Abstract
The selection of native greening plants to improve rural greening technology is crucial for enriching methods of building rural plant landscapes. However, there are few studies from the perspective of visual preference using quantitative methods. By using eye-tracking technology, this study studies students in the Central South University of Forestry and Technology and villagers in Changkou Village, Fujian Province, employing pictures of plant organs—leaves, flowers, and fruits—as stimulating materials to analyze five indicators: the total duration of fixations, the number of fixations, average duration of fixations, average pupil size and average amplitude of saccades. A number of findings came from this research First, people visually prefer leaves, followed by flowers and fruits. In terms of species, Photinia × fraseri, Metasequoia glyptostroboides, Photinia serratifolia, Cunninghamia lanceolata and Koelreuteria bipinnata have higher overall preference. Families such as Malvaceae, Fabaceae, Araliaceae, Myricaceae and Cupressaceae have stronger visual attraction than others. Second, there are distinct differences in the preference of shapes and textures of leaves: aciculiform, strip, cordiform, sector and jacket-shape are more attractive; leather-like leaves have a higher visual preference than paper-like leaves; different colors and whether leaves are cracked or not have little effect on leaf observation. Third, the preference for flowers with different inflorescence and colors is significant. Capitulum, cymes and panicles are more attractive; purple is the most preferred color, followed by white, yellow and red. Finally, there are significant differences in preferences for fruit characteristics, with medium-sized fruits and black fruits preferred, while kidney-shaped and spoon-shaped fruits are considered more attractive. Pomes, pods, samaras, and berries have received relatively more attention.
Similar content being viewed by others
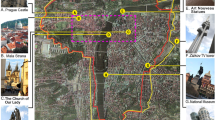
Introduction
With rapid economic growth and urbanization, unprecedented change has come to China’s rural regions. As a result, the traditional rural landscape has been greatly disturbed, affecting agricultural production and the quality of life of rural farmers. Currently, traditional urban greening technology is used primarily in China’s rural greening programs, while the application of native plants has not attracted sufficient attention, accounting for a relatively small proportion of tree species used. There are, however, major challenges in native implementation, such as vegetation type1, lack of characteristics2 and lack of seedling sources for native plants3. The consequent phenomenon of “a thousand identical villages” divorces the human environment from the natural landscape of rural areas in China. In the face of emergent scenarios and new problems, research, and development of greening technology suitable for Chinese villages has become a necessary technological breakthrough for the goal of constructing “green and livable rural villages and towns”4.
Plants are one of the most important elements of open spaces, and of environmental perception and preference1. Some scholars use the term “visual environment” to describe people’s visual perception of their surroundings2,3,4, but more prefer the term “visual landscape” to highlight the visual attributes of the landscape5,6,7. The landscape described as ‘the environment’ in this context can be anything from micro-scale to macro-scale, referring to urban parks, plants8 and plant organs. Visual preference for landscape refers to the influence of landscape and its elements on people9,10,11. Landscape perception and preference are dominated by limitations in human vision to a great extent12; however, some scholars have proposed that merits and inferiorities of the landscape could be judged by most observors13. Since visual landscape study emerged as a field of research in the West in the twentieth century, many scholars have examined characteristics of people’s preference for natural landscapes through a variety of subjective and objective methods14,15,16, concluding that characteristics of the landscape itself have a huge impact on visual preference15,17,18.
Whatever research method is adopted, most scholars use pictures as a medium for landscape research19,20,21. However, since pictures cannot fully reflect the actual viewing experience and cannot show the inherent diversity of the landscape, their value for research is limited22. A large number of studies have shown that social measurement research based on images correlates well with a parallel reaction in directly experiencing the represented landscape23,24. Compared with physical experiments, the use of pictures saves valuable research funding and provides better control over the experimental subjects. Therefore, pictures present a cost-effective medium for public visual preference research25,26. The use of eye-tracking technology to collect eye movement information to analyze people’s cognitive process and preference characteristics is therefore potentially useful within this framework27. Eye movement information mainly includes fixations, saccades and following up28. The corresponding indicators, such as the total duration of fixations, the average duration of fixations, the number of fixations and the average amplitude of saccades can be used to measure the attraction of stimulation target to subjects29,30. In addition, changes in pupil size have always been related to people’s interest in visual stimulation31.
Current research suggests that plant landscapes are images represented by vegetation, plant communities, and individual plants32, but not plant organs as landscapes. The structural features and systems of plants have been shown to have an important impact on how people interpret plants30. Plant growth status, plant coverage, seasonal changes, hierarchy, characteristics of individual plants and the choice of characteristic plants15,33 will affect viewers’ visual preference. Eye-tracking, for example, can reveal viewer visual preferences for different plant features30. Viewers have been shown to pay more attention to color34 and seasonal color changes35, indicating that color is one of the most tangible and influential aspects of visual preference. This provides a good way to adjust, directly guide and induce positive outcomes on individual mental states34. Some scholars have turned their attention to the shapes and sizes of trees36 and the stripes of the stems and leaves of bamboo in producing stimuli. General research has identified that the most popular seasonal landscape season is summer, and it has been observed that when observed in summer, features such as shapes, stems, leaves, flowers and fruits are very effective in producing positive stimuli1; however, no in-depth study has been undertaken. In effect, there is no systematic integration of people’s preferences when organs are used as plant landscapes.
The selection and application of various plants are very important in rural greening37, with plant selection crucial in producing positive effects, especially for rural areas. Choosing favored native plants does not just realize the function of rural greening, but also highlights local characteristics, maintain a unique regional flavor, supporting the cultural landscape and tourist attractions, thus improving the effect and quality of rural greening38. Consequently, the goal of this study is to select favourable native plants from the perspective of visual preference to build supporting evidence applicable to rural greening and landscape construction in China.
Methods
Stimuli
Experimental pictures were sourced either independently or from the official website of the Institute of Botany, Chinese Academy of Sciences (http://www.ibcas.ac.cn) and the website of China Botanical Garden Alliance (https://www.cubg.cn). For the screening of plant species, a list of common native plants suitable for greening in subtropical areas was identified in the relevant literature, determined on the basis of natural distribution, then 65 native plants for greening were selected according to the opinions of botany experts. Finally, 52 native plants for greening, comprising both trees and shrubs, were obtained after filtering vines and herbs (see Table 1). For experimental photography screening, 40 kinds of plants were selected from the list, including 25 trees and 15 shrubs, according to the list of selected plants, with leaves, flowers, and fruits as classification criteria. Then pictures with leaves, flowers, or fruits of the plants as the core elements were taken and downloaded, and the background of the pictures was blurred by the depth of field mode or Photoshop CS6 software to highlight the experimental theme. To minimize tonal interference between different pictures, the picture hue was processed uniformly without changing the color of the plant itself. A total of 120 experimental pictures were obtained, and the size of all pictures was 4496 × 3000 pixels (see Fig. 1). Each experimental picture reflected some specific attributes, such as leaf color, shape, texture, and cracks; flower color, shape, and inflorescence; fruit size, color, and shape (see Table 2). For the convenience of this study, fruit with a diameter greater than 5 cm was classified as a large fruit, 3–5 cm a medium fruit, 1–3 cm a small fruit, and less than 1 cm a micro fruit. In addition, to prevent the interference of primacy effect in the experiment23, one picture of leaves, one of flowers, and one of fruits of other native plants which were not in the experimental list were selected as the preheating pictures before the experiment. In total, the subjects were required to browse a total of 123 pictures in the formal experiment.
Examples of plant pictures used in the eye-tracking experiment: (a, b): leaf; (c, d): flower; (e, f): fruit. All pictures were taken by the project researcher or downloaded from the Institute of Botany, Chinese Academy of Sciences (http://www.ibcas.ac.cn) and the website of China Botanical Garden Alliance (https://www.cubg.cn).
Environment
When the eye tracker is used in a real-life background, there are many uncontrollable interferences in the environment for the subjects, and the ever-changing natural light will also lead to the deviation of the pupil detection of the subjects39,40, which makes it difficult to ensure the reliability of the data. Therefore, the experiments were carried out in the Tourism College of CSUFT’s laboratory in Hunan Province and Changkou Village Committee’s meeting room in Fujian Province. Experimental pictures were displayed using PowerPoint projection, and the subjects sat directly in view of the projection. The indoor environment was controlled to be noiseless, dimly lit41 and comfortable in temperature and humidity, ensuring that the subjects were not affected by the outside world and clearly observed the experimental pictures.
Equipment
Eye movement data were recorded using the Tobii Pro Glasses 2 wearable eye movement meter, with a lightweight design and four eye-movement cameras with resolution of 1920 × 1080@25fps. The eye movement of the subject is tracked by pupil corneal reflection and binocular dark pupil acquisition technology, which has wireless real-time observation function and supports slip compensation. If the subject touches the headset or rotates in a small range, the data quality will not be affected. The built-in gyroscope and acceleration sensor, with 0.5 precision and 100 Hz stable sampling rate, ensure extremely high data validity. In the experiment, Tobii Pro Glasses Controller software was used on one Surface tablet computer for eye movement calibration, real-time observation, eye movement video export and other operations, and the experimental pictures were shown on another computer. Eye movement data were recorded and saved to the SD card of the recording device, matched with the eye movement instrument.
Subjects
In sociological and psychological studies, it is considered that when the effective sample size of this type of experiment reaches more than 30, it is understood as a large sample experiment, and reliable sample reliability can be obtained42,43. At the same time, in similar eye tracking experiments, there are generally between 30 and 90 subjects44,45,46. At present, most researchers take people of the age of college students and above as subjects47,48, and villagers were the first visual audience of the landscape created by native greening plants.
For this study, 59 subjects—20 males and 39 females—aged between 18 and 63 years49 were selected, including 33 undergraduates and postgraduates (MAge = 21.79, SDAge = 2.176) in CSUFT of Hunan Province and 26 villagers (MAge = 47.23, SDAge = 10.359) in Changkou Village of Fujian Province. All the subjects had good naked or corrected vision, no color blindness or color weakness, and all passed the eye movement calibration test used in the experiment, meeting the experimental requirements. Participants were rewarded with a cash gift of 30–50 yuan for their participation. The experiment obtained the informed consent of all subjects, and all procedures were carried out in accordance with relevant guidelines and regulations. The academic committee of CSUFT approved the study. Specific consent was not obtained because the data were analyzed anonymously.
Pre-experiment
A preliminary experiment was conducted at Tourism College of CSUFT from March 23–24, 2021, with 12 subjects. A total of 55 species of plants were selected in the pre-experiment, with a total of 135 plant photos. 3 × 3 jigsaw puzzles were used in the material processing of the experiment, with 45 in total. According to the standards of trees, shrubs, and plant organs, there were five random jigsaw puzzles showing leaves, flowers and fruits, and each jigsaw puzzle was randomly recombined by changing the position of the plant pictures—that is, the same plant picture would be observed by subjects in different positions three times. In the experiment, participants wore eye movement equipment and sat quietly in front of the slide. A total of 12 valid sets of eye movement data were obtained.
Experimental results showed that the relative position of pictures in the jigsaw puzzle had significant influence on the participants’ response, and it was difficult to accurately obtain reliable subject preferences for local greening plants. This preliminary study showed the shortcomings of the experimental scheme, and after reflection, study and modification, the jigsaw puzzle mode was finally abandoned and replaced by a single-picture pattern as the experimental stimulus. The overall framework of the formal experiment was determined on the basis of experts' opinions.
Procedure
Before the experiment, participants were instructed to sit down before the projection and familiarize themselves with the experimental environment. The researcher wore an eye tracker and explained the experimental process and requirements to the subjects. Subjects were then instructed to carry out eye movement calibration with eye movement calibration cards. After the calibration, three preheated pictures and 120 experimental pictures were played in turn. The experimental pictures were automatically and randomly played, and each picture was displayed for 10 s. In order to reproduce the visual preference and observation habits of the subjects in real life44, the subjects did not know the specific purpose of the experiment50; this was to prevent deliberate action due to psychological suggestion. The experimental process of each subject was about 20 min. Some subjects developed eye fatigue after long periods51, which led to a decrease in the number of eye movements52 and an overall decrease in the accuracy53. Therefore, subjects were allowed to signal that they wished to pause at any time during observation49. Another eye movement calibration would be repeated once the participant continued. After the experiment, demographic characteristics of the subjects were collected, including name, age, gender and education level, and the cash gift was issued to express gratitude.
Data processing
The purpose of this experiment was to study the visual preference of the subjects for native greening plants from the aspects of plant characteristics, families, and species. Therefore, in the process of sorting and analyzing eye movement data, we first classified and coded plants by species, families, organs, and characteristics (see Table 2), using them as independent variables and eye movement indicators as dependent variables in SPSS 25.0 software for the analysis of variance (ANOVA). In addition, to explore the basic association structure of different species, we used total duration of fixations and number of fixations as variables for hierarchical cluster analysis on species.
Specifically, the eye movement data were pre-processed and exported by Tobii Pro Lab software. First, the AOI of each experimental picture was determined and drawn—that is, the leaf, flower, or fruit area. Then, according to the research requirements, the required eye movement index data were selected and the TSV format file was exported based on AOI. The eye movement indexes selected in this study were mainly fixation time and fixation number, including total duration of fixations, average duration of fixations, number of fixations, average amplitude of saccades and average pupil size. In the experiment, 54 valid eye movement data were obtained after unusable data with chaotic eye movement trajectory or low sampling rate were deleted. In the process of sorting out eye movement data, plant characteristics were first classified and coded, then data integration, classification, calculation, and analysis were carried out on this basis.
Results
Differences of eye movement indexes among different families, species, and organs
According to the results of ANOVA of eye movement data, different families, species, and organ types show significant differences in three indicators—total duration of fixations, number of fixations and average duration of fixations—and some show differences in average pupil size and average amplitude of saccades (see Table 3).
Plant organs
At the level of organ types (see Table 4), leaves have the most total duration of fixations and number of fixations (MDLeaf flower = 1.031, MDLeaf fruit = 1.767), followed by flowers (MDFlower fruit = 0.736). Fruits have the lowest fixation duration and number of fixations, but the average duration of fixations is the longest, which is significantly different from leaves (MDFruit leaf = 0.032) but has no significant difference from flowers.
Species
Among 52 kinds of native greening plants, the average trend of total duration of fixations and number of fixations is basically the same (see Fig. 2). Photinia × fraseri (MDuration = 6.692, MNumber = 22.02) has the largest mean value in these two indexes, Metasequoia glyptostroboides, Photinia serratifolia, Cunninghamia lanceolata, Koelreuteria bipinnata, Hibiscus mutabilis and Cercis chinensis are at the forefront, and the three indexes including average duration of fixations of Pinus thunbergii and Yulania biondii are in the top ten. Malus halliana, Buxus sinica, Pinus massoniana, Celtis sinensis and Jasminum mesnyi have less fixation duration and number of fixations. There are also significant differences in average pupil size and average amplitude of saccades among different species. Pinus massoniana (MPupil = 4.347, MSaccade = 5.758) has the largest mean value in both indexes. In addition, the average pupil size values of Chimonanthus praecox and Photinia × fraseri are also very high, while those of J. mesnyi, Cinnamomum camphora and K. bipinnata are lower. The average amplitude of saccades of Acer buergerianum and C. lanceolata is large, and that of B. sinica, Y. biondii and Mahonia fortunei is small.
Family
Among the corresponding 25 families, Malvaceae, Fabaceae, Araliaceae, Myricaceae and Cupressaceae rank the top five in terms of total duration of fixations and number of fixations, while Calycanthaceae, Ebenaceae, Pinaceae, Magnoliaceae and Anacardiaceae have the highest average duration of fixations, Buxaceae and Cannabaceae are in a low state among the three indexes. Araliaceae has more total duration and number of fixations, while the average duration of fixations is relatively low. There are also significant differences in the average amplitude of saccades among different families. The average amplitude of saccades of Anacardiaceae, Sapindaceae and Juglandaceae is large, while that of Buxaceae, Pittosporaceae and Cannabaceae is small (see Fig. 3).
Differences of eye movement indexes in different organs
Leaf
The ANOVA of eye movement data in response to different organ characteristics shows that the difference reflected by leaves is not obvious compared with those of flowers and fruits (see Table 5). There are significant differences in leaf shape in total duration and number of fixations (p = 0.000 < 0.05) (see Fig. 4). According to subsequent multiple comparisons, the differences between leaf shapes are from aciculiform and oval shape, among which aciculiform has the most total duration of fixations (M = 6.902) and number of fixations (M = 22.80), while oval shape (MDuration = 5.263, MNumber = 17.85) has the least. In addition, the texture of leaves is different in the total duration of fixations (p = 0.020 < 0.05, MDTexture = 0.254) (see Fig. 6), and there is no difference in eye movement indexes of other characteristics (see Figs. 5, 6 and 7).
Flowers
The inflorescences and colors of flowers show significant differences in the total duration of fixations and number of fixations (see Table 6), among which the capitulum (MDuration = 4.900, MNumber = 15.46) has the largest mean value, and there are significant differences between the catkin and other inflorescences, but the mean value of the above two indexes is the smallest (MDuration = 2.978, MNumber = 9.28). In addition, there are differences between solitary flowers, umbels and some inflorescences, and their average values are closely behind the capitulum and before the catkin (see Fig. 8). And there are differences in total duration of fixations, number of fixations and average duration of fixations between solitary flower and infinite inflorescence, but there is no difference in definite inflorescence. On the whole, solitary flower (MDuration = 4.756, MNumber = 14.19, MAverage duration = 0.373) has the largest mean in three indexes, followed by definite inflorescence and infinite inflorescence. With regard to flower colors, the total duration of fixations and the order and number of fixations are basically the same and the difference is big, but there is no difference in average duration of fixations. Purple (MDuration = 5.093, MNumber = 16.80) has the highest average value, followed by white, yellow and red. In addition, there are significant differences in the average amplitude of saccades among different colors. Multiple comparisons show that the difference mainly comes from white with the smallest average amplitude of saccades (M = 4.569). Yellow has the largest average amplitude of saccades (M = 5.119), followed by red and green (see Fig. 9).
Fruit
Virtually all other indexes show significant variance among the different characteristics of fruit (see Table 7). The mean value of two indexes of medium fruit (MDuration = 4.869, MNumber = 14.96) was the largest, followed by micro fruit and large fruit, and the smallest was small fruit. The average pupil size of large fruit is the largest (M = 4.32), while the saccade amplitude of small fruit is the largest (M = 5.007). There are also significant differences in the average fixation time of fruits of different sizes. The average duration of fixations for large fruit (M = 0.395) is the longest (see Fig. 10). In fruit color, there are distinct differences among different colors, and the order of total fixation duration and fixation times is consistent. The mean value of black fruit (MDuration = 5.419, MNumber = 16.93) is much higher than that of other colors, and the mean pupil size among different colors is also different. Brown (M = 4.202) has the highest mean value, followed by green and black (see Fig. 11). There are obvious differences in total fixation duration and number of fixations among fruits with different shapes, and the mean values of kidney-shaped fruits and spoon-shaped fruits are higher. At the same time, the average amplitude of saccades is also different, and the wing shape (M = 5.285) has the largest average amplitude of saccades, followed by spindle shape and spoon-shaped shape (see Fig. 12). From the aspect of fruit types, there are differences among different types of fruits, among which pome (MDuration = 5.761, MNumber = 18.00) has the most total duration of fixations and number of fixations, with pod, samara, and berry at the forefront. There are also obvious differences in average pupil size and average amplitude of saccades among different types. The average value of achene and aggregate fruit in the former is larger, and the average value of pome (M = 5.627) in the latter is the largest.
Differences of eye movement indicators in different educational backgrounds
The primary focus from the results is on eye movement differences between participants with university level education and above, and people with high school education and below. The data showed that there were significant differences in five eye movement indexes (p = 0.000 < 0.05), and the total duration of fixations, number of fixations, average pupil size and average amplitude of saccades of people with bachelor's education and above were much higher than those with high school education and below (MDDuration = 1.237, MDNumber = 5.57, MDPupil = 0.341, MDSaccade = 1.175), but the average duration of fixations is the opposite (see Table 8).
Cluster analysis
Cluster analysis of the total duration of fixations and number of fixations of the species involved in the study showed that all species could be divided into three clusters in terms of total duration of fixations. The first cluster contains only C. sinensis; the second cluster includes Pittosporum tobira, C. praecox, J. mesnyi, P. massoniana, B. sinica, M. halliana and Berberis thunbergia; and the remaining species are all in the third cluster (see Fig. 13). In terms of number of fixations, all species can be divided into four clusters. The first cluster contains Fokienia hodginsii, Cinnamomum japonicum, Cerasus campanulata, Choerospondias axillaris, A. buergerianum, B. thunbergii, M. halliana and C. sinensis; the second cluster contains J. mesnyi, Yulania liliiflora, B. sinica, C. praecox, P. tobira, P. massoniana and Ilex rotunda; the third cluster includes C. lanceolata, M. glyptostroboides, Y. biondii and Photinia × fraseri, and the rest of the species are included in the fourth cluster (see Fig. 14).
Discussion
Five eye movement indexes, total duration of fixations, average duration of fixations, number of fixations, average amplitude of saccades and average pupil size have been selected for analysis and summary. At present, academics in China and abroad have and applied and conducted explorations in many different fields using these indexes, concluding that total duration of fixations and number of fixations can reflect the visual preference of subjects to a certain extent. People prefer attractive stimuli with longer fixation time and more fixations54. Average pupil size is also related to preference31 as a measure, reflecting people’s sensitivity to stimulus materials30. On the contrary, the longer the average duration of fixations, the larger the information conveyed by stimulus, and the more time subjects need to spend acquiring and processing information55,56. The average amplitude of saccades reflects the range of information acquired. The larger the amplitude, the more vivid the picture featured, and the easier it is for subjects to directly reach the target area19.
The results show that there are significant differences in visual preferences among different plant organ types. People prefer leaves visually, followed by flowers, with fruits having the lowest visual preference. This is different from the research results in the majority of literature examined34,57, as there is variance between established facts and research preferences58. It was found in a study that although test subjects expressed their preference for plants with larger or colorful flowers, only one-third of the plants were blooming in the selected material59. Compared with flowers and fruits, processing leaves information60 requires less time and effort; by contrast, flowers and fruits convey a huge amount of visual information, which people take longer to process. In terms of species, Photinia × fraseri, M. glyptostroboides, P. serratifolia, C. lanceolata, K. bipinnata, H. mutabilis, C. chinensis, P. thunbergii and Y. biondii have higher overall preference, and the characteristics of P. massoniana and C. lanceolata are distinct and easily catch people’s eyes. Furthermore, at the botany family level, Malvaceae, Fabaceae, Araliaceae, Myricaceae and Cupressaceae have stronger visual appeal, and Anacardiaceae, Juglandaceae and Sapindaceae are more prominent.
The most prominent feature of plants is the differentiation of flowers and fruits in different seasons1. Therefore, although leaves have higher preference weight compared with flowers and fruits, the overall difference between leaves with different characteristics is indistinct. Obvious differences in eye movement indexes exist between different leaf shapes and textures, which influence preference. The influence of leaf shape is consistent with the research results of some academics59. Aciculiform, strip, cordiform, sector and jacket-shaped leaves are more attractive to people, and leather-like leaves have higher visual preference than paper-like leaves. However, little to no difference exists in preferences between different colors and whether leaves are cracked or not cracked. Vringer et al.61 also concluded that the color of flowers is more popular than the color of leaves.
People’s preference for flowers with different inflorescences and colors is very significant. In terms of inflorescence types, they prefer solitary flowers, finite inflorescences, and infinite inflorescences. Specifically, capitulum, cyme, panicle, corymb, and raceme are more attractive to people than the other three inflorescences. As for color, purple is the most preferred color, followed by white, yellow, and red, among which yellow and red are distinct and attention drawing, while white is the opposite. This is consistent with the conclusion of Rahnema et al.34, whose results show that red ranks first, followed by purple, orange and colorful (a combination of any of the other colors). The results of a study conducted in the United States also support this conclusion62.
Compared with leaves and flowers, all the characteristics of fruit deviate from each other, and different characteristics correlate strongly with fruit viewing results. First, there is a visual preference for medium-sized fruits, followed by micro-sized and large-sized fruits. Viewers need greater attention to acquire and process information conveyed by fruits of different sizes, and large-sized fruits can stimulate vision more effectively. Regarding color, black fruits are visually favored, followed by red, atropurpureus, green, yellow, and brown, with brown producing a stronger visual stimulation. In the analysis of other colors, white, green and yellow—which have high contrast—can create a lively atmosphere63; red provides an optimistic mood; and purple, like brown, may also stimulate vision64. In fruit shapes, kidney-shaped and spoon-shaped produced greater stimuli than other shapes, followed by wing and spherical shapes, among which the characteristics of wing and spoon-shaped are prominent and easily attract attention. From the aspect of fruit types, pome, pod, samara, and berry are paid more attention to, among which pome has the most prominent characteristics, but achene and aggregate fruit have greater visual stimulation.
There are significant differences in the duration and number of fixations among people with different educational levels. In the research process, most scholars hold the same view and think that apart from educational level16,65,66, group differences such as age67, professional background49 and cultural background68,69 will cause differences in visual preference characteristics and browsing patterns. Except for the average duration of fixations, the other four indicators show that participants with bachelor’s education and above rank much higher than those with high school education and below, which may indicate that people with a lower education level need more time to acquire and process the visual information conveyed, while higher education levels react in greater levels of sensitivity to leaves, flowers and fruits of plants, and can identify and lock the areas of interest effectively.
Conclusion
Through eye-tracking technology, viewer visual preference was examined through a sample of students and local villagers for native greening plants commonly used in subtropical areas in this study. Results found that the attraction of leaves was higher than that of flowers and fruits, and aciculiform, strip and leather-like leaves were preferred. Among the characteristics of flowers, capitulum and purple were more attractive. For fruits, medium fruits with a diameter of 3–5 cm were preferred, while black, red, and green fruits, and kidney-shaped and pome fruits received more attention. On the whole, the plants of Malvaceae, Fabaceae, Araliaceae, Myricaceae and Cupressaceae have higher visual preference, and Photinia × fraseri, M. glyptostroboides, C. lanceolata, P. serratifolia, K. bipinnata, H. mutabilis, C. chinensis, P. thunbergii and Y. biondii have stronger visual attraction, being the first choice for greening with native plants. This study is expected to provide scientific reference for the selection of native plants, improve rural greening technology, provide new scientific research ideas, and promote the improvement of settled rural environments. It will also contribute to the development of green and livable villages and towns in China.
Some limitations exist in this study that must be discussed. As only leaves, flowers and fruits are used as stimulating material from various plant organs, the research depth is limited. In future research, we should consider expanding our scope by taking individuals, populations, and communities as experimental subjects for further research, while adopting more evidence-based methodology in material selection. At the same time, the sample size of this study is limited, and follow-up studies must consider expanding the sample size to increase statistical reliability in the future. In addition, the study selected two varying groups of students and local villagers as participants, without discussing in depth the differences between them and the consequent effects on statistical results. Effective comparison methods should be adopted in follow-up research, and larger cases and sample sizes should be selected to explore the scope of the materials, to obtain a more universal conclusion.
References
Tarakci-Eren, E. & Duzenli, T. Determination of the visual preference levels and perceptual differences in the appearance of certain taxa in different seasons. Fresenius Environ. Bull. 26, 14 (2017).
Blasco, E. et al. Predicting scenic beauty of forest stands in Catalonia (North-east Spain). J. For. Res. 20, 73–78 (2009).
Ribe, R. G. Perceptions of forestry alternatives in the US Pacific Northwest: Information effects and acceptability distribution analysis. J. Environ. Psychol. 26, 100–115 (2006).
Yumin, Y., Xiaodong, Z., Yingbi, X., Haiyan, Y. & Xiang, S. Assessing the visual quality of urban waterfront landscapes: The case of Hefei China. Acta Ecol. Sin. 32, 5836–5845 (2012).
Daniel, T. C. Whither scenic beauty? Visual landscape quality assessment in the 21st century. Landsc. Urban Plan. 54(1–4), 267–281 (2001).
Schmid, W. A. The emerging role of visual resource assessment and visualisation in landscape planning in Switzerland. Landsc. Urban Plan. 54, 213–221 (2001).
Tang, Z. & Cheng, K. Study on visual impact assessment of highway landscape. J. Landsc. Res. 4, 1–3 (2012).
Naveh, Z. & Lieberman, A. S. Landscape Ecology: Theory and Application (Springer, 1994).
Arriaza, M., Cañas-Ortega, J. F., Cañas-Madueño, J. A. & Ruiz-Aviles, P. Assessing the visual quality of rural landscapes. Landsc. Urban Plan. 69, 115–125 (2004).
Dramstad, W. E., Tveit, M. S., Fjellstad, W. J. & Fry, G. L. Relationships between visual landscape preferences and map-based indicators of landscape structure. Landsc. Urban Plan. 78, 465–474 (2006).
Kahn, P. H. The Human Relationship with Nature: Development and Culture (MIT Press, 1999).
Lange, E. The limits of realism: Perceptions of virtual landscapes. Landsc. Urban Plan. 54, 163–182 (2001).
Qi, T. et al. Analysis of the influence factors of city park landscape visual quality: A case study of Zizhuyuan Park in Beijing. Hum. Geogr. 29(5), 69–74 (2014).
Berg, A. & Koole, S. L. New wilderness in the Netherlands: An investigation of visual preferences for nature development landscapes. Landsc. Urban Plan. 78, 362–372 (2006).
Kaltenborn, B. P. & Bjerke, T. Associations between environmental value orientations and landscape preferences. Landsc. Urban Plan. 59, 1–11 (2002).
Zhong, S. F., Shen, S. Y., Liao, Q. L. & Wang, W. W. Study on landscape preference for typical ornamental plant community of parks in Changsha. Guangdong Agricu. Sci. https://doi.org/10.3969/j.issn.1004-874X.2011.10.018 (2011).
Lokocz, E., Ryan, R. L. & Sadler, A. J. Motivations for land protection and stewardship: Exploring place attachment and rural landscape character in Massachusetts. Landsc. Urban Plan. 99, 65–76 (2011).
Pflüger, Y., Rackham, A. & Larned, S. The aesthetic value of river flows: An assessment of flow preferences for large and small rivers. Landsc. Urban Plan. 95, 68–78 (2010).
Guo, S. et al. Landscape visual quality assessment based on eye movement: College student eye-tracking experiments on tourism landscape pictures. Resour. Sci. 39(6), 1137–1147 (2017).
Walker, A. J. & Ryan, R. L. Place attachment and landscape preservation in rural New England: A maine case study. Landsc. Urban Plan. 86, 141–152 (2008).
Zhang, C. & Deng, C. Exploration into tourism aesthetic research: Based on visitor self-employed Photography. Tour. Sci. (2010). Available at: http://en.cnki.com.cn/Article_en/CJFDTOTAL-LUYX201004008.htm.
Palmer, J. F. & Hoffman, R. E. Rating reliability and representation validity in scenic landscape assessments. Landsc. Urban Plan. 54(1–4), 149–161 (2001).
Dachary-Bernard, J. & Rambonilaza, T. Choice experiment, multiple programmes contingent valuation and landscape preferences: How can we support the land use decision making process?. Land Use Policy 29, 846–854 (2012).
Meitner, M. J. Scenic beauty of river views in the Grand Canyon: Relating perceptual judgments to locations. Landsc. Urban Plan. 68(1), 3–13 (2004).
Coeterier, J. F. A photo validity test. J. Environ. Psychol. 3, 315–323 (1983).
Daniel, T. C. & Meitner, M. M. Representational validity of landscape visualizations: The effects of graphical realism on perceived scenic beauty of forest vistas. J. Environ. Psychol. 21, 61–72 (2001).
Duchowski, A. T. A breadth-first survey of eye-tracking applications. Behav. Res. Methods Instrum. Comput. 34, 455 (2002).
Chaparro, B. S. & Russell, M. C. Hotspots and hyperlinks: Using eye-tracking to supplement usability testing. Usability News 7(2), 1–11 (2005).
Gholami, Y., Taghvaei, S. H., Norouzian-Maleki, S. & Mansouri, S. R. Identifying the stimulus of visual perception based on eye-tracking in urban parks: Case study of Mellat Park in Tehran. J. For. Res. 26, 91–100 (2021).
Zheng, J. et al. Which ornamental features of bamboo plants will attract the people most?. Urban For. Urban Green. 61, 127101 (2021).
Hess, E. H. & Polt, J. M. Pupil size as related to interest value of visual stimuli. Science 132(3423), 349–350 (1960).
Clouston, B., Landscape Institute. Landscape Design with Plants (Elsevier, 1977).
Liu, R. & Peng, Y. Evaluation of plant landscape in the seaside green land based on public perception. J. Shenzhen Univ. (Sci. Eng.) 34, 385–392 (2017).
Rahnema, S., Sedaghathoor, S., Allahyari, M. S., Damalas, C. A. & Bilali, H. E. Preferences and emotion perceptions of ornamental plant species for green space designing among urban park users in Iran. Urban For. Urban Green. 39, 98–108 (2019).
Ho, M. C. et al. Numerical analysis on color preference and visual comfort from eye tracking technique. Math. Probl. Eng. 2015, 1–4 (2015).
Gudin, S. Overview of plant breeding. In Encyclopedia of rose science. 25–30 (Elsevier, 2003).
Zhang, M. J. & Hao, R. M. Development suggestions on native woody plants based on plant survey of main urban greenspace of Nanjing. Chin. Landsc. Arch. 31, 82–85 (2015).
Lu, S., Zheng, Y., Chen, B., Jing, J. & Lai, Y. N. Application of native woody plants in construction of plant communities in Hangzhou Landscape. J. Zhejiang Sci-Tech Univ. 33, 285–290 (2015).
Kiefer, P., Giannopoulos, I. & Raubal, M. Where am I? Investigating map matching during self-localization with mobile eye tracking in an urban environment. Trans. GIS https://doi.org/10.1111/tgis.12067 (2014).
Dong, W. et al. New research progress of eye tracking-based map cognition in cartography since 2008. Acta Geogr. Sin. 74(3), 599–614 (2019).
Hollenstein, N. et al. ZuCo, a simultaneous EEG and eye-tracking resource for natural sentence reading. Sci. Data 5, 180291 (2018).
Gomm, R. Social Research Methodology (Macmillan, 2004).
Wang, J., Lin, L., Gao, H. & Zhang, L. Differences in college students’ spatial symbol cognition of tourism map: Based on experimental data from an eye-movement tracking system. Tour. Trib. 31(3), 97–105 (2016).
Dupont, L., Ooms, K., Antrop, M. & Van Eetvelde, V. Comparing saliency maps and eye-tracking focus maps: The potential use in visual impact assessment based on landscape photographs. Landsc. Urban Plan. 148, 17–26 (2016).
Ren, X. Consensus in factors affecting landscape preference: A case study based on a cross-cultural comparison. J. Environ. Manage. 252, 109622 (2019).
Pihel, J., Ode, S. Å., Hagerhall, C. & Nyström, M. Expert and novice group differences in eye movements when assessing biodiversity of harvested forests. For. Policy Econ. 56, 20–26 (2015).
Amati, M., Ghanbari, P. E., McCarthy, C. & Sita, J. How eye-catching are natural features when walking through a park? Eye-tracking responses to videos of walks. Urban For. Urban Green. 31, 67–78 (2018).
Kim, B. & Park, J. The visual effect of signboards on the vitality of the streetscapes using eye-tracking. Sustainability 13, 30 (2020).
Dupont, L., Antrop, M. & Eetvelde, V. V. Does landscape related expertise influence the visual perception of landscape photographs? Implications for participatory landscape planning and management. Landsc. Urban Plan. 141, 68–77 (2015).
Ding, X. et al. Classifying major depression patients and healthy controls using EEG, eye tracking and galvanic skin response data. J. Affect. Disord. 251, 156–161 (2019).
Blehm, C., Seema, V. F., Khattak, A., Mitra, S. & Yee, R. W. Computer vision syndrome: A review. Surv. Ophthalmol. 50, 253–262 (2005).
Orden, K. V., Jung, T. & Makeig, S. Combined eye activity measures accurately estimate changes in sustained visual task performance. Biol. Psychol. 52, 221–240 (2000).
McGregor, D. K. & Stern, J. A. Time on task and blink effects on saccade duration. Ergonomics 39(4), 649–660 (1996).
Hall, C., Hogue, T. & Guo, K. Differential gaze behavior towards sexually preferred and non-preferred human figures. J. Sex Res. 48(5), 461–469 (2011).
Guo, F., Li, M., Hu, M., Li, F. & Lin, B. Distinguishing and quantifying the visual aesthetics of a product: An integrated approach of eye-tracking and EEG. Int. J. Ind. Ergon. 71, 47–56 (2019).
Scharinger, C., Schüler, A. & Gerjets, P. Using eye-tracking and EEG to study the mental processing demands during learning of text-picture combinations. Int. J. Psychophysiol. 158, 201–214 (2020).
Yin, F., Bai, Z. Y. & Hong-Cheng, H. E. A. Research into visitors’ landscape preference upon wetland plant community: The case of Yanghu Wetland Park. J. Cent. South Univ. For. Technol. (Soc. Sci.) 7, 37–41 (2013).
Sun, L., Shao, H., Li, S., Huang, X. & Yang, W. Integrated application of eye movement analysis and beauty estimation in the visual landscape: Quality estimation of urban waterfront park. Int. J. Pattern Recognit. Artif. Intell. 32, 1856010 (2018).
Lindemann-Matthies, P. & Bose, E. Species richness, structural diversity and species composition in meadows created by visitors of a botanical garden in Switzerland. Landsc. Urban Plan. 79, 298–307 (2007).
Keskin, M., Ooms, K., Dogru, A. O. & De Maeyer, P. Exploring the cognitive load of expert and novice map users using EEG and eye tracking. Int. J. Geo-Inf. 9, 429 (2020).
Vringer, K. & Blok, K. Long-term trends in direct and indirect household energy intensities: A factor in dematerialisation?. Energy Policy 28, 713–727 (2000).
Allen, W. The color of success: African-American college student outcomes at predominantly white and historically black public colleges and universities. Harv. Educ. Rev. 62, 26–45 (1992).
Burton, A., Bambrick, H. & Friel, S. If you don’t know how can you plan? Considering the health impacts of climate change in urban planning in Australia. Urban Clim. 12, 104–118 (2015).
Townshend, T. G. Therapeutic landscapes: An evidence-based approach to designing healing gardens and restorative outdoor spaces. HERD 40, 1–2 (2015).
Hu, L., Wen, L. I., Cao, M., Che, Y. & Yang, K. Study of urban waterfront landscape and public preference: A case study of Suzhou Creek in Shanghai. Environ. Sci. Technol. 37, 412–418 (2014).
Natori, Y. & Chenoweth, R. Differences in rural landscape perceptions and preferences between farmers and naturalists. J. Environ. Psychol. 28, 250–267 (2008).
Berg, A., Vlek, C. & Coeterier, J. F. Group differences in the aesthetic evaluation of nature development plans: A multilevel approach. J. Environ. Psychol. 18, 141–157 (1998).
Buhyoff, G. J., Wellmann, J. D., Koch, N. E., Gauthier, L. & Hultman, S. Landscape preference metrics: An international comparison. J. Environ. Manage. 16, 181–190 (1983).
Zube, E. H. & Pitt, D. G. Cross-cultural perceptions of scenic and heritage landscapes. Landsc. Plann. 8, 69–87 (2015).
Funding
The funding was provided by Ministry of Science and Technology of the People's Republic of China, (Grant Nos. 2019YFD1100404, 2019YFD1100404, 2019YFD1100404).
Author information
Authors and Affiliations
Contributions
N.D. performed the experimental work and wrote the manuscript. Y.Z. conceived the project and provided experimental and research framework. J.L. took pictures for the experiment and made suggestions on the kinds of plants for the experiment. Q.X. wrote part of this article. All authors reviewed the manuscript.
Corresponding author
Ethics declarations
Competing interests
The authors declare no competing interests.
Additional information
Publisher's note
Springer Nature remains neutral with regard to jurisdictional claims in published maps and institutional affiliations.
Supplementary Information
Rights and permissions
Open Access This article is licensed under a Creative Commons Attribution 4.0 International License, which permits use, sharing, adaptation, distribution and reproduction in any medium or format, as long as you give appropriate credit to the original author(s) and the source, provide a link to the Creative Commons licence, and indicate if changes were made. The images or other third party material in this article are included in the article's Creative Commons licence, unless indicated otherwise in a credit line to the material. If material is not included in the article's Creative Commons licence and your intended use is not permitted by statutory regulation or exceeds the permitted use, you will need to obtain permission directly from the copyright holder. To view a copy of this licence, visit http://creativecommons.org/licenses/by/4.0/.
About this article
Cite this article
Ding, N., Zhong, Y., Li, J. et al. Study on selection of native greening plants based on eye-tracking technology. Sci Rep 12, 1092 (2022). https://doi.org/10.1038/s41598-022-05114-0
Received:
Accepted:
Published:
DOI: https://doi.org/10.1038/s41598-022-05114-0
Comments
By submitting a comment you agree to abide by our Terms and Community Guidelines. If you find something abusive or that does not comply with our terms or guidelines please flag it as inappropriate.