Abstract
Polycystic ovary syndrome (PCOS) is the most common reproductive disorder in women, yet there is little consensus regarding its aetiology. Here we perform a genome-wide association study of PCOS in up to 5,184 self-reported cases of White European ancestry and 82,759 controls, with follow-up in a further ∼2,000 clinically validated cases and ∼100,000 controls. We identify six signals for PCOS at genome-wide statistical significance (P<5 × 10−8), in/near genes ERBB4/HER4, YAP1, THADA, FSHB, RAD50 and KRR1. Variants in/near three of the four epidermal growth factor receptor genes (ERBB2/HER2, ERBB3/HER3 and ERBB4/HER4) are associated with PCOS at or near genome-wide significance. Mendelian randomization analyses indicate causal roles in PCOS aetiology for higher BMI (P=2.5 × 10−9), higher insulin resistance (P=6 × 10−4) and lower serum sex hormone binding globulin concentrations (P=5 × 10−4). Furthermore, genetic susceptibility to later menopause is associated with higher PCOS risk (P=1.6 × 10−8) and PCOS-susceptibility alleles are associated with higher serum anti-Müllerian hormone concentrations in girls (P=8.9 × 10−5). This large-scale study implicates an aetiological role of the epidermal growth factor receptors, infers causal mechanisms relevant to clinical management and prevention, and suggests balancing selection mechanisms involved in PCOS risk.
Similar content being viewed by others
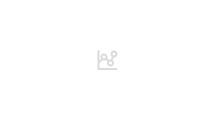
Introduction
Polycystic ovary syndrome (PCOS) is a common reproductive disorder in women that is defined by two out of three criteria: (1) menstrual irregularity (oligo-ovulation or anovulation), (2) hyperandrogenism (clinical or biochemical) and (3) polycystic ovarian morphology1,2. Phenotypic heterogeneity between cases has limited the ability to make definitive conclusions regarding its aetiology and pathophysiology. Obesity is associated with PCOS, but its causal role has yet to be determined3; alternative explanations include reverse causality (that is, PCOS increases susceptibility to weight gain) and synergistic but independent roles for obesity and PCOS in infertility4. Hence, the role of lifestyle modification to prevent or reverse the reproductive abnormalities of PCOS is not well established5,6. Furthermore, although there is extensive evidence linking insulin resistance to PCOS, it is widely considered that the cellular and molecular mechanisms of insulin resistance in PCOS differ from those in other common insulin-resistant states such as obesity and diabetes3,7. Consequently, the role of insulin sensitisation therapy in PCOS remains limited to the prevention of cardiovascular disease and type 2 diabetes (T2D)8,9.
Genetic studies could identify underlying genes and pathways, and thereby provide insights into the aetiology of PCOS. The results of candidate gene studies have been inconclusive, in large part due to underpowered studies, lack of replication and limited prior understanding of its pathogenesis10. Two, large genome-wide association studies (GWAS) for PCOS in overlapping Han Chinese populations identified in total 11 genomic loci11,12. Although these loci were enriched for candidate genes related to insulin signalling, steroid hormone regulation and T2D, and also for genes related to calcium signalling and endocytosis, the ability to make mechanistic interpretations from those findings was limited and only a few of these loci have been replicated in PCOS cases of European ancestry13,14,15,16,17. Furthermore, the striking paradox of a highly heritable yet common condition that impairs fertility has led to multiple theories for a balancing advantage of PCOS susceptibility4. Suggested mechanisms include enhanced fetal growth and development18 or reproductive advantages, such as earlier pubertal maturation19 or retarded ovarian ageing leading to a sustained reproductive lifespan20.
Here we present a large-scale GWAS for PCOS in cases and controls of Caucasian European ancestry. As well as being the largest such study to date, we use dense imputation of genotypes to better implicate the probable genes underlying the association signals. As the GWAS is based on self-reported PCOS cases, we present follow-up in additional studies of clinically validated cases. We find six genetic loci associated with PCOS, highlighting aetiological roles for the epidermal growth factor receptors (EGFRs) and for the pituitary-derived gonadotrophins. Furthermore, using a genetic instrumental variable approach (i.e., Mendelian randomization)21, we infer causal roles in PCOS aetiology for higher body mass index (BMI), higher insulin resistance and lower serum sex hormone binding globulin (SHBG) concentrations. Finally, we find a robust association between menopause age-delaying alleles and higher risk of PCOS, suggesting a potential evolutionary advantage for PCOS genetic susceptibility.
Results
Genome-wide association signals for PCOS
Six independent common signals reach genome-wide significance (logistic regression P<1 × 10−8) for association with PCOS in the meta-analysis of discovery and follow-up studies (Table 1, Fig. 1 and Supplementary Fig. 1); four are novel signals and two represent refinements of previously reported signals at the YAP1 and THADA loci. All signals show at least nominally significant (P<0.05) directionally concordant associations in the follow-up studies of clinically validated PCOS cases, with no significant heterogeneity by PCOS case definition (Supplementary Table 2).
Our strongest novel PCOS signal (rs1351592, odds ratio: 1.18 (1.13–1.23), P=1.2 × 10−12) is intronic in ERBB4/HER4, which encodes a member of the EGFR family. Notably, we find further sub-genome-wide significant signals in/near genes encoding two of the other three EGFR family members: rs7312770 (P=2.1 × 10−7) in/near ERBB3/HER3 is correlated (r2=0.40) with the reported PCOS signal (rs705702) at 12q13.2 and rs7218361 (P=9.6 × 10−7) is a low-frequency variant ∼200 kb downstream of ERRB2/HER2.
Our second strongest novel signal (rs11031006, P=1.3 × 10−9) lies near FSHB, which encodes the hormone-specific β-subunit of follicle stimulating hormone (FSH), a key promoter of ovarian follicle growth and oestrogen production. Interestingly, in deCODE samples, the PCOS-susceptibility allele at rs11031006 is also robustly associated with lower circulating FSH concentrations (β=−0.089 s.d. per allele, P=9.2 × 10−10, n=15,586 women), higher luteinizing hormone (LH) concentrations (β=0.115 s.d. per allele, P=3.6 × 10−15, n=17,469 women) and higher LH/FSH ratio (β=0.272 s.d. per allele, P=5.94 × 10−68, n=14,310 women). This variant represents the strongest association signal for FSH, LH and LH/FSH ratio at this FSHB locus. Notably, a variant rs12294144 correlated with the PCOS risk allele is reportedly associated with later age at menopause22. Furthermore, FSH signalling was implicated in PCOS in the Han Chinese GWAS study through association with the FSH receptor gene FSHR12. However, that signal is only weakly associated with PCOS in our data (Table 2, rs2268361, P=1.6 × 10−2).
Our third novel signal (rs13164856, P=3.5 × 10−9) is near RAD50, which encodes a protein involved in DNA double-strand break repair. Fourth, rs1275468 (P=1.9 × 10−8) indicates a novel PCOS signal near KRR1, which encodes a ribosome assembly factor.
Previously reported PCOS loci
Of the 11 PCOS signals reported in Han Chinese11,12, we observe directionally consistent associations for 10 variants, 6 of which are at least nominally associated (P<0.05) in our discovery GWAS samples (Table 2). Effect estimates are consistently smaller in our data, and in several instances the risk allele frequency is markedly different between these Han Chinese and white European populations. At three reported Han Chinese PCOS loci (YAP1, THADA and DENND1A), we observe different lead signals in our white European samples (Table 1). Our lead YAP1 signal, rs11225154 intronic to YAP1, is highly correlated with the reported YAP1 signal (r2=0.74 with rs1894116) and reaches genome-wide significance in our combined discovery and follow-up analysis (P=7.6 × 10−11). Our lead THADA signal, rs7563201 intronic to THADA, also reaches genome-wide significance (P=3.3 × 10−10) but is only weakly correlated with the reported THADA signal (r2=0.08 with rs13429458). Our lead DENND1A signal (rs10760321) is also weakly correlated with the reported DENND1A signal (r2=0.02 with rs2479106) but was not confirmed in our follow-up samples. These findings probably reflect differences in allelic structure between Chinese and European ancestry groups, as has been concluded by other investigators15, and limit the potential for conventional meta-analysis across these populations.
Mendelian randomization analyses
Our Mendelian randomization analyses indicate causal effects on PCOS aetiology for higher BMI (odds ratios: 1.90 per +1 s.d., 95% confidence interval: 1.55–2.34, P=2.5 × 10−9), higher insulin resistance (1.11 per +1 s.d., 1.05–1.19, P=6 × 10−4) and lower circulating SHBG concentrations (0.86 per +1 s.d., 0.78–0.93, P=5 × 10−4) (Table 3). Furthermore, the multiple allele score for menopausal age is positively associated with PCOS risk (1.60 per +1 s.d., 1.35–1.91, P=1.6 × 10−8), indicating a common biological mechanism that promotes both PCOS susceptibility and later menopause. Our sensitivity analyses show apparent dose–response effects across individual single-nucleotide polymorphisms (SNPs) in each of these scores (Fig. 2) and Funnel plots show no SNPs with outlier effects (Supplementary Fig. 3). In contrast, we find no evidence for causal effects on PCOS for birth weight (P=0.22) or age at menarche (P=0.23).
Other biological mechanisms associated with PCOS
By systematic testing of all GWAS SNPs across all known biological pathways using meta-analysis gene-set enrichment of variant associations (MAGENTA) software, we find one further pathway (ATP-binding cassette transporters) that is enriched for PCOS-associated variants. This pathway includes the genome-wide significant signal at the DNA repair gene RAD50 (rs13164856) and 37 other genes.
The PCOS-susceptibility alleles at our six PCOS loci are also consistently associated with higher anti-Mullerian hormone (AMH) concentrations in girls (cumulative score: P=8.9 × 10−5) (Supplementary Fig. 3). However, none of these six genome-wide significant PCOS loci (nor any of the four suggestive loci) overlap with reported signals of positive selection and we can find no evidence of polygenic selection on the set of six loci considered together (P=0.22) (Supplementary Note). Furthermore, these PCOS SNPs (or their proxies) are not associated with BMI (in aggregate: P=0.22).
Discussion
This large-scale genetic study reveals a number of insights into the aetiology and pathophysiology of PCOS. The findings from our Mendelian randomization analyses have perhaps most immediate relevance for treatment and prevention21, as these infer causal roles of greater BMI and insulin resistance. The role of interventions aimed at these targets in PCOS is debated. A recent US Endocrine Society Task Force found evidence that lifestyle modification reduces fasting blood glucose and insulin concentrations in women with PCOS but has uncertain effects on the key clinical features of PCOS, including reproductive outcomes5. The same conclusion was reached for the use of the insulin sensitizer Metformin in PCOS5,23. Conversely, a recent non-quantitative synthesis of dietary interventions positively concluded that weight-reducing diets have clinical benefits in PCOS24. The limitations of Mendelian randomization analyses are well-recognized; its major assumptions regarding lack of heterogeneity and pleiotropy are supported by the consistency of our findings across individual SNPs. Furthermore, the reported inverse association between the insulin resistance genetic score and BMI25 might attenuate our observed positive univariate effects of these traits on PCOS risk. Other uncertainties remain, such as possible canalization and age-specific effects. Our findings should encourage the development and testing of more effective interventions to lower BMI and insulin resistance in women with PCOS.
Our findings also infer a causal protective role of SHBG for PCOS, as has been reported for T2D26. SHBG regulates the bioavailability of testosterone. Therefore, genetic variants that lower circulating SHBG concentrations might directly modify the key hyperandrogenic phenotype of PCOS and also the related adverse metabolic profile27. Circulating SHBG concentrations rise markedly with the introduction of combined oral contraceptive pills, which are used by many women with PCOS for treatment of menstrual irregularity, acne and hirsutism28; however, there are as yet no therapeutic agents that specifically target SHBG concentrations or activity. Despite the lack of any overlap between SNPs used in the SHBG and insulin resistance scores, it remains possible that these traits might lie on the same causal pathway, in which case joint interventions might have synergistic effects.
Our novel genetic signals indicate a major role of the EGFRs in the pathogenesis of PCOS. There are four members of the EGFR family: EGFR, ERBB2, ERBB3 and ERBB4 (the last three are also known as the human epidermal receptors: HER-2, HER-3 and HER-4)29. These receptors form ligand-activated homo- or heterodimers with each other, which activates tyrosine kinase, and in cancer cells result in cell proliferation, blocking of apoptosis, activation of invasion and metastasis, and stimulation of neovascularization. EGFR signalling mediates LH-induced steroidogenesis, which in turn promotes late follicular maturation30,31. EGFRs are overexpressed in ovarian cancer32,33 and repression of ERBB2/HER-2 determines the breast cancer response to the oestrogen receptor inhibitor tamoxifen34. Small molecules or monoclonal antibodies that block EGFR activation are effective cancer chemotherapy agents29. Variable reported associations between PCOS and risks of breast, endometrial and ovarian cancers are limited by small sample sizes and confounding due to related risk factors such as nulliparity, infertility and its treatment, anovulation and obesity3. Our findings provide a possible genetic link between PCOS and cancer risk, and also suggest potential ovary-targeted pharmaceutical interventions for treatment of PCOS.
The novel PCOS locus at FSHB represents striking biological complementarity to the locus at the FSH receptor gene FSHR reported in Han Chinese12. However, the impact of that FSHR variant on FSH receptor activity is unclear and that locus shows only nominal association in our data, likely to be due to population differences in genetic architecture. Non-synonymous variants in FSHR that confer lower FSH receptor activity are inconsistently associated with PCOS35. We show that the PCOS-susceptibility allele at FSHB is robustly associated with a higher LH/FSH ratio, which is the hallmark biochemical PCOS trait that promotes ovarian androgen production and arrests follicular growth36. Although the high LH/FSH ratio observed in PCOS might be exacerbated by central feedback effects of peripheral hyperandrogenemia37, our findings establish a co-primary neuroendocrine pathogenesis of PCOS.
Our findings inform the long-standing debate regarding the evolutionary paradox of PCOS as a common yet highly heritable disorder characterized by infertility. We cannot find evidence for recent, strong positive selection of PCOS-susceptibility alleles; however, available tests may be insensitive to detect signals that affect complex traits38,39. The robust association between menopause age-raising alleles and PCOS susceptibility implicates a common mechanism that retards ovarian ageing. GWAS studies for age at menopause has highlighted a key role for DNA repair pathways22,40 and their putative relevance to PCOS is supported by the novel PCOS locus near to RAD50, a gene that is involved in DNA double-strand break repair and is mutated in the Nijmegen breakage syndrome-like disorder. Anovulation in women with PCOS is characterized by arrested follicle growth at the early antral stage, when AMH secretion from follicular granulosa cells is highest. Higher AMH concentrations consequently inhibit the recruitment of further primordial follicles, possibly representing more efficient use of the primordial ovarian pool20. This mechanism could possibly explain the consistent association we find between PCOS-susceptibility alleles and higher serum AMH concentrations, and might be a further mechanism towards slower ovarian ageing. Alternatively, higher AMH concentrations could indicate a larger ovarian primordial follicle pool size4. Such evolutionary debates are not just interesting arguments, but may be eventually informative to clinical practice. The anticipated persistence of reproductive lifespan may inform the use of artificial reproductive therapies or long-term lifestyle intervention strategies in women with PCOS.
Progress in identifying PCOS-susceptibility variants has been slow compared with other complex diseases, in part due to the relatively small collections of cases10. We demonstrate here, as previously reported for other traits41, that online self-reports of disease status is a highly efficient study design to identify large numbers of disease cases, providing sufficient power to identify robust genetic signals for PCOS. This is evident by our confirmation of previously identified PCOS signals in Han Chinese, by the highly consistent validation of our novel loci in cases defined by stringent clinical criteria and by the lack of heterogeneity in variant effect sizes between these case groups. That said, it remains important to confirm any findings of self-reported case studies in clinically validated cases.
The range of biological mechanisms that we can currently test by Mendelian randomization is limited by available GWAS findings. In particular, future analyses are needed to investigate the roles of androgen production and activity once robust genetic markers for those traits are identified. Indeed, we anticipate that future genetic instruments will allow wider and deeper testing of causal biological pathways. Although such analyses cannot infer possible developmental stage-specific effects of these pathways, the findings should encourage experimental studies that target these pathways, both to confirm the causal inferences and also to inform effective intervention and preventive strategies.
In conclusion, this genetic study reveals new biological and evolutionary insights into the pathogenesis of PCOS, including a major role of EGFRs, a co-primary neuroendocrine pathogenesis and genetic mechanisms towards slower ovarian ageing. Furthermore, the causal inferences from our Mendelian randomization analyses should support future efforts to develop and test effective interventions, to reduce body weight and insulin resistance in the treatment and prevention of PCOS.
Methods
Discovery phase
Genome-wide SNP data were available on 5,184 women of White European ancestry with self-reported PCOS and 82,759 controls from the 23andMe study (see Supplementary Table 1 and Supplementary Note for details of the 23andMe study). Imputation was performed against the 1000 Genomes reference (March 2012 v3 release), yielding ∼9 M variants that passed imputation and minor allele frequency criteria. A logistic regression model adjusting for age- and study-specific principal components was performed assuming an additive allelic model including covariates for age and the top five principal components to account for residual population structure. Test statistics were further adjusted for the observed λ-value 1.041. 23andMe participants provided informed consent to take part in this research under a protocol approved by Ethical and Independent Review Services, an accredited institutional review board.
Follow-up studies
From our discovery GWAS phase results, we selected for follow-up in additional studies: (a) all signals that showed at least suggestive associations (P<1 × 10−6) with PCOS (N=5 signals, where a signal is defined by the most significant SNP within a 1-Mb window; Table 1); (b) all possible signals for PCOS (P<1 × 10−5) located within 500 kb of signals previously reported in Han Chinese (N=3 signals; in/near YAP1, THADA and DENND1A); and (c) possible signals for PCOS near to biological candidate genes (N=2 signals; in/near ERBB2/HER2 and FSHB). Follow-up was performed in three independent studies of clinically validated PCOS cases and control women: deCODE, Rotterdam and Boston (see Supplementary Table 1 and Supplementary Note for details and parameters of follow-up studies). Separate follow-up analyses were performed using PCOS case definitions either by Rotterdam 2003 criteria1 (1,875 cases from Rotterdam and deCODE) or by NIH criteria2 (861 cases from Boston and deCODE). Final association test statistics were produced from a combined meta-analysis of 7,229 cases and 181,645 controls across non-overlapping discovery and follow-up (2,045 cases and 98,886 controls) samples; as the two PCOS groups in deCODE include overlapping cases, only deCODE cases defined by NIH criteria were included in this combined meta-analysis. The follow-up studies were approved by local research ethics committees and all participants provided informed consent.
Mendelian randomization analyses
Mendelian randomization is an analytical method to infer the unconfounded causal relationship between an exposure trait and an outcome, using genetic variants that are associated with the exposure trait and do not influence the outcome by other unrelated biological pathways (‘pleitropy’)21. In both the 23andMe and Rotterdam studies, we approximated weighted multiple allele scores (single variables summarizing multiple genetic variants associated with a risk factor, as described by Dastani et al.42), to represent genetic instrumental variables for 15 traits (birth weight, BMI, height, age at menarche, age at menopause, dehydroepiandrosterone sulphate, SHBG, total cholesterol, high-density lipoprotein cholesterol, low-protein lipoprotein cholesterol, triglycerides, systolic and diastolic blood pressure, insulin resistance and insulin secretion) based on reported GWAS signals for those traits. Each score was calibrated to a 1-s.d. change in the exposure trait, using the published effect estimates of individual alleles on those traits in the replication stages of those GWAS reports (Supplementary Table 3). To account for the multiple traits tested, we set a corrected P-value threshold (0.05/15=0.0033) to indicate statistically significant associations. To test for pleiotropy, which can invalidate inferences from Mendelian randomization, we performed sensitivity analyses to examine the consistency in causal estimates derived from individual SNPs.
Serum AMH concentrations
The cumulative influence of PCOS-associated variants on childhood serum AMH concentrations, a marker of ovarian primordial follicle pool size4, was estimated by analysis of data in 1,455 girls (aged 15 years) from the ALSPAC study43. Serum AMH concentrations (ng ml−1) were natural log transformed before analysis in an additive linear regression framework.
Tests for positive selection
Allelic variants that increase the reproductive fitness of their carriers should become more prevalent in the population. The resulting genomic characteristics of strong recent positive selection include low haplotype diversity, high linkage disequilibrium and marked shifts in allele frequency between populations. However, there is often poor consistency between signals identified from available tests38,39. We therefore looked for evidence of selection at the ten PCOS loci in Table 1, using various strategies.
We investigated whether any of the lead SNPs overlapped with signals of positive selection identified in 1000 Genomes data using the composite of multiple signals test44. None of the lead PCOS SNPs lies in any of the 424 non-overlapping regions with evidence of positive selection, a total of 19 Mb of sequence (http://www.broadinstitute.org/mpg/cmsviewer/download/cms_localized_regions_062712.txt). Three of the ten signals lie within 1 Mb of one of these regions (a total of 726 Mb of sequence), which is not more than expected by chance (P=0.56 assuming an accessible genome length of 2.6 Gb).
We tested whether the lead PCOS SNPs are more differentiated across populations compared to with randomly chosen loci, using the test described by Berg and Coop45, and Omni chip data from phase 1 of the 1000 Genomes Project46 as a reference panel (ftp://ftp-trace.ncbi.nih.gov/1000genomes/ftp/phase1/analysis_results/supporting/omni_haplotypes/). As only two of the ten PCOS SNPs were genotyped by the Omni chip, we added the remaining eight SNPs from the sequence data. Using 10,000 bootstrap replicates of SNP frequency matched in 20 bins, we find no evidence of polygenic selection in European (P=0.38), Asian (P=0.37), or combined European and Asian (P=0.42) populations.
We also tested PCOS susceptibility variants with minor allele frequency >0.2 using the integrated haplotype score38, which measures the difference in haplotype homozygosity associated with the ancestral and derived alleles, and the derived intra-allelic nucleotide diversity test38, which measures the differences in nucleotide diversity associated with the ancestral and derived alleles. We find no significant test statistics (P<0.01).
Pathway analyses
MAGENTA (https://www.broadinstitute.org/mpg/magenta/) was used to test for enrichment of genome-wide SNP associations with PCOS in pre-defined biological pathways (Gene Ontology, PANTHER, KEGG and Ingenuity) using the full discovery data set. MAGENTA implements a gene-set enrichment analysis-based approach, where each gene throughout the genome is mapped to a single index SNP with the lowest P-value within a 110-kb upstream and 40-kb downstream window. This P-value, representing a gene score, is then corrected for confounding factors such as gene size, SNP density and linkage disequilibrium (LD)-related properties in a regression model. Genes within the human leukocyte antigen region were excluded from analysis, owing to difficulties in accounting for gene density and LD patterns. Each gene is then ranked by its adjusted gene score. At a given significance threshold (95th or 75th percentiles of all gene scores), the observed number of gene scores in a given pathway, with a ranked score above the specified threshold percentile, is calculated. This observed statistic is then compared with 1,000,000 randomly permuted pathways of identical size. This generates an empirical gene-set enrichment analysis P-value for each pathway. In total, 2,529 pathways were tested for enrichment of multiple modest associations with PCOS. Significant pathways are indicated by a false discovery rate <0.05 in either model (95th or 75th percentiles).
Additional information
How to cite this article: Day, F. R. et al. Causal mechanisms and balancing selection inferred from genetic associations with polycystic ovary syndrome. Nat. Commun. 6:8464 doi: 10.1038/ncomms9464 (2015).
References
Rotterdam ESHRE/ASRM-Sponsored PCOS Consensus Workshop Group. Revised 2003 consensus on diagnostic criteria and long-term health risks related to polycystic ovary syndrome (PCOS). Hum. Reprod. 19, 41–47 (2004).
Zawadzki, J. & Dunaif, A. in Polycystic Ovary Syndrome eds Dunaif A. G. J., Haseltine F. 377–384Blackwell Scientific (1992).
Amsterdam ESHRE/ASRM-Sponsored 3rd PCOS Consensus Workshop Group. Consensus on women's health aspects of polycystic ovary syndrome (PCOS). Hum. Reprod. 27, 14–24 (2012).
Corbett, S. & Morin-Papunen, L. The polycystic ovary syndrome and recent human evolution. Mol. Cell. Endocrinol. 373, 39–50 (2013).
Domecq, J. P. et al. Lifestyle modification programs in polycystic ovary syndrome: systematic review and meta-analysis. J. Clin. Endocrinol. Metab. 98, 4655–4663 (2013).
Moran, L. J., Hutchison, S. K., Norman, R. J. & Teede, H. J. Lifestyle changes in women with polycystic ovary syndrome. Cochrane Database Syst. Rev. CD007506 (2011).
Dunaif, A. Insulin resistance and the polycystic ovary syndrome: mechanism and implications for pathogenesis. Endocr. Rev. 18, 774–800 (1997).
Wild, R. A. et al. Assessment of cardiovascular risk and prevention of cardiovascular disease in women with the polycystic ovary syndrome: a consensus statement by the Androgen Excess and Polycystic Ovary Syndrome (AE-PCOS) Society. J. Clin. Endocrinol. Metab. 95, 2038–2049 (2010).
Legro, R. S. et al. Diagnosis and treatment of polycystic ovary syndrome: an Endocrine Society clinical practice guideline. J. Clin. Endocrinol. Metab. 98, 4565–4592 (2013).
Barber, T. M. & Franks, S. Genetics of polycystic ovary syndrome. Front. Horm. Res. 40, 28–39 (2013).
Chen, Z. J. et al. Genome-wide association study identifies susceptibility loci for polycystic ovary syndrome on chromosome 2p16.3, 2p21 and 9q33.3. Nat. Genet. 43, 55–59 (2011).
Shi, Y. et al. Genome-wide association study identifies eight new risk loci for polycystic ovary syndrome. Nat. Genet. 44, 1020–1025 (2012).
Goodarzi, M. O. et al. Replication of association of DENND1A and THADA variants with polycystic ovary syndrome in European cohorts. J. Med. Genet. 49, 90–95 (2012).
Welt, C. K. et al. Variants in DENND1A are associated with polycystic ovary syndrome in women of European ancestry. J. Clin. Endocrinol. Metab. 97, E1342–E1347 (2012).
Mutharasan, P. et al. Evidence for chromosome 2p16.3 polycystic ovary syndrome susceptibility locus in affected women of European ancestry. J. Clin. Endocrinol. Metab. 98, E185–E190 (2013).
Louwers, Y. V., Stolk, L., Uitterlinden, A. G. & Laven, J. S. Cross-ethnic meta-analysis of genetic variants for polycystic ovary syndrome. J. Clin. Endocrinol. Metab. 98, E2006–E2012 (2013).
Saxena, R. et al. Han Chinese polycystic ovary syndrome risk variants in women of European ancestry: relationship to FSH levels and glucose tolerance. Hum. Reprod. 30, 1454–1459 (2015).
Abbott, D. H., Dumesic, D. A. & Franks, S. Developmental origin of polycystic ovary syndrome - a hypothesis. J. Endocrinol. 174, 1–5 (2002).
Escobar-Morreale, H. F., Luque-Ramirez, M. & San Millan, J. L. The molecular-genetic basis of functional hyperandrogenism and the polycystic ovary syndrome. Endocr. Rev. 26, 251–282 (2005).
Mulders, A. G. et al. Changes in anti-Mullerian hormone serum concentrations over time suggest delayed ovarian ageing in normogonadotrophic anovulatory infertility. Hum. Reprod. 19, 2036–2042 (2004).
Burgess, S., Butterworth, A., Malarstig, A. & Thompson, S. G. Use of Mendelian randomisation to assess potential benefit of clinical intervention. BMJ 345, e7325 (2012).
Stolk, L. et al. Meta-analyses identify 13 loci associated with age at menopause and highlight DNA repair and immune pathways. Nat. Genet. 44, 260–268 (2012).
Tang, T., Lord, J. M., Norman, R. J., Yasmin, E. & Balen, A. H. Insulin-sensitising drugs (metformin, rosiglitazone, pioglitazone, D-chiro-inositol) for women with polycystic ovary syndrome, oligo amenorrhoea and subfertility. Cochrane Database Syst. Rev. 5, CD003053 (2012).
Moran, L. J. et al. Dietary composition in the treatment of polycystic ovary syndrome: a systematic review to inform evidence-based guidelines. J. Acad. Nutr. Diet. 113, 520–545 (2013).
Scott, R. A. et al. Common genetic variants highlight the role of insulin resistance and body fat distribution in type 2 diabetes, independently of obesity. Diabetes 63, 4378–4387 (2014).
Ding, E. L. et al. Sex hormone-binding globulin and risk of type 2 diabetes in women and men. N. Engl. J. Med. 361, 1152–1163 (2009).
Daan, N. M. et al. Cardiovascular and metabolic profiles amongst different polycystic ovary syndrome phenotypes: who is really at risk? Fertil. Steril. 102, 1444–1451 (2014).
Costello, M., Shrestha, B., Eden, J., Sjoblom, P. & Johnson, N. Insulin-sensitising drugs versus the combined oral contraceptive pill for hirsutism, acne and risk of diabetes, cardiovascular disease, and endometrial cancer in polycystic ovary syndrome. Cochrane Database Syst. Rev. CD005552 (2007).
Ciardiello, F. & Tortora, G. EGFR antagonists in cancer treatment. N. Engl. J. Med. 358, 1160–1174 (2008).
Park, J. Y. et al. EGF-like growth factors as mediators of LH action in the ovulatory follicle. Science 303, 682–684 (2004).
Jamnongjit, M., Gill, A. & Hammes, S. R. Epidermal growth factor receptor signaling is required for normal ovarian steroidogenesis and oocyte maturation. Proc. Natl Acad. Sci. USA 102, 16257–16262 (2005).
Sheng, Q. & Liu, J. The therapeutic potential of targeting the EGFR family in epithelial ovarian cancer. Br. J. Cancer 104, 1241–1245 (2011).
Davies, S. et al. High incidence of ErbB3, ErbB4, and MET expression in ovarian cancer. Int. J. Gynecol. Pathol. 33, 402–410 (2014).
Hurtado, A. et al. Regulation of ERBB2 by oestrogen receptor-PAX2 determines response to tamoxifen. Nature 456, 663–666 (2008).
Chen, D. J. et al. Two follicle-stimulating hormone receptor polymorphisms and polycystic ovary syndrome risk: a meta-analysis. Eur. J. Obstet. Gynecol. Reprod. Biol. 182C, 27–32 (2014).
Mason, H. & Franks, S. Local control of ovarian steroidogenesis. Baillieres Clin. Obstet. Gynaecol. 11, 261–279 (1997).
Burt Solorzano, C. M. et al. Neuroendocrine dysfunction in polycystic ovary syndrome. Steroids 77, 332–337 (2012).
Fagny, M. et al. Exploring the occurrence of classic selective sweeps in humans using whole-genome sequencing data sets. Mol. Biol. Evol. 31, 1850–1868 (2014).
Kemper, K. E., Saxton, S. J., Bolormaa, S., Hayes, B. J. & Goddard, M. E. Selection for complex traits leaves little or no classic signatures of selection. BMC Genomics 15, 246 (2014).
Perry, J. R. et al. DNA mismatch repair gene MSH6 implicated in determining age at natural menopause. Hum. Mol. Genet. 23, 2490–2497 (2014).
Tung, J. Y. et al. Efficient replication of over 180 genetic associations with self-reported medical data. PLoS One 6, e23473 (2011).
Dastani, Z. et al. Novel loci for adiponectin levels and their influence on type 2 diabetes and metabolic traits: a multi-ethnic meta-analysis of 45,891 individuals. PLoS. Genet. 8, e1002607 (2012).
Anderson, E. L. et al. Anti-mullerian hormone is not associated with cardiometabolic risk factors in adolescent females. PLoS One 8, e64510 (2013).
Grossman, S. R. et al. Identifying recent adaptations in large-scale genomic data. Cell 152, 703–713 (2013).
Berg, J. J. & Coop, G. A population genetic signal of polygenic adaptation. PLoS. Genet. 10, e1004412 (2014).
1000 Genomes Project Consortium. et al. An integrated map of genetic variation from 1,092 human genomes. Nature 491, 56–65 (2012).
Acknowledgements
This work was supported by the Medical Research Council (U106179472, MC_U106179472, U106179471 and MC_U106179471) and the National Human Genome Research Institute of the National Institutes of Health (grant number R44HG006981 to 23andMe). The UK Medical Research Council and Wellcome Trust (092731), together with the University of Bristol, provide core support for the ALSPAC study. AMH assays in ALSPAC were funded with a grant from the US National Institute of Health (R01 DK077659). DAL works in a unit that receives funding from the University of Bristol and the UK Medical Research Council (MC_UU_12013/5). We thank the customers and employees of 23andMe for making this work possible. We are extremely grateful to all of the families who took part in the participating studies. We thank the ALSPAC team, which includes interviewers, computer and laboratory technicians, clerical workers, research scientists, volunteers, managers, receptionists and nurses, and also midwives for supporting recruitment.
Author information
Authors and Affiliations
Contributions
All authors read and approved the manuscript. Analysis: F.R.D., L.S., U.S., L.B., G.L., I.M., R.A.S. and J.R.B.P. Phenotype and genotyping: L.S., U.S., D.A.H., J.Y.T., R.S., A.B., D.B.D., B.V.H., D.A.L., W.L.M., Y.L., C.M., S.R., P.S., A.G.U., U.T., C.W., K.S. and J.S.E.L. Study design: D.A.H., J.Y.T., D.A.L., A.G.U., U.T., C.W., K.S., J.S.E.L., J.R.B.P., N.J.W. and K.K.O.
Corresponding authors
Ethics declarations
Competing interests
D.A.H. and J.Y.T. are employees of and own stock or stock options in 23andMe, Inc. The remaining authors declare no conflict of interest.
Supplementary information
Supplementary Information
Supplementary Figures 1-3, Supplementary Tables 1-2, Supplementary Notes 1-2 and Supplementary References (PDF 739 kb)
Supplementary Data 1
Variants used in Mendelian Randomisation analysis (XLSX 60 kb)
Rights and permissions
This work is licensed under a Creative Commons Attribution 4.0 International License. The images or other third party material in this article are included in the article’s Creative Commons license, unless indicated otherwise in the credit line; if the material is not included under the Creative Commons license, users will need to obtain permission from the license holder to reproduce the material. To view a copy of this license, visit http://creativecommons.org/licenses/by/4.0/
About this article
Cite this article
Day, F., Hinds, D., Tung, J. et al. Causal mechanisms and balancing selection inferred from genetic associations with polycystic ovary syndrome. Nat Commun 6, 8464 (2015). https://doi.org/10.1038/ncomms9464
Received:
Accepted:
Published:
DOI: https://doi.org/10.1038/ncomms9464
This article is cited by
-
Susceptibility loci identified in Han Chinese influence genetic predisposition of PCOS in Indian women
Molecular Biology Reports (2024)
-
Induced Pluripotent Stem Cells as a Possible Approach for Exploring the Pathophysiology of Polycystic Ovary Syndrome (PCOS)
Stem Cell Reviews and Reports (2024)
-
Body mass index stratified meta-analysis of genome-wide association studies of polycystic ovary syndrome in women of European ancestry
BMC Genomics (2024)
-
Adiposity and lipid metabolism indicators mediate the adverse effect of glucose metabolism indicators on oogenesis and embryogenesis in PCOS women undergoing IVF/ICSI cycles
European Journal of Medical Research (2023)
-
Genetic evidence for the causal association between type 1 diabetes and the risk of polycystic ovary syndrome
Human Genomics (2023)
Comments
By submitting a comment you agree to abide by our Terms and Community Guidelines. If you find something abusive or that does not comply with our terms or guidelines please flag it as inappropriate.