Abstract
Tuberculosis (TB), caused by Mycobacterium tuberculosis, spreads via aerosols and the first encounter with the immune system is with the pulmonary-resident immune cells. The role of epigenetic regulations in the immune cells is emerging and we have previously shown that macrophages capacity to kill M. tuberculosis is reflected in the DNA methylome. The aim of this study was to investigate epigenetic modifications in alveolar macrophages and T cells in a cohort of medical students with an increased risk of TB exposure, longitudinally. DNA methylome analysis revealed that a unique DNA methylation profile was present in healthy subjects who later developed latent TB during the study. The profile was reflected in a different overall DNA methylation distribution as well as a distinct set of differentially methylated genes (DMGs). The DMGs were over-represented in pathways related to metabolic reprogramming of macrophages and T cell migration and IFN-γ production, pathways previously reported important in TB control. In conclusion, we identified a unique DNA methylation signature in individuals, with no peripheral immune response to M. tuberculosis antigen who later developed latent TB. Together the study suggests that the DNA methylation status of pulmonary immune cells can reveal who will develop latent TB infection.
Similar content being viewed by others
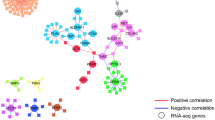
Introduction
Tuberculosis (TB) is a major global health concern, ranked as one of the top 10 causes of death worldwide and estimated to be responsible for 1.2 million deaths per year1. TB is caused by the facultative intracellular bacteria Mycobacterium tuberculosis and one-fourth of the world’s population is estimated to be infected making M. tuberculosis is one of the most successful pathogens known. M. tuberculosis is air-born and enters the lung where the bacteria are internalized by alveolar macrophages (AMs) through phagocytosis. The “checkpoint model” can be used to describe the following immunological events post infection2,3. At the first checkpoint, to establish an infection, M. tuberculosis needs to evade elimination by AMs. M. tuberculosis has developed several strategies to manipulate the host immune response to extend its survival in the phagocytes. The pathogen can arrest maturation of the phagolysosome and direct phagocytes to necrosis, which is prerequisite for the bacterium to spread. In some individuals, referred to as early clearers, the pathogen is successfully cleared by innate immune mechanisms at this initial stage of infection4,5,6. If the pathogen is replicating and cannot be cleared by the innate immune system, the second checkpoint is reached; activation of the adaptive immune system. When the infection is controlled by the adaptive immune system, asymptomatic latent TB infection has developed. From this point, there is a 5–10% lifetime risk of progression to active TB, as a result of inadequate immune control, which is the third checkpoint for M. tuberculosis7,8,9,10. The Interferon-Gamma Release Assay (IGRA) is an immunological test used to confirm whether a subject has been exposed to M. tuberculosis based on peripheral T cell release of interferon-γ (IFN-γ) in response to M. tuberculosis antigens11. The role of epigenetics in TB immune responses is emerging. Several studies have described the concept of developing trained immunity through epigenetic reprogramming, leading to proper orchestration of gene expression upon re-exposure to a pathogen or pathogen-derived products3,12,13. DNA methylation, histone modifications, and regulation of small RNAs are considered as the important players in the regulation of epigenetic modifications14. We and others have described the reprogramming of DNA methylation patterns in peripheral blood mononuclear cells (PBMCs) after exposure to live attenuated Mycobacterium bovis through the Bacillus Calmette Guérin (BCG) vaccination15,16. Further, we have demonstrated that differences in these DNA methylation patterns affect the efficacy of the macrophages to kill M. tuberculosis in vitro17. A wealth of literature focuses on adaptive immune responses in peripheral blood, but since M. tuberculosis primarily infects the lung, it is relevant to address pulmonary immunity, including alveolar T cells and AMs18,19. In this study, we have increased our focus on the pulmonary-resident immune cells and further investigated DNA methylomes of AMs and alveolar T cells in a cohort of medical students with a previously reported increased risk of M. tuberculosis exposure20. The aim of the study was to investigate epigenetic modifications in the pulmonary immune cells pre- and post-TB exposure in a natural setting. We hypothesized that recent exposure to M. tuberculosis in the lung compartment would induce epigenetic alterations in AMs and alveolar T cells. The AMs and alveolar T cells were isolated by sputum induction21 and using reduced representation of bisulfite sequencing (RRBS) the DNA methylome was investigated. We identified an altered DNA methylation profile in the pulmonary immune cells of the subjects that later developed latent TB infection as compared to those who tested negatively for latent TB throughout the study. Notably, this DNA methylation profile was identified in the immune cells before a latent TB infection could be detected with the IGRA test. The differentially methylated genes (DMGs) identified in the subjects developing latent TB infection were over-represented in the pentose phosphate pathway in AMs and in IFN-γ signaling and migration in the alveolar T cells.
Results
Study design and cohort
Medical students were invited to participate in the study and donated sputum samples before (referred here as 0 months) and after (referred here as 6 months) clinical rotations in departments with a high-risk of M. tuberculosis exposure. A schematic overview of the study design is represented in Fig. 1. Demographic information of the study subjects and IGRA results are shown in Table 1. In elaborate questionaries the health status of the study participants was evaluated, and no study participant had any conditions of immunosuppression or severe illnesses during the study. One study subject was borderline IGRA-positive22 (IGRApos) already at inclusion (0 months), IGRA results presented in supplementary Table S1. Two study subjects developed latent TB infection during the study as demonstrated with a positive IGRA test at follow-up (6 months, referred to as IGRA converters in the following text) (Table S1).
Flow chart of the study design. Study subjects donated sputum and blood samples at 0 and 6 months, which corresponded to before and after clinical rotations at departments with high-risk of M. tuberculosis exposure at hospitals in Lima, Peru. Interferon-Gamma Release Assay (IGRA) tests were taken to confirm TB-infection. Alveolar macrophages (AMs) and alveolar T cells were isolated from sputum samples. DNA was extracted and the samples were sequenced with reduced representation bisulfite sequencing (RRBS) for methylation analysis.
DNA methylation patterns in alveolar macrophages and alveolar T cells before and after TB exposure distinguish IGRA converters
To investigate the epigenetic changes in the pulmonary immune cells over time, we isolated DNA from the AMs and alveolar T cells21 collected at 0 and 6 months and performed Genome-wide Reduced Representation Bisulfite sequencing (RRBS). After filtering the data as described in the method section, we identified a total of 1,186 CpG-sites with ≥ 5 reads in the 19 samples from the AMs and 404 CpG-sites in the 19 samples from the alveolar T cells. To get an overview of the global DNA methylation distribution in the pulmonary immune cells, we made density plots of the M-values (the log2 ratio of the intensities of methylated versus unmethylated CpG-sites) obtained from CpG-sites in the AMs (1186 CpG-sites) and the alveolar T cells (404 CpG-sites), shown in Fig. 2a,c. We identified a clear difference in the methylation distribution between the cell types, AMs and alveolar T cells. The density plot showed a homogenous distribution of DNA methylation in the samples from the IGRAneg study subjects collected at both at 0 and 6 months. The sample from one study subject, that was borderline IGRApos at inclusion, followed the same global DNA methylation distribution. Whereas in the IGRA-converting study subjects, we identified a different global DNA methylation profile with more hyper- and hypomethylated CpG-sites as well as different peak densities. Notably, the sample collected at 6 months (after IGRA conversion) displayed a similar DNA methylation profile as the sample collected at inclusion. The results coincided in both the AMs and the alveolar T cells. In a subset of three donors transcriptome data was obtained and we extracted the individual expression counts from the transcriptome data using the 1,186 identified CpG-sites. The spearman’s rank analysis revealed negative correlations between the methylation and transcriptome data for each individual implying the hypermethylated genes are down-regulated and vice versa (Figure S1 a,b). To further explore the data, we performed a principal component analysis (PCA) which revealed a distinct group formation from the IGRA converters (based on the PC1 (Dim1) with 70% C.I.), including both the samples collected before and after the IGRA conversion (Fig. 2b,d). The borderline IGRApos individual on the other hand clustered with the data obtained from IGRAneg study subjects. A variance decomposition analysis between the principal components and known possible confounding variables including age, BMI, sample collection timepoint, gender, batch and IGRA status showed that in the first PCs IGRA status and BMI explained a substantial portion of the variance in both cell types (Figure S2a,b), no significant difference in the BMI of the groups was measured with a Mann–Whitney U test (Figure S2c). We proceeded with a hierarchical clustering analysis by applying the Euclidean similarity/dissimilarity matrix calculation using the M-value (Fig. 3a,b). In line with the PCA results, the data from the IGRA converters formed a separate cluster, including both the sample collected before and after IGRA conversion. The same cluster separation was found in both the samples from the AMs and from the alveolar T cells. To visualize the methylation status in the 1186 and 404 CpG-sites from the AMs and alveolar T cells respectively, we created heatmaps (Fig. 4a,b), demonstrating a clear difference in the overall methylation between IGRA converters and IGRAneg study subjects.
Unique distribution of genomic DNA methylation discriminates IGRA-converting individuals. Density plots of the distribution of M-values from CpG-sites identified in (a) alveolar macrophages (AMs) and (c) alveolar T cells. Full line represents samples collected at 0 months and dotted lines samples collected at 6 months. The IGRA status is explained by the color of the line, negative (green), converter (orange) and positive (pink). The Principal Component Analysis (PCA) of the methylation data from (b) AMs and (d) alveolar T cells. The timepoint of sample collection is explained by shape, triangle for 0 months and dot for 6 months, the IGRA-status is explained by color. The ellipses represent the 70% confidence interval (CI) in the dataset. AMs (n = 19), alveolar T cells (n = 19).
Hierarchical clustering analysis separating IGRA-converting individuals. Unsupervised hierarchical clustering dendrogram applying the Euclidian distance matrix calculation and Ward D2 method. The dendrograms show the clustering of methylome data from (a) alveolar macrophages (AMs). and (b) alveolar T cells. The scale represents the Euclidean distance. AMs (n = 19), alveolar T cells (n = 19).
Heatmaps reveal a different overall DNA methylation pattern in IGRA-converting study subjects. (a) Heatmap of all CpG-sites (1186) identified in the alveolar macrophages (AMs) of each study subject. (b) Heatmap of all CpG-sites (404) identified from the alveolar T cells. The color bar represents the M-value scale ranging from − 10 (blue) to 10 (red). AMs (n = 19), alveolar T cells (n = 19).
High number of strong DMCs identified in IGRA-converting individuals
To further explore the epigenetic reprogramming that occurred between the two time points, 0 and 6 months, we identified the differentially methylated CpG-sites (DMCs) with the strict filtering criteria (|log2 Fold Change (log2FC)|> 5 and Benjamini-Hochberg (BH) adjusted p value < 0.01) in each study subject. The DMCs were divided into three different cutoff levels: |log2FC| 10, 13 and 15 hyper- or hypomethylation in order to understand the distribution of DMCs based on the level of change. The number of DMCs in the different cutoff levels for each study subject is presented in Fig. 5a (AMs) and 5b (T cells). A high number of DMCs with a |log2FC|> 15 was identified in the IGRA converters in both cell types. To normalize the results to account for the different number of total DMCs identified (|log2FC|> 5, BH adj. p value < 0.01) we looked at the percentage of DMCs with a |log2FC|> 15. The percentage of DMCs with a |log2FC|> 15 for each cell type and study participant is shown in Fig. 5c.
Profound DNA methylome alterations identified in IGRA-converting study subjects. Lollipop plots showing the number of differentially methylated CpG-sites (DMCs) identified in the (a) alveolar macrophages (AMs) and (b) alveolar T cells of each study subject at (|log2FC| cutoff at > 10, 13 and 15. (c) The percentage of DMCs with a |log2FC|> 15 compared to the total number of DMCs (|log2FC|> 5, BH adj p value > 0.01) identified in each study subjects’ AMs and alveolar T cells.
IGRA-converting individuals undergo similar epigenetic changes in DNA methylation
The DMCs were annotated to the official (HUGO Gene Nomenclature Committee approved) Gene Symbols for further analysis and is referred to as DMGs in the following text. To identify the overlap in the DMGs with a |log2FC|> 15 between the study subjects, a Venn analysis was performed and presented in UpSet plots. The IGRA converters shared the largest intersection in both cell types, 452 DMGs in the AMs (Fig. 6) and 471 DMGs in the alveolar T cells (Supplementary Figure S3). 32 of these DMGs were overlapping between the cell types. To filter out unspecific changes we selected the DMGs that changed in the same direction, hyper- or hypomethylated, in both IGRA converters. We identified 280 (128 DMGs were hypermethylated and 152 hypomethylated) and 281 (159 DMGs were hypermethylated and 122 hypomethylated) DMGs that became hypo- or hypermethylated, in both IGRA converters in the AMs and in the alveolar T cells, respectively.
IGRA converters’ DMGs are over-represented in pathways related to metabolic reprogramming, T cell migration and IFN-γ production
In an over-representation analysis (ORA)23 using the PANTHER database24, the identified DMGs from the AMs unique to the IGRA converters were shown to be over-represented in the pentose phosphate pathway and the Ras pathway (Table 2). In an ORA of the DMGs from the alveolar T cells unique to the IGRA converters we identified enrichment in the muscarinic acetylcholine receptors 1–4 pathway and β1- and β2 adrenergic receptor signaling pathway (Table 3).
Discussion
In this study we investigated the genome-wide DNA methylation in the pulmonary immune cells in individuals at high risk of exposure to M. tuberculosis. DNA methylation has been shown to play an important role in the innate immune systems response to mycobacteria by us and others15,25,26,27,28, however, it has not yet been longitudinally studied in healthy individuals at risk of TB exposure. Two of the study subjects developed latent TB infection during the course of sample collection as demonstrated by IGRA conversion and our analyses identified a distinct genome-wide DNA methylation profile separating these two individuals from those who remained IGRAneg throughout the study. This DNA methylation profile, which, in contrary to our expectation, was present already at inclusion, was characterized by more hypo- and hypermethylated CpG-sites. From these observations, we propose the following hypotheses: (i) the IGRA converters were exposed to TB via the airways already before inclusion and the exposure had not yet developed into circulating T cell memory (resulting in a negative IGRA test) but had caused reprogramming of the DNA methylome of AMs; (ii) a different, inherent genomic DNA methylation profile as observed in the IGRA converters predispose for IGRA conversion in a high-endemic setting.
According the first hypothesis, the observed DNA methylation pattern represents a normal, protective response to TB exposure (as part of the first ‘checkpoint’), which however was breached by the infection and therefore urged the host to induce an adaptive immune response (the second ‘checkpoint’)2,3. If the second hypothesis applies, a limited capacity for inducing a host-protective epigenetic reprogramming could cause susceptibility and predisposition for developing latent TB infection. There is a large heterogeneity in the human susceptibility to develop latent or active TB upon exposure and there is limited knowledge in the determining factors29, but larger studies could investigate the correlation between DNA methylation patterns and TB susceptibility. It is also possible that the distinct DNA methylation pattern observed could be affected by M. tuberculosis manipulating the host cells epigenome26,30,31.
The two IGRA converters underwent similar epigenetic changes between the timepoints during conversion to IGRA positivity. There was a large overlap in DMGs in the IGRA converters in both cell types. The DMG intersect unique to the IGRA converters AMs showed over-representation in the pentose phosphate pathway which has previously been described to be critical in M. tuberculosis infected macrophages32,33. Infected macrophages become dependent on both glycolysis and the pentose phosphate pathway to fulfill the metabolic and bioenergetic requirements for production of cytokines, chemokines and reactive oxygen species (ROS)32,33,34. The DMGs unique to the IGRA converters’ alveolar T cells showed over-representation in the pathway for β-adrenergic and muscarinic acetylcholine receptor signaling. A functional role between β2 adrenergic receptor (β2AR) activation and IFN-γ and Tumor necrosis factor (TNF) production in T helper 1 (Th1) cells has been established in several studies, activation of β2AR by the neurotransmitter norepinephrine (NE) decreases the IFN-γ expression in Th1 cells35,36,37. However, in murine models, NE has been shown to induce rapid differentiation of naïve T cells into Th1 cells producing high levels of IFN-γ which have been correlated to early TB infection38,39. Polymorphisms in the β2AR have also been reported to be associated with TB40. The cholinergic system also has a functional role in the T cells. T cells express both muscarinic and nicotinic acetylcholine (ACh) receptors and expression of the enzyme choline acetyltransferase (ChAT) is induced in T cells during infection, T cell derived ACh is involved in T cells migration to tissues41,42,43.
One study subject was borderline IGRA positive at inclusion and this subject followed the same DNA methylation distribution and was clustering with the IGRAneg study subjects. The kinetics of the IFN-γ-reaction in relation to point of infection is not elucidated and after TB treatment the peripheral T cell memory will persist for up to 15 months44,45. According to our analysis, the epigenome of the pulmonary immune cells in this individual was more similar to that of healthy individuals, possibly indicating a historical exposure to TB and that the epigenome profile has returned to baseline.
We identify different clinical implications of the findings presented here. If the DNA methylation profile observed in the IGRA converters is an early result of M. tuberculosis infection, this could have implications as a diagnostic tool for identification of individuals that are developing latent TB infection before any of the currently available diagnostic methods. However, if the pattern we identified is not a result of bacterial infection but rather an epigenomic dysregulation predisposing individuals to convert upon exposure, this global DNA methylation pattern could have implications in identifying risk-groups who are more prone to convert upon exposure.
We recognize that this study was performed on a small number of study subjects and further investigation in larger cohorts is needed to elucidate the results presented here. We also acknowledge the limitations of the study design, we included a cohort of study subjects with risk of M. tuberculosis exposure, but no definite measurement of exposure. The observations made with IGRA converters were limited to two study subjects. However, we recently published a study that supports the finding of a differential DNA methylation pattern that can distinguish individuals with latent TB from IGRA-negative individuals in a similar high endemic setting in Lima, Peru46 (MedRxiv pre-print, 2021). We also recently showed that M. tuberculosis exposure-induced epigenetic reprogrammings are more profound in pulmonary immune cells as compared to PBMCs47 (MedRxive pre-print 2021). Here, we highlight the significance of investigating pulmonary immune cells, and the value of investigating DNA methylomes in the same study subjects, longitudinally, before and after IGRA conversion.
Methods
Ethical statement
Ethical approval was obtained from Universidad Peruana Cayetano Heredia (UPCH) Institutional Review Board (IRB), No. 103793. The sputum sample collections were performed in accordance with guidelines from the Department of Respiratory Medicine at Linköping University Hospital. The IGRA samples (QuantiFERON® TB-Gold Plus test) were collected and analyzed by medical personnel according to the guidelines at the Instituto de Medicina Tropical Alexander von Humboldt, Universidad Peruana Cayetano Heredia. All participants signed an informed consent.
Study cohort and design
This was a prospective study aimed to investigate epigenetic DNA methylome alterations in pulmonary immune cells pre- and post-M. tuberculosis exposure in a natural setting. We enrolled medical students (n = 15) in the fifth or sixth year of medical school at UPCH. The students donated sputum and blood samples before (0 months) and after (6 months) they had clinical rotations in high-risk departments of M. tuberculosis exposure. The department of infectious disease and internal medicine including the emergency department were defined as departments with high-risk of M. tuberculosis exposure. Sputum was used to isolate immune cells from the lung and the blood was used for IGRA. At inclusion, the participants filled in an individual case report form with demographic information. Participants also filled in an online questionnaire to collect background information before each session at 0 and 6 months. The questionnaire was created in the tool Survey & Report, provided by Linköping University. Samples from 10 individuals (collected at 0 and 6 months for IGRAneg (n = 7) and IGRA converters (n = 2) and at 0 months for IGRApos (n = 1)) resulted in 19 samples that were selected for each cell type (AMs and alveolar T cells), for the sequencing analysis. The sample selection was based on results in the online questionnaire and on the sample quality with regards to the DNA concentration obtained in the AMs and alveolar T cells at the two sample collections at 0 and 6 months.
Interferon gamma releasing assay with QuantiFERON® TB-Gold Plus
At inclusion and follow-up, the participants donated blood samples that were used for IGRA with the QuantiFERON® TB-Gold Plus test (SSI Diagnostica, Hillerød, Denmark) according to the manufacturer’s instructions. Tubes were filled and incubated at 37 °C for maximum 24 h and then analyzed with ELISA. The test results are presented in Supplementary Table S1 as the quantification of interferon-γ in international units per ml (IU/ml). There is a dichotomous cut-off (0.35 IU/ml) that defines a positive result, but a borderline range (0.20–0.99 IU/ml) exists22.
Sputum induction
The sputum induction21 was performed with an eFlow rapid nebulizer (PARI, Hamburg, Germany) filled with a hypertonic saline solution. The solution was prepared by mixing sterile water (Fresenius Kabi, Stockholm, Sweden) with 4% sodium chloride (B. Braun Medical AB, Stockholm, Sweden). The participants inhaled the solution for 9 min while simultaneously preforming breathing exercises in accordance with instructions from the lung clinic at Linköping University Hospital. The participants were asked to cough deeply to expectorate sputum from the lungs. The expectorates were collected into sterile 50 ml Falcon tubes (Thermo Fisher Scientific, Waltham, US) after each session. The Falcon tubes were kept on ice. The sputum inductions were performed in three replicates.
Sputum processing and CD3 and HLA-DR positive cell isolation
From the sputum samples, within 2 h, plugs containing pulmonary immune cells21 were picked and pooled. The plugs were dissolved by adding (0.1%) dithiothreitol (DTT) (Thermo Fisher Scientific) mixed in phosphate-buffered saline (PBS) (Gibco, Cambridge, UK). This was added in a volume approximately 4 times the collected sputum volume and then vortexed and placed on a tilter with ice for 20 min. The dissolved sputum sample was then filtered through 50 μm cell strainers (Sigma-Aldrich, Saint Louise, US) into a new 50 ml Falcon tube and centrifuged for 5 min at 380 g in 4 °C. The supernatant was discarded, and the pellet was resuspended in (500 µl) of an isolation buffer containing (500 mM) ethylenediaminetetraacetic acid (EDTA), (0.1%) fetal bovine serum (FBS), Ca2+ and Mg2+ free PBS (pH 7.4). From the cell pellet we did sequential positive isolation using antibody conjugated magnetic beads. First, we isolated CD3 positive cells to ensure that all T cells were isolated from the cell suspension. Secondly, from the residual cell pellet we isolated HLA-DR positive cells. We first isolate CD3 positive cells to avoid isolating HLA-DR expressing T cells during the second positive isolation. CD3 Dynabeads (25 µl) (Invitrogen Dynabeads®, Life Technologies AS, Oslo, Norway) were washed with (800 µl) PBS and placed in a DynaMag-2 (Thermo Fisher Scientific) for 1 min. The supernatant was discarded, and isolation buffer (800 µl) was added two times for additional washing. The beads were then mixed with the cell sample (500 µl) and incubated for 30 min at 4 °C while tilting. After incubation, the tube was placed in the DynaMag-2, supernatant was removed to be used to isolate HLA-DR positive cells next. The CD3 positive cells were resuspended in (200 µl) PBS.
Secondly, HLA-DR positive cells were isolated. Magnetic Pan Mouse IgG Dynabeads™ (25 µl) (cat no: 11041, Thermo Fisher) were washed with (800 µl) PBS and placed in a DynaMag-2 (Thermo Fisher Scientific) for 1 min. The supernatant was discarded and (800 µl) isolation buffer was added two times for additional washing. Monoclonal HLA-DR antibodies (5 µl) (cat no: 14–9956-82, Thermo Fisher) were added to conjugate the Dynabeads. Tubes were incubated for 40 min, then placed in the DynaMag-2 for 30 s and supernatant removed. (800 µl) isolation buffer was added two times for washing. The tube was placed in DynaMag-2 for 30 s and supernatant removed. The beads were then mixed with the supernatant from the CD3 isolation (500 µl) and incubated for 30 min at 4 °C while tilting. After incubation, the tube was placed in the DynaMag-2, supernatant was discarded, and HLA-DR positive cells were resuspended in PBS (200 µl). CD3 positive cells are referred to as alveolar T cells and HLA-DR positive cells are referred to as alveolar macrophages (AMs).
DNA and RNA extraction and quantification
DNA and RNA was extracted from the AMs and alveolar T cells with the AllPrep® DNA/RNA Mini Kit (Qiagen, Hilden, Germany) within 4 h from cell isolation. Concentration of DNA and RNA was quantified with a Qubit® 4.0 Fluorometer (Thermo Fisher Scientific), using dsDNA High Sensitivity (HS) Assay Kit or RNA HS Assay kit (Thermo Fisher Scientific). The measurement was performed according to the manufacturer's instructions.
Reduced representation bisulfite sequencing of DNA from AMs and alveolar T cells
DNA samples were sequenced with Reduced Representation Bisulfide Sequencing (RRBS) at the Bioinformatics and Expression Analysis (BEA) core facility at Karolinska Institute (KI) with Diagenode’s RRBS. The DNA was enzymatically digested, bisulfite-converted, and PCR amplified before ready for Illumina’s HiSeq 2000. The use of the restriction enzyme MspI, which cleaves CCGG from the 5′end, results in shorter sequences to analyze and is therefore cost-effective.
Transcriptome sequencing of total RNA from AMs
The library was prepared using the SMARTer® Stranded Total RNA Sample Prep Kit—HI Mammalian (Takara Bio Inc, Japan) as per manufacturer’s instructions. The ribosomal RNA was removed, and the remaining RNA was reversely transcribed to cDNA. The cDNA was amplified with specific reverse index primers (Corresponding to TruSeq HT i7 index D701-D712) and forward the index primer (Corresponding to TruSeq HT i5 index primer D502). The concentration of each library was validated with Qubit and RNA HS Assay kit (Thermo Fisher Scientific). One µl of each library were used to analyze average library length with the Agilent 2100 Bioanalyzer and the High sensitivity DNA Chip (Agilent Technologies, USA). 0.5 nM of each library were pooled. The RNA concentrations in nanomolar were calculated using the following equation:
A total of 6 samples were sequenced (three samples from the first inclusion and three samples from the second inclusion) and we added a 10% PhiX control in the Illumina NextSeq 550 sequencer (Illumina, US) using the mid output kit (v2.5, 150 cycles) (Illumina). The PhiX control library consists of a characterized bacteriophage genome with a balanced GC (45%) and AT (55%) content. PhiX is used to examine the overall performance of the sequencing. The sequencing was run with a single index, paired-end protocol, both read lengths set to 76 bp according to the manufacturer’s protocol. The sequence result was saved in FASTQ file format.
Transcriptome data processing and computational analysis
The data quality was checked with FastQC algorithm and showed a phred quality score ≥ 33 in all samples. The raw reads from FASTQ format files were aligned to the Genome Reference Consortium Human Build 38 patch release 13 (GRCh38.p13) using Rsubread (version 3.10) we used the first filtration criteria to set the read length of fragments from 50 to 700 bp to remove unwanted binding sequences. Second filtration was applied to remove the X and Y chromosomes to reduce gender biasness.
DNA methylation data processing and computational analysis
The RAW files (in fastq format) generated from the RRBS analysis, were quality checked using the fastQC48 (v0.11.9). The sequences were trimmed to remove artificially filled-in cytosines at the 3′ end using the TrimGalore (v.0.6.5)49 with a phred score cutoff of 20 and quality checked again after trimming. The trimmed sequences were aligned with the human reference genome (hg38.13) using Bowtie250 and removed the duplicates using the Bismark v.0.22.351. The methylation extractor from Bismark was used to extract the CpG methylation data from the sequences. The SAMtools (v1.7)52 package was used to sort the bam files on CpG-site chromosomal location and converted to SAM files. The methylated and unmethylated CpG counts were extracted and combined using the DMRfinder (v0.3)53 package in R (v4.0.2)54.
To read the Bismark coverage files, the edgeR (v3.32.1)55,56 package was used. The CpG-sites located in the X and Y chromosome as well as CpG-sites from mitochondrial DNA were filtered out. CpG-sites with a read coverage < 5 with both methylated and unmethylated reads were removed from the analysis. The M-values were calculated using the log2 ratio of the intensities of methylated verses unmethylated CpG-sites, Eq. (1) (with addition of + 2 to each count to avoid logarithms of zeros)57.
The CpG-sites were annotated using org.Hs.eg.db (v3.12)58 and AnnotationDbi (v1.52)59 packages using human genome hg38. After filtering and annotating the data, we identified a total of 1,186 CpG-sites in the AMs and 404 CpG-sites from alveolar T cells that were covered in all samples.
Statistical analysis
The Principal Component Analysis (PCA) was calculated using the factoExtra (v1.0.7)60 and factoMineR (v2.4)61. To examine the correlation of DNA methylation and regulation of gene expression, the Spearman’s rank correlation coefficient was calculated between the scaled M-values62 and normalized expression values for the 1,186 CpG-sites and corresponding genes using ggcorr function in GGally package in R63. Heatmaps were generated using ComplexHeatmap package (v 4.0.3). To determine which confounding variables or known sources of variation explain the total variance of the data set, we applied principal component analysis (PCA) and calculated the correlation (R2) of PCs of the independent variables (IGRA status, gender, age, BMI, batch and timepoint of sample collection). The difference of the variable BMI between the groups was calculated using Mann–Whitney U test in GraphPad Prism 9. The hierarchical clusters was estimated using the ape (v5.4-1)64 and dendextend (v1.14.0)65 packages by calculating the Euclidian similarity/dissimilarity matrix. For the identification of the individual differentially methylated CpG-sites (DMCs) the EdgeR lmFit function66 was used. Identifying differentially methylated genes in the same study subject longitudinally instead of comparing groups reduces the risk of confounding and is suitable with a small sample size. The counts of the unmethylated (Un) and methylated (Me) reads in the conditions 0 months (A) and 6 months (B) in each individual were used to calculate the Fold Change, Eq. (2). The dispersion parameter controlling the degree of biological variability was set to 0.0247.
DMCs were defined as CpG-sites with a |log2FC|> 5 and significant with the BH adjusted p value < 0.01. The lollipop plots and the upset plots were made using the ggpubr (v0.4.0)67 and the UpSetR (v1.4.0)68,69 packages. Two IGRAneg study subjects and 1 study subject had no DMCs with a |log2FC|> 15 in the AMs and alveolar T cells respectively and were therefore excluded from the analysis. The pathway analysis was performed with the DMGs with a |log2FC|> 15 and BH adjusted p value < 0.01 unique to the IGRA converters AMs and alveolar T cells respectively from the PANTHER database (v16.0)24 using the WEB-based Gene SeT AnaLysis Toolkit (WebGestalt) webserver (v2019)23. The false discovery rate (FDR) in the pathway analysis is BH adjusted p values. The significant pathways identified in the AMs are presented in Table 2 and the top 4 most significant pathways identified in the alveolar T cells are presented in Table 3.
Data availability
The datasets will be made available at the final publication.
References
World Health Organization. Global Tuberculosis Report. https://apps.who.int/iris/bitstream/handle/10665/329368/9789241565714-eng.pdf (2019).
Schön, T., Lerm, M. & Stendahl, O. Shortening the ‘short-course’ therapy-insights into host immunity may contribute to new treatment strategies for tuberculosis. J. Intern. Med. 273, 368–382 (2013).
Lerm, M. & Netea, M. G. Trained immunity: A new avenue for tuberculosis vaccine development. J. Intern. Med. https://doi.org/10.1111/joim.12449 (2016).
Verrall, A. J. et al. Early clearance of Mycobacterium tuberculosis is associated with increased innate immune responses. J. Infect. Dis. https://doi.org/10.1093/infdis/jiz147 (2019).
Verrall, A. J. et al. Early clearance of mycobacterium tuberculosis: the INFECT case contact cohort study in Indonesia. J. Infect. Dis. https://doi.org/10.1093/infdis/jiz168 (2019).
Verrall, A. J. G., Netea, M., Alisjahbana, B., Hill, P. C. & van Crevel, R. Early clearance of Mycobacterium tuberculosis: A new frontier in prevention. Immunology https://doi.org/10.1111/imm.12223 (2014).
O’Garra, A. et al. The immune response in tuberculosis. Annu. Rev. Immunol. https://doi.org/10.1146/annurev-immunol-032712-095939 (2013).
Cooper, A. M. Cell-mediated immune responses in tuberculosis. Annu. Rev. Immunol. 27, 393–422 (2009).
Lerner, T. R., Borel, S. & Gutierrez, M. G. The innate immune response in human tuberculosis. Cell. Microbiol. https://doi.org/10.1111/cmi.12480 (2015).
Pieters, J. Mycobacterium tuberculosis and the Macrophage: Maintaining a Balance. Cell Host Microbe 3, 399–407 (2008).
Pai, M. et al. Gamma interferon release assays for detection of Mycobacterium tuberculosis infection. Clin. Microbiol. Rev. https://doi.org/10.1128/CMR.00034-13 (2014).
Netea, M. G. et al. Trained immunity: A program of innate immune memory in health and disease. Science https://doi.org/10.1126/science.aaf1098 (2016).
Seeley, J. J. et al. Induction of innate immune memory via microRNA targeting of chromatin remodelling factors. Nature https://doi.org/10.1038/s41586-018-0253-5 (2018).
Villota-Salazar, N. A., Mendoza-Mendoza, A. & González-Prieto, J. M. Epigenetics: from the past to the present. Front. Life Sci. https://doi.org/10.1080/21553769.2016.1249033 (2016).
Verma, D. et al. Anti-mycobacterial activity correlates with altered DNA methylation pattern in immune cells from BCG-vaccinated subjects. Sci. Rep. 7, 1–10 (2017).
Hasso-Agopsowicz, M., Scriba, T. J., Hanekom, W. A., Dockrell, H. M. & Smith, S. G. Differential DNA methylation of potassium channel KCa3.1 and immune signalling pathways is associated with infant immune responses following BCG vaccination. Sci. Rep. https://doi.org/10.1038/s41598-018-31537-9 (2018).
Das, J., Verma, D., Gustafsson, M. & Lerm, M. Identification of DNA methylation patterns predisposing for an efficient response to BCG vaccination in healthy BCG-naïve subjects. Epigenetics 14, 589–601 (2019).
Yona, S., Viukov, S., Guilliams, M. & Misharin, A. Tissue macrophages under homeostasis. Immunity 38, 79–91 (2013).
Yao, Y. et al. Induction of autonomous memory alveolar macrophages requires T cell help and is critical to trained immunity. Cell https://doi.org/10.1016/j.cell.2018.09.042 (2018).
Pérez-Lu, J. E., Cárcamo, C. P., García, P. J., Bussalleu, A. & Bernabé-Ortiz, A. Tuberculin skin test conversion among health sciences students: A retrospective cohort study. Tuberculosis https://doi.org/10.1016/j.tube.2012.10.001 (2013).
Das, J., Idh, N., Sikkeland, L. I. B., Paues, J. & Lerm, M. DNA methylome-based validation of induced sputum as an effective protocol to study lung immunity: construction of a classifier of pulmonary cell types. Epigenetics. https://doi.org/10.1080/15592294.2021.1969499 (2021).
Jonsson, J. et al. A borderline range for quantiferon gold in-tube results. PLoS ONE https://doi.org/10.1371/journal.pone.0187313 (2017).
Liao, Y., Wang, J., Jaehnig, E. J., Shi, Z. & Zhang, B. WebGestalt 2019: gene set analysis toolkit with revamped UIs and APIs. Nucleic Acids Res. https://doi.org/10.1093/nar/gkz401 (2019).
Thomas, P. D. et al. PANTHER: A library of protein families and subfamilies indexed by function. Genome Res. https://doi.org/10.1101/gr.772403 (2003).
Wei, M. et al. NLRP3 activation was regulated by DNA methylation modification during mycobacterium tuberculosis infection. Biomed Res. Int. https://doi.org/10.1155/2016/4323281 (2016).
Sharma, G. et al. Genome-wide non-CpG methylation of the host genome during M. tuberculosis infection. Sci. Rep. https://doi.org/10.1038/srep25006 (2016).
Zheng, L. et al. Unraveling methylation changes of host macrophages in Mycobacterium tuberculosis infection. Tuberculosis https://doi.org/10.1016/j.tube.2016.03.003 (2016).
DiNardo, A. R. et al. DNA hypermethylation during tuberculosis dampens host immune responsiveness. J. Clin. Invest. https://doi.org/10.1172/JCI134622 (2020).
Cadena, A. M., Fortune, S. M. & Flynn, J. L. Heterogeneity in tuberculosis. Nat. Rev. Immunol. https://doi.org/10.1038/nri.2017.69 (2017).
Sharma, G., Upadhyay, S., Srilalitha, M., Nandicoori, V. K. & Khosla, S. The interaction of mycobacterial protein Rv2966c with host chromatin is mediated through non-CpG methylation and histone H3/H4 binding. Nucleic Acids Res. https://doi.org/10.1093/nar/gkv261 (2015).
Yaseen, I., Kaur, P., Nandicoori, V. K. & Khosla, S. Mycobacteria modulate host epigenetic machinery by Rv1988 methylation of a non-tail arginine of histone H3. Nat. Commun. https://doi.org/10.1038/ncomms9922 (2015).
Kumar, R. et al. Immunometabolism of phagocytes during mycobacterium tuberculosis infection. Front. Mol. Biosci. https://doi.org/10.3389/fmolb.2019.00105 (2019).
Cumming, B. M., Addicott, K. W., Adamson, J. H. & Steyn, A. J. C. Mycobacterium tuberculosis induces decelerated bioenergetic metabolism in human macrophages. Elife https://doi.org/10.7554/eLife.39169 (2018).
Giri, P. K., Kruh, N. A., Dobos, K. M. & Schorey, J. S. Proteomic analysis identifies highly antigenic proteins in exosomes from M. tuberculosis-infected and culture filtrate protein-treated macrophages. Proteomics https://doi.org/10.1002/pmic.200900840 (2010).
Sanders, V. M. et al. Differential expression of the beta2-adrenergic receptor by Th1 and Th2 clones: implications for cytokine production and B cell help. J. Immunol. 158, 4200–4210 (1997).
Borger, P. et al. β-Adrenoceptor-mediated inhibition of IFN-γ, IL-3, and GM-CSF mRNA accumulation in activated human T lymphocytes is solely mediated by the β2-Adrenoceptor subtype. Am. J. Respir. Cell Mol. Biol. https://doi.org/10.1165/ajrcmb.19.3.2765 (1998).
Estrada, L. D., Ağaç, D. & Farrar, J. D. Sympathetic neural signaling via the β2-adrenergic receptor suppresses T-cell receptor-mediated human and mouse CD8+ T-cell effector function. Eur. J. Immunol. https://doi.org/10.1002/eji.201646395 (2016).
Barrios-Payán, J. et al. The contribution of the sympathetic nervous system to the immunopathology of experimental pulmonary tuberculosis. J. Neuroimmunol. https://doi.org/10.1016/j.jneuroim.2016.07.012 (2016).
Swanson, M. A., Lee, W. T. & Sanders, V. M. IFN-γ production by Th1 cells generated from naive CD4 + T cells exposed to norepinephrine. J. Immunol. https://doi.org/10.4049/jimmunol.166.1.232 (2001).
Azar, A. F., Jazani, N. H., Bazmani, A., Vahhabi, A. & Shahabi, S. Polymorphisms in Beta-2 adrenergic receptor gene and association with tuberculosis. Lung https://doi.org/10.1007/s00408-016-9968-y (2017).
Fujii, T. et al. Expression and function of the cholinergic system in immune cells. Front. Immunol. https://doi.org/10.3389/fimmu.2017.01085 (2017).
Murray, K. et al. Neuroanatomy of the spleen: Mapping the relationship between sympathetic neurons and lymphocytes. PLoS ONE https://doi.org/10.1371/journal.pone.0182416 (2017).
Cox, M. A. et al. Choline acetyltransferase–expressing T cells are required to control chronic viral infection. Science https://doi.org/10.1126/science.aau9072 (2019).
Pollock, N. R. et al. Evaluation of the effect of treatment of latent tuberculosis infection on QuantiFERON-TB gold assay results. Infect. Control Hosp. Epidemiol. https://doi.org/10.1086/596606 (2009).
Dyrhol-Riise, A. M. et al. Diagnosis and follow-up of treatment of latent tuberculosis; the utility of the QuantiFERON-TB Gold In-tube assay in outpatients from a tuberculosis low-endemic country. BMC Infect. Dis. https://doi.org/10.1186/1471-2334-10-57 (2010).
Pehrson, I. et al. DNA methylomes derived from alveolar macrophages display distinct patterns in latent tuberculosis—implication for interferon gamma release assay status determination. MedRxiv https://doi.org/10.1101/2021.03.16.21253725 (2021).
Das, J., Idh, N., Pehrson, I. & Paues, J. A DNA methylome biosignature in alveolar macrophages from TB-exposed individuals predicts exposure to mycobacteria. medrxiv https://doi.org/10.1101/2021.03.16.21253732 (2021).
Andrews, S. FASTQC A Quality Control tool for High Throughput Sequence Data. Babraham Inst. (2015).
Krueger, F. Trim Galore: a wrapper tool around gutadapt and FastQC to consistently apply quality and adapter trimming to FastQ files. https://www.bioinformatics.babraham.ac.uk/projects. Babraham Institute (2015).
Langmead, B. & Salzberg, S. L. Fast gapped-read alignment with Bowtie 2. Nat. Methods https://doi.org/10.1038/nmeth.1923 (2012).
Krueger, F. & Andrews, S. R. Bismark: A flexible aligner and methylation caller for Bisulfite-Seq applications. Bioinformatics https://doi.org/10.1093/bioinformatics/btr167 (2011).
Li, H. et al. The sequence alignment/map format and SAMtools. Bioinformatics https://doi.org/10.1093/bioinformatics/btp352 (2009).
Gaspar, J. M. & Hart, R. P. DMRfinder: Efficiently identifying differentially methylated regions from MethylC-seq data. BMC Bioinform. https://doi.org/10.1186/s12859-017-1909-0 (2017).
Rstudio, T. RStudio: Integrated Development for R. Rstudio Team, PBC, Boston, MA. http://www.rstudio.com/ (2020). https://doi.org/10.1145/3132847.3132886.
Robinson, M. D., McCarthy, D. J. & Smyth, G. K. edgeR: A Bioconductor package for differential expression analysis of digital gene expression data. Bioinformatics https://doi.org/10.1093/bioinformatics/btp616 (2009).
McCarthy, D. J., Chen, Y. & Smyth, G. K. Differential expression analysis of multifactor RNA-Seq experiments with respect to biological variation. Nucleic Acids Res. https://doi.org/10.1093/nar/gks042 (2012).
Du, P. et al. Comparison of Beta-value and M-value methods for quantifying methylation levels by microarray analysis. BMC Bioinform. https://doi.org/10.1186/1471-2105-11-587 (2010).
Marc Carlson. org.Mm.eg.db: Genome wide annotation for Mouse. R package version 3.8.2. Bioconductor (2019).
Pagès, H., Carlson, M., Falcon, S. & Maintainer, N. L. Package ‘AnnotationDbi’. Bioconductor Packag. Maint. (2017).
Kassambara, A. & Mundt, F. factoextra: Extract and Visualize the Results of Multivariate Data Analyses. Package Version 1.0.7. R Packag. version (2020).
Lê, S., Josse, J. & Husson, F. FactoMineR: An R package for multivariate analysis. J. Stat. Softw. https://doi.org/10.18637/jss.v025.i01 (2008).
Kvist, J. et al. A comprehensive epigenomic analysis of phenotypically distinguishable, genetically identical female and male Daphnia pulex. BMC Genom. https://doi.org/10.1186/s12864-019-6415-5 (2020).
Rodríguez-Tomàs, E. et al. Identification of potential metabolic biomarkers of rectal cancer and of the effect of neoadjuvant radiochemotherapy. PLoS ONE https://doi.org/10.1371/journal.pone.0250453 (2021).
Paradis, E. & Schliep, K. Ape 5.0: An environment for modern phylogenetics and evolutionary analyses in R. Bioinformatics https://doi.org/10.1093/bioinformatics/bty633 (2019).
Galili, T. dendextend: An R package for visualizing, adjusting and comparing trees of hierarchical clustering. Bioinformatics https://doi.org/10.1093/bioinformatics/btv428 (2015).
Smyth, G. K., Chen, Y., Pal, B. & Visvader, J. E. Differential methylation analysis of reduced representation bisulfite sequencing experiments using edgeR. F1000Research https://doi.org/10.12688/f1000research.13196.1 (2017).
Kassambara, A. Package ‘ggpubr’: ‘ggplot2’ Based Publication Ready Plots. R Packag. version 0.4.0 (2020).
Lex, A., Gehlenborg, N., Strobelt, H., Vuillemot, R. & Pfister, H. UpSet: Visualization of intersecting sets. IEEE Trans. Vis. Comput. Graph. https://doi.org/10.1109/TVCG.2014.2346248 (2014).
Conway, J. R., Lex, A. & Gehlenborg, N. UpSetR: An R package for the visualization of intersecting sets and their properties. Bioinformatics https://doi.org/10.1093/bioinformatics/btx364 (2017).
Acknowledgements
We would like to show our appreciation to the medical personnel at Instituto de Medicina Tropical Alexander von Humboldt, Universidad Peruana Cayetano Heredia, for assistance in sample collections and analysis of QuantiFERON® TB-Gold Plus test. We thank the Bioinformatics and Expression Analysis core facility (BEA) at Karolinska Institute that preformed the sequencing analysis. We thank the Swedish National Infrastructure for Computing (SNIC) at National Supercomputing Centre (NSC), Linköping University for the computing systems enabling the data handling, partially funded by the Swedish Research Council through grant agreement No. 2018-05973. The work was supported by grants from the Swedish Research Council No. 2018-04246 and the Consejo Nacional de Ciencia, Tecnología e Innovación Tecnológica CONCYTEC and Cienciactiva No. 106-2018-FONDECYT. J.D is a postdoctoral fellow supported through the Medical Infection and Inflammation Center (MIIC) at Linköping University.
Funding
Open access funding provided by Linköping University.
Author information
Authors and Affiliations
Contributions
N.I., J.P., I.P., C.U.-G., M.M.-A. and M.L. wrote the ethical application and conceived the study. I.P., M.N. and A.T. performed all sample collections. J.D., L.K. and S.S. performed the bioinformatic analyses. M.L., L.K., J.D. and I.P. wrote the manuscript. M.M.-A. and C.U.-G. supervised the project in Peru.
Corresponding author
Ethics declarations
Competing interests
The authors declare no competing interests.
Additional information
Publisher's note
Springer Nature remains neutral with regard to jurisdictional claims in published maps and institutional affiliations.
Rights and permissions
Open Access This article is licensed under a Creative Commons Attribution 4.0 International License, which permits use, sharing, adaptation, distribution and reproduction in any medium or format, as long as you give appropriate credit to the original author(s) and the source, provide a link to the Creative Commons licence, and indicate if changes were made. The images or other third party material in this article are included in the article's Creative Commons licence, unless indicated otherwise in a credit line to the material. If material is not included in the article's Creative Commons licence and your intended use is not permitted by statutory regulation or exceeds the permitted use, you will need to obtain permission directly from the copyright holder. To view a copy of this licence, visit http://creativecommons.org/licenses/by/4.0/.
About this article
Cite this article
Karlsson, L., Das, J., Nilsson, M. et al. A differential DNA methylome signature of pulmonary immune cells from individuals converting to latent tuberculosis infection. Sci Rep 11, 19418 (2021). https://doi.org/10.1038/s41598-021-98542-3
Received:
Accepted:
Published:
DOI: https://doi.org/10.1038/s41598-021-98542-3
Comments
By submitting a comment you agree to abide by our Terms and Community Guidelines. If you find something abusive or that does not comply with our terms or guidelines please flag it as inappropriate.