. Rep. 10, 15057. https://doi.org/10.1038/s41598-020-71895-x (2020).
Schwarz, A. P. et al. Reference gene validation in the brain regions of young rats after pentylenetetrazole-induced seizures. Biomedicines https://doi.org/10.3390/biomedicines8080239 (2020).
Gubern, C. et al. Validation of housekeeping genes for quantitative real-time PCR in in-vivo and in-vitro models of cerebral ischaemia. BMC Mol. Biol. 10, 57. https://doi.org/10.1186/1471-2199-10-57 (2009).
Hailer, N. P., Grampp, A. & Nitsch, R. Proliferation of microglia and astrocytes in the dentate gyrus following entorhinal cortex lesion: A quantitative bromodeoxyuridine-labelling study. Eur. J. Neurosci. 11, 3359–3364. https://doi.org/10.1046/j.1460-9568.1999.00808.x (1999).
Steward, O., Kelley, M. S. & Torre, E. R. The process of reinnervation in the dentate gyrus of adult rats: Temporal relationship between changes in the levels of glial fibrillary acidic protein (GFAP) and GFAP mRNA in reactive astrocytes. Exp. Neurol. 124, 167–183. https://doi.org/10.1006/exnr.1993.1187 (1993).
Schmued, L. C., Stowers, C. C., Scallet, A. C. & Xu, L. Fluoro-Jade C results in ultra high resolution and contrast labeling of degenerating neurons. Brain Res. 1035, 24–31. https://doi.org/10.1016/j.brainres.2004.11.054 (2005).
Ramakers, C., Ruijter, J. M., Deprez, R. H. & Moorman, A. F. Assumption-free analysis of quantitative real-time polymerase chain reaction (PCR) data. Neurosci. Lett. 339, 62–66. https://doi.org/10.1016/s0304-3940(02)01423-4 (2003).
Ruijter, J. M. et al. Amplification efficiency: linking baseline and bias in the analysis of quantitative PCR data. Nucleic Acids Res. 37, e45. https://doi.org/10.1093/nar/gkp045 (2009).
Acknowledgements
We thank Charlotte Nolte-Uhl and Heike Korff for technical support.
Funding
Open Access funding enabled and organized by Projekt DEAL. This work was supported by Deutsche Forschungsgemeinschaft (DFG CRC 1080 to T.D.).
Author information
Authors and Affiliations
Contributions
J.S., M.P. acquired data, J.S. and D.D.T. analyzed data, T.D. and D.D.T. conceived the study, D.D.T. supervised the study, J.S., T.D. and D.D.T. wrote the manuscript with contributions from all authors. All authors were involved in data interpretation and critically revising the manuscript. All authors read and approved the final manuscript.
Corresponding author
Ethics declarations
Competing interests
TD received funding from Novartis for a lecture on human brain anatomy. The other authors declare that the research was conducted in the absence of any commercial or financial relationships that could be constructed as a potential conflict of interest.
Additional information
Publisher's note
Springer Nature remains neutral with regard to jurisdictional claims in published maps and institutional affiliations.
Supplementary Information
Rights and permissions
Open Access This article is licensed under a Creative Commons Attribution 4.0 International License, which permits use, sharing, adaptation, distribution and reproduction in any medium or format, as long as you give appropriate credit to the original author(s) and the source, provide a link to the Creative Commons licence, and indicate if changes were made. The images or other third party material in this article are included in the article's Creative Commons licence, unless indicated otherwise in a credit line to the material. If material is not included in the article's Creative Commons licence and your intended use is not permitted by statutory regulation or exceeds the permitted use, you will need to obtain permission directly from the copyright holder. To view a copy of this licence, visit http://creativecommons.org/licenses/by/4.0/.
About this article
Cite this article
Schlaudraff, J., Paul, M.H., Deller, T. et al. Precise measurement of gene expression changes in mouse brain areas denervated by injury. Sci Rep 12, 22530 (2022). https://doi.org/10.1038/s41598-022-26228-5
Received:
Accepted:
Published:
DOI: https://doi.org/10.1038/s41598-022-26228-5
Comments
By submitting a comment you agree to abide by our Terms and Community Guidelines. If you find something abusive or that does not comply with our terms or guidelines please flag it as inappropriate.