Abstract
The X-chromosome has long been hypothesized to have a disproportionate influence on the brain based on its enrichment for genes that are expressed in the brain and associated with intellectual disability. Here, we verify this hypothesis through partitioned heritability analysis of X-chromosome influences (XIs) on human brain anatomy in 32,256 individuals from the UK Biobank. We first establish evidence for dosage compensation in XIs on brain anatomy—reflecting larger XIs in males compared to females, which correlate with regional sex-biases in neuroanatomical variance. XIs are significantly larger than would be predicted from X-chromosome size for the relative surface area of cortical systems supporting attention, decision-making and motor control. Follow-up association analyses implicate X-linked genes with pleiotropic effects on cognition. Our study reveals a privileged role for the X-chromosome in human neurodevelopment and urges greater inclusion of this chromosome in future genome-wide association studies.
This is a preview of subscription content, access via your institution
Access options
Access Nature and 54 other Nature Portfolio journals
Get Nature+, our best-value online-access subscription
$29.99 / 30 days
cancel any time
Subscribe to this journal
Receive 12 print issues and online access
$209.00 per year
only $17.42 per issue
Buy this article
- Purchase on Springer Link
- Instant access to full article PDF
Prices may be subject to local taxes which are calculated during checkout




Similar content being viewed by others
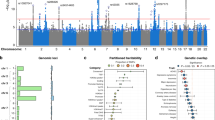
Data availability
No data were collected as part of this study. All genetic and phenotypic data (including neuroimaging data) are available from the UKB via their standard data access procedure, as described at https://www.ukbiobank.ac.uk/register-apply. The Neurosynth data for topic-based meta-analyses are available at https://neurosynth.org/analyses/topics/. The PsychENCODE data for developmental expression analyses are available at http://development.psychencode.org. XWA summary statistics are available at https://osf.io/cqxdj/.
Code availability
No custom software was used in this study. All relevant software and code are described in the text and can be found at the URLs or references cited.
References
Grasby, K. L. et al. The genetic architecture of the human cerebral cortex. Science 367, eaay6690 (2020).
Biton, A. et al. Polygenic architecture of human neuroanatomical diversity. Cereb. Cortex 30, 2307–2320 (2020).
Zhao, B. et al. Genome-wide association analysis of 19,629 individuals identifies variants influencing regional brain volumes and refines their genetic co-architecture with cognitive and mental health traits. Nat. Genet. 51, 1637–1644 (2019).
Smith, S. M. et al. An expanded set of genome-wide association studies of brain imaging phenotypes in UK Biobank. Nat. Neurosci. 24, 737–745 (2021).
Wise, A. L., Gyi, L. & Manolio, T. A. eXclusion: toward integrating the X chromosome in genome-wide association analyses. Am. J. Hum. Genet. 92, 643–647 (2013).
Lyon, M. F. Gene action in the X-chromosome of the mouse (Mus musculus L.). Nature 190, 372–373 (1961).
Nguyen, D. K. & Disteche, C. M. Dosage compensation of the active X chromosome in mammals. Nat. Genet. 38, 47–53 (2006).
Zechner, U. et al. A high density of X-linked genes for general cognitive ability: a run-away process shaping human evolution? Trends Genet. 17, 697–701 (2001).
Raznahan, A. et al. Globally divergent but locally convergent X- and Y-chromosome influences on cortical development. Cereb. Cortex 26, 70–79 (2016).
Yang, J. et al. Genome partitioning of genetic variation for complex traits using common SNPs. Nat. Genet. 43, 519–525 (2011).
Yang, J., Lee, S. H., Goddard, M. E. & Visscher, P. M. GCTA: a tool for genome-wide complex trait analysis. Am. J. Hum. Genet. 88, 76–82 (2011).
Fischl, B. et al. Whole brain segmentation: automated labeling of neuroanatomical structures in the human brain. Neuron 33, 341–355 (2002).
Fischl, B. et al. Automatically parcellating the human cerebral cortex. Cereb. Cortex 14, 11–22 (2004).
Glasser, M. F. et al. A multi-modal parcellation of human cerebral cortex. Nature 536, 171–178 (2016).
Lerch, J. P. et al. Studying neuroanatomy using MRI. Nat. Neurosci. 20, 314–326 (2017).
Hofer, E. et al. Genetic correlations and genome-wide associations of cortical structure in general population samples of 22,824 adults. Nat. Commun. 11, 4796 (2020).
Matoba, N., Love, M. I. & Stein, J. L. Evaluating brain structure traits as endophenotypes using polygenicity and discoverability. Hum. Brain Mapp. https://doi.org/10.1002/hbm.25257 (2020).
Forde, N. J. et al. Sex differences in variability of brain structure across the lifespan. Cereb. Cortex 30, 5420–5430 (2020).
Ritchie, S. J. et al. Sex differences in the adult human brain: evidence from 5216 UK Biobank participants. Cereb. Cortex 28, 2959–2975 (2018).
Finucane, H. K. et al. Partitioning heritability by functional annotation using genome-wide association summary statistics. Nat. Genet. 47, 1228–1235 (2015).
van der Meer, D. et al. Quantifying the polygenic architecture of the human cerebral cortex: extensive genetic overlap between cortical thickness and surface area. Cereb. Cortex 30, 5597–5603 (2020).
Evans, L. M. et al. Comparison of methods that use whole genome data to estimate the heritability and genetic architecture of complex traits. Nat. Genet. 50, 737–745 (2018).
Ge, T. et al. Phenome-wide heritability analysis of the UK Biobank. PLoS Genet. 13, e1006711 (2017).
Kharabian Masouleh, S., Eickhoff, S. B., Hoffstaedter, F., Genon, S. & Alzheimer’s Disease Neuroimaging Initiative. Empirical examination of the replicability of associations between brain structure and psychological variables. eLife 8, e43464 (2019).
Romero-Garcia, R., Atienza, M., Clemmensen, L. H. & Cantero, J. L. Effects of network resolution on topological properties of human neocortex. NeuroImage 59, 3522–3532 (2012).
Alexander-Bloch, A. F. et al. On testing for spatial correspondence between maps of human brain structure and function. NeuroImage 178, 540–551 (2018).
Váša, F. et al. Adolescent tuning of association cortex in human structural brain networks. Cereb. Cortex 28, 281–294 (2017).
Yeo, B. T. T. et al. The organization of the human cerebral cortex estimated by intrinsic functional connectivity. J. Neurophysiol. 106, 1125–1165 (2011).
Yarkoni, T., Poldrack, R. A., Nichols, T. E., Van Essen, D. C. & Wager, T. D. Large-scale automated synthesis of human functional neuroimaging data. Nat. Methods 8, 665–670 (2011).
Rubin, T. N. et al. Decoding brain activity using a large-scale probabilistic functional-anatomical atlas of human cognition. PLoS Comput. Biol. 13, e1005649 (2017).
Sjöstedt, E. et al. An atlas of the protein-coding genes in the human, pig, and mouse brain. Science 367, eaay5947 (2020).
Deciphering Developmental Disorders Study. Prevalence and architecture of de novo mutations in developmental disorders. Nature 542, 433–438 (2017).
Martin, H. C. et al. The contribution of X-linked coding variation to severe developmental disorders. Nat. Commun. 12, 627 (2021).
Li, M. et al. Integrative functional genomic analysis of human brain development and neuropsychiatric risks. Science 362, eaat7615 (2018).
Reinhold, K. & Engqvist, L. The variability is in the sex chromosomes: brief communication. Evolution 67, 3662–3668 (2013).
Young, A. I. Solving the missing heritability problem. PLoS Genet. 15, e1008222 (2019).
Schmitt, J. E. et al. A comprehensive quantitative genetic analysis of cerebral surface area in youth. J. Neurosci. 39, 3028–3040 (2019).
Cadwell, C. R., Bhaduri, A., Mostajo-Radji, M. A., Keefe, M. G. & Nowakowski, T. J. Development and arealization of the cerebral cortex. Neuron 103, 980–1004 (2019).
Woodbury-Smith, M. et al. Mutations in RAB39B in individuals with intellectual disability, autism spectrum disorder, and macrocephaly. Mol. Autism 8, 59 (2017).
Kenwrick, S., Watkins, A. & De Angelis, E. Neural cell recognition molecule L1: relating biological complexity to human disease mutations. Hum. Mol. Genet. 9, 879–886 (2000).
Graves, J. A. M., Gécz, J. & Hameister, H. Evolution of the human X—a smart and sexy chromosome that controls speciation and development. Cytogenet. Genome Res. 99, 141–145 (2002).
Vallender, E. J. & Lahn, B. T. How mammalian sex chromosomes acquired their peculiar gene content. Bioessays 26, 159–169 (2004).
Balaton, B. P. et al. Derivation of consensus inactivation status for X-linked genes from genome-wide studies. Biol. Sex Differ. 6, 35 (2015).
Sudlow, C. et al. UK Biobank: an open access resource for identifying the causes of a wide range of complex diseases of middle and old age. PLoS Med. 12, e1001779 (2015).
Alfaro-Almagro, F. et al. Image processing and quality control for the first 10,000 brain imaging datasets from UK Biobank. NeuroImage 166, 400–424 (2018).
Glasser, M. F. et al. The minimal preprocessing pipelines for the Human Connectome Project. NeuroImage 80, 105–124 (2013).
Fischl, B. FreeSurfer. NeuroImage 62, 774–781 (2012).
Ségonne, F. et al. A hybrid approach to the skull stripping problem in MRI. NeuroImage 22, 1060–1075 (2004).
Dale, A. M., Fischl, B. & Sereno, M. I. Cortical surface-based analysis. I. Segmentation and surface reconstruction. NeuroImage 9, 179–194 (1999).
Fischl, B., Sereno, M. I. & Dale, A. M. Cortical surface-based analysis. II. Inflation, flattening, and a surface-based coordinate system. NeuroImage 9, 195–207 (1999).
Desikan, R. S. et al. An automated labeling system for subdividing the human cerebral cortex on MRI scans into gyral based regions of interest. NeuroImage 31, 968–980 (2006).
Bycroft, C. et al. The UK Biobank resource with deep phenotyping and genomic data. Nature 562, 203–209 (2018).
O’Connell, J. et al. Haplotype estimation for biobank-scale data sets. Nat. Genet. 48, 817–820 (2016).
Chang, C. C. et al. Second-generation PLINK: rising to the challenge of larger and richer datasets. Gigascience 4, 7 (2015).
Burnham, K. P. & Anderson, D. R. Model Selection and Multimodel Inference: A Practical Information-Theoretic Approach. (Springer Science & Business Media, 2002).
Finucane, H. K. et al. Heritability enrichment of specifically expressed genes identifies disease-relevant tissues and cell types. Nat. Genet. 50, 621–629 (2018).
Speed, D., Hemani, G., Johnson, M. R. & Balding, D. J. Improved heritability estimation from genome-wide SNPs. Am. J. Hum. Genet. 91, 1011–1021 (2012).
Yang, J. et al. Common SNPs explain a large proportion of the heritability for human height. Nat. Genet. 42, 565–569 (2010).
Seidlitz, J. et al. Morphometric similarity networks detect microscale cortical organization and predict inter-individual cognitive variation. Neuron 97, 231–247.e7 (2018).
Poldrack, R. A. et al. Discovering relations between mind, brain, and mental disorders using topic mapping. PLoS Comput. Biol. 8, e1002707 (2012).
Winkler, T. W. et al. Quality control and conduct of genome-wide association meta-analyses. Nat. Protoc. 9, 1192–1212 (2014).
Mallard, T. T. et al. Multivariate GWAS of psychiatric disorders and their cardinal symptoms reveal two dimensions of cross-cutting genetic liabilities. Preprint at bioRxiv https://doi.org/10.1101/603134 (2020).
Mallard, T. T. et al. tem-level genome-wide association study of the Alcohol Use Disorders Identification Test in three population-based cohorts. Am. J. Psychiatry https://doi.org/10.1176/appi.ajp.2020.20091390 (2021).
de Leeuw, C. A., Mooij, J. M., Heskes, T. & Posthuma, D. MAGMA: generalized gene-set analysis of GWAS data. PLoS Comput. Biol. 11, e1004219 (2015).
Watanabe, K., Taskesen, E., van Bochoven, A. & Posthuma, D. Functional mapping and annotation of genetic associations with FUMA. Nat. Commun. 8, 1826 (2017).
Kuleshov, M. V. et al. Enrichr: a comprehensive gene set enrichment analysis web server 2016 update. Nucleic Acids Res. 44, W90–W97 (2016).
Piñero, J. et al. The DisGeNET knowledge platform for disease genomics: 2019 update. Nucleic Acids Res. 48, D845–D855 (2020).
Langfelder, P. & Horvath, S. WGCNA: an R package for weighted correlation network analysis. BMC Bioinformatics 9, 559 (2008).
Acknowledgements
This research was supported by the Intramural Research Program of the National Institute of Mental Health (NIH annual report number ZIA MH002949-04), and conducted using the UKB resource under application number 22875. We thank the UKB participants for making this study possible.
Author information
Authors and Affiliations
Contributions
T.T.M. and A.R. conceived and designed the study. A.R. oversaw the study. T.T.M. was the lead analyst responsible for conducting all genetically informative analyses. S.L. conducted the topographic annotation analyses and spin tests. J.S. assisted with the expression analyses in the PsychENCODE dataset. T.T.M. and S.L. prepared the figures and tables. T.T.M. prepared the genetic data, while J.S., Z.M., D.M. and A.T. prepared the neuroimaging data. T.T.M. and A.R. led the writing of the manuscript, and all authors provided valuable feedback and advice during its preparation.
Corresponding author
Ethics declarations
Competing interests
The authors declare no competing interests.
Additional information
Peer review information Nature Neuroscience thanks Jason Stein and the other, anonymous, reviewer(s) for their contribution to the peer review of this work.
Publisher’s note Springer Nature remains neutral with regard to jurisdictional claims in published maps and institutional affiliations.
Extended data
Extended Data Fig. 1 Distributions of X-linked heritability in males and females for regional measures of brain anatomy.
Raincloud-style plot that considers all regional measures of brain anatomy where the full dosage compensation model was the best fitting model (n = 1,840 sex-stratified heritability estimates). X-linked heritability for males and females was independently estimated within each sex in a model-naïve manner (Methods). The median X-linked heritability in males is 2.37 times larger (bootstrapped 95% CI = 1.70-3.25) than the median X-linked heritability in females (two-sided Wilcoxon P = 1.18e-23). Summary statistics for males: minimum = 1e-6, Q1 = 3.98e-4, median = 6.34e-3, Q3 = 1.3e-2, maximum = 3.64e-2. Summary statistics for females: minimum = 1e-6, Q1 = 1e-6, median = 2.67e-3, Q3 = 7.48e-3, maximum = 3.34e-2.
Extended Data Fig. 2 Regional patterning of X-chromosome dosage compensation for cortical volume (CV).
a, Categorical map showing the best-fitting X-chromosome dosage compensation model for each cortical region. NDC = no dosage compensation, EV = equal variance, FDC = full dosage compensation. b, Continuous map showing regional variation in the strength of statistical evidence for X-chromosome dosage compensation on CV, as indexed by ΔAICDC (Methods). ΔAICDC equals zero when the EV model fits best, and departures from zero reflect quantitative evidence for either NDC or FDC. Values ≤ -10 are indicative of strong evidence for NDC while values ≥ +10 are indicative of strong evidence for FDC. Any values less than −10 or greater than 10 were set to −10 and 10, respectively, for illustration.
Extended Data Fig. 3 Regional patterning of X-chromosome dosage compensation for cortical thickness (CT).
a, Categorical map showing the best-fitting X-chromosome dosage compensation model for each cortical region. NDC = no dosage compensation, EV = equal variance, FDC = full dosage compensation. b, Continuous map showing regional variation in the strength of statistical evidence for X-chromosome dosage compensation on CT, as indexed by ΔAICDC (Methods). ΔAICDC equals zero when the EV model fits best, and departures from zero reflect quantitative evidence for either NDC or FDC. Values ≤ -10 are indicative of strong evidence for NDC while values ≥ +10 are indicative of strong evidence for FDC. Any values less than −10 or greater than 10 were set to −10 and 10, respectively, for illustration.
Extended Data Fig. 4 Regional patterning of X-chromosome influences (XIs) on cortical volume (CV).
a, Continuous map of total SNP heritability \((h_{\mathrm{g}}^2)\) for regional CV. b, Continuous map of X-linked heritability \((h_{\mathrm{x}}^2)\) for regional CV. c, Continuous map of regional ratio between the observed proportion of total heritability assigned to the X-chromosome, and the expected proportion from X-chromosome size (XI ratio). Values greater than one reflect enriched XIs and values less than one reflect depleted XIs (Methods).
Extended Data Fig. 5 Regional patterning of X-chromosome influences (XIs) on cortical thickness (CT).
a, Continuous map of total SNP heritability \((h_{\mathrm{g}}^2)\) for regional CT. b, Continuous map of X-linked heritability \((h_{\mathrm{x}}^2)\) for regional CT. c, Continuous map of regional ratio between the observed proportion of total heritability assigned to the X-chromosome, and the expected proportion from X-chromosome size (XI ratio). Values greater than one reflect enriched XIs and values less than one reflect depleted XIs (Methods).
Extended Data Fig. 6 Comparison of the enrichment ratio for X-chromosome influences (XIs) on three morphological features of the cortex.
a, Ranked scatter plot of the regional XI enrichment ratio for cortical surface area, volume, and thickness, where the 358 cortical regions are ranked by their XI enrichment ratio for surface area. b,c,d, Scatter plots of the regional XI enrichment ratio for (b) surface area and volume, (c) thickness and volume, and (d) thickness and surface area.
Extended Data Fig. 7 Comparison of the enrichment Z statistic for X-chromosome influences (XIs) on three morphological features of the cortex.
a, Ranked scatter plot of the regional XI enrichment Z-statistic for cortical surface area, volume, and thickness, where the 358 cortical regions are ranked by their XI enrichment Z-statistic for surface area. b,c,d, Scatter plots of the regional XI enrichment Z-statistic for (b) surface area and volume, (c) thickness and volume, and (d) thickness and surface area.
Extended Data Fig. 8 Statistically-significant depletion and enrichment of X-chromosome influences (XIs) on three morphological features of the cortex.
a,b,c, Categorical maps showing the regions of statistically significant depletion or enrichment of XIs (after correction for multiple comparisons) on (a) cortical surface area, (b) cortical volume, and (c) cortical thickness.
Extended Data Fig. 9 Sensitivity analyses for observed X-chromosome influences (XIs) on cortical surface area (SA).
a, Cross-region of interest (ROI) correlation in the XI Z-statistic for SA between first and second releases of UKB neuroimaging data. b, Distribution of cross-ROI correlations in the XI Z-statistic for SA for 100 split-halves of the UKB dataset. c, Cross-ROI correlation in the XI Z-statistic for SA between UKB analyses differing in minor allele frequency (MAF) threshold. d, Continuous map of XI enrichment ratio for SA as computed in the 308-parcellation. e, Observed cross-ROI correlation (dashed line) in the 308-parcellation between XI Z-statistics computed in the 308-parcellation vs. those projected into the 308-parcellation from values computed in the primary HCP atlas. Density plot shows a null distribution of these correlations from 10,000 rotational permutations ‘spins’ of the 308 map. The observed correlation (two-sided Pearson’s r = .76) is significantly elevated relative to this null distribution (Pspin < 1e-4) (Methods).
Extended Data Fig. 10 X-linked SNPs associated with regional surface area phenotypes with enriched X-chromosome influences (XIs).
An X-chromosome-wide Manhattan plot of the minimum P-value for each SNP across all tested phenotypes. The x-axis refers to the chromosomal position, the y-axis refers to the significance of the SNP-based association test as a -log10 P-value, the horizontal dashed line denotes genome-wide significance (P = 5e-8), and the horizontal two-dashed line denotes a more conservative significance threshold accounting for all tested phenotypes (P = 8.93e-10).
Supplementary information
Supplementary Tables
Supplementary Tables 1–7.
Rights and permissions
About this article
Cite this article
Mallard, T.T., Liu, S., Seidlitz, J. et al. X-chromosome influences on neuroanatomical variation in humans. Nat Neurosci 24, 1216–1224 (2021). https://doi.org/10.1038/s41593-021-00890-w
Received:
Accepted:
Published:
Issue Date:
DOI: https://doi.org/10.1038/s41593-021-00890-w
This article is cited by
-
Females exhibit smaller volumes of brain activation and lower inter-subject variability during motor tasks
Scientific Reports (2023)
-
Sex differences in number of X chromosomes and X-chromosome inactivation in females promote greater variability in hearing among males
Biology of Sex Differences (2022)
-
Shared genetic architecture between schizophrenia and subcortical brain volumes implicates early neurodevelopmental processes and brain development in childhood
Molecular Psychiatry (2022)
-
In search of sex-related mediators of affective illness
Biology of Sex Differences (2021)