Abstract
Accumulating evidences have suggested the potential association between Int7G24A (rs334354) polymorphism and cancer risk. However, results from epidemiological studies are controversial. We thus conducted this meta-analysis to clarify the association. Relevant studies were identified on electronic databases according to the inclusion criteria. A total of 13 case-control studies containing 4092 cases and 5909 controls were included in our meta-analysis. Odds ratios (ORs) with 95% confidence intervals (CIs) were applied to assess the association. The results of the overall population had suggested that Int7G24A polymorphism had an increased risk for cancer, reaching significant levels in the 2 genetic models (allele model, OR = 1.25, 95% CI 1.09-1.42, P = 0.001; dominant model, OR = 1.24, 95% CI 1.06-1.46, P < 0.008). Besides, significant association was found among Asian population (allele model, OR = 1.27, 95% CI 1.11-1.45, P < 0.001; dominant model, OR = 1.28, 95% CI 1.11-1.49, P < 0.001), whereas there was non-significant relationship detected among Caucasian population (allele model, OR = 1.08, 95% CI 0.92-1.26, P = 0.352; dominant model, OR = 1.05, 95% CI 0.87-1.26, P = 0.639). The present meta-analysis had suggested that Int7G24A polymorphism of gene TGFBR1 involved in the transforming growth factor beta (TGF-β) signaling pathway had a significantly increased risk for cancer development.
Similar content being viewed by others
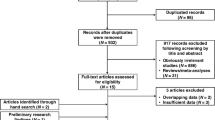
Introduction
Cancer has become the leading cause of death in developed countries and the second leading cause of death in developing countries, according to the data provided by the International Agency for Research on Cancer (IARC)1,2. In the next decades, the burden of cancer will be heavier since the world population is increasing and the problem of ageing is getting worse3. A number of measures have been recommended for the cancer prevention, which have made great progress. However, the etiology of cancer still remains unclear.
In the recent years, interest in the genetic susceptibility to cancers has led to a growing attention to the study of polymorphisms of genes involved in tumourigenesis. TGF-β is one of the most potent inhibitors of proliferation in epithelial, neuronal and hematopoietic cells4,5. Several important biological events are governed by this growth factor, such as cell growth, tissue differentiation, production and degradation of extracellular matrix, morphogenesis and apoptosis4,6. Alterations of TGF-β superfamily signaling have been implicated in various human pathologies, including cancer, developmental disorders, cardiovascular and autoimmune diseases6,7,8. As a key member within the TGF-β signaling way, the gene polymorphism of TGF-β receptor type I (TGFBR1) had been reported to be related with cancer risk9,10,11.
Among the polymorphism variations of gene TGFBR1, Int7G24A (rs334354), representing a G to A transversion in the +24 position of the donor splice site in intron 7, had been firstly found to be possibly related with cancer risk by Chen in 199912. After that, several studies had reported the potential associations between Int7G24A rs334354 genotype and the risk for kidney, breast and lung cancer13,14. However, the relationship remains inconclusive, possibly due to the limited sample size. Thus, meta-analysis was applied for combining the studies with small sample size to draw a more reliable conclusion by calculating a pooled risk estimate. A meta-analysis of 3 studies conducted 9 years ago indicated that Int7G24A might be a tumor susceptibility allele for non-small cell lung cancer (NSCLC), kidney and bladder cancer15. Another meta-analysis performed by Zhang found non-significant association between Int7G24A rs334354 polymorphism with colorectal cancer16. However, there were more rigorous case-control studies on the association between Int7G24A with colorectal cancer conducted these years. Therefore, we performed an updated meta-analysis of all available case–control studies applying 5 genetic models to obtain a more reliable conclusion. Besides, subgroup analysis by ethnicity, genotyping method and cancer type were also conducted for further study. To our knowledge, this is the most comprehensive meta-analysis with the most included studies regarding the Int7G24A rs334354 polymorphisms and cancer risk.
Results
Characteristics of studies
In this meta-analysis, 13 studies12,13,14,17,18,19,20,21,22,23,24,25,26 were identified on the electronic databases (PubMed, Embase, Web of Science and Chinese National Knowledge Infrastructure) according to the inclusion and exclusion criteria. The study identification and selection progression was summarized in Fig. 1. Totally, 13 studies containing 4092 cases and 5909 controls were included in our meta-analysis and their main characteristics were shown in Table 1. These studies included 4 colorectal studies, 2 breast cancer studies, 1 acute lymphocytic leukemia studies (including T-lineage and B-lineage), 1 cervix cancer study, 1 non-small cell lung cancer study (NSCLC), 1 osteosarcoma study, 1 renal cell carcinoma (RCC) and transitional cell carcinoma study of upper urinary tract and bladder (TCC) study, 1 esophageal squamous cell carcinoma (ESCC) study and 1gastric cardia adenocarcinoma (GCA) study. Among the 13 studies, 5 studies were performed in China, 1 in German, 3 in Sweden, 2 in USA, 1 in Netherlands and 1 in Spain. Of these studies, there were 5 studies of Asian, 5 studies of Caucasian and 3 studies of mixed ethnicity (both of them were the mixed population of Caucasian and African-American). As for the control source, 5 studies applied population-based (PB) control, while the other 8 studies performed their studies employing hospital-based (HB) control. In addition, genotyping methods were various between studies, among which 6 studies applied polymerase chain reaction-restriction fragment length polymorphism (PCR-RFLP), 4 studies used polymerase chain reaction-single strand conformation polymerase (PCR-SSCP) and 3 studies employed TaqMan. The genotype distributions in the controls of all studies were in agreement with Hardy–Weinberg equilibrium (HWE) except for 1 study24. The estimated quality of all included studies was in the range of 3-9 scores. The ratings had been reported in Table 1.
Flow chart of the study identification and selection progression.
The terms “N” in the boxes represent the number of corresponding studies. The term “same data” means the studies which reported their results based on the same data. The term “insufficient data” refers to the studies which did not provide enough data for us to calculate the ORs and 95% CIs of the association between Int7G24A polymorphism and cancer risk.
Association between Int7G24A rs334354 polymorphism and cancer risk
In our meta-analysis, the Q test of ORGA/GG and OR AA/GG were Ph < 0.001 and Ph = 0.168. Thus, the random-effect model was applied to calculate the summary ORs. Under logistic regression, the ORGA/GG and OR AA/GG were 1.19 (95% CI 1.03-1.38, P = 0.021) and 1.80 (95% CI 1.30-2.49, P < 0.001). The parameters θ2 and θ3 in the logistic regression equation were 0.08 (95% CI 0.01-0.14) and 0.26 (95% CI 0.11-0.40). In addition, plots of study-specific estimates and 95% CIs of the two parameters, θ2 and θ3, had been shown in Fig. 2. According to our results, the P value of 0.711 for the null hypothesis had suggested there was no difference between ORGA/GG and OR AA/GG. Therefore, the best genetic model was indicated to be dominant model according to the above algorithm. Besides, in order to explore whether A allele could increase the risk for cancer or not, allele model (A vs. G) would also be conducted. Forest plots of meta-analysis on the association between Int7G24A rs334354 polymorphism and cancer risk applying the 2 models were displayed in Figs 3 and 4. The overall effects of Int7G24A rs334354 mutation on the risk for cancer were investigated in 13 studies with 4092 cases and 5909 controls. When the dominant model was applied, significantly increased risk was found with OR of 1.24 (95% CI 1.06-1.46, P = 0.008). As for the allele model, increased risk was determined with OR of 1.25 (95% CI 1.09-1.42), reaching a significant level (P = 0.001). However, heterogeneity were confirmed in both of the two models (Ph < 0.001 for dominant model, Ph = 0.001 for allele model).
Plots of the study-specific estimates and 95% CIs of the two parameters
, A) for θ2; B) for θ3. θ2 is the logarithmic scale of ORGA/GG and θ3 is the logarithmic scale of ORAA/GG derived from logistic regression. The triangles and horizontal lines correspond to the study-specific estimates and 95% CIs.
Forest plots of OR with 95% CI for Int7G24A rs334354 polymorphism and cancer risk applying allele model (A vs. G).
The triangles and horizontal lines correspond to the study-specific ORs and 95% CIs. The gray areas reflect the study-specific weight. The hollow diamonds represent the pooled OR and 95% CI of the overall population. The vertical solid lines show the OR of 1 and the vertical dashed lines indicate the corresponding pooled ORs.
Forest plots of OR with 95% CI for Int7G24A rs334354 polymorphism and cancer risk applying dominant model (GA + AA vs. GG).
The triangles and horizontal lines correspond to the study-specific ORs and 95% CIs. The gray areas reflect the study-specific weight. The hollow diamonds represent the pooled OR and 95% CI of the overall population. The vertical solid lines show the OR of 1 and the vertical dashed lines indicate the corresponding pooled OR.
Subgroup analyses
Results of subgroup analyses had been shown in Figs 5 and 6. To assess the potential effects of specific study characteristics on the association between Int7G24A polymorphism and cancer risk, we pooled the ORs and 95% CIs from the subgroups of ethnicity, control source, genotyping method, type of sample, type of cancer and sample size. When stratified by ethnicity, significant association between Int7G24A rs334354 polymorphism and cancer risk was detected among the Asian population and the mixed population in both of the allele model (Asian population, OR = 1.27, 95% CI 1.11-1.45, P < 0.001; mixed population, OR = 2.02, 95% CI 1.52-2.68, P < 0.001) and dominant model (Asian population, OR = 1.28, 95% CI 1.11-1.49, P < 0.001; mixed population, OR = 2.27, 95% CI 1.64-3.15, P < 0.001), while non-significant relationship was detected for the Caucasian population (allele model, OR = 1.08, 95% CI 0.92-1.26, P = 0.352; dominant model, OR = 1.05, 95% CI 0.87-1.26, P = 0.639). Notably, no heterogeneity was detected in this subgroup analysis. When stratified by control source, Int7G24A rs334354 had been found to have an increased risk for cancer risk for population-based (PB) group (OR = 1.19, 95% CI 1.04-1.35, P = 0.01) and hospital-based (HB) group (OR = 1.32, 95% CI = 1.06-1.63, P = 0.012) in allele model. Heterogeneity was confirmed in HB group with P < 0.001. Applying dominant model, we found significant relationship only in PB group (OR = 1.22, 95% CI 1.07-1.39, P = 0.003) but not in HB group (OR = 1.29, 95% CI 0.99-1.68, P = 0.057), with heterogeneity found in HB group (Ph < 0.001). According to the type of sample for genotyping, significantly increased relationship was found using allele model (blood sample group, OR = 1.13, 95% CI 1.00-1.27, P = 0.031; tissue sample group, OR = 1.62, 95% CI 1.27-2.07, P = 0.150), while only the tissue sample group was detected to increase the risk for cancer (blood sample group, OR = 1.11, 95% CI 0.96-1.29, P = 0.028; tissue sample group, OR = 1.68, 95% CI 1.21-2.34,P = 0.086). Subgroup analysis was conducted among 3 methods for genotyping. In the PCR-RFLP group and PCR- SSCP group, significantly increased associations between Int7G24A rs334354 polymorphism and cancer risk were found in allele model (PCR-RFLP group, OR = 1.19. 95% CI 1.05-1.35, P = 0.005; PCR- SSCP group, OR = 1.81, 95% CI 1.37-2.38, P < 0.001) and dominant model (PCR-RFLP group, OR = 1.19. 95% CI 1.04-1.36, P = 0.011; PCR- SSCP group, OR = 1.88, 95% CI 1.27-2.79, P < 0.002), with no heterogeneity confirmed. In contrast, no relationship was detected in TaqMan group applying allele model (OR = 1.07, 95% CI 0.81-1.41, P = 0.649, Ph = 0.011) and dominant model (OR = 1.03, 95% CI 0.73-1.46, P = 0.865, Ph = 0.006). In this subgroup analysis, the studies on cancer type were further studied. However, no significant association was found between Int7G24A rs334354 and colorectal cancer in the two models (allele model, OR = 1.08, 95% CI 0.88-1.33, P = 0.469; dominant model OR = 1.03, 95% CI 0.78-1.36, P = 0.834). As for the breast cancer group, significant association was only found in allele model (OR = 1.34, 95% CI 1.15-1.56, P = 0.314) but not dominant model (OR = 1.53, 95% CI 0.65-3.59, P = 0.326). Among the group of other type cancer, Int7G24A polymorphism was confirmed to increase the risk for cancer (allele model, OR = 1.25, 95% CI 1.09-1.42, P < 0.001; dominant model, OR = 1.34, 95% CI 1.14-1.57, P < 0.001). Moreover, in order to explore the confounding effect of sample size on the studying association, we also conducted the subgroup analysis according to sample size. As for the group with sample size larger than 1000, significantly increased association was found in both allele model (OR = 1.18, 95% CI 1.06-1.31, P = 0.002) and dominant model (OR = 1.18, 95% CI 1.06-1.32, P = 0.003). In contrast, no association was detected among the group with sample size smaller than 300 (allele model, OR = 1.34, 95% CI 0.75-2.40, P = 0.322; dominant model, OR = 1.35, 95% CI 0.64-2.84, P = 0.431). Among the group with sample size larger than 300 (including 300) and smaller than 1000 (including 1000), increased relationship was only found in allele model (OR = 1.63, 95% CI 1.10-2.42, P = 0.016) but not dominant model (OR = 1.59, 95% CI 0.92-2.77, P = 0.100). Detailed results were shown in Figs 5 and 6.
Meta-analysis of the association between Int7G24A polymorphism and cancer risk applying allele model (A vs. G).
The triangles and horizontal lines correspond to the subgroup-specific ORs and 95% CIs. The vertical solid line shows the OR of 1. Especially, “Other” indicates other kind of cancer in addition to breast cancer and colorectal cancer, including 2 ALL studies (T-lineage and B-lineage), 1 cervix cancer study, 1 NSCLC study, 1 osteosarcoma study, 1 RCC study, 1 TCC study, 1 ESCC study, 1 colon adenoma study and 1 GCA study; “Mixed” means mixed population with Caucasian and African-American.
Meta-analysis of the association between Int7G24A polymorphism and cancer risk applying dominant model.
The triangles and horizontal lines correspond to the subgroup-specific ORs and 95% CIs. The vertical solid line shows the OR of 1. Especially, “Other” indicates other kind of cancer in addition to breast cancer and colorectal cancer, including 2 ALL studies (T-lineage and B-lineage), 1 cervix cancer study, 1 NSCLC study, 1 osteosarcoma study, 1 RCC study, 1 TCC study, 1 ESCC study, 1 colon adenoma study and 1 GCA study; “Mixed” means mixed population with Caucasian and African-American.
Sensitivity analyses
Sensitivity analyses were used to evaluate the sensitivity of this meta-analysis. Firstly, fixed-effect model and quality-effect model were compared with random-effect models and the conclusions remained unchanged in allele model (see Fig. 5, random-effect model, OR = 1.25, 95% CI = 1.09-1.42, P = 0.001; fixed-effect model, OR = 1.20, 95% CI = 1.12-1.29, P < 0.001; quality-effect model, OR = 1.57, 95% CI = 1.06-1.46, P < 0.001) and dominant model (see Fig. 6, random-effect model, OR = 1.24, 95% CI = 1.06-1.46, P = 0.001; fixed-effect model, OR = 1.20, 95%CI = 1.10-1.30, P < 0.001; quality-effect model, OR = 1.19, 95% CI = 1.01-1.41, P < 0.001). Secondly, we applied leave-one-out method by excluding one study in turn to evaluate the stability of the obtained conclusions. The results of the leave-one-out method had been shown in Figs 7 and 8.The statistical significance of the results was not altered when any single study was omitted, confirming the stability of the results. Especially, the genotype distributions of control groups in 1 study24 did not follow HWE, but the corresponding pooled ORs was not significantly altered by the exclusion of this study. Therefore, the results of this meta-analysis were stable and robust.
Publication bias
Publication bias was assessed through the visual inspection of funnel plots and with tests of Begg rank correlation and Egger regression asymmetry. The shape of funnel plots did not reveal any evidence of obvious asymmetry in all comparisons in overall population. In addition, the Egger test (P = 0.464 for allele model, P = 0.287 for dominant model) and Begg test (P = 0.669 for allele model, P = 0.502 for dominant model) did not suggest evidence of publication bias at a significant level of P = 0.05.
Power calculation
Based on the data in HapMap database (http://www.hapmap.org), G allele distributions of Int7G24A variant were 78% for Utah residents with ancestry from Northern and Western Europe (CEU) and 51% for Han Chinese in Beijing (CHB) and A allele distributions were 22% for CEU and 49% for CHB. In our meta-analysis, the minor allele frequency (MAF) of Int7G24A variant was set to be 0.22. Power analyses were performed on the basis of the least effect size suggested in our meta-analysis (dominant model, OR = 1.24) under the assumption for the alpha value of 0.01 and the number of case-control pairs of 4092. When the allele frequency of A allele was set to be 0.49, our meta-analysis had a power of 98.6% to detect an OR of 1.24 for the association between Int7G24A polymorphism and cancer risk. When the allele frequency of A was set to be 0.22, our meta-analysis had a power of 99.7% to detect an OR of 1.24 for the association between Int7G24A polymorphism and cancer risk.
Discussion
Evidence of epidemiology studies, mechanism researches and animal experiments had confirmed the important role for genetic polymorphism in the development and progression of cancer, especially for the genes involved in tumorigenesis27,28. Although a multitude of novel genetic factors that may contribute to the susceptibility of cancer have been identified by the genome-wide association studies (GWAS) and epidemiological studies in the past few years, there is still a great need to further explore other genetic factors which may lead to the susceptibility of cancer but with low-penetrance effect. The transforming growth factor β (TGF-β) signaling pathway is a key player in metazoan biology and its misregulation can result in tumor development29,30. On one side, in normal and premalignant cells, TGF-β enforces homeostasis and suppresses tumor progression directly through cell-autonomous tumor-suppressive effects (cytostasis, differentiation, apoptosis) or indirectly through effects on the stroma (suppression of inflammation and stroma-derived mitogens). On the other side, when cancer cells lose TGF-β tumor-suppressive responses, they can use TGF-β to their advantage to initiate immune evasion, growth factor production, differentiation into an invasive phenotype and metastatic dissemination or to establish and expand metastatic colonies4,6. As an indispensable member of the TGF-β family, several mutations in the gene had been found to be related with cancer risk, including polymorphism of Int7G24A rs33435414,31,32,33. Since the identification of the potential association between Int7G24A (rs334354) polymorphism and cancer risk18, an increasing number of relevant studies had been conducted with results suggesting the important role of Int7G24A rs334354 mutation in cancer development12,13,19. However, the conclusions from these studies were inconsistent and controversial, primarily resulting from the insufficient sample size to give the right answer. In our meta-analysis, after combing all relevant studies, 13 studies including 4092 cases and 5909 controls were studied. Power analysis indicated that we had a power of 98.6% under the allele frequency of 0.49 and 99.7% under the allele frequency of 0.22 to detect an OR of 1.24 for the association between Int7G24A polymorphism and cancer risk, based on the sample size of our analysis. The results of the overall population had indicated that Int7G24A rs334354 polymorphism had an increased risk for cancer development, reaching significant levels at both of the 2 genetic models. With respect to subgroup analysis, the association still remained significant in many groups.
Traditionally, most meta-analyses on gene-disease studies would test multiple genetic models, which did not estimate the magnitude of effect of a molecular association, leading to improper and confused conclusions. Thus, in our meta-analysis, a method to choose the best genetic model for case-control genetic association studies was applied. Several methods34,35,36 have been considered and the method developed by Bagos et al.36 was determined. The method, making use of the binary structure of the data and by treating the genotypes as independent variables in a logistic regression, was a simple and commonly used methodology that performs satisfactorily and flexibly. This methodology was reported to avoid multiple comparisons and directly tested the most probable model of genetic inheritance in meta-analyses of gene–disease association studies. In our analysis, dominant model was indicated to be the best genetic model for clarifying the association between Int7G24A polymorphism and cancer risk. Besides, allele model was conducted to explore the difference between A carriers and G carriers on the risk for cancer. Thus, allele model and dominant model were applied in our meta-analysis.
Heterogeneity between studies is common in the meta-analysis of genetic association studies. In the present meta-analysis, heterogeneity was determined by Q-test and statistically significant heterogeneity was confirmed within allele model (Ph = 0.001) and dominant model (Ph < 0.001). To explore the potential heterogeneity among studies, subgroup analyses were conducted in our meta-analysis. In the subgroup analysis by ethnicity, the heterogeneity was effectively removed, suggesting that the present heterogeneity was partly derived from ethnicity. It is well known that genotype distributions vary between populations and genetic studies on the genotype-disease association are generally performed on specific population. With respect to Int7G24A rs334354, the genotype frequencies also differ between ethnicities (G allele: 78% for CEU, 51% for CHB; A allele: 22% for CEU, 49% for CHB). Thus, conclusions of genetic studies performed in various countries might be different. In our work, significant association was found among Asian population (allele model, OR = 1.27, 95% CI 1.11-1.45, P < 0.001; dominant model, OR = 1.28, 95% CI 1.11-1.49, P < 0.001), whereas there was non-significant relationship was detected in the two genetic models among Caucasian population (allele model, OR = 1.08, 95% CI 0.92-1.26, P = 0.352; dominant model, OR = 1.05, 95% CI 0.87-1.26, P = 0.639). This result had indicated that the carcinogenesis of Int7G24A polymorphism might be effective only among Asian population but not Caucasian population. Interestingly, it was noted that when stratified by control population, heterogeneity appeared only in the hospital-based (HB) group but not the population-based (PB) group. Besides, in HB group (allele model, OR = 1.32, 95% CI = 1.06-1.63, P = 0.012; dominant model, OR = 1.29, 95% CI 0.99-1.68, P = 0.057), there was a great risk to develop cancer compared with PB group (allele model, OR = 1.19, 95% CI 1.04-1.35, P = 0.01; OR = 1.22, 95% CI 1.07-1.39, P = 0.003). Selection bias might be the reason of this result. On one side, since the HB group were hospital patients, they could not validly represent the exposure situation of the overall population. On the other side, mostly, the HB controls were not randomly selected from the whole patient population. Thus, results from the population-based controls were thought to be more reliable.
In addition, analysis by cancer type was conducted for further study. Present meta-analysis included 4 studies of colorectal cancer, 2 studies of breast cancer and 7 studies of other type of cancer. As for colorectal cancer, no significant association with Int7G24A rs334354 polymorphism was found in both allele model and dominant model (allele model, OR = 1.08, 95% CI = 0.88-1.33; dominant model, OR = 1.03, 95% CI = 0.78-1.36), compared with the previous meta-analysis including 3 studies by Zhang in 201216 (heterozygote model, OR = 0.97, 95% CI = 0.67-1.42; homozygote model, OR = 1.68, 95% CI = 1.14-2.47; recessive model, pooled OR = 1.71, 95% CI = 1.17-2.51). In Zhang’s study, colon adenoma cases were misclassified into colorectal cancer group23. Strictly speaking, colon adenoma should be labeled as the precancerous lesion of colorectal cancer more than one kind of it. Thus, heterogeneity will occur when combining colon adenoma cases with colorectal cancer cases. In our meta-analysis, colon adenoma cases were excluded since the outcome of interest is cancer. However, false positive might appear resulting from the lack of relevant studies and the small sample size. More relevant studies were needed to gain a more reliable.
Some limitations should be considered for our meta-analysis. Firstly, potential biases were hardly inevitable in our analysis. Our restriction on searching studies published in indexed journals could bring in biases such as time-lag bias and publication bias, though the publication bias was not found in the present meta-analysis. Besides, non-differential misclassification bias was possible because the included controls were not uniform. These controls were likely to develop cancer in subsequent years though no clinical symptoms was observed at the time of investigation. Moreover, lack of information for the adjustments of major confounders including age, smoking status, drinking status and environmental factors might cause confounding bias. Secondly, the number of included studies for Int7G24A rs334354 polymorphism was limited for further analysis due to shortage of original studies. More larger and well-designed studies were needed to confirm our results. Thirdly, there are only three ethnicity groups (Asian, Caucasian, mixed) included in the present paper. Thus, it was doubtful whether the obtained conclusions were generalizable to other population.
In conclusion, the present meta-analysis of 13 studies including 4092 cases and 5909 controls suggested that Int7G24A rs334354 polymorphism of gene TGFBR1 involved in the TGF-β signaling pathway had a significantly increased risk on the risk for cancer in both of the two genetic models. Additionally, compared with Caucasian population, Asian population with Int7G24A polymorphism had been found to have a greater risk for the development of cancer. However, well-designed studies with larger sample size and more ethnic groups are required to validate the risk identified in the current meta-analysis.
Methods
Search strategy
In this paper, we conducted a literature search on PubMed (Medline), Embase, Web of Science and Chinese National Knowledge Infrastructure (CNKI) from January 1966 through August 2014 for case-control studies examining the association between Int7G24A (rs334354) polymorphism of TGFBR1 and the risk for cancer, applying the search terms “TGFBR1 or transforming growth factor receptor 1 or type 1 TGF-beta receptor”, “polymorphism or mutation or variation or genotype or SNP” and “cancer or tumor or neoplasm or carcinoma”. Besides, reference lists from retrieved articles were also reviewed. Only articles published in the English and Chinese were considered. We conducted our meta-analysis according to the PRISMA checklists and followed the guideline37.
Inclusion criteria
Studies meeting the following criteria were included in the meta-analysis: (1) the study design was case-control (2) the outcome of interest was cancer, (3) the study evaluated the association between Int7G24A rs334354 polymorphism and the risk for cancer, (4) the study reported sufficient data to calculate odds ratios (ORs) and their 95% confidence intervals (CI), (5) the study should be human research. Additionally, we excluded reviews, editorials, non-human studies and letters without sufficient data. When multiple reports based on the same study were published, only the most recent or complete report could be used.
Data extraction
Eligibility evaluation and data extraction were carried out independently by two reviewers (W. W. and Y.T.). Discrepancies were adjudicated by discussion with a third reviewer (Q.L.). The following information was extracted from all the identified studies: name of first author, year of publication, country where the study was performed, ethnicity, type of control source, type of cancer, method for genotyping, total number of cases and controls and the frequencies of every genotype.
Quality assessment
The qualities of the included studies were accessed by two authors respectively according to a set of predetermined criteria (Table 2), which was extracted and modified from previous studies38. In this scale for quality assessment, six items, including the representativeness of cases, source of controls, ascertainment of cancer, quality control of genotyping methods, sample size and HWE, were carefully checked. P value less than 0.01 was considered significant departure from HWE. The total scores ranged from 0 to 10, with higher scores indicating better quality. Two investigators scored the studies independently and solved disagreement through discussions.
Statistical analyses
The heterogeneity among studies was estimated by the Cochran Q test, which confirmed the heterogeneity at a significance level of P ≤ 0.10. Fisher’s exact test was used to check for deviations from Hardy–Weinberg equilibrium (HWE) among the controls in each study39. A method for meta-analysis of case-control genetic association studies using logistic regression developed by Bagos et al.36 was conducted in our paper. This method, making use of the binary structure of the data and by treating the genotypes as independent variables in a logistic regression, was a simple and commonly used methodology that performs satisfactorily and flexibly. Considering A is the allele reported to be related with increased risk for disease, parameters θ2 and θ3 are log ORGA/GG and log OR AA/GG, respectively, as defined by the following logistic regression model:

where πij is the disease risk for the jth genotype in the ith study and zi2 and zi3 are dummy variables indicating genotypes GA and AA, respectively. If there was heterogeneity on at least one of these odds ratios (ORGA/GG and OR AA/GG), random-effect model36 would be used to pool the effect, whereas fixed-effect model would be applied. The appropriate genetic model was determined based on the following relationship between θ2 and θ3, as follow:
-
1
No association: θ2 = θ3 = 0 (ORGA/GG = ORAA/GG = 1);
-
2
Recessive model: θ2 = 0 (ORGA/GG = 1) and θ3 ≠ 0 (ORAA/GG ≠ 1);
-
3
Dominant model: θ2 ≠ 0, θ3 ≠ 0 and θ2 = θ3 (ORGA/GG = ORAA/GG ≠ 1);
-
4
Multiplicative codominant model: θ2 ≠ 0, θ3 ≠ 0 and 2θ2 = θ3 (OR2GA/GG = ORAA/GG).
Once the best genetic model was identified, the three genotypes were collapsed into two groups to obtain the pooled results. Notably, Among the 13 studies, there were 2 studies13,18 which had cells with no count. Considering the potential risk of inflating the magnitude of the pooled effect after the exclusion of studies with zero cell counts, these studies will be included in our meta-analysis40. A common way to deal with this problem is to add 0.5 to each cell of the 2 × 2 table for the study41. In our work, this correction to all cell counts was automatically added by STATA42. If there were more than one type of cancer reported in one study, total number of participants reported to be cancer case would be extracted and compared them with the control group.
To explore the potential heterogeneity among studies, subgroup analyses were conducted according to ethnicity, control source, genotyping method, type of sample, type of cancer and sample size. In addition, sensitivity analyses were employed to find potential origins of heterogeneity and to examine the influence of various exclusions on the combined OR. Besides, the results from quality-effect model was introduced to be compared with those from random-effect model and fixed-effect model43 to evaluate the sensitivity of our results. Publication bias was evaluated through the visual inspection of funnel plots and with tests of Begg rank correlation44 and Egger regression asymmetry45. P < 0.05 was considered to be representative of a significant statistical publication bias. Forest plots were applied to assess the association between Int7G24A rs334354 polymorphism and cancer risk. Power analysis was performed to calculate the power for our meta-analysis to detect the estimated risk of the association between Int7G24A polymorphism and cancer risk. In addition to the power calculation (using Quanto version 1.2.4) and quality-effect modeling (applying MetaXL version 2.2 software), all the other statistical analyses were performed with STATA version 12.0 software (Stata Corporation, College Station, Texas, United States). All reported probabilities (P values) were two-sided.
Additional Information
How to cite this article: Wu, W. et al. Association between Int7G24A rs334354 polymorphism and cancer risk: a meta-analysis of case-control studies. Sci. Rep. 5, 11350; doi: 10.1038/srep11350 (2015).
References
Siegel, R., Ma, J., Zou, Z. & Jemal, A. Cancer statistics, 2014. CA Cancer J Clin 64, 9–29 (2014).
Ferlay, J. et al. Estimates of worldwide burden of cancer in 2008: GLOBOCAN 2008. Int J Cancer 127, 2893–2917 (2010).
Yancik, R. Population Aging and Cancer: A Cross‐National Concern. The Cancer Journal 11, 437–441 (2005).
Massagué, J. TGFβ in cancer. Cell 134, 215–230 (2008).
Gold, L. I. The role for transforming growth factor-beta (TGF-beta) in human cancer. Crit Rev Oncogen 10, 303–360 (1998).
Massagué, J., Blain, S. W. & Lo, R. S. TGFβ signaling in growth control, cancer and heritable disorders. Cell 103, 295–309 (2000).
Epstein, F. H., Blobe, G. C., Schiemann, W. P. & Lodish, H. F. Role of transforming growth factor β in human disease. New Engl J Med 342, 1350–1358 (2000).
Lönn, P., Morén, A., Raja, E., Dahl, M. & Moustakas, A. Regulating the stability of TGFβ receptors and Smads. Cell Res 19, 21–35 (2009).
Cox, D. G., Penney, K., Guo, Q., Hankinson, S. E. & Hunter, D. J. TGFB1 and TGFBR1 polymorphisms and breast cancer risk in the Nurses’ Health Study. BMC Cancer 7, 175 (2007).
Pasche, B. et al. TGFBR1* 6A and cancer: a meta-analysis of 12 case-control studies. J Clin Oncol 22, 756–758 (2004).
Castillejo, A. et al. TGFB1 and TGFBR1 polymorphic variants in relationship to bladder cancer risk and prognosis. Int J Cancer 124, 608–613 (2009).
Chen, T. et al. Int7G24A variant of transforming growth factor-beta receptor type I is associated with invasive breast cancer. Clin Cancer Res 12, 392–397 (2006).
Chen, T. P. et al. An intronic variant of the TGFBR1 gene is associated with carcinomas of the kidney and bladder. Int J Cancer 112, 420–425 (2004).
Song, B. et al. TGFBR1* 6A and Int7G24A variants of transforming growth factor-β receptor 1 in Swedish familial and sporadic breast cancer. Brit J Cancer 97, 1175–1179 (2007).
Zhang, H.-T. Int7G24A variant of the TGFBR1 gene and cancer risk: A meta-analysis of three case-control studies. Lung Cancer 49, 419–420 (2005).
Zhang, X. L. et al. The association of polymorphisms on TGFBR1 and colorectal cancer risk: a meta-analysis. Mol Biol Rep 39, 2567–2574 (2012).
Castillejo, A. et al. The Int7G24A variant of transforming growth factor-beta receptor type I is a risk factor for colorectal cancer in the male Spanish population: a case-control study. BMC Cancer 9, 406 (2009).
Chen, T. et al. Structural alterations of transforming growth factor‐β receptor genes in human cervical carcinoma. Int J Cancer 82, 43–51 (1999).
Dai, L. et al. A case-control study of childhood acute lymphoblastic leukaemia and polymorphisms in the TGF-beta and receptor genes. Pediatr Blood Cancer 52, 819–823 (2009).
Forsti, A. et al. Polymorphisms in the Transforming Growth Factor Beta 1 Pathway in Relation to Colorectal Cancer Progression. Gene Chromosome Canc 49, 270–281 (2010).
Guo, W. et al. Association of polymorphisms in transforming growth factor-beta receptors with susceptibility to gastric cardia adenocarcinoma. Mol Biol Rep 39, 4301–4309 (2012).
Hu, Y. S. et al. Int7G24A variant of transforming growth factor-beta receptor 1 is associated with osteosarcoma susceptibility in a Chinese population. Med Oncol 28, 622–625 (2011).
Skoglund Lundin, J. et al. TGFBR1 variants TGFBR1*6A and Int7G24A are not associated with an increased familial colorectal cancer risk. Brit J Cancer 100, 1674–1679 (2009).
Zhang, H.-T. et al. Mutational analysis of the transforming growth factor β receptor type I gene in primary non-small cell lung cancer. Lung Cancer 40, 281–287 (2003).
Zhang, Y. J. et al. Genetic polymorphisms of transforming growth factor-beta 1 and its receptors and colorectal cancer susceptibility: A population-based case-control study in China. Cancer Lett 275, 102–108 (2008).
Dong, Z. M. et al. Correlation of TGF-breceptor type 1 gene 6A and Int7G24A polymorphisms with the risk of esophageal squamous cell carcinoma. Carcinogenesis,Teratogenesis & Mutagenesis 23, 16–21 (2011). (in chinese).
Risch, N. & Merikangas, K. The future of genetic studies of complex human diseases. Science 273, 1516–1517 (1996).
Ponder, B. A. Cancer genetics. Nature 411, 336–341 (2001).
Herpin, A., Lelong, C. & Favrel, P. Transforming growth factor-β-related proteins: an ancestral and widespread superfamily of cytokines in metazoans. Dev Comp Immunol 28, 461–485 (2004).
Bierie, B. & Moses, H. L. Transforming growth factor beta (TGF-β) and inflammation in cancer. Cytokine Growth F R 21, 49–59 (2010).
Moore-Smith, L. & Pasche, B. TGFBR1 signaling and breast cancer. J Mammary Gland Biol 16, 89–95 (2011).
Rosman, D. S., Bromberg, Y., Weinstein, A. & Reiss, M. Phenotypic diversity of disease-associated transforming growth factor-β (TGF-β) type I receptor gene (TGFBR1) mutants. Cancer Res 71, 2118–2118 (2011).
Tomsic, J. et al. Allele-specific expression of TGFBR1 in colon cancer patients. Carcinogenesis 31, 1800–1804 (2010).
Minelli, C., Thompson, J. R., Abrams, K. R., Thakkinstian, A. & Attia, J. The choice of a genetic model in the meta-analysis of molecular association studies. Int J Epidemiol 34, 1319–1328 (2005).
Thakkinstian, A., McElduff, P., D’Este, C., Duffy, D. & Attia, J. A method for meta‐analysis of molecular association studies. Stat Med 24, 1291–1306 (2005).
Bagos, P. G. & Nikolopoulos, G. K. A method for meta-analysis of case-control genetic association studies using logistic regression. Stat Appl Genet Mol Biol 6, 1, 10.2202/1544-6115.1281 (2007).
Moher, D., Liberati, A., Tetzlaff, J. & Altman, D. G. Preferred reporting items for systematic reviews and meta-analyses: the PRISMA statement. Ann Intern Med 151, 264–269 (2009).
Guo, J., Jin, M., Zhang, M. & Chen, K. A genetic variant in miR-196a2 increased digestive system cancer risks: a meta-analysis of 15 case-control studies. PLoS One 7, e30585 (2012).
Munafò, M. R., Clark, T. G. & Flint, J. Assessing publication bias in genetic association studies: evidence from a recent meta-analysis. Psychiatry Res 129, 39–44 (2004).
Friedrich, J. O., Adhikari, N. K. & Beyene, J. Inclusion of zero total event trials in meta-analyses maintains analytic consistency and incorporates all available data. BMC Med Res Methodol 7, 5 (2007).
Liu, X. et al. Association between smoking and p53 mutation in lung cancer: a meta-analysis. Clin Oncol 26, 18–24 (2014).
Sterne, J. A., Bradburn, M. J. & Egger, M. Systematic Reviews in Health Care: Meta-Analysis in Context 2nd edn (eds Egger, M. et al. ) Ch. 18, 347–369, 10.1002/9780470693926.ch18 (John Wiley & Sons, 2008).
Doi, S. A. & Thalib, L. A quality-effects model for meta-analysis. Epidemiology 19, 94–100 (2008).
Begg, C. B. & Mazumdar, M. Operating characteristics of a rank correlation test for publication bias. Biometrics 50, 1088–1101 (1994).
Egger, M., Smith, G. D., Schneider, M. & Minder, C. Bias in meta-analysis detected by a simple, graphical test. BMJ 315, 629–634 (1997).
Acknowledgements
The financial supports from National Basic Research Program of China (973 Program, No. 2015CB352100), Program for New Century Excellent Talents in University (No. NCET-13-0232) and National Natural Science Foundation of China (NSFC, No. 81302497) are greatly acknowledged.
Author information
Authors and Affiliations
Contributions
All authors contributed significantly to this work. W.W., Y.T. and Q.L. designed the research study; W.W., Y.T., Q.Z., G.Y. and X.W. performed the research study and collected the data; W.W., Y.T. and Q.Z. analyzed the data; W.W., Y.T. and Q.L. wrote the paper; Q.Z., G.Y. X.P. and X.W. prepared Tab. 1-2 and Figs 1–8. All authors reviewed the manuscript. In addition, all authors approved the final draft.
Ethics declarations
Competing interests
The authors declare no competing financial interests.
Rights and permissions
This work is licensed under a Creative Commons Attribution 4.0 International License. The images or other third party material in this article are included in the article’s Creative Commons license, unless indicated otherwise in the credit line; if the material is not included under the Creative Commons license, users will need to obtain permission from the license holder to reproduce the material. To view a copy of this license, visit http://creativecommons.org/licenses/by/4.0/
About this article
Cite this article
Wu, W., Tong, Y., Wei, X. et al. Association between Int7G24A rs334354 polymorphism and cancer risk: a meta-analysis of case-control studies. Sci Rep 5, 11350 (2015). https://doi.org/10.1038/srep11350
Received:
Accepted:
Published:
DOI: https://doi.org/10.1038/srep11350
This article is cited by
Comments
By submitting a comment you agree to abide by our Terms and Community Guidelines. If you find something abusive or that does not comply with our terms or guidelines please flag it as inappropriate.