Abstract
Forest restoration is fundamental to overcoming biodiversity crises and climate change. In tropical forests, animals can improve forest recovery as they disperse >70% of tree species. However, representing animals in restoration and climate change policies remains challenging because a quantitative assessment of their contribution to forest and carbon recovery is lacking. Here we used individual-based models to assess frugivore-mediated seed rain in open areas along a fragmentation gradient. Movements of large birds were limited in landscapes with <40% forest cover, although small birds continued to disperse seeds. Large birds disperse seeds of late-successional species with higher carbon storage potential. Their restricted movement therefore reduced potential biomass in future forests by 38%. Maintaining forest cover >40% is essential to optimizing animals’ contribution to restoration success. Active restoration (for example, planting trees) is required in more fragmented landscapes to achieve carbon and biodiversity targets.
Similar content being viewed by others
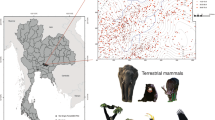
Main
Natural climate solutions (NCS) present opportunities to address climate change while promoting biodiversity conservation and human well-being. The IPCC suggests that to prevent global temperature increases beyond 2 °C, NCS must remove and store around 10 GtCO2 from the atmosphere annually, of which ~62% is associated with forests1,2,3. In this context, forest restoration has gained massive global expansion with the promotion of the UN Decade on Ecosystem Restoration4,5,6,7. However, forest restoration remains a complex social and ecological challenge, particularly in tropical regions that are central to achieving climate and biodiversity goals1,8. Natural forest regeneration often presents an ecologically responsible and cost-effective method for recovery relative to active planting. Yet, this is not always possible in degraded regions where the soil is too depleted or the seed bank has been irreparably changed, and it remains unclear which ecosystems can recover to their full maturity without active intervention. Therefore, a critical outstanding challenge in restoration ecology is identifying where animals can contribute to the natural recovery of carbon storage potential and where active management interventions are needed.
A growing body of evidence suggests that animals can underpin the carbon cycle and therefore NCS9,10,11. In particular, animals can be determinants of carbon recovery in tropical restoration projects, given that they are essential for seed dispersal12,13. Seed arrival at degraded sites is a key step limiting ecological recovery in many tropical forests14. In these regions, frugivorous animals are responsible for dispersing 70% to 90% of tree species7,8, so animal movement restrictions can present a primary barrier to natural ecological recovery. However, their contributions to the deposition of seeds across an area (that is, seed rain) may vary depending on the composition of animals in the region12, as well as their diets15, their traits13,16 and the barriers to movement17. Explicit consideration of each of these interacting factors is necessary for generating a unified, predictive understanding of the frugivore-mediated seed rain in degraded regions. For example, large frugivores tend to consume seeds from trees that often are long-lived species with large sizes and dense wood, which are critical for carbon storage18,19. Given that the movement of larger frugivores is disproportionately affected by fragmentation17, the limitation of their movements may strongly limit the recovery of large, late-succession trees, ultimately reducing the carbon storage potential of recovering ecosystems20,21. However, empirical evidence for these trends is limited to local-scale studies that provide mixed trends22,23,24,25, and we still lack quantitative information about the importance of animal movement in facilitating forest carbon recovery in fragmented landscapes.
To address this, we developed a regional-scale study to quantify seed dispersal by birds and the resulting impacts on natural forest recovery across a large gradient of forest fragmentation in the Atlantic Forest, Brazil (Fig. 1). This approach enables us to map seed rain across fragmented landscapes to explore which areas receive sufficient seed dispersal, enabling natural forest regeneration while enhancing carbon storage and biodiversity. Conversely, we can also identify the areas where seed rain is insufficient to improve forest management and promote natural regeneration of healthy forest systems. Specifically, we assess (1) how landscape-scale forest cover and isolation determine animal-induced seed rain in open areas and the potential carbon storage of the resultant forests and (2) how bird traits interact with forest cover to determine the species composition and carbon storage potential of regenerating forests.
a, Location of the landscapes in South America. The black polygons represent Brazilian states. The red fill shows the state of Sao Paulo. The orange squares show the locations of the study landscapes. b, Specific locations of the ten landscapes in Sao Paulo state. The green polygons show the forest areas, and white indicates deforested areas. c, Forest cover of the ten landscapes. Each landscape is 2 km2. The squares are organized from low forest cover in the upper left to high forest cover in the lower right (from 9% to 61% forest). Green represents forest cover, yellow indicates isolated trees or corridors and white indicates open areas. Credit: Esri, GEBCO, Garmin, NaturalVue.
The Atlantic Forest presents a unique opportunity to explore potential forest regeneration in fragmented landscapes. This region is a hotspot for tropical forest restoration26 and biodiversity27. The Atlantic Forest is highly fragmented, with only 12% of the original forest remaining28,29,30, and is targeted for 12 million hectares of reforestation by 203031,32,33. We chose ten landscapes spanning a fragmentation gradient from 9% to 61% forest cover (Supplementary Table 1). Along this gradient, we built three hierarchical Bayesian models for critical components of the seed dispersal process: fruit ingestion (frugivory), the movements of frugivores between forest fragments and the time needed for seed deposition (gut passage time (GPT)). Using the estimated parameters for each species across the fragmentation gradient, we conducted simulations to estimate the potential seed rain deposited by each bird species in open areas across the fragmentation gradient (Extended Data Fig. 1). We then evaluated the seed rain and the potential carbon stock of forests that would result from the composition of dispersed species. By providing an integrated understanding of frugivore contributions to seed rain, it is possible to determine where animals contribute to the natural recovery of forests and where active management efforts are necessary to meet restoration goals while contributing to carbon sequestration31,32,33. By better matching restoration strategies to the local landscape and diversity context, fund investments can be optimized to maximize the influence of restoration in addressing current climate and biodiversity crises.
Results
We found that the contribution of frugivorous birds to potential seed rain and carbon storage restoration changed along the fragmentation gradient. Specifically, seed deposition and potential aboveground biomass (AGB) increased nonlinearly with increasing forest cover (R2seed = 0.83, R2biomass = 0.55) and decreased with increasing forest isolation (R2seed = 0.79, R2biomass = 0.62; Fig. 2 and Supplementary Table 2). Seed deposition and AGB decreased in landscapes with approximately 40% forest cover (Fig. 2 and Supplementary Table 3). For forest isolation, seed deposition strongly decreased at distances >133 m from forest, while potential AGB had a stronger limitation and declined beyond 112 m (Supplementary Table 3). By comparing the carbon stock potential of regenerating communities between the two extremes of the fragmentation gradient, we found that regenerating tree communities in fragmented landscapes harbour up to 38% less carbon than those in less fragmented landscapes.
a,b, The number of seeds deposited in open areas per landscape as a function of forest cover (percentage of forest cells) (a) and isolation of forest (distance in metres to the nearest forest patch averaged across cells) (b). c,d, The same relationships were tested for potential AGB derived from the seed rain generated by birds (in Mg per landscape). Each point depicts a result of the ten simulations performed per landscape scenario, where the same x-axis value indicates a unique landscape of the ten tested. The lines represent fitted mean tendency, and the shaded areas indicate 95% confidence intervals. The vertical dashed lines depict inflection points in the responses.
In general, bird body mass and gape size had an inverse relationship with the contribution of frugivores to seed dispersal into open areas (coefbody = −2.31, P < 0.001; coefgape = −0.54, P < 0.001; Fig. 3a,b), which became more abrupt in highly fragmented landscapes (Supplementary Table 4). Small birds with small gapes dispersed more seeds, especially in highly fragmented landscapes (<40% forest cover; Supplementary Table 4). However, they primarily dispersed plant species with lower carbon storage capacity (lower wood density and height). As a result, despite depositing a larger number of seeds into open areas, their contribution to potential AGB was lower, especially in landscapes with high forest cover (>40%; Fig. 3d,e). Birds with a higher degree of frugivory contributed more to seed deposition and potential AGB, but only in landscapes with high forest cover (Fig. 3d–f and Supplementary Table 4).
a–f, The points represent the contributions of bird species with different body masses (in grams, log-transformed) (a,d), gape widths (in mm) (b,e) and degrees of frugivory (low (n = 14,180), middle (n = 7,280) and high (n = 1,920)) (c,f). Each point (n = 23,570) represents an individual bird simulated in each landscape in each repetition. The forest cover of the landscapes is represented with colours. In the scatter plots, the points and lines are coloured from light green to dark blue (9–61% forest cover); in the box plots, the landscapes are grouped into low (light green, 0% to 30%), intermediate (green, 30% to 50%) and high (grey-blue, >50%) forest cover. The centre lines in the box plots show the median values, the box limits show the upper and lower quartiles, the whiskers represent 1.5× the interquartile range and the points represent the outliers.
Across all landscapes, our simulations predicted strong decay in the number of seeds deposited (coef = −1,598.9, P < 0.001; Supplementary Table 2) and potential biomass restoration (coef = −0.99, P < 0.001; Supplementary Table 2) as the distance to the forest increased (Fig. 4). This trend was apparent in all landscapes, irrespective of the percentage of forest cover in each landscape. This decay is pronounced at distances smaller than 133 m from the forest edge, with low contributions afterwards (Fig. 4 and Supplementary Tables 2 and 3).
a, Number of seeds deposited in each pixel. b, Potential AGB from forest regeneration per pixel. The grey dashed lines show the inflection thresholds. The lines and points are coloured according to the percentage of forest cover remaining in each transect. The magenta line represents the mean tendency. c, Community-level seed shadows in each landscape are organized from low forest cover (upper left) to high forest cover (lower right). We provide the results from one repetition as an example, with green representing forest and red indicating the number of seeds in each pixel, from high numbers of seeds deposited in dark magenta to low numbers in light magenta.
Discussion
An open question in forest restoration ecology is in which areas animals can contribute strongly to trigger natural restoration and which areas require active restoration22,24,34. Studies in temperate regions suggest that natural regeneration is often more efficient than active intervention, but there is no such consensus in tropical regions, where natural regeneration can be highly variable22,23,24. The effectiveness of natural regeneration of tropical forest ecosystems is limited, in its initial stage, by the dispersal of seeds into degraded areas35,36. Especially in tropical ecosystems, seed dispersal mediated by animals plays a fundamental role in determining the species that can regenerate15,16. Natural regeneration therefore depends directly on the interaction between seed-dispersing frugivores and the surrounding landscape structure, especially when the goal is to restore biodiversity and carbon storage potential. Here we provide evidence that the functional composition of frugivorous bird communities and landscape structure interact to drive the regeneration of functional, diverse forests. For example, natural regeneration in landscapes with healthy populations of large frugivorous birds is expected to receive diverse seed rain with many large seeds, promoting a higher carbon sequestration potential in the regenerating forests. However, we observed that this potential will not be achieved if large birds cannot move across the landscape. Overall, our results highlight that the restricted movement of frugivorous birds across fragmented landscapes can reduce carbon recovery by up to 38%. This information is valuable for designing restoration projects as an effective NCS for mitigating climate change and biodiversity loss37.
To assess the most appropriate management strategies for natural regeneration, it is necessary to identify the composition and configuration of landscapes that enable or obstruct animals’ contribution to seed rain in open areas35,38,39,40. Recent evidence highlights the correlation between landscape forest cover and forest regeneration41. However, data that untangle the mechanisms underpinning this pattern and its effects have not been explored. By integrating frugivore diversity and landscape configuration, we were able to identify tipping points where natural regeneration is no longer sufficient and active intervention practices are required to restore functional forests with high carbon sequestration capacity. In the Atlantic Forest, 5.5 million hectares have been identified as having the potential for natural regeneration42. However, all these regrowing forests will not achieve high diversity and carbon sequestration potential when animal movement is limited, particularly when the aim is to promote carbon sequestration. We found that natural regeneration, induced by animal-mediated seed dispersal, can foster a restoration strategy in landscapes with >40% forest cover and where fragments are separated by <133 m. In these landscapes, bird movement can facilitate rich seed rain in open areas, which ultimately defines a diverse composition of regenerating forests. In contrast, natural regeneration may be less effective in more fragmented landscapes, as the contribution of birds to seed rain decreases significantly when the distance from the forest edge exceeds 133 m, especially if the objective is to restore the carbon sequestration potential of the forest, which is strongly limited by forest distance (112 m). The ecosystem service of carbon sequestration is more susceptible to forest fragmentation, and recovering the full carbon storage potential may require additional interventions in isolated fragments. These tipping points are likely to be specific to this important study region, but evaluating such thresholds across different forested landscapes will be critical for maximizing the effectiveness of forest regeneration efforts across tropical regions.
A forest cover threshold of 30–40% has been previously recommended to preserve the diversity of small mammals, amphibians and birds in the Brazilian Atlantic Forest43,44,45. Our study demonstrates that maintaining landscape forest cover at >40% is also crucial for restoring key ecosystem functions performed by animals, such as seed dispersal. We therefore recommend that restoration policies and management (such as the Atlantic Forest Restoration Pact, which aims to restore and reforest 12 million ha by 203031,32,33) should integrate this threshold more broadly to optimize restoration planning. For example, we suggest that restoration projects located in areas with forest cover >40% and distance between fragments <133 m could rely more heavily on natural regeneration, potentially including assisted natural regeneration or hybrid strategies such as applied nucleation to speed up the recovery time46, depending on the duration and severity of prior land uses. However, restoration projects located in areas with <40% forest cover are likely to require active restoration strategies to enable forest recovery and to promote the recovery of forest carbon stocks.
Beyond landscape composition, our results suggest that restoration projects should also consider management strategies to restore diverse plant–frugivore interactions, especially those between large birds and large-seeded trees. We found that small frugivores were the main seed dispersers across all landscapes. However, they may not be the most important for restoring forest carbon stock, and even their seed dispersal behaviour changed with increasing forest fragmentation. These results highlight the changing ecological behaviour of different types of frugivores with fragmentation17. Typically, large frugivores eat more fruits and disperse seeds further than small frugivores in continuous forests47, but given their preference for forested areas, their seed dispersal patterns can be reversed in fragmented forests20,21. Ensuring trait matching across the full spectrum of plant–animal functional traits can therefore promote the dispersal of a diverse range of species into open areas48,49.
To guarantee that species spanning the full range of frugivory traits interact across the landscape, local management strategies that aim to attract frugivores and facilitate animal movement should be implemented. Some examples of these strategies include establishing living fences, planting shelter and fruit trees, enriching forest nucleation with fruiting trees, establishing feeders and perches for animals, offering seeds embedded in pulp, and, importantly, preventing poaching46,50,51,52. These strategies can increase frugivorous bird visitation rates to regeneration sites, facilitate frugivory interactions and increase the frequency of long-distance seed dispersal events50,52,53,54,55,56. Implementing these strategies in restoration areas is more economical than the cost associated with large-scale tree planting, which is traditionally implemented in active restoration projects42,57. In combination, these strategies will encourage the colonization of frugivorous animals into restoration sites, supporting the regeneration of native tree species and their mutualists and ultimately aiding the potential recovery of forest carbon stocks.
Our objective here was to evidence the determinant role of animals in fostering forest restoration by shaping the composition of the forest that can regenerate in fragmented landscapes. However, to improve our estimates and to extrapolate to global conditions, we need to include the effects of resource distribution, post-dispersal processes and the contribution of other taxonomic groups that are important seed dispersers. For example, fruit spatial and temporal distribution impact the number and composition of seeds dispersed into the deforested matrix58,59. In our study, we used the tree species composition of a single representative conserved forest in the region to inform the species pool that can remain in forest fragments and naturally regenerate in the region. However, fragmentation can change forest composition, allowing the inclusion of invasive species or decreasing large-seeded trees60,61. Hence, this forest composition may not represent the entire regional tree species pool of fragmented forest. Future work should therefore explore the impacts of changes in species composition and the spatial distribution of fruit resources in fragmented landscapes. In addition, birds usually prefer resting on perches rather than on the ground53,62. When perches are present, bird activity in the matrix increases, promoting a more even distribution of seeds throughout open areas52,53,54. Although birds are the major frugivorous group, other taxonomic groups (such as aerial and terrestrial mammals and reptiles) can substantially shape seed rain in open areas, either by seed dispersal or by predation mechanisms (for example, rodents and bats)63. However, we need more information on their interactions, traits and local movements to include these groups. Currently, large datasets on frugivory are biased towards birds64. In addition, tracking animal movements in local landscapes is especially difficult for mammals, which require capture and high-resolution GPS tags. Finally, other post-dispersal processes, such as seed predation, desiccation, competition, environmental filters and herbivory, can alter seedling establishment and therefore the composition of regenerating forest65. These post-dispersal processes are also influenced by animals, which may have adverse effects, especially in the early stages of restoration66. However, these processes must be carefully evaluated, since herbivores also act as seed dispersers and regulate the growth of strongly competitive species, promoting greater diversity67. Contrasting evidence regarding the effects of herbivores on restoration may be related to the health of the trophic chain in the areas to be restored, where the presence of predators can regulate the adverse effects of herbivores and promote their diversifying effect on the composition of the forest to be regenerated68.
Recognizing all the limitations mentioned above allows us to define future work priorities to improve estimates of the carbon potential in regenerating forests along the fragmentation gradient. An important next step is to gather empirical information to validate our predictions in the field. Obtaining data on seed rain density, as well as identifying plants and animals across various landscape configurations, requires a huge data collection effort. Fortunately, new technologies such as high-resolution satellite images69,70,71,72, GPS trackers to study precise animal movement patterns73 and the advancement of new DNA sequencing technologies74,75,76 can facilitate the description of resource abundance, animal behaviour and frugivory interactions. These technologies can also be used to rapidly validate the models by empirically measuring seed deposition by species with diverse traits77 and to measure derived properties such as potential carbon stock recovery or genetic flow78.
Natural forest regeneration presents a cost-effective opportunity to recover functional forests in fragmented landscapes. Our findings highlight that the recovery of tropical forests is contingent on the movement of frugivorous animals that are required for seed dispersal. At least ~40% tree cover is required to allow sufficient animal movement to enhance animal-mediated seed rain for the full recovery of tropical forest diversity and functionality across this region. In areas where animals are able to disperse seeds freely, natural regeneration is likely to be more cost-effective than active planting79, reducing implementation costs by up to 77% (ref. 42). However, in more fragmented areas that are below this tree cover threshold, restricted animal movement can reduce the spread of larger tree seeds and reduce the potential carbon regeneration by approximately 38% compared with less fragmented areas. Forest restoration policies should therefore explicitly consider mechanisms that enhance animal movement and landscape connectivity thresholds to optimize forest restoration as both a climate change solution and an effective conservation strategy.
Methods
We explored the potential contribution of birds to forest restoration by applying an individual-based model. This model simulates seed deposition by birds in open areas inside ten landscapes located along a forest fragmentation gradient in the Atlantic Forest. The model comprises three components of the seed dispersal process that define the potential locations of seed deposition: (1) frugivore diet (bird–plant interactions), (2) bird movement and (3) GPT. The frugivory interaction component defines which plant species are consumed by each bird species and therefore defines the potential composition of regenerating forests12,15. The movement component defines the birds’ movement trajectories according to their ability to move between forest patches and open areas17. The GPT determines how long a consumed seed takes to be digested and defecated80. This time, in conjunction with the bird trajectory, defines the possible sites of seed deposition. To parameterize the models, we fitted a hierarchical model to each process. The hierarchical structure of the models allows us to use morphological traits that indirectly influence all processes, such as body size, hand-wing index, tail length and tarsus length, to characterize the average response of species. These traits influence species mobility and foraging strategy81,82. We also included the phylogenetic relationships among species to improve predictions for those species with little information83. In addition, each model included relevant variables that influence each process. Finally, we simulated the potential interactions, movement and seed deposition for each bird individual in open areas inside each landscape (Extended Data Fig. 1).
Probability that a bird interacts with a fruiting plant
To predict the probability that a bird species interacts with a plant species and eats its fruits, we used a probabilistic approach that uses functional traits to predict the occurrence of interaction (that is, seed size must be smaller than gape width to ensure ingestion). We fitted a hierarchical model with Bayesian estimation to the Atlantic frugivory dataset64. The Atlantic frugivory dataset comprises more than 3,804 frugivory interactions between 232 birds and 511 plant species across the Atlantic Forest of Brazil64. Our approach fits a logistic Bernoulli model that relates the occurrence (1) or lack (0) of interaction between a bird (i) and a plant (j) species as a function of the log-ratio of trait matching between the bird and the plant species (that is, trait matching between seed size and gape width). The model assumes an optimal range of interaction probability (λij), contingent on the trait-matching relationship with the quadratic polynomial of the log-ratio (that is, log(traiti/traitj) + log(traiti/traitj)2). This hump-shaped relationship between interaction probability and the log ratio of consumer–resource traits is interpreted as an optimal phenotypic match between species84. In other words, a defined range of trait-matching values enhances the probability of interaction between partner species (equation (1)):
where i refers to the birds, j refers to the plants, TM refers to trait matching between seed size and gape width defined as \({\mathrm{TM}}=\log \left(\frac{{{\mathrm{gape}}\; {\mathrm{width}}}_{i}}{{\mathrm{seed}}\; {\mathrm{diameter}}_{j}}\right)\) and \({{\mathrm{TM}}}_{{ij}}^{2}\) is the second-order orthogonal polynomial. Parameter β1i represents the intercept for each bird, β2i and β3i define the strength of the relationship of trait matching for each bird, and λij is the mean probability of interaction between a bird i and a plant j.
Probability of bird movement between forest patches and open matrix
To predict the movement of birds between forest fragments in landscapes with different levels of fragmentation, we used the joint species movement model approach proposed by ref. 17. We calibrated the joint species movement models for bird movements in a subset of six landscapes with different levels of fragmentation, and on the basis of the estimated parameters, we predicted the movement for the remaining four landscapes. Each landscape differs in forest cover and forest isolation. Within each landscape, we selected a 600 m × 600 m plot. The plots were located at least 3 km apart (see refs. 3,17 for details of bird census methodology). In each plot, short-distance bird movements were monitored from September to December 2014 and September to December 2015, totalling 72 hours of observation per plot. Two observers were located at a vantage point location for four and a half hours and followed bird movements using 10 × 42 binoculars and a chronometer. Once a bird was spotted, the observers identified the species and tracked its movement, recording the time spent flying and perching (in seconds). A movement step was considered a discrete movement of an individual from one location, where it had been stationary, to another location. A movement track was defined as a consecutive sequence of steps. To identify the sequence of cells used, each landscape was divided into a grid of 10 m × 10 m cells (that is, 3,600 cells), and the identities of the origin and destination cell were recorded. We recorded the cells used by each bird between consecutive flights until the bird left the study plot or was lost. Using high-resolution satellite imagery (0.5 m × 0.5 m) of each plot, we related bird movements to forest cover. To estimate the probability of movement between different landscape covers, we modelled the probability psinm that bird i in landscape s moves from cell n to cell m (equation (2)):
where dnm is the Euclidean distance between the grid cells n and m, and the parameter αsi > 0 models the typical flight distance of species i in landscape s. The variable h1sm is an indicator of whether grid cell m of landscape s has remnant trees (1) or is an open area (0). The parameter γ1si measures the movement bias of the species to remnant tree areas compared with open areas. Similarly, the variable h2sm is an indicator of whether grid cell m belongs to the forest habitat (1) or not (0), and the parameter γ2si measures the movement bias of the species to forest habitat compared with open habitat. The normalizing constant knsi is defined so that the probabilities sum to unity over the target cells—that is, that \({\sum }_{m}{p}_{{sinm}}=1\) for all n, i and s. The model does not include the possibility of the individual leaving the study plot. It therefore models the next location on the condition that the individual does not leave the study plot. We thus truncated the data so that they did not involve steps outside the study plot. The likelihood of the data was computed as the product of the movement probabilities over all the steps observed in the data.
Probability that a bird deposits a seed in a given time
GPT—that is, the time interval between seed ingestion and defecation—is a main determinant of the seed dispersal functions of an animal85,86. The GPT has a critical impact on the spatial pattern of seed dispersal generated by a frugivore, as well as on seed survival and subsequent seed germination87. To determine the time interval between seed ingestion and defecation, we developed a hierarchical model that linearly relates the time a seed takes to pass through the animal gut (GPT) and the animal’s body mass (equation (3)). We fitted the model to the dataset published by ref. 80, which includes 432 events of seed retention time between 112 bird species consuming 249 plant species. We decided to include traits from the bird and plant species to capture the complexity of the digestion process. For the birds, we used body mass, as it has been previously identified as the main determinant of GPT80. For the plants, we used seed size (mm), as it can influence the GPT inside the same individual (Supplementary Fig. 1):
where mgij is the mean passage time for seed j in bird i, θ1i is the intercept for each bird i, θ2i represents the force of the relationship between body mass (BM) and mean passage time for bird i, θ3i represents the force of the relationship between seed size (SS) and mean passage time for bird i, and ε is the standard error that draws from a truncated Cauchy distribution ε ~ C(0,10) to avoid negative time values.
Model parametrizations
To parameterize all three hierarchical models, we followed a joint species model approach83,88, where species-level parameters (β.i) are sampled from a multivariate normal whose mean is derived from species functional traits, and with a variance–covariance matrix (ϑ2) modified by species phylogeny83 (Extended Data Fig. 1). We used body mass, gape width, hand-wing index, tail length, tarsus length and frugivory dependence as traits that constrain bird movement ability and foraging strategy. Species’ functional traits were obtained from the Elton traits database85 and AVONET86 and indirectly influenced all the modelled processes. We built a consensus phylogenetic correlation matrix obtained from Birdtree89 with the package ape90 from 100 phylogenetic trees provided by ref. 89. We fitted the model using a Markov chain Monte Carlo sampling scheme. We ran three chains for 10,000–30,000 iterations. This setup was sufficient to achieve convergence (\(\hat{R} < 1.1\)) for the vast majority of parameters (Supplementary Table 5). To evaluate the model performance, we used the Tjur R2 metric for the frugivory model and the R2 for Bayesian regression models proposed by ref. 91 for the movement and GPT models. The models were fitted using the RSTAN package92, and the hierarchical model structures for frugivory interactions (SM1), movements (SM2), seed retention times (SM3) and simulation code (SM4) are provided in the Figshare repository at https://doi.org/10.6084/m9.figshare.c.7086625.v1. All models had a high level of chain convergence and good performance (frugivory model: RTjur = 0.39; movement model: RGelman = 0.7; GPT model: RGelman = 0.97; Supplementary Table 5). On the basis of these models, we were able to simulate movement for a total of 36 bird species that were the most commonly recorded moving between the fragments (Supplementary Table 1).
Seed dispersal simulations
We performed the simulations in ten square landscapes from the Cantareira-Mantiqueira Corridor, a priority area for the conservation of the Atlantic Forest. The region is predominantly composed of forest patches and a matrix of pasture for extensive cattle production (that is, open areas). We chose landscapes representative of the region, with multiple forest patches and an open pasture matrix with remnant trees and corridors (bird perches) and different forest cover percentages (from 9% to 61%; Fig. 1 and Supplementary Table 1). The square landscapes measured 1,800 m × 1,800 m and were subdivided into 8,100 cells of 20 m × 20 m to enable the movement simulations. Each cell contained information about its location (and Euclidean distance to the rest of cells) and the type of habitat it held (forest or open areas). In open-area cells, we calculated their isolation from forest fragments (measured as their minimum distance to the nearest forest fragment or perch, as adapted from ref. 93 by ref. 30). To this end, we used the function distance from the package raster94. To characterize the level of fragmentation of each landscape, we measured the percentage of cells with forest habitat and the mean distance of pasture cells (that is, open areas) to forest patches (isolation from forest). For the simulations, we obtained the bird relative abundance in each of these landscapes from ref. 95 and the plant composition from a representative conserved forest of the Cantareira-Mantiqueira Corridor sampled by Pompeu96. The plant composition represents the potential species found in conserved forests in the region, which serve as a source of seeds to be dispersed in open areas and that can naturally regenerate in this region. However, forest fragmentation can change the species composition and abundance of the tree species, altering the regenerating community in open areas60,61. Before performing the simulations, we classified bird species into five classes according to their trait similarity. We used hierarchical clustering with the ward.D2 agglomeration method and Euclidian distance. In cases where we did not have abundance or fruit consumption parameters for a specific bird, we used the mean value of the class to which the species belongs.
To perform a simulation for any randomly selected bird species from the pool of species that were recorded moving between fragments in each landscape (Supplementary Table 1), we performed a number of steps. Specifically, we first chose a bird individual from the bird pool of species in each landscape on the basis of their relative abundance95. We then randomly selected a source cell with forest cover, in which the bird eats the fruit and seeds of a given plant species and starts its movement across the landscape; we chose the plant species to be consumed according to the probability of interaction estimated by the interaction probability model. We calculated these probabilities by drawing parameter values from the 95% confidence intervals corresponding to the parameter distribution and the traits of the selected bird species and the plant species present in the forest. Next, we randomly drew the number of fruits consumed from a Poisson distribution that has as a parameter the mean number of fruits consumed for different size classes of birds. We collected information on fruits consumed from 107 plant species by 3,339 individuals of 171 bird species in the Atlantic Forest (Supplementary Table 6, SM4). We used the average fruit consumed per species. When we did not have information for each species, we used the average fruit consumed for different size classes of birds. Once we had chosen the food plant species and the number of consumed fruits, we simulated the movement track by calculating the probability of the selected bird moving from the source cell to any other cell in the landscape by using landscape class, bird traits and the parameter values from the 95% confidence interval of the parameter distribution estimated by the movement model; we randomly chose a target cell using these probabilities. As we calibrated the model in six landscapes and predicted the movements in ten landscapes, we used the movement parameters estimated for the landscape that was most similar in terms of landscape structure—that is, we set parameters estimated in low-forest-cover landscapes to simulate the probability of movements in the landscapes with less forest cover. By doing so, we recognized that the landscape structure modifies the bird’s movement patterns17. We allowed consecutive movements of the bird in the landscape for three hours, which represents the period of greatest activity for birds97. We then calculated the time of each movement by dividing the distance of the movement by the speed of the bird taken from data presented in ref. 17. Finally, to identify in which cell the seeds were deposited, we calculated the GPT for the selected seed and bird species by using seed size, bird traits and parameter drawing from the 95% confidence interval of the parameter distribution estimated by the GPT model, and we determined the bird’s position when the GPT was equal to the time passed in the simulated movements.
The combination of bird feeding, moving and seed deposition events constitute an individual movement track. From each movement track, we recorded the identities of the species involved, the number of seeds deposited and the identity of the source and target cells. We ran ten repetitions for each landscape. In each repetition, we simulated 100 movement tracks, representing 100 individual movements, and obtained resultant raster maps that represent the seed shadow of the bird community.
Calculating potential AGB
Using the assumption that the species identity of deposited seeds will determine the potential composition of forests regenerating in each pixel cell, we predicted future forest composition. The forest composition regenerating in each pixel draws from the species pool of our single reference conserved forest, possibly not representing the entire regional tree species pool. We estimated the potential carbon stock that can regenerate in each cell. As not all deposited seeds recruit, given other post-dispersal processes, we estimated the carbon potential that a given species can have per hectare, considering its natural densities in the reference forest. For each species, we calculated the AGB per hectare using an equation that relates basal area per hectare in the reference forest (absolute dominance (DoA)), wood density (ρ) and height (H), estimated from the forests of the region18. By using the DoA, we have an estimation of the sum of the basal area of the individuals of each species sampled in a hectare; it therefore reflects the natural densities of the species. With values of the potential biomass per hectare of each species, we were then able to estimate the potential biomass representation of each species for the pixel size in our study area. We assigned the potential species biomass per pixel if seeds of the species were deposited in the pixel (equation (4)):
where AGBi ha−1 is the AGB for species i per ha, DoAi is the basal area per hectare, ρi is the wood density and Hi is the maximum height. We used mean wood density values for each species obtained from ref. 98. Whenever this mean value of wood density was not available at the species level, we used the genus average instead. To determine the carbon concentration in AGB, we used the mean estimation of 40% water content in total biomass to get the dry biomass and 48.5% carbon in the dry biomass to get the carbon content following ref. 99.
For each landscape repetition, we summed the AGB potential of seed rain from all open-area cells and transformed it into AGB potential (Mg) per landscape. To be clear, this is a potential AGB value that relies on seed rain composition alone and does not include subsequent germination success or environmental filtering. In addition, for each frugivore species, we estimated their contribution to seed deposition into open areas and to AGB (expressed as a percentage with respect to values derived from the activity of all frugivores present in the community).
Data analysis
To understand the contribution of the frugivorous bird community to the restoration potential of open areas along a forest fragmentation gradient, we built models that relate (1) the number of seeds and the potential AGB at each landscape to the percentage of forest cover and isolation from forest, (2) bird species traits to seed rain and carbon potential contribution in each landscape (we related species-specific basis traits (gape width, body mass and frugivory dependence) to the percentage of seeds deposited in open areas and the potential AGB contributed according to the seed rain they generated in each landscape) and (3) the number of seeds deposited and carbon potential as forest distance increases.
To fit these models, we used generalized linear mixed-effects models and linear mixed-effects models by maximum likelihood estimation (Laplace approximation) using the lme4 package in R100. For seed deposition, we used a Poisson distribution for the residuals and a log link function. For AGB, we used a normal distribution for the residuals and an identity link function. For the percentage of seeds, we used a binomial distribution with a logit link function. We explored the second- and third-order polynomial forms for the exploratory variables and selected the model with a better fit. For models that relate species traits and distance, we used the landscape as a random effect. For graphical reasons, we plotted each landscape by its percentage of forest cover. We evaluated model performance by using the pseudo-R2 for generalized mixed-effect models using the function r.squaredGLMM from the package MuMIn101. All statistical significance tests used a two-tailed P value for α = 0.05. On the basis of the model predictions, we found the inflection point to identify forest cover and isolation thresholds where the predicted curves change their behaviour. To identify the inflection points, we used the function bede from the package inflection102. This function implements a geometric method to identify the points where the right and left distances of the curve to a cord that joins the extreme points are equal103.
Inclusion and ethics statement
This manuscript was developed in collaboration with local researchers who are authors of this manuscript. This manuscript has implications for regional restoration policies; therefore, local and regional research relevant to our study area was included.
Reporting summary
Further information on research design is available in the Nature Portfolio Reporting Summary linked to this article.
Data availability
All datasets used in this analysis are published and freely available in Figshare at https://doi.org/10.6084/m9.figshare.c.7086625.v1 (ref. 104). These include Data 1 to run the frugivory interactions model, Data 2 to run the frugivory movement model, Data 3 to run the GPT model and Data 4 to run the simulation code.
Code availability
The code for the models and model parameterization is published and freely available in Figshare at https://doi.org/10.6084/m9.figshare.c.7086625.v1 (ref. 104). This includes SM1, hierarchical model code for frugivory interactions; SM2, hierarchical model code for bird movement; SM3, hierarchical model code for GPT interactions; and SM4, simulation code.
References
Girardin, C. A. et al. Nature-based solutions can help cool the planet—if we act now. Nature 593, 191–194 (2021).
Miles, L. et al. Nature-Based Solutions for Climate Change Mitigation (UNEP and IUCN, 2021).
Griscom, B. W. et al. Natural climate solutions. Proc. Natl Acad. Sci. USA 114, 11645–11650 (2017).
Mulligan, J. et al. CarbonShot: Federal Policy Options for Carbon Removal in the United States (World Resources Institute, 2020).
Lippke, B. et al. The plant a trillion trees campaign to reduce global warming—fleshing out the concept. J. Sustain. For. 40, 1–31 (2021).
Dave, R. et al. Second Bonn Challenge Progress Report: Application of the Barometer in 2018 (IUCN, 2019).
Fleischman, F. et al. How politics shapes the outcomes of forest carbon finance. Curr. Opin. Environ. Sustain. 51, 7–14 (2021).
Seddon, N. et al. Understanding the value and limits of nature-based solutions to climate change and other global challenges. Phil. Trans. R. Soc. B 375, 20190120 (2020).
Schmitz, O. J. et al. Animals and the zoogeochemistry of the carbon cycle. Science 362, eaar3213 (2018).
Schmitz, O. J. et al. Trophic rewilding can expand natural climate solutions. Nat. Clim. Change 13, 324–333 (2023).
Beltrán, L. C. & Howe, H. F. The frailty of tropical restoration plantings. Restor. Ecol. 28, 16–21 (2020).
Paula Mateus, D. et al. Defaunation impacts on seed survival and its effect on the biomass of future tropical forests. Oikos 127, 1526–1538 (2018).
Estrada-Villegas, S. et al. Animal seed dispersal recovery during passive restoration in a forested landscape. Phil. Trans. R. Soc. B 378, 20210076 (2023).
Palma, A. C. et al. Enhancing plant diversity in secondary forests. Front. For. Glob. Change 3, 571352 (2020).
Herrera, J. P. Interactions between plants and primates shape community diversity in a rainforest in Madagascar. J. Anim. Ecol. 85, 982–993 (2016).
Camargo, P. H. S. A. et al. Fruit traits of pioneer trees structure seed dispersal across distances on tropical deforested landscapes: implications for restoration. J. Appl. Ecol. 57, 2329–2339 (2020).
Ramos, D. L. et al. Forest and connectivity loss drive changes in movement behavior of bird species. Ecography 43, 1203–1214 (2020).
Bello, C. et al. Defaunation affects carbon storage in tropical forests. Sci. Adv. 1, e1501105 (2015).
Osuri, A. M. & Sankaran, M. Seed size predicts community composition and carbon storage potential of tree communities in rain forest fragments in India’s Western Ghats. J. Appl. Ecol. 53, 837–845 (2016).
Cubiña, A. & Aide, T. M. The effect of distance from forest edge on seed rain and soil seed bank in a tropical pasture. Biotropica 33, 260–267 (2001).
Martínez-Garza, C. et al. Seed rain in a tropical agricultural landscape. J. Trop. Ecol. 25, 541–550 (2009).
Crouzeilles, R. et al. Ecological restoration success is higher for natural regeneration than for active restoration in tropical forests. Sci. Adv. 3, e1701345 (2017).
Selwyn, M., Pino, J. & Espelta, J. M. Disentangling the importance of intrinsic and extrinsic seed dispersal factors in forest restoration success: a global review. Restor. Ecol. 31, e13868 (2023).
Meli, P. et al. A global review of past land use, climate, and active vs. passive restoration effects on forest recovery. PLoS ONE 12, e0171368 (2017).
Reid, J. L. et al. Multi-scale habitat selection of key frugivores predicts large-seeded tree recruitment in tropical forest restoration. Ecosphere 12, e03868 (2021).
Brancalion, P. et al. Global restoration opportunities in tropical rainforest landscapes. Sci. Adv. 5, eaav3223 (2019).
Almeida-Neto, M. et al. Vertebrate dispersal syndromes along the Atlantic forest: broad-scale patterns and macroecological correlates. Glob. Ecol. Biogeogr. 17, 503–513 (2008).
Strassburg, B. B. N. et al. Global priority areas for ecosystem restoration. Nature 586, 724–729 (2020).
Araújo, M. B. Biodiversity hotspots and zones of ecological transition. Conserv. Biol. 16, 1662–1663 (2002).
Ribeiro, M. C. et al. The Brazilian Atlantic Forest: how much is left, and how is the remaining forest distributed? Implications for conservation. Biol. Conserv. 142, 1141–1153 (2009).
Atlantic Forest Restoration Pact (AFRP, 2023); https://www.pactomataatlantica.org.br/
Brancalion, P. H. et al. Governance innovations from a multi-stakeholder coalition to implement large-scale forest restoration in Brazil. World Dev. Perspect. 3, 15–17 (2016).
Melo, F. P. L. et al. Priority setting for scaling-up tropical forest restoration projects: early lessons from the Atlantic Forest Restoration Pact. Environ. Sci. Policy 33, 395–404 (2013).
Reid, J. L., Fagan, M. E., and & Zahawi, R. A. Positive site selection bias in meta-analyses comparing natural regeneration to active forest restoration. Sci. Adv. 4, eaas9143 (2018).
González-Castro, A., Yang, S. & Carlo, T. A. How does avian seed dispersal shape the structure of early successional tropical forests? Funct. Ecol. 33, 229–238 (2019).
Palma, A. C. et al. Dispersal and recruitment limitations in secondary forests. J. Veg. Sci. 32, e12975 (2021).
Chazdon, R. L. et al. Carbon sequestration potential of second-growth forest regeneration in the Latin American tropics. Sci. Adv. 2, e1501639 (2016).
Aavik, T. & Helm, A. Restoration of plant species and genetic diversity depends on landscape-scale dispersal. Restor. Ecol. 26, S92–S102 (2018).
Hewitt, N. & Kellman, M. Tree seed dispersal among forest fragments: II. Dispersal abilities and biogeographical controls. J. Biogeogr. 29, 351–363 (2002).
Ibáñez, I. et al. Assessing the integrated effects of landscape fragmentation on plants and plant communities: the challenge of multiprocess–multiresponse dynamics. J. Ecol. 102, 882–895 (2014).
Arroyo-Rodríguez, V. et al. Landscape-scale forest cover drives the predictability of forest regeneration across the Neotropics. Proc. R. Soc. B 290, 20222203 (2023).
Crouzeilles, R. et al. Achieving cost-effective landscape-scale forest restoration through targeted natural regeneration. Conserv. Lett. 13, e12709 (2020).
Melo, I. et al. A review of threshold responses of birds to landscape changes across the world. J. Field Ornithol. 89, 303–314 (2018).
Banks-Leite, C. et al. Using ecological thresholds to evaluate the costs and benefits of set-asides in a biodiversity hotspot. Science 345, 1041–1045 (2014).
Shennan‐Farpón, Y., Visconti, P. & Norris, K. Detecting ecological thresholds for biodiversity in tropical forests: knowledge gaps and future directions. Biotropica 53, 1276–1289 (2021).
Morán-López, T. et al. Forest recovery through applied nucleation: effects of tree islet size and disperser mobility on tree recruitment in a temperate landscape. For. Ecol. Manage. 550, 121508 (2023).
Tamme, R. et al. Predicting species’ maximum dispersal distances from simple plant traits. Ecology 95, 505–513 (2014).
Chazdon, R. L. et al. The potential for species conservation in tropical secondary forests. Conserv. Biol. 23, 1406–1417 (2009).
González-Varo, J. P., Arroyo, J. M. & Jordano, P. The timing of frugivore-mediated seed dispersal effectiveness. Mol. Ecol. 28, 219–231 (2019).
Silva, W. R. et al. Inducing seed dispersal by generalist frugivores: a new technique to overcome dispersal limitation in restoration. J. Appl. Ecol. 57, 2340–2348 (2020).
Vergne, D. C. et al. Isolated trees with high crown coverage and densities increase pasture seed rain. Acta Bot. Brasilica 30, 486–494 (2016).
Herrera, J. M. & García, D. The role of remnant trees in seed dispersal through the matrix: being alone is not always so sad. Biol. Conserv. 142, 149–158 (2009).
Pizo, M. A. & dos Santos, B. T. Frugivory, post-feeding flights of frugivorous birds and the movement of seeds in a Brazilian fragmented landscape. Biotropica 43, 335–342 (2011).
Sasal, Y. & Morales, J. M. Linking frugivore behavior to plant population dynamics. Oikos 122, 95–103 (2013).
Synes, N. W. et al. Prioritising conservation actions for biodiversity: lessening the impact from habitat fragmentation and climate change. Biol. Conserv. 252, 108819 (2020).
Fedriani, J. M. et al. Assisting seed dispersers to restore oldfields: an individual-based model of the interactions among badgers, foxes and Iberian pear trees. J. Appl. Ecol. 55, 600–611 (2018).
Catterall, C. & Harrison, D. A. Rainforest Restoration Activities in Australia’s Tropics and Subtropics (Rainforest CRC, 2006).
Morales, J. M. & Morán López, T. Mechanistic models of seed dispersal by animals. Oikos 2022, e08328 (2021).
Quitián, M. et al. Functional responses of avian frugivores to variation in fruit resources between natural and fragmented forests. Funct. Ecol. 33, 399–410 (2019).
Lopes Melo, F. P., Lemire, D. & Tabarelli, M. Extirpation of large-seeded seedlings from the edge of a large Brazilian Atlantic Forest fragment. Ecoscience 14, 124–129 (2007).
Herrero-Jáuregui, C. et al. Past and present effects of habitat amount and fragmentation per se on plant species richness, composition and traits in a deforestation hotspot. Biol. Conserv. 276, 109815 (2022).
Carrière, S. M. et al. Seed rain beneath remnant trees in a slash-and-burn agricultural system in southern Cameroon. J. Trop. Ecol. 18, 353–374 (2002).
Jordano, P. in Seeds: The Ecology of Regeneration in Plant Communities (ed. Fenner, M.) 125–165 (CABI, 2000).
Bello, C. et al. Atlantic frugivory: a plant–frugivore interaction data set for the Atlantic Forest. Ecology 98, 1729 (2017).
Grman, E. et al. Dispersal and establishment filters influence the assembly of restored prairie plant communities. Restor. Ecol. 23, 892–899 (2015).
Xu, C. et al. Herbivory limits success of vegetation restoration globally. Science 382, 589–594 (2023).
Souza, Y. et al. Large mammalian herbivores modulate plant growth form diversity in a tropical rainforest. J. Ecol. 110, 845–859 (2022).
Villar, N. Trophic cascades help restore vegetation. Science 382, 516–517 (2023).
Wang, X., Tang, J., and & Whitty, M. DeepPhenology: estimation of apple flower phenology distributions based on deep learning. Comput. Electron. Agric. 185, 106123 (2021).
Diao, C. & Li, G. Near-surface and high-resolution satellite time series for detecting crop phenology. Remote Sens. 14, 1957 (2022).
Gray, R. E. J. & Ewers, R. M. Monitoring forest phenology in a changing world. Forests 12, 297 (2021).
Armstrong, J. B. et al. Resource waves: phenological diversity enhances foraging opportunities for mobile consumers. Ecology 97, 1099–1112 (2016).
Wild, T. A. et al. Internet on animals: Wi‐Fi‐enabled devices provide a solution for big data transmission in biologging. Methods Ecol. Evol. 14, 87–102 (2022).
Banerjee, P. et al. Plant–animal interactions in the era of environmental DNA (eDNA)—a review. Environ. DNA 4, 987–999 (2022).
Kress, W. J. et al. DNA barcodes for ecology, evolution, and conservation. Trends Ecol. Evol. 30, 25–35 (2015).
Evans, D. M. et al. Merging DNA metabarcoding and ecological network analysis to understand and build resilient terrestrial ecosystems. Funct. Ecol. 30, 1904–1916 (2016).
González-Varo, J. P. et al. Unravelling seed dispersal through fragmented landscapes: frugivore species operate unevenly as mobile links. Mol. Ecol. 26, 4309–4321 (2017).
Shryock, D. F. et al. Landscape genetic approaches to guide native plant restoration in the Mojave Desert. Ecol. Appl. 27, 429–445 (2017).
Benedicto‐Royuela, J. et al. What is the value of biotic seed dispersal in post‐fire forest regeneration? Conserv. Lett. 17, e12990 (2023).
Yoshikawa, T., Kawakami, K. & Masaki, T. Allometric scaling of seed retention time in seed dispersers and its application to estimation of seed dispersal potentials of theropod dinosaurs. Oikos 128, 836–844 (2019).
Stiles, F. G., Altshuler, D. L. & Dudley, R. Wing morphology and flight behavior of some North American hummingbird species. Auk 122, 872–886 (2005).
Stiles, F. G. Phylogenetic constraints upon morphological and ecological adaptation in hummingbirds (Trochilidae): why are there no hermits in the paramo. Ornitol. Neotrop. 15, 191–198 (2004).
Ovaskainen, O. et al. How to make more out of community data? A conceptual framework and its implementation as models and software. Ecol. Lett. 20, 561–576 (2017).
Rohr, R. P. et al. Modeling food webs: exploring unexplained structure using latent traits. Am. Nat. 176, 170–177 (2010).
Traveset, A., Robertson, A. & Rodríguez-Pérez, J. in Seed Dispersal: Theory and Its Application in a Changing World (ed. Dennis, A. J.) 78–103 (CABI, 2007).
Schupp, E. W., Jordano, P. & Maria Gomez, J. Seed dispersal effectiveness revisited: a conceptual review. N. Phytol. 188, 333–353 (2010).
Charalambidou, I., Santamaria, L. & Langevoord, O. Effect of ingestion by five avian dispersers on the retention time, retrieval and germination of Ruppia maritima seeds. Funct. Ecol. 17, 747–753 (2003).
Ovaskainen, O. et al. Joint species movement modeling: how do traits influence movements? Ecology 100, e02622 (2019).
Jetz, W. et al. Global distribution and conservation of evolutionary distinctness in birds. Curr. Biol. 24, 919–930 (2014).
Paradis, E. et al. ape: Analyses of Phylogenetics and Evolution R package version 5.7 (2019).
Gelman, A. et al. R-squared for Bayesian regression models. Am. Stat. 73, 307–309 (2019).
Carpenter, B. et al. Stan: a probabilistic programming language. J. Stat. Softw. 76, 10.18637 (2017).
Baddeley, A. & Turner, R. spatstat: an R package for analyzing spatial point patterns. J. Stat. Softw. 12, 1–42 (2005).
Hijmans, R. J. et al. raster: Geographic Data Analysis and Modeling R package version (2015).
Barros, F. M. et al. Matrix type and landscape attributes modulate avian taxonomic and functional spillover across habitat boundaries in the Brazilian Atlantic Forest. Oikos 128, 1600–1612 (2019).
Pompeu, P. V. Composição e Estrutura de uma Floresta Ombrófila Densa Ao Longo de um Gradiente Altitudinal na Serra da Mantiqueira, Minas Gerais (ed. Mestrado, D. d.) (Univ. Federal de Lavras, 2011).
Robbins, C. S. Effect of time of day on bird activity. Stud. Avian Biol. 6, 275–286 (1981).
Chave, J. et al. Tree allometry and improved estimation of carbon stocks and balance in tropical forests. Oecologia 145, 87–99 (2005).
Higuchi, N. et al. Aboveground biomass estimate for Amazonian dense tropical moist forests. Mem. Fac. Agric. Kagoshima 30, 43–54 (1994).
Bates, D., Maechler, M. & Bolker, B. lme4: Linear Mixed-Effects Models Using S4 Classes R package version 0.999375-39 (2011); http://CRAN.R-project.org/package=lme4
Bartoń, K. MuMIn: Multi-Model Inference R package version 1.47. 1 (2022).
Christopoulos, D. T. inflection: Finds the Inflection Point of a Curve R package version 1.3.6 (2022).
Christopoulos, D. T. On the efficient identification of an inflection point. Int. J. Math. Sci. Comput. 6, 13–20 (2016).
Bello, C. Frugivores enhance potential carbon recovery in fragmented landscapes. Figshare https://doi.org/10.6084/m9.figshare.c.7086625.v1 (2024).
Acknowledgements
C.B. was supported by ETH Career Seed Award 22-2 Seed 010. C.B., D.H.D. and T.W.C. were supported by DOB Ecology and the Bernina Foundation. T.M.-L. was supported by a Juan de la Cierva Incorporación (IJC2020-043765-I), funded by the Spanish Ministry of Science and by the government of the Asturias Principality (REWILDING project, FEDER, GRUPIN (AYUD/2021/51261)). D.L.R. was funded by Coordination for the Improvement of Higher Education Personnel (CAPES). D.H.D. was supported by a fellowship from the Alexander von Humboldt Stiftung, Germany. We thank J. van den Hoogen, C. Chrysa and A. C. Palma for their contribution.
Funding
Open access funding provided by Swiss Federal Institute of Technology Zurich.
Author information
Authors and Affiliations
Contributions
C.B., T.W.C., D.L.R., T.M.-L. and D.H.D. conceived and designed the study. C.B., D.L.R. and T.M.-L. performed the experiments and analysed the data. D.L.R., M.A.P. and T.M.-L. contributed material and analysis tools. C.B., D.H.D., D.L.R. and T.W.C. drafted the paper with input from all co-authors. All authors read and approved the final version of the paper.
Corresponding author
Ethics declarations
Competing interests
The authors declare no competing interests.
Peer review
Peer review information
Nature Climate Change thanks Ana Palma, J. Leighton Reid and the other, anonymous, reviewer(s) for their contribution to the peer review of this work.
Additional information
Publisher’s note Springer Nature remains neutral with regard to jurisdictional claims in published maps and institutional affiliations.
Extended data
Extended Data Fig. 1 Methodological diagram.
The upper panel shows the structure of the models developed for each process: source limitation (a), frugivory interactions (b), animal movement (c), seed retention (d). In the model structure, blue squares represent the data and the green circles the parameters estimated by the model. We can observe a hierarchical structure of the models where phylogeny and traits indirectly influence the mean response of the species, while variables such as trait matching or cover type directly influence the processes. The parameters estimated in each model, and the plant and bird composition in each landscape, are intergraded in simulation of seed rainfall in each landscape cover (e). Figure adapted with permission from ref. 18, AAAS.
Supplementary information
Supplementary Information
Supplementary Fig. 1, Tables 1–6 and references for fruit consumption.
Rights and permissions
Open Access This article is licensed under a Creative Commons Attribution 4.0 International License, which permits use, sharing, adaptation, distribution and reproduction in any medium or format, as long as you give appropriate credit to the original author(s) and the source, provide a link to the Creative Commons licence, and indicate if changes were made. The images or other third party material in this article are included in the article’s Creative Commons licence, unless indicated otherwise in a credit line to the material. If material is not included in the article’s Creative Commons licence and your intended use is not permitted by statutory regulation or exceeds the permitted use, you will need to obtain permission directly from the copyright holder. To view a copy of this licence, visit http://creativecommons.org/licenses/by/4.0/.
About this article
Cite this article
Bello, C., Crowther, T.W., Ramos, D.L. et al. Frugivores enhance potential carbon recovery in fragmented landscapes. Nat. Clim. Chang. (2024). https://doi.org/10.1038/s41558-024-01989-1
Received:
Accepted:
Published:
DOI: https://doi.org/10.1038/s41558-024-01989-1