Abstract
Causal machine learning (ML) offers flexible, data-driven methods for predicting treatment outcomes including efficacy and toxicity, thereby supporting the assessment and safety of drugs. A key benefit of causal ML is that it allows for estimating individualized treatment effects, so that clinical decision-making can be personalized to individual patient profiles. Causal ML can be used in combination with both clinical trial data and real-world data, such as clinical registries and electronic health records, but caution is needed to avoid biased or incorrect predictions. In this Perspective, we discuss the benefits of causal ML (relative to traditional statistical or ML approaches) and outline the key components and steps. Finally, we provide recommendations for the reliable use of causal ML and effective translation into the clinic.
This is a preview of subscription content, access via your institution
Access options
Access Nature and 54 other Nature Portfolio journals
Get Nature+, our best-value online-access subscription
$29.99 / 30 days
cancel any time
Subscribe to this journal
Receive 12 print issues and online access
$209.00 per year
only $17.42 per issue
Buy this article
- Purchase on Springer Link
- Instant access to full article PDF
Prices may be subject to local taxes which are calculated during checkout
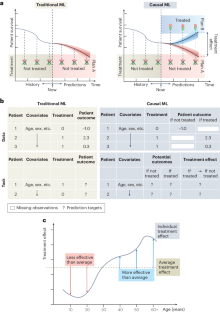


Similar content being viewed by others
References
Kaddour, J., Lynch, A., Liu, Q., Kusner, M. J. & Silva, R. Causal machine learning: a survey and open problems. Preprint at arXiv https://doi.org/10.48550/arXiv.2206.15475 (2022).
Yoon, J., Jordon, J. & van der Schaar, M. GANITE: estimation of individualized treatment effects using generative adversarial nets. In Proc. 6th International Conference on Learning Representations (ICLR, 2018).
Evans, W. E. & Relling, M. V. Pharmacogenomics: translating functional genomics into rational therapeutics. Science 286, 487–491 (1999).
Esteva, A. et al. A guide to deep learning in healthcare. Nat. Med. 25, 24–29 (2019).
Kopitar, L., Kocbek, P., Cilar, L., Sheikh, A. & Stiglic, G. Early detection of type 2 diabetes mellitus using machine learning-based prediction models. Sci. Rep. 10, 11981 (2020).
Alaa, A. M., Bolton, T., Di Angelantonio, E., Rudd, J. H. & van der Schaar, M. Cardiovascular disease risk prediction using automated machine learning: a prospective study of 423,604 UK Biobank participants. PLoS ONE 14, e0213653 (2019).
Cahn, A. et al. Prediction of progression from pre-diabetes to diabetes: development and validation of a machine learning model. Diabetes/Metab. Res. Rev. 36, e3252 (2020).
Zueger, T. et al. Machine learning for predicting the risk of transition from prediabetes to diabetes. Diabetes Technol. Ther. 24, 842–847 (2022).
Krittanawong, C. et al. Machine learning prediction in cardiovascular diseases: a metaanalysis. Sci. Rep. 10, 16057 (2020).
Xie, Y. et al. Comparative effectiveness of SGLT2 inhibitors, GLP-1 receptor agonists, DPP-4 inhibitors, and sulfonylureas on risk of major adverse cardiovascular events: Emulation of a randomised target trial using electronic health records. Lancet Diabetes Endocrinol. 11, 644–656 (2023).
Deng, Y. et al. Comparative effectiveness of second line glucose lowering drug treatments using real world data: emulation of a target trial. BMJ Med. 2, e000419 (2023).
Kalia, S. et al. Emulating a target trial using primary-care electronic health records: sodium glucose cotransporter 2 inhibitor medications and hemoglobin A1c. Am. J. Epidemiol. 192, 782–789 (2023).
Petito, L. C. et al. Estimates of overall survival in patients with cancer receiving different treatment regimens: emulating hypothetical target trials in the Surveillance, Epidemiology, and End Results (SEER)–Medicare linked database. JAMA Netw. Open 3, e200452 (2020).
Rubin, D. B. Estimating causal effects of treatments in randomized and nonrandomized studies. J. Educ. Psychol. 66, 688–701 (1974).
Rubin, D. B. Causal inference using potential outcomes: design, modeling, decisions. J. Am. Stat. Assoc. 100, 322–331 (2005).
Robins, J. M. Correcting for non-compliance in randomized trials using structural nested mean models. Commun. Stat. 23, 2379–2412 (1994).
Robins, J. M. Robust estimation in sequentially ignorable missing data and causal inference models. In 1999 Proceedings of the American Statistical Association on Bayesian Statistical Science 6–10 (2000).
Holland, P. W. Statistics and causal inference. J. Am. Stat. Assoc. 81, 945–960 (1986).
Pearl, J. Causality: Models, Reasoning, and Inference (Cambridge University Press, 2009).
Hemkens, L. G. et al. Interpretation of epidemiologic studies very often lacked adequate consideration of confounding. J. Clin. Epidemiol. 93, 94–102 (2018).
Dang, L. E. et al. A causal roadmap for generating high-quality real-world evidence. J. Clin. Transl. Sci. 7, e212 (2023).
Petersen, M. L. & van der Laan, M. J. Causal models and learning from data: integrating causal modeling and statistical estimation. Epidemiology 25, 418–426 (2014).
van der Laan, M. J. & Rubin, D. Targeted maximum likelihood learning. Int. J. Biostatistics 2, 11 (2006).
Hirano, K. & Imbens, G. W. in Applied Bayesian Modeling and Causal Inference from Incomplete-Data Perspectives: An Essential Journey with Donald Rubin’s Statistical Family (eds Gelman, A. & Meng, X.-L.) Ch. 7 (John Wiley & Sons, 2004).
Specht, L. et al. Modern radiation therapy for Hodgkin lymphoma: field and dose guidelines from the international lymphoma radiation oncology group (ILROG). Int. J. Radiat. Oncol. Biol. Phys. 89, 854–862 (2014).
van Geloven, N. et al. Prediction meets causal inference: the role of treatment in clinical prediction models. Eur. J. Epidemiol. 35, 619–630 (2020).
Kennedy, E. H. Towards optimal doubly robust estimation of heterogeneous causal effects. Electron. J. Stat. 17, 3008–3049 (2023).
Imbens, G. W. & Rubin, D. B. Causal Inference in Statistics, Social, and Biomedical Sciences (Cambridge University Press, 2015).
Chen, J., Vargas-Bustamante, A., Mortensen, K. & Ortega, A. N. Racial and ethnic disparities in health care access and utilization under the Affordable Care Act. Med. Care 54, 140–146 (2016).
Cinelli, C., Forney, A. & Pearl, J. A crash course in good and bad controls. Sociol. Methods Res. https://doi.org/10.1177/00491241221099552 (2022).
Laffers, L. & Mellace, G. Identification of the average treatment effect when SUTVA is violated. Department of Economics SDU. Discussion Papers on Business and Economics No. 3 (University of Southern Denmark, 2020).
Huber, M. & Steinmayr, A. A framework for separating individual-level treatment effects from spillover effects. J. Bus. Econ. Stat. 39, 422–436 (2021).
Syrgkanis, V. et al. Machine learning estimation of heterogeneous treatment effects with instruments. In Proc. 33rd International Conference on Neural Information Processing Systems (eds Wallach, H. M. & Larochelle, H.) 15193–15202 (NeurIPS, 2019).
Frauen, D. & Feuerriegel, S. Estimating individual treatment effects under unobserved confounding using binary instruments. In Proc. 11th International Conference on Learning Representations (ICLR, 2023).
Lim, B. Forecasting treatment responses over time using recurrent marginal structural networks. In Proc. Advances in Neural Information Processing Systems 31 (eds Bengio, H. et al.) (NeurIPS, 2018).
Liu, R., Yin, C. & Zhang, P. Estimating individual treatment effects with time-varying confounders. In Proc. IEEE International Conference on Data Mining (ICDM) 382–391 (IEEE, 2020).
Li, R. et al. G-Net: a deep learning approach to G-computation for counterfactual outcome prediction under dynamic treatment regimes. In Proc. Machine Learning for Health (eds Roy, S. et al.) 282–299 (PMLR, 2021).
Bica, I., Alaa, A. M., Jordon, J. & van der Schaar, M. Estimating counterfactual treatment outcomes over time through adversarially balanced representations. In Proc. 8th International Conference on Learning Representations 11790–11817 (ICLR, 2020).
Liu, R., Hunold, K. M., Caterino, J. M. & Zhang, P. Estimating treatment effects for time-to-treatment antibiotic stewardship in sepsis. Nat. Mach. Intell. 5, 421–431 (2023).
Melnychuk, V., Frauen, D. & Feuerriegel, S. Causal transformer for estimating counterfactual outcomes. In Proc. 39th International Conference on Machine Learning (eds Chaudhuri, K. et al.) 15293–15329 (PMLR, 2022).
Schulam, P. & Saria, S. Reliable decision support using counterfactual models. In Proc. 31st International Conference on Neural Information Processing Systems (eds von Luxburg, U. et al.) 1696–1706 (NeurIPS, 2017).
Vanderschueren, T., Curth, A., Verbeke, W. & van der Schaar, M. Accounting for informative sampling when learning to forecast treatment outcomes over time. In Proc. 40th International Conference on Machine Learning (eds Krause, A. et al.) 34855–34874 (PMLR, 2023).
Seedat, N., Imrie, F., Bellot, A., Qian, Z. & van der Schaar, M. Continuous-time modeling of counterfactual outcomes using neural controlled differential equations. In Proc. 39th International Conference on Machine Learning (eds Chaudhuri, K. et al.) 19497–19521 (PMLR, 2022).
Hess, K., Melnychuk, V., Frauen, D. & Feuerriegel, S. Bayesian neural controlled differential equations for treatment effect estimation. In Proc. 12th International Conference on Learning Representations (ICLR, 2024).
Hatt, T., Berrevoets, J., Curth, A., Feuerriegel, S. & van der Schaar, M. Combining observational and randomized data for estimating heterogeneous treatment effects. Preprint at arXiv https://doi.org/10.48550/arXiv.2202.12891 (2022).
Colnet, B. et al. Causal inference methods for combining randomized trials and observational studies: a review. Stat. Sci. 39, 165–191 (2024).
Kallus, N., Puli, A. M. & Shalit, U. Removing hidden confounding by experimental grounding. In Proc. 32nd Conference on Neural Information Processing Systems (eds Bengio, S. et al.) 10888–10897 (NeurIPS, 2018).
van der Laan, M. J., Polley, E. C. & Hubbard, A. E. Super learner. Stat. Appl. Genet. Mol. Biol. 6, 25 (2007).
van der Laan, M. J. & Rose, S. Targeted Learning: Causal Inference for Observational and Experimental Data 1st edn (Springer, 2011).
Zheng, W. & van der Laan, M. J. in Targeted Learning: Causal Inference for Observational and Experimental Data 1st edn, 459–474 (Springer, 2011).
Díaz, I. & van der Laan, M. J. Targeted data adaptive estimation of the causal dose–response curve. J. Causal Inference 1, 171–192 (2013).
Luedtke, A. R. & van der Laan, M. J. Super-learning of an optimal dynamic treatment rule. Int. J. Biostatistics 12, 305–332 (2016).
Künzel, S. R., Sekhon, J. S., Bickel, P. J. & Yu, B. Metalearners for estimating heterogeneous treatment effects using machine learning. Proc. Natl Acad. Sci. USA 116, 4156–4165 (2019).
Curth, A. & van der Schaar, M. Nonparametric estimation of heterogeneous treatment effects: From theory to learning algorithms. In Proc. 24th International Conference on Artificial Intelligence and Statistics (eds Banerjee, A. & Fukumizu, K.) 1810–1818 (PMLR, 2021).
Athey, S. & Imbens, G. Recursive partitioning for heterogeneous causal effects. Proc. Natl Acad. Sci. USA 113, 7353–7360 (2016).
Wager, S. & Athey, S. Estimation and inference of heterogeneous treatment effects using random forests. J. Am. Stat. Assoc. 113, 1228–1242 (2018).
Athey, S., Tibshirani, J. & Wager, S. Generalized random forests. Ann. Stat. 47, 1148–1178 (2019).
Shalit, U., Johansson, F. D. & Sontag, D. Estimating individual treatment effect: generalization bounds and algorithms. In Proc. 34th International Conference on Machine Learning (eds Precup, D. & Teh, Y. W.) 3076–3085 (PMLR, 2017).
Shi, C., Blei, D. & Veitch, V. Adapting neural networks for the estimation of treatment effects. In Proc. 33rd International Conference on Neural Information Processing Systems (eds Wallach, H. M. et al.) 2496–2506 (NeurIPS, 2019).
Bach, P., Chernozhukov, V., Kurz, M. S. & Spindler, M. DoubleML: an object-oriented implementation of double machine learning in Python. J. Mach. Learn. Res. 23, 2469–2474 (2022).
Foster, D. J. & Syrgkanis, V. Orthogonal statistical learning. Ann. Stat. 51, 879–908 (2023).
Kennedy, E. H., Ma, Z., McHugh, M. D. & Small, D. S. Nonparametric methods for doubly robust estimation of continuous treatment effects. J. R. Stat. Soc. Series B Stat. Methodol. 79, 1229–1245 (2017).
Nie, L., Ye, M., Liu, Q. & Nicolae, D. VCNet and functional targeted regularization for learning causal effects of continuous treatments. In Proc. 9th International Conference on Learning Representations (ICLR, 2021).
Bica, I., Jordon, J. & van der Schaar, M. Estimating the effects of continuous-valued interventions using generative adversarial networks. In Proc. 34th Annual Conference on Neural Information Processing Systems (eds Larochelle, H. et al.) (NeurIPS, 2020).
Hill, J. L. Bayesian nonparametric modeling for causal inference. J. Computational Graph. Stat. 20, 217–240 (2011).
Schwab, P., Linhardt, L., Bauer, S., Buhmann, J. M. & Karlen, W. Learning counterfactual representations for estimating individual dose-response curves. In Proc. 34th AAAI Conference on Artificial Intelligence 5612–5619 (AAAI, 2020).
Schweisthal, J., Frauen, D., Melnychuk, V. & Feuerriegel, S. Reliable off-policy learning for dosage combinations. In Proc. 37th Annual Conference on Neural Information Processing Systems (NeurIPS, 2023).
Melnychuk, V., Frauen, D. & Feuerriegel, S. Normalizing flows for interventional density estimation. In Proc. 40th International Conference on Machine Learning (eds Krause, A. et al.) 24361–24397 (PMLR, 2023).
Banerji, C. R., Chakraborti, T., Harbron, C. & MacArthur, B. D. Clinical AI tools must convey predictive uncertainty for each individual patient. Nat. Med. 29, 2996–2998 (2023).
Alaa, A. M. & van der Schaar, M. Bayesian inference of individualized treatment effects using multi-task Gaussian processes. In Proc. 31st Annual Conference on Neural Information Processing Systems (eds von Luxburg, U. et al.) 3425–3433 (NeurIPS, 2017).
Alaa, A., Ahmad, Z. & van der Laan, M. Conformal meta-learners for predictive inference of individual treatment effects. In Proc. 37th Annual Conference on Neural Information Processing Systems (eds Oh, A. et al.) (NeurIPS, 2023).
Curth, A., Svensson, D., Weatherall, J. & van der Schaar, M. Really doing great at estimating CATE? A critical look at ML benchmarking practices in treatment effect estimation. In Proc. 35th Conference on Neural Information Processing Systems Datasets and Benchmarks Track (eds Vanschoren, J. & Yeung, S.-K.) (NeurIPS, 2021).
Boyer, C. B., Dahabreh, I. J. & Steingrimsson, J. A. Assessing model performance for counterfactual predictions. Preprint at arXiv https://doi.org/10.48550/arXiv.2308.13026 (2023).
Keogh, R. H. & van Geloven, N. Prediction under interventions: evaluation of counterfactual performance using longitudinal observational data. Preprint at arXiv https://doi.org/10.48550/arXiv.2304.10005 (2023).
Curth, A. & van der Schaar, M. In search of insights, not magic bullets: towards demystification of the model selection dilemma in heterogeneous treatment effect estimation. In Proc. 40th International Conference on Machine Learning (eds Krause, A. et al.) 6623–6642 (PMLR, 2023).
Sharma, A., Syrgkanis, V., Zhang, C. & Kıcıman, E. DoWhy: addressing challenges in expressing and validating causal assumptions. Preprint at arXiv https://doi.org/10.48550/arXiv.2108.13518 (2021).
Vokinger, K. N., Feuerriegel, S. & Kesselheim, A. S. Mitigating bias in machine learning for medicine. Commun. Med. 1, 25 (2021).
Petersen, M. L., Porter, K. E., Gruber, S., Wang, Y. & van der Laan, M. J. Diagnosing and responding to violations in the positivity assumption. Stat. Methods Med. Res. 21, 31–54 (2012).
Jesson, A., Mindermann, S., Shalit, U. & Gal, Y. Identifying causal-effect inference failure with uncertainty-aware models. In Proc. 34th Conference on Neural Information Processing Systems (eds Larochelle, H. et al.) 11637–11649 (NeurIPS, 2020).
Rudolph, K. E. et al. When effects cannot be estimated: redefining estimands to understand the effects of naloxone access laws. Epidemiology 33, 689–698 (2022).
Cornfield, J. et al. Smoking and lung cancer: recent evidence and a discussion of some questions. J. Natl Cancer Inst. 22, 173–203 (1959).
Frauen, D., Melnychuk, V. & Feuerriegel, S. Sharp bounds for generalized causal sensitivity analysis. In Proc. 37th Annual Conference on Neural Information Processing Systems (eds Oh, A. et al.) (NeurIPS, 2023).
Kallus, N., Mao, X. & Zhou, A. Interval estimation of individual-level causal effects under unobserved confounding. In Proc. 22nd International Conference on Artificial Intelligence and Statistics (eds Chaudhuri, K. & Sugiyama, M.) 2281–2290 (PMLR, 2019).
Jin, Y., Ren, Z. & Candès, E. J. Sensitivity analysis of individual treatment effects: a robust conformal inference approach. Proc. Natl Acad. Sci. USA 120, e2214889120 (2023).
Dorn, J. & Guo, K. Sharp sensitivity analysis for inverse propensity weighting via quantile balancing. J. Am. Stat. Assoc. 118, 2645–2657 (2023).
Oprescu, M. et al. B-learner: quasi-oracle bounds on heterogeneous causal effects under hidden confounding. In Proc. 40th International Conference on Machine Learning (eds Krause, A. et al.) 26599–26618 (PMLR, 2023).
Hernán, M. A. & Robins, J. M. Using big data to emulate a target trial when a randomized trial is not available. Am. J. Epidemiol. 183, 758–764 (2016).
Xu, J. et al. Protocol for the development of a reporting guideline for causal and counterfactual prediction models in biomedicine. BMJ Open 12, e059715 (2022).
Fournier, J. C. et al. Antidepressant drug effects and depression severity: a patient-level meta-analysis. JAMA 303, 47–53 (2010).
Booth, C. M., Karim, S. & Mackillop, W. J. Real-world data: towards achieving the achievable in cancer care. Nat. Rev. Clin. Oncol. 16, 312–325 (2019).
Chien, I. et al. Multi-disciplinary fairness considerations in machine learning for clinical trials. In Proc. 2022 ACM Conference on Fairness, Accountability, and Transparency (FACCT '22) 906–924 (ACM, 2022).
Ross, E. L. et al. Estimated average treatment effect of psychiatric hospitalization in patients with suicidal behaviors: a precision treatment analysis. JAMA Psychiatry 81, 135–143 (2023).
Cole, S. R. & Stuart, E. A. Generalizing evidence from randomized clinical trials to target populations: the ACTG 320 trial. Am. J. Epidemiol. 172, 107–115 (2010).
Hatt, T., Tschernutter, D. & Feuerriegel, S. Generalizing off-policy learning under sample selection bias. In Proc. 38th Conference on Uncertainty in Artificial Intelligence (eds Cussens, J. & Zhang, K.) 769–779 (PMLR, 2022).
Sherman, R. E. et al. Real-world evidence—what is it and what can it tell us. N. Engl. J. Med. 375, 2293–2297 (2016).
Norgeot, B. et al. Minimum information about clinical artificial intelligence modeling: the MI-CLAIM checklist. Nat. Med. 26, 1320–1324 (2020).
Von Elm, E. et al. The strengthening the reporting of observational studies in epidemiology (STROBE) statement: Guidelines for reporting observational studies. Lancet 370, 1453–1457 (2007).
Nie, X. & Wager, S. Quasi-oracle estimation of heterogeneous treatment effects. Biometrika 108, 299–319 (2021).
Chernozhukov, V. et al. Double/debiased machine learning for treatment and structural parameters. Econom. J. 21, C1–C68 (2018).
Morzywołek, P., Decruyenaere, J. & Vansteelandt, S. On a general class of orthogonal learners for the estimation of heterogeneous treatment effects. Preprint at arXiv https://doi.org/10.48550/arXiv.2303.12687 (2023).
Acknowledgements
S.F. acknowledges funding via Swiss National Science Foundation Grant 186932.
Author information
Authors and Affiliations
Contributions
All authors contributed to conceptualization, manuscript writing and approval of the manuscript.
Corresponding author
Ethics declarations
Competing interests
The authors declare no competing interests.
Peer review
Peer review information
Nature Medicine thanks Matthew Sperrin and the other, anonymous, reviewer(s) for their contribution to the peer review of this work. Primary Handling Editor: Karen O’Leary, in collaboration with the Nature Medicine team.
Additional information
Publisher’s note Springer Nature remains neutral with regard to jurisdictional claims in published maps and institutional affiliations.
Rights and permissions
Springer Nature or its licensor (e.g. a society or other partner) holds exclusive rights to this article under a publishing agreement with the author(s) or other rightsholder(s); author self-archiving of the accepted manuscript version of this article is solely governed by the terms of such publishing agreement and applicable law.
About this article
Cite this article
Feuerriegel, S., Frauen, D., Melnychuk, V. et al. Causal machine learning for predicting treatment outcomes. Nat Med 30, 958–968 (2024). https://doi.org/10.1038/s41591-024-02902-1
Received:
Accepted:
Published:
Issue Date:
DOI: https://doi.org/10.1038/s41591-024-02902-1