Abstract
In recent years, artificial intelligence (AI) methods have prominently proven their use in solving complex problems. Across science and engineering disciplines, the data-driven approach has become the fourth and newest paradigm. It is the burgeoning of findable, accessible, interoperable, and reusable (FAIR) data generated by the first three paradigms of experiment, theory, and simulation that has enabled the application of AI methods for the scientific discovery and engineering of compounds and materials. Here, we introduce a recipe for a data-driven strategy to speed up the virtual screening of two-dimensional (2D) materials and to accelerate the discovery of new candidates with targeted physical and chemical properties. As a proof of concept, we generate new 2D candidate materials covering an extremely large compositional space, downselect 316,505 likely stable 2D materials, and predict the key physical properties of these new 2D candidates. Finally, we hone in on the most propitious candidates of functional 2D materials for energy conversion and storage.
Similar content being viewed by others
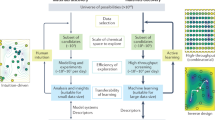
Introduction
Prior to data-driven approaches, the evolution of material discovery has experienced three paradigms1. The first paradigm is rooted in experiments performed by trial and error methods, the second paradigm utilizes the transformation of the experimental results into theory, and the third paradigm is based on the computational simulation of theoretical models. Each paradigm arose under favor of the outputs of the previous paradigms. In recent years, the rapid growth of available data generated by the previous three paradigms and the rise of highly capable algorithms have enabled the application of artificial intelligence (AI), thereby starting the fourth and latest paradigm of material discovery. The key impact of data-driven discovery is that it strikingly accelerates material discovery by substantially decreasing the required computational power, thus it removes the boundaries of the compositional screening space. AI2,3,4,5,6,7 and virtual screening8,9,10,11,12,13,14,15,16 methods have, in recent years, been successfully applied to sub-fields of chemical and material sciences to discover new chemical compounds and materials with exceptional functionalities. By having unique structural properties, every sub-field yet requires tailored procedures for virtual screening. Moreover, in order to apply AI methods in disparate material sub-fields, firstly, it is necessary to produce a sufficient amount of high fidelity and quality data from experimental or computational studies17.
One of the thriving sub-fields of materials science is two-dimensional (2D) materials. Having exceptional and tunable properties the 2D materials hold strong promise for semiconductor, energy, and health applications18,19. Since the 2010 Nobel prize-winning discovery of graphene20 with a simple 2D structure of carbon atoms but with attractive and complex physics, only a few hundred distinct 2D materials have successfully been synthesized21. Despite a modest number of experimentally realized compounds, very recently, new 2D materials repositories that are based on robust quantum simulations have been emerging14,22,23,24. The in silico repositories have been generated through the two sequential approaches of designing new 2D materials: exfoliation of layers from three-dimensional (3D) bulk structures and combinatorial exchange of atoms in 2D structures. The first approach is based on screening of exfoliated layered materials from 3D materials databases25,26,27. The outputs of this approach are new 2D materials with structures that can be used as 2D prototypes. The second approach involves the exchange of one or more atoms of a known 2D prototype with atoms of another chemical element. The outputs of the second method are new 2D materials that have the same crystal prototype as the original material but are composed of different chemical elements. Irrespective of the two approaches, the resource intensive physics-based calculations have restricted the boundaries of the chemical search space for new 2D materials, such that the number of 2D materials that could have been studied with density functional theory (DFT) calculations, the work-horse computational method28, reaches to only a few thousand during the past years. Albeit the DFT-calculated 2D materials databases consist of only a few thousand materials, they are remarkable FAIR data resources for data-driven AI methods. Among the major goals of AI, machine learning (ML) is a felicitous method that shows great promise in explorations of extremely vast search spaces of feasible chemical compounds in form of molecules, 2D, and 3D materials.
In this study, we introduce a recipe for AI-aided virtual screening of 2D materials. The recipe systematically generates candidate materials covering an unprecedented chemical space of compounds, identifies likely stable materials, and predicts key properties of the stable materials candidates. As a proof of concept, we used this recipe to generate a Virtual 2D Materials Database (V2DB) that contains 316,505 likely stable 2D materials with predicted properties. We provide a detailed description below, alongside the open-source codes and data, and expect that the recipe will be useful for future data-driven AI-aided discoveries of new and functional 2D materials.
Results
Workflow overview
We followed three steps to effectively utilize the power of AI for the large-scale virtual screening effort and to create the V2DB (Fig. 1). First, by employing 22 different 2D crystal prototypes and 52 different chemical elements from the periodic table, we applied a brute-force method to generate a systematic library of more than 72 million 2D compounds. Next, using three sequential filtering layers (i.e., symmetry, neutrality, and stability), we identified ~316 thousand (0.4% of the total) likely stable 2D materials. Finally, using artificial neural networks (ANN), we predicted their key materials properties that are relevant to energy conversion and storage.
Step 1: The generation of all possible 2D material compositions with 2D prototype structures by brute-force elemental substitution method. Step 2: The downselection of likely stable 2D materials through symmetry, neutrality, and stability filters. Step 3: The prediction of key physical properties of stable 2D materials using ML.
Generation of new 2D materials
We systematically generated 2D materials in three consecutive steps. First, we identified the base structures (i.e., prototypes) from the training data (Fig. 2a). Secondly, we grouped elements according to their assumed electrical charge states in the compounds (Fig. 2b). Thirdly, we generated all possible new 2D materials by brute-force elemental substitution (see “Methods”) using the prototype and element grouping information. A heatmap of the chemical screening space is shown in Fig. 3. As a result, we obtained 72,522,240 unverified candidate 2D materials.
Filtering
To discard geometrical duplicates, assure neutrality and stability of the newly generated compounds, we applied the following three filters (see “Methods”):
-
Symmetry filter: removes geometrical duplicate copies of compounds.
-
Neutrality filter: removes compounds with a nonzero net charge.
-
Stability filter: removes compounds that are predicted to be unstable.
After applying three layers of filters, we obtained 316,505 validated candidate 2D materials (Fig. 4). Table 1 shows the remaining number of materials after applying filters to each prototype of 2D compounds.
Property prediction
AI-aided materials design approaches that effectively utilize the complex physical and chemical knowledge on known materials hold a strong promise for new scientific discoveries that would not have been possible in other ways29,30. We developed ANN models for the prediction of important physicochemical and electronic properties, including the stability, heat of formation, energy above convex hull, nature and numerical value of band gap, valance band maximum (VBM), conduction band minimum (CBM), metal work function, and magnetic state (NM: nonmagnetic, FM: ferromagnetic, and AFM: antiferromagnetic), of filtered, likely stable 2D materials. We used a total of 2226 2D materials from the Computational 2D Materials Database (C2DB)23 to train and validate our ML models (see “Methods”). We used the property data of 2D materials as obtained by the most commonly applied theoretical method, that is DFT calculations employing the Perdew–Burke-Ernzerhof (PBE) exchange-correlation functional of the generalized gradient approximation. As inputs to our ML models, we solely used basic chemical features that require almost no computational cost. We trained different and independent ANN models for the prediction of each target property (see “Methods”). Figure 5 shows the cross-validation performance of regression and classification models that are used to predict the properties of newly generated 2D materials. The details of the cross-validation results are provided in the Supplementary Information Section 3.1.
Virtual screening
The application of AI methods to accelerate the search for suitable materials for high-tech applications is an emerging and prominent approach. An exciting arena of materials design is the development of new functional materials for energy conversion and storage. Multi-scale high-throughput computational screening studies have recently been utilized as systematic approaches31,32 in order to accelerate the discovery of new 2D energy materials for photovoltaics33,34,35 as well as photocatalytic solar fuel generation through the conversion of feedstock molecules, including H2O12,31,36,37,38, CO237,39,40, and N237. To illustrate the use of AI methods for the virtual screening of candidate 2D materials, Fig. 6 shows an assortment of 2D materials that are categorized, through a cognitive determination of their intrinsic physicochemical and electronic properties, for different energy conversion and storage applications. In Fig. 6, the AI-predicted light-absorbing 2D materials, which would convert energy from the sun into a flow of charge carriers either to directly generate electric power or to drive the chemical conversion reactions of small molecules (i.e., H2O, CO2, and N2) into useful products, are shown using different colors. To identify the best candidates for each application, we used the ML-predicted band gap, VBM, and CBM as descriptors. For instance, the 2D materials with a band gap between 0.75 and 1.75 eV are promising candidates for efficient single-junction photovoltaic cells41,42. Although some of the remainder of the semiconducting 2D materials with other band gaps should not be precluded, as they still would be interesting for multi-junction solar cells. To identify efficient 2D materials for the selective photocatalytic conversion of H2O43, CO244, and N245, we used the following band gap, VBM, and CBM values, all in units of eV:
The 2D materials that are suitable for efficient photovoltaic cells are shown in magenta. The candidate 2D materials for the photoelectrochemical splitting of water into oxygen and hydrogen are shown in blue. The candidate 2D materials that are predicted to be photocatalytically active for CO2 reduction and inactive for oxygen evolution from water are shown in red. The candidate 2D materials that are predicted to be photocatalytically active for N2 reduction and inactive for oxygen evolution from water are shown in both red and green. For the classification of the promising materials for different energy applications, we used the ML-predicted band gap, VBM, and CBM of the 2D materials and the alignment of the frontier energy bands with respect to the redox potentials of the chemical conversion reactions under study, typically at pH = 0 in aqueous solution vs. NHE, 25 ∘C, and 1-atmosphere gas pressure. The values of the properties are predicted by using the final ML models that have been trained on the entire training data.
All: band gap < 1.75 and VBM < −5.67.
H2O: CBM > –4.44.
CO2: –4.47 < CBM < –4.44.
N2: –4.53 < CBM < –4.44.
In this study, we used the high-throughput DFT-PBE calculated data to train our ML models. Using this functional, the predictions from recent codes and pseudopotentials have been found to agree very well for elemental crystals46. However, it is also known that the PBE functional is usually not the best method available to predict the electronic properties of materials47,48,49. Although a variety of more accurate ab initio treatments are available, due to the computational efficiencies, they are currently not easily applicable for the study of large numbers of materials. Alternatively, it is possible to rescale PBE predictions to the predictions of more accurate methods23,47,49,50. Accordingly, we applied a regression study between PBE and G0W0 results on 188 instances of 2D materials from C2DB that have been calculated using both methods. Figure 7 shows the regression results associated with the below equations of band gap, VBM, and CBM.
Using Eqs. 1–3, we estimated the G0W0 values of the respective electronic properties of the 2D materials and included these in the V2DB.
Discussion
In this work, we illustrated the usefulness of AI methods for the design of new 2D materials and the discovery of good candidates for some exemplified photovoltaic and photocatalytic energy applications. As a proof of concept, we performed an AI-aided discovery of stable 2D materials. We screened more than 72 million virtually generated 2D materials, and using ML methods we determined the thermochemical, electronic, and magnetic properties of ~316 thousand likely stable 2D materials. We demonstrated the use of AI to work on a very large chemical space of feasible compounds. We identified thousands of promising candidate materials for photovoltaics and photocatalytic conversion of small molecules into fuels, all essentially by using only 22 different 2D crystal prototypes and a very modest size of quantum mechanical simulation data of 2226 2D compounds. Additionally, we showed that the basic elemental and structural information is effectual in determining the stability and the other key properties of 2D materials. Moreover, we validated the robustness of our approach by comparing the predicted properties of materials with data from an external database. Furthermore, to assist the future virtual screening efforts on the exponentially scaling virtual search space of material compositions, we provided the full details of a recipe for 2D material design through generation, filtering, and property prediction of compounds. The recipe is generic and tunable to work on new simulation and/or experimental datasets, particularly interesting by virtue of an anticipated future abundance of high fidelity and quality data on the structures and properties of 2D materials.
It is still an open question how far the data-driven virtual material screening methods can be generalized. A recent study by Kauwe et al.51 shows that ML can successfully be used to extrapolate the DFT-calculated properties of materials beyond the training set. Another recent study by Xiong et al.52, however, shows that the performance of ML models on the predictions of the unexplored materials is lower than their cross-validation performance on the training set. To investigate the extrapolation performance of our ML models, we developed a heatmap of the represented compounds in our training data. In Fig. 3, the strongly represented regions of the chemical space are shown with dark colors, whereas the weakly represented regions are shown with light colors. The white color shows the regions that have not been found in the training data. According to our cross-validation results, there is a relation between the accuracy of ML model predictions and the number of instances of prototypes and elements of the 2D materials. For example, the error in predictions of the band gap becomes significantly large for the prototypes that are represented by only a few instances, such as BN, GeSe, ISb, and SnS (see Supplementary Fig. 9), in the training data. We further analyzed the most extreme deviations in results when compared to an external dataset of 2D materials (see Supplementary Table 5). According to this analysis, our ML model used for the prediction of the band gaps is most erroneous when extrapolating the data on the GeSe prototype. The shortness of instances for this prototype, as shown in Fig. 3, has already signaled this conclusion. Similarly, for the CdI2 prototype, the model adequately predicts all 2D materials with the exception of compounds that contain oxygen or fluorine atoms. The poor performance of the model on the extrapolated data can be explained by considering the cross-validation prediction errors of the oxygen containing compounds in the CdI2 and the absence of the fluorine containing compounds on this crystal prototype in training data. As a result, the newly generated 2D materials with elements and prototypes that are poorly represented in the training data are expected to be prone to uncertainties in their predicted properties. For this reason, the predicted data on these materials should be handled with care. In this respect, the chemical space heatmap shown in Fig. 3 is a useful guideline when considering the reliability of the predictions on the entire compound space of 2D materials found in the V2DB.
Defining the chemical screening space and the filtering thresholds are determining points for the robustness of a predictive model. In our recipe, the generation and filtering processes of the 2D materials are all tunable even when using the present training data. Therefore, a further increase in the robustness of the ML models can be achieved by: (i) choosing to work on with prototypes that are sufficiently represented in the training data, (ii) choosing to work on with chemical elements that are sufficiently represented in the training data, (iii) keeping the atomic compositional variance of the 2D unit cells small, (iv) tightening the stability thresholds (e.g., ΔH and ΔHhull) to reduce the false-positive rate during the stability predictions.
The stability of a compound hints its synthesizability but does not necessarily ensure it. The synthesis of a 2D material is a complex process and depends on many factors beyond the assessment of its stability. In particular, one can expect that synthesizing 2D materials that consist of a mixture of chemical elements may prove to be challenging. Therefore, the newly predicted candidates may, for instance, be filtered according to the diversity of the atoms they contain. Moreover, a further selection of the 2D materials for experimental studies is possible by taking into account the toxicity and the abundance of the constituent elements53.
Methods
Training data
Training data is the base resource for both the generation, such as during the selection of prototypes and elements, of new materials and the development of ML models that are used to predict the stability and key properties of the generated materials. The following points should be considered when deciding upon the usefulness of a training dataset of 2D materials computed features:
-
preferably computed using the same physics-based method;
-
having information about the uncertainty of the employed computational method to generate data;
-
having traceable material structure information (i.e., space group, lattice parameters, and atom coordinates);
-
having well-established stability information on compounds;
-
having sufficiently large number of data instances;
-
having ample diversity in compound structures and chemical compositions.
In consideration of the above points, to generate the V2DB, we collected the training data from the C2DB23, an openly accessible 2D materials database with the DFT-calculated structures and information on the stability, electronic, and magnetic properties of compounds that have been obtained via a trustworthy workflow. The C2DB contains the data on 3331 2D materials. We filtered the DFT-computed data using the following criteria:
-
include materials that belong to the selected prototypes (Fig. 2a);
-
include materials that contain only the selected chemical elements (Fig. 2b);
-
include materials that have dynamic stability data (Supplementary Information Sections 1.2 and 1.3);
-
include materials that hßave DFT(PBE) band gap data.
A total of 2226 2D materials from the C2DB have successfully met all of the above criteria.
Selection of prototypes
The crystal prototypes are used as categorical references of structures. Materials that have the same prototype show similar build architecture in terms of the crystal symmetry (i.e., space group and positions of atoms in the unit-cell). The selection of prototypes is the first step of the new compound generation process, and together with the selection of elements step that is explained next, it concurrently determines the borders of the chemical search space of the compounds. We followed the below steps during the selection of prototypes:
-
identification of prototypes;
-
grouping prototypes according to unit-cell configurations (Fig. 2a);
-
selection of prototypes by unit-cell groups (Table 1).
The prototypes were carefully defined in the training data, and therefore we used them as are. In other situations when the prototype information is not explicitly provided, for instance in an emerging new dataset of 2D materials, the compounds that satisfy the below conditions can be considered to exist with the same prototype structure:
-
same space group;
-
same number of atoms in unit-cell.
In the next step, the identified prototypes are grouped according to the number of “A” (positively charged; cations) and “B” (negatively charged; anions) type atoms found in the unit cell. Finally, a cutoff for the maximum number of atoms per unit-cell is set. The number of atoms allowed in a unit cell exponentially affects the total number of new materials to be generated (see Table 1). For this reason, it is advised to set a small cutoff value, unless there is sufficiently large and homogeneously distributed data among different compositions of materials.
As shown in Fig. 2a, by setting a cutoff of six atoms per unit-cell, four different unit-cell groups are selected: AB, ABB, AABB, AABBBB. These unit-cell groups accommodate all of the 22 different crystal prototypes that are used as base structures during the process of candidate 2D material generation.
Selection of elements
Along with the crystal prototypes, the chemical elements are the most important descriptors of materials. They are effective during both, in defining the size of chemical search space, and in developing the new ML models that are aimed at the prediction of material properties. To reliably predict the properties of newly generated 2D materials, the chemical elements of the generated compounds must be sufficiently present in the training data. Therefore, the chemical elements are selected by deciding on a minimum number of instances of different compounds within the training data that contain those elements. Accordingly, there is usually a trade-off between the total number of materials to be generated and the accuracy of the ML models to be developed. High threshold values for the minimum number of instances for chemical elements will naturally decrease the total number of newly generated compounds, but they will facilitate a better estimation of the properties of materials using AI methods. Thus, the threshold values can be adjusted according to own discretion.
After an analysis of the number of occurrences of the chemical elements in the 2D materials of the prototypes, we selected elements that appeared in a minimum of ten different DFT-calculated compounds (see Supplementary Fig. 4). As shown in Fig. 2b, after applying this threshold we selected 42 A-type and 10 B-type, therefore a total of 52 different chemical elements from the periodic table.
Brute-force elemental substitution
We applied a brute-force elemental substitution method to generate a systematic and complete library of new 2D materials. Our brute-force elemental substitution algorithm systematically generates all possible candidate materials through the substitution of elements shown in Fig. 2b within the groups of prototypes shown in Fig. 2a. First, the atoms are classified into two categories of A and B depending on their anticipated electronic charges. Atoms from the “A” category always have positive charges, whereas atoms from the “B” category always have negative charges. Using these two categories, we defined simple substitution rules as follows:
-
A-type atom can only be replaced by an A-type atom.
-
B-type atom can only be replaced by a B-type atom.
Using a brute-force elemental substitution approach on the selected list of prototypes and chemical elements, we generated 72,522,240 new, but yet unfiltered 2D materials.
Symmetry filtering
The brute-force method generates all possible compositions of 2D compounds and disregards the symmetry of individual crystals. Therefore, the symmetrically identical duplicates should be identified and unified by removing the exact copies of compounds. For this purpose, we developed an algorithm that detects the duplicates in the generated 2D materials. The algorithm assigns symmetry labels to compounds based on the crystal prototypes and the atomic compositions. All compounds that share the same prototype structure have the same symmetry label. To define the symmetry labels for prototypes, we analyzed the symmetry in the unit-cell by considering pairs of atoms that belong to the same charge category, as shown in Fig. 2b. The 2D material is labeled with “Z” when no pairs of atoms are matched in its unit-cell (Table 1). The labels “X” and “Y” are used when pairs of A- and B-type atoms, respectively, are identified as symmetric according to a cut-plane that divides the unit cell into two symmetric pieces. For all the crystal prototypes and unit-cells considered in the current study, we used a total of five symmetry labels, as explained below:
-
Z: No symmetry in the prototype.
-
X: “A” atoms are symmetric.
-
Y: “B” atoms are symmetric.
-
XY: All “A” and “B” atoms are symmetric.
-
XYY: “A” atoms are symmetric, two “BB” atom couples are symmetric independently (specifically for AABBBB unit-cell group structures).
Using the symmetry filtering, we detected and removed the duplicate copies from the whole set of generated compounds. A total of 10,321,920 structurally unique 2D materials have sifted through the symmetry filter.
Neutrality filtering
The neutrality filter considers all of the possible charge combinations of constituting elements for the compound under investigation, as calculated by different charge contributions of its A- and B-type atoms. In a condition when a no charge neutral composition can be achieved between the constituting atoms of the compound, the 2D material is removed from the list of candidates. We used Greenwood’s tabulated data54 as a reference of possible charge states of the atoms in our 2D material compositions. In addition to a reference table of elemental electrical charges, we applied the following criteria:
-
A-type atoms can only have positive electrical charge(s).
-
B-type atoms can only have negative electrical charge(s).
After applying the neutrality filter, the compounds that are not charge neutral are removed from the list of candidates, and a total of 9,732,136 2D materials remained.
Stability filtering
One of the important challenges of virtual material discovery is the uncertainty of the experimental synthesizability of the newly designed compounds. An approach to mitigate this uncertainty is to estimate the stability of the newly designed material. In simple terms, the stability of a material is defined as the ability to maintain the designed atomic configuration under specific physical and chemical conditions. A recent study55 demonstrated the applicability of an ML approach to predict the thermodynamic stability of 2D materials. However, a more comprehensive and accurate stability filter requires the inclusion of the three key factors of energy, phonon, and dynamic stability56. Considering these three factors, we developed ML models in order to identify the likely stable materials from the newly generated and filtered library of candidate 2D materials. To determine the stability of the compounds, we used the following three criteria on the ML-predicted properties, that is “is stable”, “heat of formation (ΔH)”, and “energy above convex hull (ΔHhull)”:
-
is stable = True.
-
ΔH < 0.2 eV/atom.
-
ΔHhull < 0.2 eV/atom.
“Is stable” is a binary property data that is derived from a combination of thermodynamic and dynamic stability levels of materials as learned from the training data (see Supplementary Information Section 1.2). Fundamentally, both the ΔH and ΔHhull must be negative in order to consider that material as thermodynamically stable. However, noting the accuracy of the DFT methodology using the PBE functional is ~0.2 eV/atom57, we used 0.2 eV/atom as a high cutoff in order to maximize the recall. Yet, we note that it is possible to use the low cutoff of –0.2 eV/atom to maximize the precision of the ML model. After applying the stability filter, a total of 316,505 2D materials have remained as likely stable candidates. The technical details of our ML models that are developed and used for the task of stability filtering are provided in the Supplementary Information Section 1.2.
ML model development
All machine learning models are developed using the scikit-learn machine learning library on python 3.6. A separate ANN has been trained for each target material property. Importantly, as input features for our ML models, we used only the basic element level information, which is non-DFT-calculated and can directly be extracted from the atomic composition of materials. The following features are used for our ML models (see Supplementary Information Section 2.1):
-
Atom per unit-cell: total number of atoms in the unit-cell of the 2D compound.
-
Prototype vector: one-hot vector of the 2D crystal prototypes.
-
Chemical composition vector: a vector with a ratio information of each chemical element within the unit-cell of the 2D compound, as calculated individually for A- and B-type atoms.
-
Electronegativity vector: the geometric mean of the Pauling scale electronegativity of the chemical elements within the unit-cell of the 2D compound, as calculated individually for A- and B-type atoms.
We tuned each ML model independently by optimizing the hyper-parameters using a grid search method (see Supplementary Information Section 2.2). We used a 20-Fold cross-validation technique for evaluating our ML models. To reduce bias, we trained the final ML models, which are used for the identification of likely stable 2D material candidates and the prediction of their key properties, using the entire DFT dataset. It should be noted that the labeling procedure applied here is deterministic and only the basic features with element level information are used. Therefore, it is expected that the predicted properties for materials that have the same prototype and the same chemical formula will be labeled with exactly the same values. Further information on the development of ML models is provided in the Supplementary Information Section 2.
ML model validation
Very recently, 2Dmatpedia24, a new 2D material database has been announced. The 2Dmatpedia contains a total of 6351 2D compounds with properties that were calculated using DFT. Although there are some differences in the threshold parameters used for the DFT calculations, essentially the 2Dmatpedia and the C2DB have a similar computational methodology, in terms of the use of PBE exchange-correlation functional, atomic pseudopotentials, and structural optimizations. Therefore, we used the 2Dmatpedia database to validate the accuracy of our ML model for the prediction of the band gap, which is the only comparable DFT-calculated property between 2Dmatpedia and C2DB. To screen for the mutual materials in our V2DB and the 2Dmatpedia, we first developed an algorithm that compares the materials based on their chemical formula and the space group. However, the formula and space group information are not sufficient enough to confirm the identicality of the materials from the two sources. Therefore, we also paired the materials by comparing their three-dimensional structure views. As a result, we identified a total of 103 matched materials from the two databases of V2DB and 2Dmatpedia. Twenty-seven of these compounds were not found in C2DB, therefore they were not included in the training set. The predicted band gaps of the matched materials have MAE of 0.438 eV. Considering that there is a cumulative effect of the difference between the C2DB and 2Dmatpedia with MAE = 0.132 eV, and the cross-validation error of our ML model for band gap predictions with MAE = 0.135 eV, the result is promising for the applicability of our ML model. It is also important to note that the validation data comprises only 5 out of the 22 crystal prototypes of the generated chemical space of the 2D materials. Therefore, a larger and sufficiently diverse data will provide a more comprehensive validation. The distributions of the band gap energy differences between the V2DB and 2Dmatpedia databases are provided in Supplementary Figs. 11 and 12. Additionally, we analyzed the most extreme errors (see Supplementary Tables 4 and 5) and discussed the possible weaknesses of the model for generalizability above in the “Discussion” section.
Data availability
V2DB is stored in the comma-separated values (CSV) format and it contains chemical formula, crystal prototype, and physicochemical, electronic and magnetic property information of 2D materials as further explained in Supplementary Table 1. V2DB is openly accessible at the Harvard Dataverse Repository58 and AMD research group website (https://www.amdlab.nl/database/V2DB/).
Code availability
The reproducibility of the generation algorithm of 2D materials and the property prediction algorithms can be verified by executing the provided scripts on Code Ocean59.
References
Agrawal, A. & Choudhary, A. Perspective: Materials informatics and big data: realization of the fourth paradigm of science in materials science. APL Mater. 4, 053208 (2016).
Fischer, C. C., Tibbetts, K. J., Morgan, D. & Ceder, G. Predicting crystal structure by merging data mining with quantum mechanics. Nat. Mater. 5, 641 (2006).
Ramprasad, R., Batra, R., Pilania, G., Mannodi-Kanakkithodi, A. & Kim, C. Machine learning in materials informatics: recent applications and prospects. NPJ Comput. Mater. 3, 54 (2017).
Zhou, Q. et al. Learning atoms for materials discovery. Proc. Natl Acad. Sci. 115, E6411–E6417 (2018).
Schmidt, J., Marques, M. R. G., Botti, S. & Marques, M. A. L. Recent advances and applications of machine learning in solid-state materials science. NPJ Comput. Mater. 5, 1–36 (2019).
Himanen, L., Geurst, A., Foster, A. S. & Rinke, P. Data-driven materials science: status, challenges, and perspectives. Adv. Sci. 6, 1900808 (2019).
Noh, J. et al. Inverse design of solid-state materials via a continuous representation. Matter 1, 1370–1384 (2019).
Curtarolo, S. et al. The high-throughput highway to computational materials design. Nat. Mater. 12, 191–201 (2013).
Hachmann, J. et al. Lead candidates for high-performance organic photovoltaics from high-throughput quantum chemistry–the Harvard Clean Energy Project. Energy Environ. Sci. 7, 698–704 (2014).
Meredig, B. et al. Combinatorial screening for new materials in unconstrained composition space with machine learning. Phys. Rev. B 89, 094104 (2014).
Pyzer-Knapp, E. O., Suh, C., Gómez-Bombarelli, R., Aguilera-Iparraguirre, J. & Aspuru-Guzik, A. What is high-throughput virtual screening? A perspective from organic materials discovery. Annu. Rev. Mater. Res. 45, 195–216 (2015).
Singh, A. K., Mathew, K., Zhuang, H. L. & Hennig, R. G. Computational screening of 2D materials for photocatalysis. J. Phys. Chem. Lett. 6, 1087–1098 (2015).
Gómez-Bombarelli, R. et al. Design of efficient molecular organic light-emitting diodes by a high-throughput virtual screening and experimental approach. Nat. Mater. 15, 1120 (2016).
Mounet, N. et al. Two-dimensional materials from high-throughput computational exfoliation of experimentally known compounds. Nat. Nanotechnol. 13, 246 (2018).
Castelli, I. E., Thygesen, K. S. & Jacobsen, K. W. Calculated optical absorption of different perovskite phases. J. Mater. Chem. A 3, 12343–12349 (2015).
Yu, L. & Zunger, A. Identification of potential photovoltaic absorbers based on first-principles spectroscopic screening of materials. Phys. Rev. Lett. 108, 068701 (2012).
Sorkun, M. C., Khetan, A. & Er, S. AqSolDB, a curated reference set of aqueous solubility and 2D descriptors for a diverse set of compounds. Sci. Data 6, 1–8 (2019).
Briggs, N. et al. A roadmap for electronic grade 2D materials. 2D Mater. 6, 022001 (2019).
Li, S. et al. New opportunities for emerging 2D materials in bioelectronics and biosensors. Curr. Opin. Biomed. Eng. 13, 32–41 (2019).
Novoselov, K. S. et al. Electric field effect in atomically thin carbon films. Science 306, 666–669 (2004).
Bhimanapati, G. R. et al. Recent advances in two-dimensional materials beyond graphene. ACS Nano 9, 11509–11539 (2015).
Ashton, M., Paul, J., Sinnott, S. B. & Hennig, R. G. Topology-scaling identification of layered solids and stable exfoliated 2D materials. Phys. Rev. Lett. 118, 106101 (2017).
Haastrup, S. et al. The computational 2D materials database: high-throughput modeling and discovery of atomically thin crystals. 2D Mater. 5, 042002 (2018).
Zhou, J. et al. 2DMatPedia, an open computational database of two-dimensional materials from top-down and bottom-up approaches. Sci. Data 6, 86 (2019).
Jain, A. et al. Commentary: the materials project: a materials genome approach to accelerating materials innovation. APL Mater. 1, 011002 (2013).
Gražulis, S. et al. Crystallography Open Database (COD): an open-access collection of crystal structures and platform for world-wide collaboration. Nucleic Acids Res. 40, D420–D427 (2011).
Bergerhoff, G. & Brown, I. D. Crystallographic databases. International Union of Crystallography 360, 77–95 (1987).
Jain, A., Shin, Y. & Persson, K. A. Computational predictions of energy materials using density functional theory. Nat. Rev. Mater. 1, 15004 (2016).
Butler, K. T., Davies, D. W., Cartwright, H., Isayev, O. & Walsh, A. Machine learning for molecular and materials science. Nature 559, 547–555 (2018).
Tabor, D. P. et al. Accelerating the discovery of materials for clean energy in the era of smart automation. Nat. Rev. Mater. 3, 5–20 (2018).
Zhang, X., Chen, A. & Zhou, Z. High-throughput computational screening of layered and two-dimensional materials. Wiley Interdiscip. Rev.: Computational Mol. Sci. 9, e1385 (2019).
Momeni, K. et al. Multiscale computational understanding and growth of 2D materials: a review. NPJ Comput. Mater. 6, 1–18 (2020).
Ma, X. Y., Lewis, J. P., Yan, Q. B. & Su, G. Accelerated discovery of two-dimensional optoelectronic octahedral oxyhalides via high-throughput Ab initio calculations and machine learning. J. Phys. Chem. Lett. 10, 6734–6740 (2019).
Zhou, J. et al. Discovery of hidden classes of layered electrides by extensive high-throughput material screening. Chem. Mater. 31, 1860–1868 (2019).
Jin, H. et al. Discovery of novel two-dimensional photovoltaic materials accelerated by machine learning. J. Phys. Chem. Lett. 11, 3075-3081 (2020).
Zhang, X. et al. Computational screening of 2D materials and rational design of heterojunctions for water splitting photocatalysts. Small Methods 2, 1700359 (2018).
Zhan, C., Sun, W., Xie, Y., Jiang, D. & Kent, P. R. Computational discovery and design of MXenes for energy applications: status, successes, and opportunities. ACS Appl. Mater. interfaces 11, 24885–24905 (2019).
Ge, L. et al. Predicted optimal bifunctional electrocatalysts for both HER and OER using chalcogenide heterostructures based on machine learning analysis of in silico quantum mechanics based high throughput screening. J. Phys. Chem. Lett. 11, 869-876 (2020).
Shin, H., Ha, Y. & Kim, H. 2D covalent metals: a new materials domain of electrochemical CO2 conversion with broken scaling relationship. J. Phys. Chem. Lett. 7, 4124–4129 (2016).
Xiao, Y. & Zhang, W. High throughput screening of M 3 C 2 MXenes for efficient CO 2 reduction conversion into hydrocarbon fuels. Nanoscale 12, 7660–7673 (2020).
Green, M. A., Emery, K., Hishikawa, Y., Warta, W. & Dunlop, E. D. Solar cell efficiency tables (Version 45). Prog. Photovoltaics: Res. Appl. 23, 1–9 (2015).
Nayak, P. K., Mahesh, S., Snaith, H. J. & Cahen, D. Photovoltaic solar cell technologies: analysing the state of the art. Nat. Rev. Mater. 4, 269 (2019).
Chakrapani, V. et al. Charge transfer equilibria between diamond and an aqueous oxygen electrochemical redox couple. Science 318, 1424–1430 (2007).
Benson, E. E., Kubiak, C. P., Sathrum, A. J. & Smieja, J. M. Electrocatalytic and homogeneous approaches to conversion of CO2 to liquid fuels. Chem. Soc. Rev. 38, 89–99 (2009).
Deng, J., Iñiguez, J. A. & Liu, C. Electrocatalytic nitrogen reduction at low temperature. Joule 2, 846–856 (2018).
Lejaeghere, K. et al. Reproducibility in density functional theory calculations of solids. Science 351, aad3000 (2016).
Demiroglu, I., Tosoni, S., Illas, F. & Bromley, S. T. Bandgap engineering through nanoporosity. Nanoscale 6, 1181–1187 (2014).
Chen, W. & Pasquarello, A. Band-edge positions in G W: Effects of starting point and self-consistency. Phys. Rev. B 90, 165133 (2014).
Ergönenc, Z., Kim, B., Liu, P., Kresse, G. & Franchini, C. Converged G W quasiparticle energies for transition metal oxide perovskites. Phys. Rev. Mater. 2, 024601 (2018).
Pilania, G., Gubernatis, J. E. & Lookman, T. Multi-fidelity machine learning models for accurate bandgap predictions of solids. Comput. Mater. Sci. 129, 156–163 (2017).
Kauwe, S. K., Graser, J., Murdock, R. & Sparks, T. D. Can machine learning find extraordinary materials? Comput. Mater. Sci. 174, 109498 (2020).
Xiong, Z. et al. Evaluating explorative prediction power of machine learning algorithms for materials discovery using k-fold forward cross-validation. Comput. Mater. Sci. 171, 109203 (2020).
Kuhar, K., Pandey, M., Thygesen, K. S. & Jacobsen, K. W. High-throughput computational assessment of previously synthesized semiconductors for photovoltaic and photoelectrochemical devices. ACS Energy Lett. 3, 436–446 (2018).
Greenwood, N. N. & Earnshaw, A. Chemistry of the Elements (Pergamon Press, Oxford, 1984).
Schleder, G. R., Acosta, C. M. & Fazzio, A. Exploring two-dimensional materials thermodynamic stability via machine learning. ACS Appl. Mater. Interf. 12, 20149-20157 (2020).
Malyi, O. I., Sopiha, K. V. & Persson, C. Energy, phonon, and dynamic stability criteria of two-dimensional materials. ACS Appl. Mater. Interf. 11, 24876–24884 (2019).
Pandey, M. & Jacobsen, K. W. Heats of formation of solids with error estimation: The mBEEF functional with and without fitted reference energies. Phys. Rev. B 91, 235201 (2015).
Sorkun, M. C., Astruc, S., Koelman, J. M. V. A. & Er, S. V2DB: Virtual 2D materials database. Harvard Dataverse. https://doi.org/10.7910/DVN/SNCZF4 (2020).
Sorkun, M. C., Astruc, S., Koelman, J. M. V. A. & Er, S. The virtual 2D materials database (V2DB). Code Ocean. https://doi.org/10.24433/CO.7049461.v1 (2020).
Acknowledgements
We acknowledge funding from the initiative “Computational Sciences for Energy Research” of Shell and the Netherlands Organization for Scientific Research (NWO) grant no 15CSTT05. This work was sponsored by NWO Exact and Natural Sciences for the use of supercomputer facilities.
Author information
Authors and Affiliations
Contributions
M.C.S. and S.A. developed codes for the generation, the filtering, and the property prediction of V2DB. S.E. devised and supervised the project. All authors contributed to the writing of the manuscript.
Corresponding author
Ethics declarations
Competing interests
The authors declare no competing interests.
Additional information
Publisher’s note Springer Nature remains neutral with regard to jurisdictional claims in published maps and institutional affiliations.
Supplementary information
Rights and permissions
Open Access This article is licensed under a Creative Commons Attribution 4.0 International License, which permits use, sharing, adaptation, distribution and reproduction in any medium or format, as long as you give appropriate credit to the original author(s) and the source, provide a link to the Creative Commons license, and indicate if changes were made. The images or other third party material in this article are included in the article’s Creative Commons license, unless indicated otherwise in a credit line to the material. If material is not included in the article’s Creative Commons license and your intended use is not permitted by statutory regulation or exceeds the permitted use, you will need to obtain permission directly from the copyright holder. To view a copy of this license, visit http://creativecommons.org/licenses/by/4.0/.
About this article
Cite this article
Sorkun, M.C., Astruc, S., Koelman, J.M.V.A. et al. An artificial intelligence-aided virtual screening recipe for two-dimensional materials discovery. npj Comput Mater 6, 106 (2020). https://doi.org/10.1038/s41524-020-00375-7
Received:
Accepted:
Published:
DOI: https://doi.org/10.1038/s41524-020-00375-7
This article is cited by
-
Printed polymer platform empowering machine-assisted chemical synthesis in stacked droplets
Nature Communications (2024)
-
Graphene nanoparticles as data generating digital materials in industry 4.0
Scientific Reports (2023)
-
Descriptor engineering in machine learning regression of electronic structure properties for 2D materials
Scientific Reports (2023)
-
MatHub-2d: A database for transport in 2D materials and a demonstration of high-throughput computational screening for high-mobility 2D semiconducting materials
Science China Materials (2023)
-
Pettifor maps of complex ternary two-dimensional transition metal sulfides
npj Computational Materials (2022)