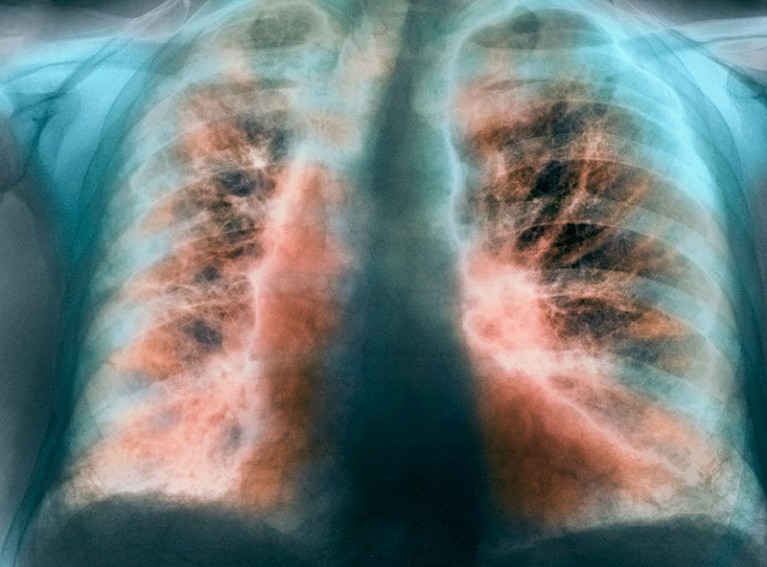
A drug candidate identified using AI is now in clinical trials to treat idiopathic pulmonary fibrosis, a condition that causes scarring of the lungs.Credit: Science Photo Library
For decades, researchers have sought a fast track to drug discovery. Yet the process has been getting slower, as well as riskier and more costly. It generally takes 12–15 years from the initiation of a discovery programme to the point at which national drug-regulatory agencies grant marketing approval1. Some nine in ten drugs that enter clinical trials don’t get approved. Estimates suggest it costs about US$2.5 billion to bring a drug to market2, after accounting for the costs of successful and failed programmes across the board.
Although established pharmaceutical companies are struggling to innovate, claims are mounting that the disruptor du jour — generative artificial intelligence (AI) — is radically shortening the stages that come before clinical trials, when drugs are tested in people. But is it?
Science and the new age of AI: a Nature special
Drug development involves a number of specific steps. It often starts with the identification of a biological target responsible for a disease — possibilities include DNA, RNA, a protein receptor or an enzyme — and then screening for molecules that might interact with it. This is called the ‘discovery’ stage. For most drugs, the resulting candidates are small molecules, and medicinal chemists work to improve their activity and reduce any associated problems. If this is successful, the researchers develop a lead molecule to take into the next stage — preclinical testing. This involves tests that help scientists to understand how a drug candidate is transported, broken down and excreted by an animal’s body. The work also answers questions of safety and dosage, before a drug is approved for clinical trials.
Together, the discovery and preclinical stages take an average of six years1. In February 2022, researchers at Boston Consulting Group (BCG), based in Massachusetts, reported an examination of the research pipelines of 20 relatively new AI-intensive pharmaceutical companies between 2010 and 2021. Using publicly available data, the BCG group determined that about 15 drug candidates had reached clinical-trial stage. It then reconstructed the development timelines of eight of these3. The consultants found that all eight had reached clinical trials within a decade. Five had done so in less than the historical average time.
Another report, released in June and co-authored by BCG and the research funder Wellcome, says that AI could yield “time and cost savings of at least 25–50%” in drug discovery up to the preclinical stage (see go.nature.com/46nkwcm).
AI can help to speed up drug discovery — but only if we give it the right data
One pharmaceutical company, Insilico Medicine, which is jointly headquartered in New York City and Hong Kong, announced last February that it had progressed to phase I clinical trials with an AI-designed drug candidate. The molecule targets idiopathic pulmonary fibrosis, a serious disease that leads to untreatable lung scarring. The drug candidate had completed the discovery and preclinical stages in just 30 months. In June, the company began phase II trials, which study how well a candidate works in more detail.
These are noteworthy developments, and they will no doubt drive investment. Although the technology is still relatively young, the 20 AI-intensive companies in BCG’s 2022 analysis already had 158 drug candidates in discovery and preclinical development. That compared with 333 at the world’s 20 biggest pharma companies as measured by revenue.
However, these claims have come from the companies themselves. Until they can be independently verified, some caution is in order. The findings need to be published in the peer-reviewed literature and authenticated by researchers unaffiliated with the companies involved.
And there are other challenges to realizing the benefits of AI. Systems based on generative AI that suggest candidate drug molecules work by using patterns learnt from training data to generate new data with similar characteristics. This can cause problems. When responding to user questions, the chatbot ChatGPT sometimes fabricates answers; in drug discovery, the equivalent problem leads it to suggest substances that are impossible to make. Such problems can be overcome by hand coding knowledge of molecular structures, and with the help of other AI tools.
AI and science: what 1,600 researchers think
Ultimately, for AI systems to learn and improve, someone needs to make and test the molecules they suggest. The results must then be fed back into the AI systems. Academic computational groups can help to some extent by predicting molecules’ properties, but the predicted values can only partially validate models. Pharmaceutical companies do have the means to make and test the molecules suggested by their AI systems. However, they tend to keep their results secret, partly to avoid being ‘scooped’ by rivals. The necessity of rigour, safety, efficacy and trust in new drugs means that a way forwards must be found — a point echoed last month by researchers at the pharmaceutical company Amgen in Thousand Oaks, California4.
None of this changes the fact that the drug-discovery process has always involved a lot of luck. Even if AI does reduce the time and cost involved in getting a compound into — and perhaps through — preclinical testing, most drug candidates will still fail at later stages. But anything that can speed up the process represents a win. Industry and academia must leverage each other’s strengths to determine how AI can be used to best effect.