Abstract
Soil CH4 fluxes are driven by CH4-producing and -consuming microorganisms that determine whether soils are sources or sinks of this potent greenhouse gas. To date, a comprehensive understanding of underlying microbiome dynamics has rarely been obtained in situ. Using quantitative metatranscriptomics, we aimed to link CH4-cycling microbiomes to net surface CH4 fluxes throughout a year in two grassland soils. CH4 fluxes were highly dynamic: both soils were net CH4 sources in autumn and winter and sinks in spring and summer, respectively. Correspondingly, methanogen mRNA abundances per gram soil correlated well with CH4 fluxes. Methanotroph to methanogen mRNA ratios were higher in spring and summer, when the soils acted as net CH4 sinks. CH4 uptake was associated with an increased proportion of USCα and γ pmoA and pmoA2 transcripts. We assume that methanogen transcript abundance may be useful to approximate changes in net surface CH4 emissions from grassland soils. High methanotroph to methanogen ratios would indicate CH4 sink properties. Our study links for the first time the seasonal transcriptional dynamics of CH4-cycling soil microbiomes to gas fluxes in situ. It suggests mRNA transcript abundances as promising indicators of dynamic ecosystem-level processes.
Similar content being viewed by others
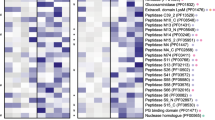
Introduction
CH4 is a powerful greenhouse gas [1]. Between 41% and 53% of global CH4 emissions derive from aquatic systems. Therein freshwater wetlands are the largest single source, emitting about 138–165 Tg CH4 yr−1 [2, 3]. Since 1700, between 54% and 57% of the wetlands were lost due to drainage to gain agricultural land, such as grasslands [4, 5]. Drainage lowers the water table, altering water content and oxygen availability. These altered soil physical conditions, in turn, substantially affect the soil microbiota and activity and thus the soils’ greenhouse gas fluxes [6, 7]. Drained former wetlands are a large source of CO2 but can also emit substantial amounts of CH4, depending on their dynamic hydrological status throughout the year [4, 5].
More than two-thirds of global CH4 emissions derive from microbial production [8]. CH4-producing microbes (i.e., methanogens) are mostly anaerobic Archaea that inhabit anoxic environments [8, 9]. Four types of methanogens can be characterized according to their substrate specificity. Acetoclastic methanogens utilize acetate, hydrogenotrophic methanogens utilize H2/CO2 and formate, and methylotrophic methanogens utilize methanol/methylamines to form CH4 [9]. Recently, methoxydotrophic methanogens that utilize methoxylated aromatic compounds were proposed as a novel methanogenic group [10, 11]. In soils, acetoclastic and hydrogenotrophic methanogens are considered the predominant sources of CH4 [9, 12]. However, recent research indicates that methanogenesis from methylated compounds also contributes to CH4 emissions from soils and wetlands [13, 14].
Up to 90% of CH4 produced in oxygen-limited soils can be mitigated through oxidation by aerobic methane-oxidizing Bacteria (MOB) within the lineages Alphaproteobacteria, Gammaproteobacteria, and Verrucomicrobia [15,16,17]. CH4 oxidation can also be conducted anaerobically by Bacteria of the NC10 phylum and Archaea in the ANME group that couple oxidation of CH4 to the reduction of other electron acceptors such as nitrite (NC10), nitrate (ANME-2d), or ferric iron [18,19,20]. Aerobic methanotrophs are considered the main oxidizers in wetland soils since alternative electron acceptors favoring anaerobic methanotrophs are often scarce in wetland soils. Tracing stable isotopes and radioisotopes can link CH4 consumption to active methanotrophs [21,22,23,24,25]. For instance, incubating soil cores with 13C-CH4 identified γ-proteobacterial subgroups as the main active methanotrophs in a riparian floodplain [22]. Additionally, methanotrophs provide the only known biological sink for atmospheric CH4 [26]. However, it is not fully understood which microorganisms oxidize CH4 at atmospheric concentrations in soils. Bacteria of upland soil clusters (USC)α and USCγ have been identified as likely important atmospheric MOBs in upland soils [15, 27,28,29], while well-known methanotrophic lineages may also oxidize atmospheric CH4 in anoxic paddy soils [30]. A study using stable-isotope labeled CH4 identified type II methanotrophs related to Methylocapsa acidophila active in grassland and forest soils at low CH4 concentrations [25].
Presumably, the combined net activities of methanogens and methanotrophs determine whether wetland soils act as net sources or sinks for CH4 [31]. However, linking CH4-cycling microbiome dynamics of soils in situ to CH4 fluxes, especially at the transcriptional level, has rarely been achieved [32]. DNA- and RNA-based meta-omics techniques have provided insight into the microbiome compositions of soils. However, DNA is long-term stable; extracted soil DNA may therefore partially originate from persistent extracellular DNA of dead organisms [33, 34]. In contrast, ribosomal RNA (rRNA) acts as a proxy for ribosomes. Even though dormant cells can contain high loads of ribosomes [35, 36], RNA-SIP studies [37, 38] indicate that approximately 94% of microbial taxa in soil are active and synthesize new rRNA [39]. Still, rRNA content does not necessarily reflect the gene expression. Hence, although rRNA is a good proxy for potential active soil microbiome, it may not relate well to ecosystem processes. The simultaneous sequencing of mRNA and rRNA potentially can overcome this issue [40] because messenger RNA (mRNA), can serve as a proxy for transcriptional activity. Other metatranscriptome studies indicate that mRNA is more responsive to environmental factors than rRNA [41, 42]. For instance, methanogen-related mRNA, but not SSU rRNA, decreased in soil microcosms exposed to drought [43]. The relationship between the abundances of rRNA and mRNA of CH4-cycling microbes and CH4 fluxes has not been studied in situ. We thus aim to explore differences between small subunit (SSU) rRNA and mRNA transcripts of the CH4-cycling microbiomes and their links to gas fluxes.
Another drawback of meta-omics techniques is that they usually yield only relative abundances. However, the relationship between absolute abundances and relative abundances is not predictable [44]. It is thus challenging to relate ecosystem processes to relative abundances. Studies have applied absolute quantification for metatranscriptomes in marine microbiomes [45, 46]. Recently, a quantification approach that uses total RNA to infer absolute from relative abundance has been developed for metatranscriptomics [47].
In this study, we aimed to link transcriptional dynamics of CH4-cycling microbiomes to CH4 fluxes in two grassland soils. These soils were wetlands in the past but have been drained for agricultural use several decades ago. We used quantitative metatranscriptomics to analyze ribosomal rRNA and mRNA [40, 47] of 60 soil samples taken from different soil depths during autumn, winter, spring, and summer. In addition, we measured CH4 and CO2 net surface fluxes from the two sites. We aimed to (a) evaluate the RNA content of the soils as a marker for microbial activity, (b) examine the CH4 fluxes of the two in grasslands throughout a year, (c) study the composition and abundance of SSU rRNA and mRNA transcripts of CH4-cycling microbes, and (d) link microbiome composition of CH4-cycling organisms to net surface CH4 fluxes across seasons.
Materials and methods
Site description
The experiment was conducted in the framework of the Biodiversity Exploratories project for long-term functional ecosystem research [48]. Samples were taken at two grassland sites (LI and HI) located in the Biosphere Reserve „Schorfheide-Chorin“ (Supplementary Table S1). Both sites are drained peatlands with a histosolic soil type (according to WRB 2015 [49]). The upper 30 cm of the peat soils was highly degraded. The two sites differ in the intensity of grassland management; the low land-use intensity site (LI) was mowed once or twice a year, while the high land-use intensity site (HI) was grazed by cows (400–700 livestock units * grazed days ha-1 y-1) and additionally mowed sometimes once a year. Vegetation on LI was dominated by Poa trivialis (60%) and Alopecurus pratensis (25%); vegetation on HI was dominated by Poa pratensis aggr. (32 %), Trifolium repens (15%) and Agrostis stolonifera (10%).
Soil Sampling
On each site, an area of 1 m × 7 m was sampled at all four seasons: autumn (11/09/2017), winter (03/08/2018), spring (05/30/2018), and summer (09/13/2018). At each sampling date, three spatial replicate samples were taken between 12:00 and 13:00 at each site from the upper 10 cm and the 20–30 cm layer. Each soil sample was a mixture of the respective soil layer from three soil cores, taken close to each other (5–10 cm). The replicates were located at least 1 m apart from each other. At each seasonal sampling, the replicates were taken at least 1 m apart from replicates taken during the previous sampling campaigns. In spring, additional samples were taken at sunrise (05:00) and sunset (21:30), but only at the HI site. Samples for RNA, ammonium (NH4+), and nitrate (NO3−) extraction were immediately frozen at −80 °C and subsequently stored as follows: RNA: −80 °C, NH4+, and NO3− −20 °C. Samples for determination of Cmic, Nmic, pH, and soil water content were transported on ice and subsequently stored at −20 °C. Redox potentials were measured with Mansfeld redox electrodes with an Ag/AgCl-reference electrode and a handheld ORP-meter GMH3531 (ecoTech, Bonn, Germany). For equilibration. the electrodes were placed in the soil 24 h before sampling. Redox potentials were measured at soil depths of 5 cm and 25 cm.
Determination of soil properties
Gravimetric soil water content was determined by drying 3–6 g soil at 65 °C to constant weight. Soil pH was determined by mixing 10 g dried sieved soil with 25 ml 0.01 M CaCl2 solution; pH of the suspension was then measured with a glass electrode (pH Electrode LE438, Mettler Toledo, Columbus, OH, USA). For total carbon and total nitrogen, samples were sieved (< 2 mm) and air-dried, ground in a ball mill (RETSCH MM200, Retsch, Haan, Germany), and analyzed in an elemental analyzer (VarioMax, Hanau, Germany) at 1100 °C. Inorganic carbon was determined with the same elemental analyzer after the organic carbon had been removed by combustion of soil samples at 450 °C for 16 h. Organic carbon concentration was calculated as the difference between total carbon and inorganic carbon. Microbial biomass carbon (Cmic) and nitrogen (Nmic) were determined by the chloroform-fumigation-extraction method (CFE) [50]. For this, frozen soils were thawed (at 4 °C for 10 h), then 5 g field moist soils were fumigated with ethanol-free CHCl3 for 24 h in a desiccator. C and N were extracted with 40 ml 0.5 M K2SO4, shaken horizontally (30 min, 150 rpm), and centrifuged (30 min, 4400 g) to separate extract from the soil. Non-fumigated soil samples were treated identically. Aliquots of the extracts were dissolved (1:4 extract:deionized. H2O) and measured on a TOC/TN analyzer (Multi N/C 2100S, Analytik Jena AG, Jena, Germany). A kEC factor [51] and a kEN factor [52] were used to calculate Cmic and Nmic, respectively. The organic C and N content determined from non-fumigated samples were used as a measure for the extractable C (EOC) and N (EN) which can be considered as microbially available resource in soil [53]. Mineral nitrogen in the forms of ammonium (NH4+) and nitrate (NO3−) was determined in the non-fumigated, non-diluted extracts with an AutoAnalyzer 3 (Bran & Luebbe, Norderstedt, Germany).
Gas fluxes
On each sampling date, gas emissions were measured with four closed chambers per site. With each chamber, the measurements were repeated four to six times per day and site, resulting in 15–24 net surface rate measurements. Excessive vegetation was removed before pressing the stainless steel chambers (A = 150 cm2, V = 1800 ml) into the soil [54]. The chambers had a sharp-edged bottom, which allowed the installation in the organic soils without compacting the soil. Gas samples (12 ml) were taken with syringes from the headspace immediately, 20, 40, and 60 min after closing the chambers via a three-way stopcock, and transferred into pre-evacuated exetainers (5.9 ml, Labco Lt, UK). Gas concentrations were measured on an Agilent 7890 gas chromatograph equipped with a flame ionization detector (for CH4) coupled with a methanizer (for CO2) (Agilent Technologies Inc., Santa Clara, CA, USA). Gas flux rates were calculated by the slope of the regression line of a linear regression of the gas concentration against time [27].
RNA extraction, library preparation, and sequencing
Total nucleic acids were extracted using a phenol/chloroform/isoamylalcohol protocol [40]. The extracts were subsequently treated with DNase to remove DNA (DNase I, Zymo Research, Freiburg, Germany). RNA concentrations were measured with the Qubit RNA HS Assay Kit (Qubit3.0 Fluorometer, Invitrogen, Waltham, MA, USA.). RNA extracts were cleaned with the MEGAclear kit (Thermo Fisher Scientific, Waltham, MA, USA); the quality of the RNA was verified by agarose gel electrophoresis and bioanalyzer (2100 Bioanalyzer, Agilent, Santa Clara CA, USA). We enriched the mRNA fraction and diluted inhibitory substances in the RNA extracts using the MessageAmp II-Bacteria RNA Amplification Kit (Thermo Fisher Scientific, MA, USA, input: 12.5 ng RNA). This method was previously validated for the preparation of metatranscriptomes [55]. Sequencing libraries were prepared with NEBNext Ultra II RNA Library Prep Kit for Illumina (New England Biolabs, Ipswich, MA, USA; input 60 ng). Manufacturer’s instructions were followed except for Step 4, where fragmentation time was adjusted to 3 min and a size selection step with HighPrep PCR beads (MagBio Genomics Inc., Gaithersburg, USA) was introduced (desired insert size 250 bp). Libraries were paired-end sequenced with a NextSeq 550 System using the NextSeq 500/550 High Output Kit v2.5 (300 Cycles) (Illumina, San Diego, CA, USA).
Bioinformatic processing and statistics
Reverse and forward sequences were overlapped with a minimum overlap of 10 or 5 bp with FLASH [56]. The sequences were filtered to a minimum mean quality score of 25 with PrinseqLite [57]. Sequences were then sorted into SSU rRNA, LSU rRNA, and non-rRNA fractions with SortMeRNA [58]. The SSU rRNA fraction was randomly subsampled to 200000 sequences with USEARCH [59]. Sequences were taxonomically classified against the SilvaMod128 databases [60] with BlastN [61] using a lowest common ancestor (LCA) algorithm in MEGAN (min score 155; top percent 2.0; min support 1 [62]). The non-rRNA fraction was aligned against the NCBI_nr database (retrieved 12/03/2020) with Diamond [63]. The sequences were taxonomically and functionally aligned with LCA in MEGAN (2011, min score 155; top percent 4; min support 1 [62]). Absolute abundances were calculated from read counts according to Söllinger et al. [47]. This calculation integrates the relative read abundance obtained from metatranscriptomics with the amount of mRNA and SSU rRNA extracted from the soil, respectively, and the average number of transcripts per µg RNA. At mRNA level, methanogenesis transcripts refer to sequences assigned to the SEED category “methanogenesis”. Methanotrophy transcripts refer to sequences assigned to the SEED category “Particulate methane monooxygenase (pMMO)”. To classify pmoA sequences, the non-rRNA fraction was searched against a pmoA database [64] and taxonomically classified with MEGAN as described in reference [64]. To assess the transcriptional activity of CH4-cycling microbes throughout the years, we binned mRNAs taxonomically classified as methanogens (Euryarchaeota) and alpha and gammaproteobacterial methanotrophs, respectively to then analyze the functionally assigned mRNAs using SEED and KEGG.
Statistical analyses were performed in R [65]. Distance-based redundancy analysis was performed on the Bray–Curtis dissimilarity matrix read counts of the 60 samples (function “dbrda” in the vegan package [66]). Counts were Hellinger-transformed beforehand. We tested the following parameters: site (HI; LI), depth (“0–10 cm”, “20–30 cm”), season (“autumn”,”winter”, “spring”, “summer”), temperature, water content, nitrite, and nitrate. Continuous variables were z-scaled. The difference of transcript abundances and the ratio of methanotrophs to methanogens between seasons at one and the same site was assessed by ANOVA and subsequent post-hoc Tukey’s test, resulting in adjusted p-values. We used the arithmetic mean of methanogenesis and methanotroph transcript abundances from the upper (0–10 cm) and the lower (20–30 cm) of one sample. Significant differences between seasons were identified with the R package “multcompView” with p-adjusted <0.05 [67].
Results and discussion
Highly dynamic CH4 fluxes across the year
We measured net surface fluxes from two grasslands across one day during autumn, winter, spring, and summer to assess their seasonal variation, in particular CH4. Daytime did not affect CH4 emissions (Supplementary Fig. 1A). In contrast, CH4 and CO2 fluxes were highly dynamic throughout the year (Fig. 1). While the soils emitted CH4 in autumn and winter (7.0 and 6.9 mg C m−2d−2, in autumn in LI and HI, respectively), they took up CH4 in spring and summer (−0.9 and −0.8 mg C m−2d−2, in summer in LI and HI, respectively) (Fig. 1A). CO2 fluxes showed an opposite trend, with higher CO2 emissions in spring and summer than in autumn and winter (Fig. 1B). The opposing trends of CO2 and CH4 fluxes reflected the changes in soil physicochemical properties across the year (Fig. 1C, D, Supplementary Table S2). Especially water content and temperature were likely the key factors in regulating gas turnover. High water content and low redox potentials in autumn and winter (Fig. 1C, Supplementary Table S2) likely favored anaerobic microbial processes, such as methanogenesis, while at the same time hampering aerobic microbial processes such as respiration (Supplementary Fig. 2). Low temperatures in winter likely resulted in smaller CH4 fluxes compared to autumn (Fig. 1A, D). In spring and summer, soils had lower water content and positive redox potential favoring aerobic over anaerobic degradation processes. Generally, mean CO2 net surface emissions were about 1.5 times higher than IPCC default emission factors [68, 69]. Our observed higher emissions may have been due to the degraded peat at the studied site. Soils with highly disturbed peat have been reported to have higher CO2 emissions than less degraded peat soils [70]. Next to soil water content, also temperature may have caused relatively high CO2 emissions as spring and summer 2018 were dry and hot compared to the long-term average. High temperatures increase organic matter decomposition and CO2 emissions [71, 72].
Gas fluxes of CH4 (A), CO2 (B), gravimetric soil water content (C), and temperature (D) in the soils of the grassland site with low (yellow, LI) and high (turquoise, HI) land-use intensity in autumn (aut) 2017 and winter (win), spring (spr), and summer (sum) 2018. In A and B, one point shows the average of 4–6 repeated measurements of one chamber across one day; the mean and median are indicated with a black and gray line, respectively. In C, one point represents the mean and standard deviation of three replicates taken at noon, n = 3. In D, points represent the temperature measured at 12:00 in 5 cm and 20 cm soil depth, respectively.
Net surface CH4 emissions rates in autumn and winter were lower compared to IPCC default emission factors [68]. However, we measured emissions at only four days and may have not accounted for high emissions after heavy rainfall events. Net CH4 uptake rates in spring and summer were in the range of other herbaceous and temperate ecosystems (0.36 and 0.47 ± 0.63 mg C m−2d−1) [73, 74] and higher than in pastures (mean 0.05 mg C m−2d−1) [74]. The beginning drought in 2018 caused low soil water content (Supplementary Table S2), favoring CH4 oxidation. The soil water content of the upper layer was mostly within the optimal range for atmospheric CH4 oxidation [75].
Our results underscore the high temporal variability of greenhouse gas emissions from temperate drained peatlands and their dependence on dynamic soil physicochemical properties, like temperature and soil moisture, which are themselves linked to seasons. Moreover, depending on the time of the year and conditions in the soil such sites can be net sinks for CH4 as well as net sources. This versatility regarding CH4 sink and source functions requires further long-term monitoring of such groundwater-impacted and organic-rich drained grassland soils in postglacial landscapes to ensure proper consideration in global budgets.
Linking metatranscriptomics and microbial biomass
We quantified soil total RNA content to examine if it reflects microbial biomass in the soils. Total RNA and Nmic and Cmic were determined from 60 top- and subsoil samples. They exhibited similar dynamics across seasons. Overall, total RNA per gram soil was positively correlated with both Nmic and Cmic (rNmic = 0.68, rCmic = 0.54, p < 0.001, Fig. 2, Supplementary Fig. 3). The RNA content correlated better with Nmic, than with Cmic, likely due to the high nitrogen content of the RNA. This finding supports the validity of RNA as a proxy for living microorganisms and the use of RNA content to infer transcript abundances per gram soil from relative transcript abundances obtained in metatranscriptomics [47]. Through this quantitative approach, one can overcome challenges typically associated with the interpretation of relative abundance data in ‘meta-omics’ datasets. A recent study used this quantitative approach and found that absolute transcript abundance correlated better to ecosystem processes than relative transcript frequencies [47].
Correlation between RNA content and microbial nitrogen content (Nmic) per g soil dry weight (DW) in the soils of the grassland sites with low (LI, yellow) and high (HI, turquoise) land-use intensity. Linear regression RNA = 1.8182 + 0.0197 Nmic, df = 58 (dashed lines show 95% CI). The “r” denotes the Pearson correlation coefficient. Significance codes: ***p < 0.001, n = 60.
Spatial and seasonal dynamics in CH4-cycling (micro-)biomes
High-throughput sequencing of metatranscriptomes yielded approximately 20 million paired-end reads per sample [76]. Three-domain analysis based on SSU rRNA reads revealed that the (micro-)biomes of the 60 samples were dominated by Bacteria, followed by eukaryotes and Archaea (Supplementary Tables S3 and S4, Supplementary Fig. 4). The community composition of all taxa in the soil samples exhibited a clear site- and depth-specific pattern (Fig. 3A), with site and depth explaining 20.0% and 19.6% of the variance, respectively (p < 0.001, Supplementary Table S5). Site-specific differences are likely attributed to site-specific soil properties, such as pH, texture, organic carbon, and nitrogen content, and land-use intensity (Supplementary Table S1). Depth is generally considered to be associated with differences in oxygen and nutrient availability. Eukaryotes were usually higher abundant in the upper soil layer, compared with the lower soil layer (Supplementary Fig. 4).
Distance-based redundancy analysis (dbRDA) of the Bray–Curtis dissimilarity matrix of all 39,854 bacterial, archaeal and eukaryotic taxa (A) and the 287 CH4-cycling Archaea and Bacteria (B) in the soils of the grassland sites with low (LI, yellow) and high (HI, turquoise) land-use intensity from the upper (0–10 cm) and the deeper soil layer (20–30 cm) taken in autumn (aut) 2017 and winter (win), spring (spr) and summer (sum) 2018. Samples from autumn, winter, spring, and summer are depicted as circles, diamonds, upward-pointing triangles, and downward-pointing triangles, respectively.
The composition of CH4-cycling microbes was also influenced by site, season, and depth (Fig. 3B). Site had the most explanatory power (14.0%, p < 0.001), but season, depth, and water content accounted for 6.5%, 5.7%, and 5.3% (p < 0.001) of the variance, respectively (Supplementary Table S6). Thus, the seasonal variability of the CH4 fluxes was accompanied by seasonal changes in CH4-cycling community composition. The seasonal effect likely resulted from varying precipitation, water table depth, and plant growth activity throughout the year. Especially the drought in spring and summer may have strongly affected the CH4-cycling microorganisms by lowering the soil water content. Oxygen diffusion into dry soils is much faster than into water-saturated soils, resulting in a higher O2 availability, which, in turn, is a fundamental factor shaping CH4-cycling community composition [77].
Methanogen community composition and transcriptional activity
We aimed to evaluate if SSU rRNA and mRNA abundances of CH4-cycling microbes reflected the seasonal changes in CH4 fluxes of the soils. For this purpose, we integrated the total RNA content and metatranscriptomes [47] to infer methanogen SSU rRNA and mRNA transcript abundances per gram soil (Fig. 4A, C). Generally, methanogen SSU rRNA abundances were higher in autumn and winter and the deeper soil layer, with abundances up to 1.4 ×1010 transcripts g−1 soil (Fig. 4A). Most methanogen families in the soils were class II methanogens, e.g., Methanosarcinaceae, Methanosaetaceae (now Methanotrichaceae) (Fig. 4B) which generally possess more antioxidant features than class I methanogens [78]. The predominance of class II methanogens likely reflected the dynamic water and redox status across seasons (Fig. 1C, Supplementary Table S2).
Absolute abundances (SSU rRNA transcripts g−1 soil DW) of methanogenic Archaea (A), the relative abundance of SSU rRNA transcripts belonging to methanogenic Archaea normalized to the total amount of SSU rRNA transcripts belonging to methanogenic Archaea (B), and transcript abundances (mRNA transcripts g−1 soil DW) of mRNA of methanogenesis pathways (C) in soils from the upper (0–10 cm) and the deeper soil layer (20–30 cm) of the grassland sites with low (LI, yellow) and high (HI, turquoise) land-use intensity taken in autumn (aut) 2017 and winter (win), spring (spr) and summer (sum) 2018. In A, B, and C, columns show means per season and depth of the upper (0–10 cm) and the deeper soil layer (20–30 cm) in LI and HI. “unclassified methanogens” contain methanogens unclassified at the class level and low abundance methanogenic groups. Bars represent the means of three replicates. In C, error bars represent the means and the standard deviations of three replicates. Linear correlation of absolute abundances of methanogenesis mRNA transcripts with CH4 fluxes (D). In D, points represent seasonal means across both depths; samples from autumn, winter, spring, and summer are depicted as circles, diamonds, upward-pointing triangles, and downward-pointing triangles, respectively. The “r” denotes the Pearson correlation coefficient. Significance codes: *p < 0.05, **p < 0.01, ns not significant. DW dry weight. We refer to Supplementary Fig. 5 and 6 showing the absolute and relative abundances of methanogen SSU rRNA in the individual samples, respectively.
Methanogenesis mRNA transcripts were generally less abundant in spring and summer (0.21 and 0.43 * 107 transcripts g−1 in summer in LI and HI, respectively) than in autumn and winter (5.6 and 3.6 * 107 transcripts g−1in winter in LI and HI, respectively) (Fig. 4C). According to Tukey’s HSD test, methanogenesis transcript abundances were significantly lower (p < 0.05) in spring and summer compared to autumn and winter, in both LI and HI (Supplementary Tables S7 and 8). This drop in methanogenesis mRNA agrees with the cessation of CH4 emissions from the soils in spring in summer; both correlated significantly with each other (r = 0.87, p < 0.01, Fig. 4D). In contrast, the abundances of methanogen SSU rRNA transcripts and CH4 fluxes did not correlate significantly (Supplementary Fig. 7). Our results indicate that methanogenesis mRNA transcripts are better indicators of net CH4 fluxes than methanogen SSU rRNA transcripts (Fig. 4D, Supplementary Fig. 7). We thus underscore studies that have found mRNA more responsive to environmental factors than rRNA [41, 42].
We only sampled two sites and cannot make statistically assured statements about the influence of land-use intensity. Nevertheless, we observed some site-specific patterns. Methanogen SSU rRNA transcript abundances were higher in HI than in LI soils (Fig. 4A) despite similar methanogenesis mRNA transcript abundances (Fig. 4C). The taxonomic composition may influence the transcriptional activity of methanogenesis transcripts (Fig. 4B). The strictly acetoclastic Methanosaetaceae (Methanothrix) were more pronounced in HI than in LI (Fig. 4B). Methanosaeta have lower growth rates and can grow at lower acetate concentrations than the metabolically diverse Methanosarcina [79]. In turn, the share of hydrogenotrophic methanogens, such as Methanocellaceae, Methanoregulaceae, and Methanobacteriaceae, was higher in LI than in HI. The energy yield of hydrogenotrophic methanogenesis is larger than that of acetoclastic methanogenesis [9, 80]. The varying proportions of acetoclastic and hydrogenotrophic methanogens and lower acetate concentrations may explain lower transcriptional activity at HI compared to LI. Messenger RNA transcripts that were unambiguously attributed to a certain methanogenesis pathway, support that the share of acetoclastic mRNAs was lower in LI than in HI (Supplementary Fig. 8). However, large-scale studies, that include more sites would be needed to explore this effect further.
The consistent presence throughout the year of the obligate methylotrophic Methanomassiliicoccales (up to 14% of the methanogen SSU rRNA in the topsoils, Fig. 4B) points to methylated compounds as additional substrates for methanogenesis in both sites. The contribution of methanogenesis from methylated compounds to terrestrial CH4 emissions is considered to be small [9]. However, recent research suggests it to be more important [10, 13, 81, 82]. For instance, the methylotrophic Methanomassiliicoccales were the second most abundant methanogenic group in Zoige peatlands [83] and also highly abundant in wetlands in northeast Germany [84].
Furthermore, we wanted to know if methanogens exhibited a differential gene expression across seasons. For this purpose, we assessed broad functional categories of mRNA transcripts taxonomically binned to Euryarchaeota. Methanogen transcript profiles had similar seasonal patterns in both soils. For instance, protein biosynthesis and transcription were upregulated in methanogens during winter (Supplementary Fig. 9). The upregulation of the protein biosynthesis machinery in soil microbiomes was recently attributed to diminished enzymatic reaction rates of metabolic enzymes at colder temperatures [85]. Likewise, our results point to a temperature-dependent regulation of central cellular processes in the here studied methanogens.
High spatio-temporal dynamics of methanotrophs
The aerobic methanotrophs in the soils, assessed by SSU rRNAs, mostly belonged to canonical MOBs, i.e., Methylococcaceae, Crenotrichaceae, Methylocystaceae (Fig. 5A, B). They were generally higher abundant in topsoils, as compared to subsoils, except of summer (Fig. 5A). Anaerobic methanotrophic bacteria (Ca. Methylomirabilis) and Archaea (ANME-2d) comprised a substantial part of the methanotroph community (up to 20% of all methanotrophs in subsoil) (Fig. 5B). They were present mainly in the deeper soil layer (20–30 cm), which was likely due to their sensitivity to oxygen [86]. Across seasons, methanotroph abundance (aerobic and anaerobic) was highest in autumn and winter (Fig. 5A), resembling seasonal dynamics of methanogens.
Absolute abundances (SSU rRNA transcripts g−1 soil DW) of methanotrophic microorganisms (Archaea and Bacteria) (A), proportion of SSU rRNA transcripts belonging to methanotrophic microorganisms normalized to the total amount of SSU rRNA transcripts belonging to methanogenic Archaea and methanotrophs (B), and the proportion of pmoA groups normalized to the total amount of pmoA transcripts (C). Columns show means per seasons and depth in soils from the upper (0–10 cm) and the deeper soil layer (20–30 cm) of the grassland sites with low (LI) and high (HI) land-use intensity taken in autumn (aut) 2017 and winter (win), spring (spr) and summer (sum) 2018. “unclassified Methylococcales” contain Methylococcales unclassified at the family level and low abundance Methylococcales families. “pmoA like” = unclassified pmoA-like sequences. Bars represent the means of three replicates. Abbreviations: DW dry weight. We refer to Supplementary Figs. 10–12 showing the absolute and relative abundances of methanotroph SSU rRNA and the pmoA composition in the individual samples, respectively.
In addition to SSU rRNA, we assessed the active MOBs using transcripts of the most widespread functional marker, the alpha subunit of the pMMO [64]. While the same clades were detected, their relative abundance was sometimes different to the SSU rRNA derived MOB profiles (Fig. 5C). For instance, Methylococcales SSU rRNA transcripts (type I) clearly dominated in LI (up to 96% of all methanotroph SSU rRNA) but comprised less than 50% of pmoA transcripts (Fig. 5C). Generally, type II methanotrophs were more abundant in the pmoA than in the SSU rRNA transcripts. Studies assessing SSU rRNA composition might generally underrepresent this group. Especially in autumn and winter, the pmoA transcripts were dominated by canonical MOBs that probably feed on the CH4 produced by the methanogens. Remarkably, the proportions of pmoA transcripts classified as USCα, USCγ, and pmoA2 increased in spring and summer in both sites (Fig. 5C). These pmoAs are assumed to be associated with atmospheric MOBs [15, 28, 87, 88]. Their increase matched the net CH4 uptake of the soils in spring and summer (Fig. 1A). The relative abundance of USCα and γ pmoA and pmoA2 transcripts was up to 34%. Still, other type I and type II pmoA sequences dominated the soils. Recently, atmospheric CH4 oxidation in paddy soils was attributed to canonical CH4 oxidizers rather than USCα and USCγ [30]. Thus, also the detected type I and type II methanotrophs might be involved in atmospheric CH4 oxidation in spring and summer. However, it is also possible that CH4 is still produced in deeper soil layers and that the canonical CH4 oxidizers feed on this CH4. To complicate matters even more, the, yet only isolate of USCα methanotrophs, Methylocapsa gorgona, can grow at both atmospheric and elevated CH4 concentrations [89].
Similar as with methanogens, we wanted to explore differences in expression of general functions of methanotrophs across seasons. Transcripts taxonomically binned to gamma and alphaproteobacterial methanotrophs showed an upregulation of protein synthesis and processing as well as transcription and RNA processing in autumn and winter (Supplementary Fig. 13). This is strikingly similar to the gene expression in methanogens, providing further support that protein biosynthesis apparatus might be larger at lower temperatures [85]. In some samples only a few mRNAs could be functionally assigned. This must be considered when interpreting these results.
Functional transcript abundances as a proxy for soil net surface CH4 fluxes
We have shown above that the abundance of methanogenesis-related mRNAs was a good estimator of CH4 fluxes in the studied soils (Fig. 4D). We now aimed to integrate methanotroph and methanogen markers to assess if a comprehensive understanding of soil CH4 fluxes can be derived from quantitative metatranscriptomics.
The pMMO mRNA transcripts of both sites correlated positively with methanogenesis transcripts (r = 0.62, p < 0.001) but there was no significant correlation in SSU rRNA transcripts across sites (Supplementary Fig. 14). The correlation of mRNA transcripts suggests that methanotrophs predominantly use CH4 derived from methanogenesis in the soil. They thus act as a filter mitigating CH4 emission to the atmosphere [31]. However, there is seasonal variation; the pMMO to methanogenesis mRNA ratio was higher in spring and summer than in autumn and winter (4.8 and 3.6 in winter, and 30.0 and 12.1 in summer, in LI and HI, respectively) (Fig. 6B). Such a ratio may thus indicate whether soils are CH4 sources or sinks. A high methanotroph to methanogen ratio may hint at a soil being a CH4 sink, while a low ratio may hint at a soil being a net CH4 source. Yet, it is necessary to consider transcriptional activity since the ratio of methanotroph to methanogen SSU rRNA was not indicative of soils’ CH4 fluxes (Fig. 6A). Furthermore, the MOB community composition could be an additional indicator for soil CH4 uptake since a high proportion of atmospheric CH4 oxidizers in the pmoA transcripts was linked to net CH4 uptake of the soils.
The ratio of methanotroph to methanogen SSU rRNA transcripts (A) and pMMO to methanogenesis mRNA transcripts (B). The ratio was calculated with mean transcript abundances of the upper (0–10 cm) and the deeper soil layer (20–30 cm) of one soil sample. of the grassland sites with low (LI, yellow) and high (HI, turquoise) land-use intensity taken in autumn (aut) 2017 and winter (win), spring (spr) and summer (sum) 2018. Statistically significant categories of the ratios between seasons were tested with an ANOVA and subsequent post-hoc Tukey’s test at p-adjusted < 0.05 level.
Conclusions
This study is, to our knowledge, the first that uses quantitative metatranscriptomics to link CH4 fluxes from grasslands with CH4-cycling microbiomes through all seasons of the year. We validated mRNA transcripts rather than SSU rRNA transcripts to be necessary for linking microbial activity to soil net surface CH4 fluxes in the two studied soils measured on a daily time scale. If this holds for annual rates based on temporarily highly resolved real-time data, requires more research. Still, since the abundance of mRNA of methanogenesis pathways correlated well with the net CH4 fluxes, it may thus be feasible to estimate soil CH4 fluxes using mcr transcript abundances when additionally considering the transcript ratio of methanotroph and methanogen key enzymes. The latter is suggested by the different ratios between the seasons in both grasslands.
Soils are the largest biological sink for atmospheric CH4, an important ecosystem function given the increasing concentration of atmospheric CH4 [1]. However, its magnitude and controlling factors are currently poorly constrained [3, 27]. Our study adds to the growing body of literature (e.g., [30]) that suggests that in soils with internal CH4 formation, such as the drained peatlands investigated here, many methanotroph groups contribute to atmospheric CH4 oxidation as compared to upland soils that are permanent net sinks of CH4. Stable isotope probing may be well suited to investigate this further [21,22,23,24,25].
We investigated 60 samples by RNAseq, a technique currently still restricted in terms of throughput and costs. Two RT qPCR studies found a relationship between mcrA transcript abundances and CH4 fluxes in a paddy soil and a peat bog, respectively [90, 91]. Parallel RT qPCRs of mcrA and pmoA transcripts might thus currently be also viable tools to estimate CH4 fluxes of soils from many samples and sites, respectively. Nevertheless, more large-scale studies, such as the one presented here, are encouraged to further investigate the link between methanogens and methanotrophs and CH4 fluxes across different soil types and seasons, especially when considering the ever decreasing costs of sequencing and further automatization in bioinformatics workflows.
Data availability
All raw sequencing data have been deposited in NCBI sequence read archive under BioProject ID PRJNA741868.
References
Canadell JG, Monteiro PMS, Costa, MH, Cotrim da Cunha L, Cox PM, Eliseev AV, et al. Global carbon and other biogeochemical cycles and feedbacks. In: Masson-Delmotte V, Zhai P, Pirani A, Connors SL, Péan C, Berger S, et al. editors. Climate Change 2021: The Physical Science Basis. Contribution of Working Group I to the Sixth Assessment Report of the Intergovernmental Panel on Climate Change. Cambridge University Press; 2021, in press.
Rosentreter JA, Borges AV, Deemer BR, Holgerson MA, Liu S, Song C, et al. Half of global methane emissions come from highly variable aquatic ecosystem sources. Nat Geosci. 2021;14:225–30.
Saunois M, Stavert AR, Poulter B, Bousquet P, Canadell JG, Jackson RB, et al. The global methane budget 2000 – 2017. Earth Syst. Sci Data. 2020;12:1561–623.
Lamentowicz M, Gałka M, Pawlyta J, Lamentowicz Ł, Goslar T, Miotk-Szpiganowicz G. Climate change and human impact in the southern Baltic during the last millennium reconstructed from an ombrotrophic bog archive. Stud Quat. 2011;28:3–16.
Davidson NC. How much wetland has the world lost? Long-term and recent trends in global wetland area. Mar Freshw Res. 2014;65:934–41.
Oertel C, Matschullat J, Zurba K, Zimmermann F, Erasmi S. Greenhouse gas emissions from soils - a review. Geochemistry. 2016;76:327–52.
Liesack W, Schnell S, Revsbech NP. Microbiology of flooded rice paddies. FEMS Microbiol Rev. 2000;24:625–45.
Conrad R. The global methane cycle: recent advances in understanding the microbial processes involved. Environ Microbiol Rep. 2009;1:285–92.
Lyu Z, Shao N, Akinyemi T, Whitman WB. Methanogenesis. Curr Biol. 2018;28:R727–R732.
Kurth JM, Nobu MK, Tamaki H, de Jonge N, Berger S, Jetten MSM, et al. Methanogenic archaea use a bacteria-like methyltransferase system to demethoxylate aromatic compounds. ISME J. 2021;15:3549–65.
Mayumi D, Mochimaru H, Tamaki H, Yamamoto K, Yoshioka H, Suzuki Y, et al. Methane production from coal by a single methanogen. Science. 2016;354:222–6.
Bridgham SD, Cadillo-Quiroz H, Keller JK, Zhuang Q. Methane emissions from wetlands: Biogeochemical, microbial, and modeling perspectives from local to global scales. Glob Chang Biol. 2013;19:1325–46.
Narrowe AB, Borton MA, Hoyt DW, Smith GJ, Daly RA, Angle JC, et al. Uncovering the diversity and activity of methylotrophic methanogens in freshwater wetland soils. mSystems. 2019;4:e00320–19.
Zalman CA, Meade N, Chanton J, Kostka JE, Bridgham SD, Keller JK. Methylotrophic methanogenesis in Sphagnum-dominated peatland soils. Soil Biol Biochem. 2018;118:156–60.
Knief C. Diversity and habitat preferences of cultivated and uncultivated aerobic methanotrophic bacteria evaluated based on pmoA as molecular marker. Front Microbiol. 2015;6:1346.
Le Mer J, Roger P. Production, oxidation, emission and consumption of methane by soils: a review. Eur J Soil Biol. 2001;37:25–50.
Wieczorek AS, Drake HL, Kolb S. Organic acids and ethanol inhibit the oxidation of methane by mire methanotrophs. FEMS Microbiol Ecol. 2011;77:28–39.
Welte CU, Rasigraf O, Vaksmaa A, Versantvoort W, Arshad A, Op den Camp HJM, et al. Nitrate- and nitrite-dependent anaerobic oxidation of methane. Environ Microbiol Rep. 2016;8:941–55.
Cui M, Ma A, Qi H, Zhuang X, Zhuang G. Anaerobic oxidation of methane: An ‘active’ microbial process. Microbiol Open. 2015;4:1–11.
Ettwig KF, Zhu B, Speth D, Keltjens JT, Jetten MSM, Kartal B. Archaea catalyze iron-dependent anaerobic oxidation of methane. Proc Natl Acad Sci USA. 2016;113:12792–6.
Stiehl-Braun PA, Hartmann AA, Kandeler E, Buchmann N, Niklaus PA. Interactive effects of drought and N fertilization on the spatial distribution of methane assimilation in grassland soils. Glob Chang Biol 2011;17:2629–39.
Bodelier PLE, Meima-Franke M, Hordijk CA, Steenbergh AK, Hefting MM, Bodrossy L, et al. Microbial minorities modulate methane consumption through niche partitioning. ISME J. 2013;7:2214–28.
Karbin S, Hagedorn F, Dawes MA, Niklaus PA. Treeline soil warming does not affect soil methane fluxes and the spatial micro-distribution of methanotrophic bacteria. Soil Biol Biochem. 2015;86:164–71.
Stiehl-Braun PA, Powlson DS, Poulton PR, Niklaus PA. Effects of N fertilizers and liming on the micro-scale distribution of soil methane assimilation in the long-term Park Grass experiment at Rothamsted. Soil Biol Biochem. 2011;43:1034–41.
Menyailo OV, Hungate BA, Abraham WR, Conrad R. Changing land use reduces soil CH4 uptake by altering biomass and activity but not composition of high-affinity methanotrophs. Glob Chang Biol. 2008;14:2405–19.
Ciais P, Sabine C, Bala G, Bopp L, Brovkin V, Canadell J, et al. Carbon and Other Biogeochemical Cycles. In: Stocker TF, Qin D, Plattner G-K, Tignor M, Allen SK, Boschung J, et al. editors. Climate Change 2013 the Physical Science Basis: Working Group I Contribution to the Fifth Assessment Report of the Intergovernmental Panel on Climate Change. New York, NY: Cambridge University Press; 2013, 465–570.
Täumer J, Kolb S, Boeddinghaus RS, Wang H, Schöning I, Schrumpf M, et al. Divergent drivers of the microbial methane sink in temperate forest and grassland soils. Glob Chang Biol. 2021;27:929–40.
Kolb S. The quest for atmospheric methane oxidizers in forest soils. Environ Microbiol Rep. 2009;1:336–46.
Kolb S, Horn MA. Microbial CH4 and N2O consumption in acidic wetlands. Front Microbiol. 2012;3:78.
Cai Y, Zheng Y, Bodelier PLE, Conrad R, Jia Z. Conventional methanotrophs are responsible for atmospheric methane oxidation in paddy soils. Nat Commun. 2016;7:11728.
Dean JF, Middelburg JJ, Röckmann T, Aerts R, Blauw LG, Egger M, et al. Methane feedbacks to the global climate system in a warmer world. Rev Geophys. 2018;56:207–50.
Levy-Booth DJ, Giesbrecht IJW, Kellogg CTE, Heger TJ, D’Amore DV, Keeling PJ, et al. Seasonal and ecohydrological regulation of active microbial populations involved in DOC, CO2, and CH4 fluxes in temperate rainforest soil. ISME J. 2019;13:950–63.
Lombard N, Prestat E, van Elsas JD, Simonet P. Soil-specific limitations for access and analysis of soil microbial communities by metagenomics. FEMS Microbiol Ecol. 2011;78:31–49.
Carini P, Marsden PJ, Leff JW, Morgan EE, Strickland MS, Fierer N. Relic DNA is abundant in soil and obscures estimates of soil microbial diversity. Nat Microbiol. 2016;2:16242.
Blazewicz SJ, Barnard RL, Daly RA, Firestone MK. Evaluating rRNA as an indicator of microbial activity in environmental communities: limitations and uses. ISME J. 2013;7:2061–8.
Sukenik A, Kaplan-Levy RN, Welch JM, Post AF. Massive multiplication of genome and ribosomes in dormant cells (akinetes) of Aphanizomenon ovalisporum (Cyanobacteria). ISME J. 2012;6:670–9.
Schwartz E, Hayer M, Hungate BA, Koch BJ, McHugh TA, Mercurio W, et al. Stable isotope probing with 18O-water to investigate microbial growth and death in environmental samples. Curr Opin Biotechnol. 2016;41:14–18.
Angel R, Conrad R. Elucidating the microbial resuscitation cascade in biological soil crusts following a simulated rain event. Environ Microbiol. 2013;15:2799–815.
Papp K, Mau RL, Hayer M, Koch BJ, Hungate BA, Schwartz E. Quantitative stable isotope probing with H218O reveals that most bacterial taxa in soil synthesize new ribosomal RNA. ISME J. 2018;12:3043–5.
Urich T, Lanzén A, Qi J, Huson DH, Schleper C, Schuster SC. Simultaneous assessment of soil microbial community structure and function through analysis of the meta-transcriptome. PLoS One. 2008;3:e2527.
Peng J, Wegner CE, Liesack W. Short-term exposure of paddy soil microbial communities to salt stress triggers different transcriptional responses of key taxonomic groups. Front Microbiol. 2017;8:400.
Peng J, Wegner CE, Bei Q, Liu P, Liesack W. Metatranscriptomics reveals a differential temperature effect on the structural and functional organization of the anaerobic food web in rice field soil. Microbiome. 2018;6:169.
Abdallah RZ, Wegner CE, Liesack W. Community transcriptomics reveals drainage effects on paddy soil microbiome across all three domains of life. Soil Biol Biochem. 2019;132:131–42.
Gloor GB, Macklaim JM, Pawlowsky-Glahn V, Egozcue JJ. Microbiome datasets are compositional: and this is not optional. Front Microbiol. 2017;8:2224.
Moran MA, Satinsky B, Gifford SM, Luo H, Rivers A, Chan LK, et al. Sizing up metatranscriptomics. ISME J. 2013;7:237–43.
Gifford SM, Sharma S, Rinta-Kanto JM, Moran MA. Quantitative analysis of a deeply sequenced marine microbial metatranscriptome. ISME J. 2011;5:461–72.
Söllinger A, Tveit AT, Poulsen M, Noel SJ, Bengtsson M, Bernhardt J, et al. Holistic assessment of rumen microbiome dynamics through quantitative metatranscriptomics reveals multifunctional redundancy during key steps of anaerobic feed degradation. mSystems 2018;3:e00038–18.
Fischer M, Bossdorf O, Gockel S, Hänsel F, Hemp A, Hessenmöller D, et al. Implementing large-scale and long-term functional biodiversity research: the biodiversity exploratories. Basic Appl Ecol. 2010;11:473–85.
IUSS Working Group WRB. World reference base for soil resources 2014, update 2015 international soil classification system for naming soils and creating legends for soil maps. World Soil Resour Reports No 106. Rome: FAO; 2015.
Vance ED, Brookes PC, Jenkinson DS. An extraction method for measuring soil microbial biomass C. Soil Biol Biochem. 1987;19:703–7.
Joergensen RG, Mueller T. The fumigation-extraction method to estimate soil microbial biomass: calibaration of the kEN value. Soil Biol Biochem. 1996;28:33–37.
Brookes PC, Landman A, Pruden G, Jenkinson DS. Chloroform fumigation and the release of soil nitrogen: a rapid direct extraction method to measure microbial biomass nitrogen in soil. Soil Biol Biochem. 1985;17:837–42.
Bamminger C, Zaiser N, Zinsser P, Lamers M, Kammann C, Marhan S. Effects of biochar, earthworms, and litter addition on soil microbial activity and abundance in a temperate agricultural soil. Biol Fertil Soils. 2014;50:1189–1200.
Koch O, Tscherko D, Kandeler E. Seasonal and diurnal net methane emissions from organic soils of the Eastern Alps, Austria: Effects of soil temperature, water balance, and plant biomass. Arct Antarct Alp Res. 2007;39:438–48.
Tveit AT, Urich T, Svenning MM. Metatranscriptomic analysis of arctic peat soil microbiota. Appl Environ Microbiol. 2014;80:5761–72.
Magoč T, Salzberg SL. FLASH: fast length adjustment of short reads to improve genome assemblies. Bioinformatics. 2011;27:2957–63.
Schmieder R, Edwards R. Quality control and preprocessing of metagenomic datasets. Bioinformatics. 2011;27:863–4.
Kopylova E, Noé L, Touzet H. SortMeRNA: Fast and accurate filtering of ribosomal RNAs in metatranscriptomic data. Bioinformatics. 2012;28:3211–7.
Edgar RC. Search and clustering orders of magnitude faster than BLAST. Bioinformatics. 2010;26:2460–1.
Lanzén A, Jørgensen SL, Huson DH, Gorfer M, Grindhaug SH, Jonassen I, et al. CREST - Classification resources for environmental sequence tags. PLoS One. 2012;7:e49334.
Altschul SF, Gish W, Miller W, Myers EW, Lipman DJ. Basic local alignment search tool. J Mol Biol. 1990;215:403–10.
Huson DH, Beier S, Flade I, Górska A, El-Hadidi M, Mitra S, et al. MEGAN community edition - interactive exploration and analysis of large-scale microbiome sequencing data. PLoS Comput Biol. 2016;12:e1004957.
Buchfink B, Xie C, Huson DH. Fast and sensitive protein alignment using DIAMOND. Nat Methods. 2014;12:59–60.
Dumont MG, Lüke C, Deng Y, Frenzel P. Classification of pmoA amplicon pyrosequences using BLAST and the lowest common ancestor method in MEGAN. Front Microbiol. 2014;5:34.
R Core Team. R: a language and environment for statistical computing. Vienna, Austria: R Foundation for Statistical Computing; 2018.
Oksanen J, Blanchet f. G, Friendly M, Kindt R, Legendre P, McGlinn D, et al. vegan: Community ecology package. 2020. R package version 2.5-7. https://CRAN.R-project.org/package=vegan.
Graves S, Piepho H-P, Selzer L. multcompView: Visualizations of paired comparisons. 2019. R package version 0.1-8. https://CRAN.R-project.org/package=multcompView.
Günther A, Barthelmes A, Huth V, Joosten H, Jurasinski G, Koebsch F, et al. Prompt rewetting of drained peatlands reduces climate warming despite methane emissions. Nat Commun. 2020;11:1644.
IPCC Task Force on National Greenhouse Gas Inventories. Methodological guidance on lands with wet and drained soilds, and constructed wetlands for wastewater treatment. 2013 Supplement to the 2006 IPCC Guidelines for National Greenhouse Gas Inventories: Wetlands. 2014.
Tiemeyer B, Albiac Borraz E, Augustin J, Bechtold M, Beetz S, Beyer C, et al. High emissions of greenhouse gases from grasslands on peat and other organic soils. Glob Chang Biol. 2016;22:4134–49.
Kirschbaum MUF. The temperature dependence of soil organic matter decomposition, and the effect of global warming on soil organic C storage. Soil Biol Biochem. 1995;27:753–60.
Knorr W, Prentice IC, House JI, Holland EA. Long-term sensitivity of soil carbon turnover to warming. Nature 2005;433:298–301.
Dutaur L, Verchot LV. A global inventory of the soil CH4 sink. Glob Biogeochem Cycles. 2007;21:GB4013.
McDaniel MD, Saha D, Dumont MG, Hernández M, Adams MA. The effect of land-use change on soil CH4 and N2O fluxes: A global meta-analysis. Ecosystems. 2019;22:1424–43.
Gulledge J, Schimel JP. Moisture control over atmospheric CH4 consumption and CO2 production in diverse Alaskan soils. Soil Biol Biochem. 1998;30:1127–32.
Tveit AT, Urich T, Frenzel P, Svenning MM. Metabolic and trophic interactions modulate methane production by Arctic peat microbiota in response to warming. Proc Natl Acad Sci USA. 2015;112:E2507–E2516.
Conrad R. Methane production in soil environments - anaerobic biogeochemistry and microbial life between flooding and desiccation. Microorganisms 2020;8:881.
Lyu Z, Lu Y. Metabolic shift at the class level sheds light on adaptation of methanogens to oxidative environments. ISME J. 2018;12:411–23.
Smith KS, Ingram-Smith C. Methanosaeta, the forgotten methanogen? Trends Microbiol. 2007;15:150–5.
Whitman WB, Bowen TL, Boone DR. The methanogenic bacteria. In: Rosenberg E, DeLong EF, Lory S, Stackebrandt E, Thompson F editors. The prokaryotes: other major lineages of bacteria and the archaea. Berlin, Heidelberg: Springer; 2014, pp 123–63.
Conrad R. Importance of hydrogenotrophic, aceticlastic and methylotrophic methanogenesis for methane production in terrestrial, aquatic and other anoxic environments: a mini review. Pedosphere. 2020;30:25–39.
Söllinger A, Urich T. Methylotrophic methanogens everywhere - physiology and ecology of novel players in global methane cycling. Biochem Soc Trans. 2019;47:1895–907.
Yang S, Liebner S, Winkel M, Alawi M, Horn F, Dörfer C, et al. In-depth analysis of core methanogenic communities from high elevation permafrost-affected wetlands. Soil Biol Biochem. 2017;111:66–77.
Weil M, Wang H, Bengtsson M, Köhn D, Günther A, Jurasinski G, et al. Long-term rewetting of three formerly drained peatlands drives congruent compositional changes in pro- and eukaryotic soil microbiomes through environmental filtering. Microorganisms. 2020;8:550.
Söllinger A, Seneca J, Dahl MB, Motleleng LL, Prommer J, Verbruggen E, et al. Down-regulation of the microbial protein biosynthesis machinery in response to weeks, years, and decades of soil warming. Sci Adv. 2022;8:eabm3230.
Luesken FA, Wu ML, Op den Camp HJM, Keltjens JT, Stunnenberg H, Francoijs KJ, et al. Effect of oxygen on the anaerobic methanotroph ‘Candidatus Methylomirabilis oxyfera’: Kinetic and transcriptional analysis. Environ Microbiol. 2012;14:1024–34.
Baani M, Liesack W. Two isozymes of particulate methane monooxygenase with different methane oxidation kinetics are found in Methylocystis sp. strain SC2. Proc Natl Acad Sci. 2008;105:10203–8.
Yimga MT, Dunfield PF, Ricke P, Heyer J, Liesack W. Wide distribution of a novel pmoA-like gene copy among type II methanotrophs, and its expression in Methylocystis strain SC2. Appl Environ Microbiol. 2003;69:5593–602.
Tveit AT, Hestnes AG, Robinson SL, Schintlmeister A, Dedysh SN, Jehmlich N, et al. Widespread soil bacterium that oxidizes atmospheric methane. Proc Natl Acad Sci USA. 2019;116:8515–24.
Freitag TE, Toet S, Ineson P, Prosser JI. Links between methane flux and transcriptional activities of methanogens and methane oxidizers in a blanket peat bog. FEMS Microbiol Ecol. 2010;73:157–65.
Qin H, Tang Y, Shen J, Wang C, Chen C, Yang J, et al. Abundance of transcripts of functional gene reflects the inverse relationship between CH4 and N2O emissions during mid-season drainage in acidic paddy soil. Biol Fertil Soils. 2018;54:885–95.
Acknowledgements
We thank the managers of the three Exploratories, Miriam Teuscher, Juliane Vogt, Kirsten Reichel-Jung, Iris Steitz, Sandra Weithmann and all former managers for their work in maintaining the plot and project infrastructure; Christiane Fischer and Victoria Grießmeier for giving support through the central office, Andreas Ostrowski for managing the central data base, and Markus Fischer, Eduard Linsenmair, Dominik Hessenmöller, Daniel Prati, Ingo Schöning, François Buscot, Ernst-Detlef Schulze, Wolfgang W. Weisser and the late Elisabeth Kalko for their role in setting up the Biodiversity Exploratories project. We thank the administration of the Hainich national park, the UNESCO Biosphere Reserve Swabian Alb and the UNESCO Biosphere Reserve Schorfheide-Chorin as well as all land owners for the excellent collaboration. The work has been funded by the DFG Priority Program 1374 “Biodiversity-Exploratories” (DFG- KO2912/12-1, MA4436/2-1, UR198/3-1, UR198/5-1). Field work permits were issued by the responsible state environmental offices of Baden-Württemberg, Thüringen, and Brandenburg. We thank Markus Fischer and the botany core team for providing vegetation record data. We thank Lars Jensen and Lisa Hagenau for their support during library preparation and sequencing. We thank Juliette Blum and Fabian Stache (University Hohenheim) for performing microbial biomass analyses. We also are grateful for the support by ZALF technicians, especially Sigune Weinert and Paul Reim. We thank Uta Schumacher and Markus Rubenbauer for helping to coordinate the field work. And we thank Andrea Söllinger, Tilman Schmider, Sebastian Petters, Klary Rychly, Vjera Kovacevic, Micha Weil, Simon Weddell, Karolin Müller, Felix Müller and Eva Aderjan for help and support during field sampling. We also thank Mathilde Borg Dahl, Tjorven Hinzke, Caterina Penone, and Haitao Wang for advice with statistical analysis.
Funding
Open Access funding enabled and organized by Projekt DEAL.
Author information
Authors and Affiliations
Contributions
The study was designed by TU, SK, and SM. Sampling was performed and coordinated by JT assisted by TU, SM, SK. Gas flux and Cmic, Nmic analysis were performed by SM. RNA extractions and amplifications were performed by JT and VG. Sequencing and library preparation was performed by CJ and AK assisted by JT, VG, and TU. Data analysis was performed by JT, VG, TU, SM, and SK. The manuscript was written by JT and TU, assisted by all co-authors.
Corresponding author
Ethics declarations
Competing interests
The authors declare no competing interests.
Additional information
Publisher’s note Springer Nature remains neutral with regard to jurisdictional claims in published maps and institutional affiliations.
Supplementary information
Rights and permissions
Open Access This article is licensed under a Creative Commons Attribution 4.0 International License, which permits use, sharing, adaptation, distribution and reproduction in any medium or format, as long as you give appropriate credit to the original author(s) and the source, provide a link to the Creative Commons license, and indicate if changes were made. The images or other third party material in this article are included in the article’s Creative Commons license, unless indicated otherwise in a credit line to the material. If material is not included in the article’s Creative Commons license and your intended use is not permitted by statutory regulation or exceeds the permitted use, you will need to obtain permission directly from the copyright holder. To view a copy of this license, visit http://creativecommons.org/licenses/by/4.0/.
About this article
Cite this article
Täumer, J., Marhan, S., Groß, V. et al. Linking transcriptional dynamics of CH4-cycling grassland soil microbiomes to seasonal gas fluxes. ISME J 16, 1788–1797 (2022). https://doi.org/10.1038/s41396-022-01229-4
Received:
Revised:
Accepted:
Published:
Issue Date:
DOI: https://doi.org/10.1038/s41396-022-01229-4
This article is cited by
-
Metabolic profiles outperform the microbiota in assessing the response of vaginal microenvironments to the changed state of HPV infection
npj Biofilms and Microbiomes (2024)
-
Characterization and environmental applications of soil biofilms: a review
Environmental Chemistry Letters (2024)
-
Soil microbial ecology through the lens of metatranscriptomics
Soil Ecology Letters (2024)
-
Asynchronous responses of microbial CAZymes genes and the net CO2 exchange in alpine peatland following 5 years of continuous extreme drought events
ISME Communications (2022)