Abstract
Background:
The objective of this study is to construct a preoperative nomogram predicting lymph node metastasis (LNM) in early-cervical cancer patients.
Methods:
Between 2009 and 2012, 493 early-cervical cancer patients received hysterectomy and pelvic/para-aortic lymphadenectomy. Patients who were diagnosed during 2009–2010 were assigned to a model-development cohort (n=304) and the others were assigned to a validation cohort (n=189). A multivariate logistic model was created from preoperative clinicopathologic data, from which a nomogram was developed and validated. A predicted probability of LNM<5% was defined as low risk.
Results:
Age, tumour size assessed by magnetic resonance imaging, and LNM assessed by positron emission tomography/computed tomography were independent predictors of nodal metastasis. The nomogram incorporating these three predictors demonstrated good discrimination and calibration (concordance index=0.878; 95% confidence interval (CI), 0.833−0.917). In the validation cohort, the discrimination accuracy was 0.825 (95% CI, 0.736−0.895). In the model-development cohort, 34% of them were classified as low risk and negative predictive value (NPV) was 99.0%. In the validation cohort, 38% were identified as low risk and NPV was 95.8%. Integrating the model-development and validation cohorts, negative likelihood ratio was 0.094 (95% CI, 0.036−0.248).
Conclusion:
A robust nomogram predicting LNM in early cervical cancer was developed. This model may improve clinical trial design and help physicians to decide whether lymphadenectomy should be performed.
Similar content being viewed by others
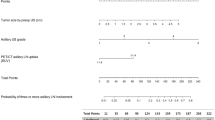
Main
In 2008, cervical cancer was the third most frequently diagnosed cancer and the fourth leading cause of cancer death in women worldwide (Jemal et al, 2011). In Korea, it is the most common female genital malignancy, accounting for 9.8% of newly diagnosed malignancies in women (Lee et al, 2012, 2013).
The definitive primary treatment for early cervical cancer consists of concurrent chemoradiation or radical hysterectomy with pelvic and/or para-aortic lymphadenectomy (Landoni et al, 1997). In selected cases, radical trachelectomy with pelvic and/or para-aortic lymphadenectomy can be used to preserve fertility (Diaz et al, 2008). Surgery is widely selected, because it removes the primary disease, preserves ovarian function, and permits better targeting of adjuvant therapy through accurate surgical staging. Although not included in the FIGO (International Federation of Gynecology and Obstetrics) staging system, lymph node metastasis (LNM) is a significant prognostic factor in early cervical cancer. Thus, information on the lymph node (LN) status is necessary to determine the treatment strategy (Landoni et al, 1997). Lymphadenectomy has been used to assess LN status in early cervical cancer; however, the therapeutic role of lymphadenectomy is debated (Morice et al, 1999; Pieterse et al, 2007; Shah et al, 2011). Lymph node metastasis develops in only 15−25% of early-cervical cancer patients (Berek and Novak, 2012); a significant portion of patients undergo lymphadenectomy unnecessarily and suffer from procedure-related morbidity. Much effort has been made to reduce the lymphadenectomy-related morbidity in these patients. Recent data suggest that sentinel LN (SLN) biopsy could be established as a method of LN staging in patients with early cervical cancer (Bats et al, 2011; Lecuru et al, 2011). However, the technique has not yet been fully validated and it involves surgery under general anaesthesia (Hertel, 2010; Koh et al, 2013). Therefore, it would be very useful to identify patients with a low likelihood of LNM preoperatively. Furthermore, the ability to identify low-risk patients may be useful in clinical trial design. This study aimed to construct and validate a nomogram that could predict LNM in early-cervical cancer patients and to use the nomogram to identify patients at a low risk for LNM.
Materials and methods
Patients
After obtaining institutional review board approval (S2013-0643-0001), patients were identified from a computerised database of cervical cancer patients who underwent surgery at the Asan Medical Center between March 2009 and December 2012. The inclusion criteria were as follows: pathologically confirmed cervical cancer, age >18 years and <80 years, a clinical diagnosis of FIGO stage IA2-IIA disease, radical hysterectomy (type II or III) or radical trachelectomy with pelvic and/or para-aortic lymphadenectomy, pelvic magnetic resonance imaging (MRI) and fluorine-18 fluorodeoxyglucose positron emission tomography/computed tomography (PET/CT) performed within 3 weeks before lymphadenectomy, and no history of chemotherapy or radiotherapy. From a database of 576 patients with FIGO stage IA2-IIA cervical cancer, 493 patients satisfied the eligibility criteria. Before analysis, patients were allocated to a model-development set (n=304; March 2009–August 2011) and a validation set (n=189; September 2011—December 2012) based on surgery dates (Figure 1).
Patients received exact staging, including physical examination, complete blood count, urinalysis, liver function test, serum squamous cell carcinoma antigen (SCC Ag), chest X-ray, intravenous pyelography, and sigmoidoscopy. Demographic and clinicopathologic data were obtained from medical records. Bilateral pelvic and/or para-aortic lymphadenectomy was performed as described previously (Park et al, 2010).
Preoperative assessment
To identify variables predicting LNM, the following factors were included based on data from previous studies (Kodama et al, 1991; Takeda et al, 2002; Grigiene et al, 2007): age, FIGO stage, histology, parametrial invasion, tumour size, potential LNM based on MRI, potential LNM based on PET/CT, and pretreatment serum SCC Ag. Tumour size and parametrial invasion were assessed by MRI. The MRI criteria for metastatic LN included a short axis diameter (⩾1 cm), the presence of necrotic portions, lobulated or speculated margins, heterogeneous enhancement, or loss of fatty hilum. The settings and conditions of the PET/CT scanning process were described in Supplementary Data (online only). Scans were interpreted based on the criteria of the International Harmonization Project in Lymphoma (Juweid et al, 2007). The results of preoperative biopsy, pelvic MRI, and whole-body PET/CT were interpreted blindly without knowledge of LN involvement by pathologists, radiologists, and nuclear medicine physicians, respectively.
Statistical analysis
Age, tumour size, and serum SCC Ag were considered as continuous variables. FIGO stage, histology, parametrial invasion, LNM assessed by MRI, and LNM assessed by PET/CT were considered as categorical variables. The histological subtype was classified as SCC or non-SCC.
The nomogram was built as described previously (Iasonos et al, 2008; Shim et al, 2013). Before developing the nomogram, low risk was defined as a predicted probability of developing LNM of <5% (Lyman et al, 2005; Berek and Hacker, 2010). To develop a robust and well-calibrated nomogram predicting the risk of LNM, a logistic regression model was built using a development cohort of 304 patients and validated with a cohort of 189 patients. Grouping of categorical variables was done a priori. A logistic regression model was developed to predict LNM with a bootstrap method. First, the bivariate relationship between risk factors and LNM was assessed in the model-development cohort. Next, the predictive values obtained by univariate analyses were tested by bootstrap resampling, in which a logistic regression model with a backward elimination procedure included 1000 repetitions. The criterion for inclusion of predictors in the final logistic model was a 50% relative frequency of selection by bootstrap resampling. To assess the fit of the model, the concordance index was used to measure discrimination by calculating the area under the receiver operating characteristics curve, and the Hosmer–Lemeshow test was employed to assess calibration. For external validation, the model was applied on a validation cohort of 189 patients. Using the same methods, the discrimination and calibration of the model were tested. Finally, the LNM rate of groups previously identified as low risk by the nomogram was assessed in the development and validation cohorts. The negative likelihood ratio (LR−) was calculated using the following formula: LR−=(1−sensitivity)/specificity. On the basis of Bayes’ theorem, the LR− was converted into the negative post-test probability (i.e., the negative predictive value (NPV)) at an appointed prevalence of LNM (Bayes, 1991). All analyses were performed using SPSS version 19.0 (SPSS, Chicago, IL, USA) and R version 3.0.0 (http://cran.r-project.org/mirrors.html). A P-value <0.05 was considered significant.
Results
Patient populations
The characteristics of the model-development and validation cohorts are summarised in Table 1. The LNM frequency for both the model-development and the validation cohort was 20.1% (6 out of 304 and 38 out of 189, respectively).
Model development for the prediction of LNM
Table 2 shows the results of the logistic regression model for predicting LNM. Univariate analysis showed that age, stage, SCC Ag, tumour size measured by MRI, and LNM assessed by PET/CT were significantly associated with LNM. After a bootstrap resampling procedure with 1000 repetitions, the final model yielded three statistically significant predictors: age, tumour size, and LNM by PET/CT. A nomogram was constructed based on this logistic regression model (Figure 2). The point value assigned to each factor was proportional to the hazard ratio that was derived from its own β-coefficients by regression analysis. Internal validation was performed using the bootstrapping correction technique. After 1000 repetitions, the bootstrap-corrected concordance index for the model was 0.878 (95% confidence interval (CI), 0.833−0.917) (Figure 3A). In the validation cohort, the discrimination accuracy of the model was 0.825 (95% CI, 0.736−0.895; Figure 3B). Figure 3C and D show the calibration plots of the nomogram for the model-development and validation cohorts, respectively. The dashed line indicates the performance of an ideal nomogram and the solid line indicates the performance of the present nomogram. The filled dots were derived from a subcohort of the present database. When plotting the probabilities of LNM predicted by the nomogram against the actual probabilities, the calibration curve lay close to the dashed line. The Hosmer–Lemeshow test yielded a P-value of 0.566 for the model-development cohort, showing that the nomogram was well fitted. For the validation cohort, the nomogram also fitted the data well (P=0.411, Hosmer–Lemeshow test).
Nomogram predicting LNM in patients with early cervical cancer.The nomogram incorporates three variables. Points for each prognostic variable were allocated according to the scale shown here. A total score was determined by adding individual parameter points and used to calculate the predicted probability of LNM. A total score of 38.7 was assigned a value of 0.05 and was defined as low risk for nodal metastasis. Abbreviations: MRI=magnetic resonance imaging; PET/CT=positron emission tomography/computed tomography.
Performance of the nomogram for predicting LNM in patients with early cervical cancer. (A) After 1000 repetitions, the bootstrap-corrected concordance index of the model was 0.878 (95% CI, 0.833−0.917) in the model-development cohort. (B) In the validation cohort, the bootstrap-corrected concordance index of the model was 0.825 (95% CI, 0.736−0.895). (C) Calibration plots of the nomogram for the model-development cohort. (D) Calibration plots of the nomogram for the validation cohort. Dashed line=ideal reference value where predicted probabilities match actual probabilities of LNM; solid line indicates performance of the current nomogram; filled dots indicate calculations from a subcohort of the present database; vertical bar indicates 95% CI.
Identification of patients at low risk of nodal metastasis
The low-risk group was predefined as having a predicted probability of LNM of <5%. The nomogram classified 102 out of 304 patients (34%) in the model-development cohort as low risk. In that group, the predicted probability of LNM was 3.20% and the actual metastasis rate was 0.98% (1 out of 102). Thirteen patients were FIGO stage IA2, 76 were IB1, and three were IIA1. Only one patient with stage IB1 had LNM. In the validation cohort, 72 out of 189 patients (38%) were classified as low risk. In that group, the predicted probability of LNM was 3.21% and the actual metastasis rate was 4.17% (3 out of 72). After combining the model-development and validation cohorts, the LR− for low-risk criteria was estimated. The LR− of the study population was 0.094 (95% CI, 0.036−0.248). This LR− can be converted into a NPV of 0.984 (95% CI, 0.958−0.994) based on Bayes’ theorem, using a nodal metastasis prevalence of 15%.
Table 3 shows a brief description of the four patients with metastatic LN falsely identified as low risk. There is discrepancy in tumour size between preoperative MRI and postoperative pathologic evaluation in these patients. The four patients received adjuvant concurrent chemoradiation and none have recurred to date.
Discussion
The present study suggests that the risk of LNM may be determined before surgery using preoperative variables, including age, tumour size assessed by MRI, and LNM assessed by PET/CT. Incorporating these three variables, a nomogram for preoperative risk assessment of nodal metastasis was constructed, in which patients with predicted probability of LNM<5% were defined as a low-risk group. In the model-development and validation cohorts, the actual LNM rate of the low-risk group defined by our nomogram was remarkably low. Hence, lymphadenectomy may not be performed in patients classified as low risk by this nomogram.
The most important benefit of the nomogram is that risk can be assessed by non-invasive procedures before surgery. Individualised prediction based on the nomogram, which incorporates preoperative variables, could help inform decision-making by physicians and patients. Although lymphadenectomy is the standard criterion to evaluate the nodal status of cervical cancer, the therapeutic value of this procedure is debated in the absence of level 1 evidence (Morice et al, 1999; Pieterse et al, 2007; Shah et al, 2011). Theoretically, after complete LN removal, patients who are truly negative for LNM will not benefit from lymphadenectomy (Sakuragi, 2007). As the LNM rate in early cervical cancer is 15−25%, ∼80% of the patients might receive little benefit from lymphadenectomy and less aggressive surgery may be proposed.
Lymphadenectomy may result in morbidities such as vessel injuries, nerve injuries, infection, lymphocysts, and lymphoedema (Matsuura et al, 2006). Efforts to identify node-negative patients by SLN techniques have decreased the frequency of these complications. Recent data suggest that SLN biopsy may decrease the demand for pelvic lymphadenectomy in early-cervical cancer patients (Lecuru et al, 2011). However, SLN biopsy is not routinely performed, because there is a lack of consistent data on the intraoperative pathological evaluation, the role of micrometastasis in LN, and procedure standards (Hertel, 2010; Bats et al, 2011).
With respect to diagnostic performance, the prediction accuracy of our nomogram was comparable to recently published data from a prospective, multicentre study of SLN biopsy. The SENTICOL study showed that given a LNM prevalence of 17.9%, the detection sensitivity was 92.0% and the NPV was 98.2% (Lecuru et al, 2011). After combining the model-development and validation cohorts in our study, the sensitivity and NPV were 96.0% (95 out of 99) and 97.7% (170 out of 174), respectively, with a LNM prevalence of 20.1% (99 out of 493). Assuming a prevalence of 17.9%, as in the SENTICOL study, the NPV of our low-risk criteria would be 0.980 (95% CI, 0.949−0.992), which is similar to the performance observed in the SENTICOL study. We believe that we can more accurately identify the node-negative patients by combined use of SLN biopsy and the nomogram.
Regarding the radiologic assessment of LNM, previous studies indicate that PET/CT may be superior to CT and MRI (Choi et al, 2010; Kidd et al, 2010). A recent meta-analysis of 41 studies reported that PET or PET/CT had an overall higher diagnostic performance than CT or MRI for LNM detection in cervical cancer patients (Choi et al, 2010), PET or PET/CT had the highest pooled sensitivity (82%) and specificity (95%), whereas CT had 50% and 92%, and MRI had 56% and 91%, respectively. In the present study, PET/CT improved the detection sensitivity and specificity to 72.1% and 84.4%, respectively, whereas MRI had a 55.7% sensitivity and 76.1% specificity. The model was also tested using the LN status determined by MRI instead of PET/CT. The variables incorporated in the multivariate analysis were age, tumour size, SCC Ag (categorical), and LN status assessed by MRI. The concordance indices for the MRI-based model were 0.811 (95% CI, 0.753−0.863) and 0.770 (95% CI, 0.682−0.846) for the model-development cohort and validation cohort, respectively (Table 4). Although the observed metastasis rates of the predicted low-risk group using the MRI-based model were 0% (0 out of 46) and 4.2% (1 out of 24) in the development and validation cohorts, respectively, the MRI-based model predicted rates of 15.1% (46 out of 304) in the model-development cohort and 12.7% (24 out of 189) in the validation cohort. Therefore, we chose the multivariate model based on PET/CT.
The four patients incorrectly classified as low risk by our nomogram had small metastatic LN (<7 mm in diameter). Although PET/CT could be useful in detecting LN metastases, the sensitivity of PET/CT for detecting microscopic LN metastases was much lower (34−53%) in patients with negative morphological imaging (Wright et al, 2005; Kang et al, 2010). Sironi et al (2006) suggested that 5 mm was the size threshold for the detection of metastatic LN by PET/CT. Recently, emerging modalities such as hybrid PET/MRI and MRI using nanoparticle contrast agents have been shown to have higher diagnostic value than PET/CT for detecting LN metastases in cervical cancer patients (Rockall et al, 2005; Kim et al, 2009). The risk of LNM may be more accurately predicted by incorporating these modalities into the nomogram. In addition, the SLN concept could be used to confirm the results of the nomogram, particularly the case of small tumours (up to stage IB1). Thus, false-negative patients identified by the nomogram would be diagnosed as metastasised by SLN biopsy with a high probability.
These results do not indicate that routine lymphadenectomy is beneficial for non-low-risk patients. Although the actual LNM rate was 29.8% in the non-low-risk group, the therapeutic value of lymphadenectomy in this group must be evaluated in clinical trials. In addition, the role of SLN biopsy and the prognostic value of metastatic nodal resection should be assessed in this risk group and not in the entire population. Our study indicates that patients defined as low risk by our nomogram should be excluded from such trials.
This study has limitations inherent to retrospective chart reviews. Known factors associated with LNM, such as detection of human papilloma virus by genotyping and LVSI, were not included in the present study (van Nagell et al, 1978; Garzetti et al, 1998). Notably, three of the four patients incorrectly classified as low risk had LVSI on final pathologic examination. LVSI could not be assessed in the vast majority of the patients in our study population before surgery, because punch biopsies, not cone biopsies, were performed in 52.7% of the patients (260 out of 493). Importantly, the FIGO classification does not include LVSI, because pathologists do not always agree on whether LVSI is present (Pecorelli et al, 2009). If these variables can be accurately estimated and incorporated into the present model, they may increase its performance index. In addition, although we validated the model using external data from our institution and the model was well fitted, the model must be validated using data from other institutions to assess its generalisability. A multicentre prospective observational study conducted by the Korean Gynecologic Oncology Group will be used to validate the prediction model for LNM in early cervical cancer.
In conclusion, a robust model incorporating preoperative variables to predict LNM in patients with early cervical cancer was developed and validated. The model accurately identifies patients at low risk of LNM. This new tool may be useful to clinicians and patients when deciding whether lymphadenectomy should be performed, and may be useful in designing clinical trials. A prospective validation study in a heterogeneous population will be undertaken to assess the predictive accuracy of the model.
Change history
07 January 2014
This paper was modified 12 months after initial publication to switch to Creative Commons licence terms, as noted at publication
References
Bats AS, Buenerd A, Querleu D, Leblanc E, Darai E, Morice P, Marret H, Gillaizeau F, Mathevet P, Lecuru F SENTICOL Collaborative Group (2011) Diagnostic value of intraoperative examination of sentinel lymph node in early cervical cancer: a prospective, multicenter study. Gynecol Oncol 123 (2): 230–235.
Bayes T (1991) An essay towards solving a problem in the doctrine of chances. 1763. MD Comput 8 (3): 157–171.
Berek JS, Hacker NF (2010) Berek & Hacker’s Gynecologic Oncology. Wolters Kluwer/Lippincott Williams & Wilkins Health: Philadelphia.
Berek JS, Novak E (2012) Berek & Novak’s Gynecology. Wolters Kluwer Health/Lippincott Williams & Wilkins: Philadelphia.
Choi HJ, Ju W, Myung SK, Kim Y (2010) Diagnostic performance of computer tomography, magnetic resonance imaging, and positron emission tomography or positron emission tomography/computer tomography for detection of metastatic lymph nodes in patients with cervical cancer: meta-analysis. Cancer Sci 101 (6): 1471–1479.
Diaz JP, Sonoda Y, Leitao MM, Zivanovic O, Brown CL, Chi DS, Barakat RR, Abu-Rustum NR (2008) Oncologic outcome of fertility-sparing radical trachelectomy versus radical hysterectomy for stage IB1 cervical carcinoma. Gynecol Oncol 111 (2): 255–260.
Garzetti GG, Ciavattini A, Lucarini G, Goteri G, Menso S, De Nictolis M, Romanini C, Biagini G (1998) The role of human papillomavirus DNAs in cervical carcinoma and risk of lymph node metastasis: association with 72-kilodalton metalloproteinase immunostaining. Cancer 82 (5): 886–892.
Grigiene R, Valuckas KP, Aleknavicius E, Kurtinaitis J, Letautiene SR (2007) The value of prognostic factors for uterine cervical cancer patients treated with irradiation alone. BMC Cancer 7: 234.
Hertel H (2010) Cervical cancer and the role of lymph node staging cons sentinel concept. Int J Gynecol Cancer 20 (11 Suppl 2): S37–S38.
Iasonos A, Schrag D, Raj GV, Panageas KS (2008) How to build and interpret a nomogram for cancer prognosis. J Clin Oncol 26 (8): 1364–1370.
Jemal A, Bray F, Center MM, Ferlay J, Ward E, Forman D (2011) Global cancer statistics. CA Cancer J Clin 61 (2): 69–90.
Juweid ME, Stroobants S, Hoekstra OS, Mottaghy FM, Dietlein M, Guermazi A, Wiseman GA, Kostakoglu L, Scheidhauer K, Buck A, Naumann R, Spaepen K, Hicks RJ, Weber WA, Reske SN, Schwaiger M, Schwartz LH, Zijlstra JM, Siegel BA, Cheson BD Imaging Subcommittee of International Harmonization Project in L (2007) Use of positron emission tomography for response assessment of lymphoma: consensus of the Imaging Subcommittee of International Harmonization Project in Lymphoma. J Clin Oncol 25 (5): 571–578.
Kang S, Kim SK, Chung DC, Seo SS, Kim JY, Nam BH, Park SY (2010) Diagnostic value of (18)F-FDG PET for evaluation of paraaortic nodal metastasis in patients with cervical carcinoma: a metaanalysis. J Nucl Med 51 (3): 360–367.
Kidd EA, Siegel BA, Dehdashti F, Rader JS, Mutch DG, Powell MA, Grigsby PW (2010) Lymph node staging by positron emission tomography in cervical cancer: relationship to prognosis. J Clin Oncol 28 (12): 2108–2113.
Kim SK, Choi HJ, Park SY, Lee HY, Seo SS, Yoo CW, Jung DC, Kang S, Cho KS (2009) Additional value of MR/PET fusion compared with PET/CT in the detection of lymph node metastases in cervical cancer patients. Eur J Cancer 45 (12): 2103–2109.
Kodama S, Kanazawa K, Honma S, Tanaka K (1991) Age as a prognostic factor in patients with squamous cell carcinoma of the uterine cervix. Cancer 68 (11): 2481–2485.
Koh WJ, Greer BE, Abu-Rustum NR, Apte SM, Campos SM, Chan J, Cho KR, Cohn D, Crispens MA, DuPont N, Eifel PJ, Gaffney DK, Giuntoli RL 2nd, Han E, Huh WK, Lurain JR 3rd, Martin L, Morgan MA, Mutch D, Remmenga SW, Reynolds RK, Small W Jr., Teng N, Tillmanns T, Valea FA, McMillian NR, Hughes M (2013) Cervical cancer. J Natl Compr Cancer Network 11 (3): 320–343.
Landoni F, Maneo A, Colombo A, Placa F, Milani R, Perego P, Favini G, Ferri L, Mangioni C (1997) Randomised study of radical surgery versus radiotherapy for stage Ib-IIa cervical cancer. Lancet 350 (9077): 535–540.
Lecuru F, Mathevet P, Querleu D, Leblanc E, Morice P, Darai E, Marret H, Magaud L, Gillaizeau F, Chatellier G, Dargent D (2011) Bilateral negative sentinel nodes accurately predict absence of lymph node metastasis in early cervical cancer: results of the SENTICOL study. J Clin Oncol 29 (13): 1686–1691.
Lee WC, Lee SY, Koo YJ, Kim TJ, Hur SY, Hong SR, Kim SS, Kee MK, Rhee JE, Lee JS, Choi HS, Cho CH, Kim KT, Park JS (2013) Establishment of a Korea HPV cohort study. J Gynecol Oncol 24 (1): 59–65.
Lee YH, Choi KS, Lee HY, Jun JK (2012) Current status of the National Cancer Screening Program for cervical cancer in Korea, 2009. J Gynecol Oncol 23 (1): 16–21.
Lyman GH, Giuliano AE, Somerfield MR, Benson AB 3rd, Bodurka DC, Burstein HJ, Cochran AJ, Cody HS 3rd, Edge SB, Galper S, Hayman JA, Kim TY, Perkins CL, Podoloff DA, Sivasubramaniam VH, Turner RR, Wahl R, Weaver DL, Wolff AC, Winer EP American Society of Clinical Oncology (2005) American Society of Clinical Oncology guideline recommendations for sentinel lymph node biopsy in early-stage breast cancer. J Clin Oncol 23 (30): 7703–7720.
Matsuura Y, Kawagoe T, Toki N, Tanaka M, Kashimura M (2006) Long-standing complications after treatment for cancer of the uterine cervix--clinical significance of medical examination at 5 years after treatment. Int J Gynecol Cancer 16 (1): 294–297.
Morice P, Castaigne D, Pautier P, Rey A, Haie-Meder C, Leblanc M, Duvillard P (1999) Interest of pelvic and paraaortic lymphadenectomy in patients with stage IB and II cervical carcinoma. Gynecol Oncol 73 (1): 106–110.
Park JY, Kim DY, Kim JH, Kim YM, Kim YT, Nam JH (2010) Further stratification of risk groups in patients with lymph node metastasis after radical hysterectomy for early-stage cervical cancer. Gynecol Oncol 117 (1): 53–58.
Pecorelli S, Zigliani L, Odicino F (2009) Revised FIGO staging for carcinoma of the cervix. Int J Gynaecol Obstet 105 (2): 107–108.
Pieterse QD, Kenter GG, Gaarenstroom KN, Peters AA, Willems SM, Fleuren GJ, Trimbos JB (2007) The number of pelvic lymph nodes in the quality control and prognosis of radical hysterectomy for the treatment of cervical cancer. Eur J Surg Oncol 33 (2): 216–221.
Rockall AG, Sohaib SA, Harisinghani MG, Babar SA, Singh N, Jeyarajah AR, Oram DH, Jacobs IJ, Shepherd JH, Reznek RH (2005) Diagnostic performance of nanoparticle-enhanced magnetic resonance imaging in the diagnosis of lymph node metastases in patients with endometrial and cervical cancer. J Clin Oncol 23 (12): 2813–2821.
Sakuragi N (2007) Up-to-date management of lymph node metastasis and the role of tailored lymphadenectomy in cervical cancer. Int J Clin Oncol 12 (3): 165–175.
Shah M, Lewin SN, Deutsch I, Burke WM, Sun X, Herzog TJ, Wright JD (2011) Therapeutic role of lymphadenectomy for cervical cancer. Cancer 117 (2): 310–317.
Shim SH, Lee SW, Park JY, Kim YS, Kim DY, Kim JH, Kim YM, Kim YT, Nam JH (2013) Risk assessment model for overall survival in patients with locally advanced cervical cancer treated with definitive concurrent chemoradiotherapy. Gynecol Oncol 128 (1): 54–59.
Sironi S, Buda A, Picchio M, Perego P, Moreni R, Pellegrino A, Colombo M, Mangioni C, Messa C, Fazio F (2006) Lymph node metastasis in patients with clinical early-stage cervical cancer: detection with integrated FDG PET/CT. Radiology 238 (1): 272–279.
Takeda M, Sakuragi N, Okamoto K, Todo Y, Minobe S, Nomura E, Negishi H, Oikawa M, Yamamoto R, Fujimoto S (2002) Preoperative serum SCC, CA125, and CA19-9 levels and lymph node status in squamous cell carcinoma of the uterine cervix. Acta Obstet Gynecol Scand 81 (5): 451–457.
van Nagell JR Jr, Donaldson ES, Wood EG, Parker JC Jr (1978) The significance of vascular invasion and lymphocytic infiltration in invasive cervical cancer. Cancer 41 (1): 228–234.
Wright JD, Dehdashti F, Herzog TJ, Mutch DG, Huettner PC, Rader JS, Gibb RK, Powell MA, Gao F, Siegel BA, Grigsby PW (2005) Preoperative lymph node staging of early-stage cervical carcinoma by [18F]-fluoro-2-deoxy-D-glucose-positron emission tomography. Cancer 104 (11): 2484–2491.
Author information
Authors and Affiliations
Corresponding author
Ethics declarations
Competing interests
The authors declare no conflict of interest.
Additional information
The results of this manuscript were presented in part at the 2013 ASCO Annual Meeting, which was held from 31 May to 4 June 2013, at the McCormick Place Convention Center in Chicago, IL, USA.
This work is published under the standard license to publish agreement. After 12 months the work will become freely available and the license terms will switch to a Creative Commons Attribution-NonCommercial-Share Alike 3.0 Unported License.
Supplementary Information accompanies this paper on British Journal of Cancer website
Supplementary information
Rights and permissions
From twelve months after its original publication, this work is licensed under the Creative Commons Attribution-NonCommercial-Share Alike 3.0 Unported License. To view a copy of this license, visit http://creativecommons.org/licenses/by-nc-sa/3.0/
About this article
Cite this article
Kim, DY., Shim, SH., Kim, SO. et al. Preoperative nomogram for the identification of lymph node metastasis in early cervical cancer. Br J Cancer 110, 34–41 (2014). https://doi.org/10.1038/bjc.2013.718
Received:
Revised:
Accepted:
Published:
Issue Date:
DOI: https://doi.org/10.1038/bjc.2013.718
Keywords
This article is cited by
-
A preoperative nomogram predicting risk of lymph node metastasis for early-stage cervical cancer
BMC Women's Health (2023)
-
The role of lymph nodes in cervical cancer: incidence and identification of lymph node metastases—a literature review
International Journal of Clinical Oncology (2021)
-
The feasibility of 18F-FDG PET/CT for predicting pathologic risk status in early-stage uterine cervical squamous cancer
Cancer Imaging (2020)
-
Progress in the Study of Lymph Node Metastasis in Early-stage Cervical Cancer
Current Medical Science (2018)
-
Comment on ‘Pre-operative nomogram for the identification of lymph node metastasis in early cervical cancer’
British Journal of Cancer (2014)