Abstract
Beta hypersynchrony was recently introduced into clinical practice in Parkinson’s disease (PD) to identify the best stimulation contacts and for adaptive deep brain stimulation (aDBS) sensing. However, many other oscillopathies accompany the disease, and beta power sensing may not be optimal for all patients. The aim of this work was to study the potential clinical usefulness of beta power phase-amplitude coupling (PAC) with high frequency oscillations (HFOs). Subthalamic nucleus (STN) local field potentials (LFPs) from externalized DBS electrodes were recorded and analyzed in PD patients (n = 19). Beta power and HFOs were evaluated in a resting-state condition; PAC was then studied and compared with the electrode contact positions, structural connectivity, and medication state. Beta-HFO PAC (mainly in the 200–500 Hz range) was observed in all subjects. PAC was detectable more specifically in the motor part of the STN compared to beta power and HFOs. Moreover, the presence of PAC better corresponds to the stimulation setup based on the clinical effect. PAC is also sensitive to the laterality of symptoms and dopaminergic therapy, where the greater PAC cluster reflects the more affected side and medication “off” state. Coupling between beta power and HFOs is known to be a correlate of the PD “off” state. Beta-HFO PAC seems to be more sensitive than beta power itself and could be more helpful in the selection of the best clinical stimulation contact and probably also as a potential future input signal for aDBS.
Similar content being viewed by others
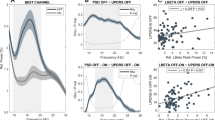
Introduction
Beta power hypersynchrony (13–35 Hz) in motor circuits has been a well-known pathophysiological marker of hypokinesia and rigidity in Parkinson’s disease (PD), correlating with the severity of these main motor symptoms and suppressible by dopaminergic treatment as well as by deep brain stimulation (DBS)1,2,3,4. A novel therapeutic approach called adaptive deep brain stimulation (aDBS), based on this main parkinsonian state biomarker, has been successfully introduced into clinical practice5,6,7. Together with this new technique based on local field potential (LFP) sensing, a new type of DBS electrode have been used, called “directional leads,” with segmented contacts making it possible to more precisely focus the volume of tissue activated within the targeted structure8,9. However, there are still some points and limitations to be addressed.
There is an individual oscillatory reactivity related to DBS. Frequency peaks in the STN vary among subjects. Some patients do not demonstrate beta power reduction as a response to DBS10. Equally, PD is a heterogeneous disease with differing severity of main motor symptoms and varying numbers of non-motor symptoms. Borderline phenotypes, such as tremor-dominant (TD) and postural instability gait disorders (PIGD), with distinct patterns of progression can be distinguished11. It is known that aDBS based on beta power sensing is not optimal for all patients. Individual patient-specific PD neural markers sensitive to DBS therapy might be more suitable for aDBS due to symptom heterogeneity12,13,14. Many other oscillopathies linked to PD have been described, including low frequencies and gamma band power reduction and changes in high-frequency oscillations (HFOs)14,15. Pathological cross-frequency interactions between different frequency ranges have been shown to play an important pathophysiological role. Coupling between the phase of slow activities and the amplitude of fast frequencies, such as the phase amplitude coupling (PAC) between beta and HFOs, have been described as linked to PD main motor symptoms16. HFOs and their cross-frequency and PAC have the potential to provide new biomarkers with direct implications in novel DBS therapy strategies15,17,18.
Compared to the conventional DBS leads that were used for recordings in the majority of the patients in this study, as subject recruitment started before the new systems were commercially available in our country, the new directional leads have a higher number of contacts and stimulation setting options. The earlier programing approach based on best clinical effect testing is now more complicated and time consuming. LFP evaluation of the beta power amplitude has been recommended as a tool for clinical practice for predicting the most efficient stimulation contact19. However, as mentioned above, beta power does not seem to be optimal for all the patients and PD symptoms. A search for new and more specific biomarkers is therefore necessary.
The aim of this work was to study the occurrence of beta-HFO PAC and its potential use in clinical practice.
Results
We analyzed resting-state STN LFPs in 19 PD patients in the immediate postoperative period. First, the beta power characteristics were evaluated. The PAC was then calculated with a focus on relations between beta and HFO. The measures were next compared to:
-
the exact electrode position using the Lead-DBS software,
-
later stimulation contacts with best clinical effect on PD motor symptoms, chosen after the standard clinical postoperative testing and initial setting,
-
structural connectivity estimation based on the Human Connectome Project atlas.
-
Finally, the effect of dopaminergic medication was evaluated.
Beta power characteristics are shown in Table 1. Typical beta power peaks were not clearly detectable in 9 patients. Among the other 10 patients, the power of the beta peak was higher in the more affected STN in 6 cases (p = 0.031, the paired Wilcoxon signed-rank test, data with not normal distribution tested by the Kolmogorov-Smirnov test). However, the effect of laterality was not significant across the whole patient group (p = 0.432).
We were able to detect beta-HFO PAC in all of our patients. PAC was present in different HFO frequencies among the patients; moreover, there were lateralization differences within the same patients. This could be explained by the side difference in the severity of PD symptoms. Larger clusters of beta-HFO PAC were present in the more affected STN (p = 0.036, the paired Wilcoxon signed-rank test, data with not normal distribution tested by the Kolmogorov-Smirnov test) and the HFO frequency was lower than in the less affected STN (p = 0.024, paired the Wilcoxon signed-rank test, data with not normal distribution tested by the Kolmogorov-Smirnov test). Details are presented in Table 2.
Beta-HFO PAC dependency on the lead location
PAC was higher and more clearly detectable in the bipolar contact pairs localized within the motor part of the STN than in the other contacts (see Fig. 1). Contacts with the smallest distance to the STN motor part sweet spot (left STN = [−11 −14 −7] mm, right STN = [10.83 −13.31 −7.01] mm)20, were marked as optimal based on the lead localization approach. Other contacts were marked as suboptimal. The difference in the distance between the first and second closest contact to the sweet spot was significant across patients (left STN: p = 0.0001, right STN: p = 0.0001). Because the data did not have a normal distribution (tested by the Kolmogorov-Smirnov test), the paired Wilcoxon signed-rank test was used. The comparison between beta-HFO PAC clusters in optimal and suboptimal contacts was then assessed. The cluster size in the optimal contacts was significantly larger than the cluster size in the suboptimal contacts for both the left STN (p = 0.0005) and the right STN (p = 0.001). The cluster size variable did not have a normal distribution (tested by the Kolmogorov-Smirnov test) across patients; the paired Wilcoxon signed-rank test was therefore used.
A, B present contacts localized in the motor part of the left and right STN, noted as optimal. PAC is present bilaterally mainly in 200–500 HFOs range. C, D present contacts localized next to the motor part of the left and right STN, where no clear PAC is present, noted as suboptimal. In lead visualization orange color represents the motor part of the STN, blue is the associative part, and yellow corresponds to limbic part. Red color highlights contact pairs used for PAC calculation.
Beta power and beta-HFO PAC comparison for future best clinical contact selection
We evaluated beta power peak occurrence first. There were 9 cases for which beta power parameter was non-conclusive (see Table 1 and Fig. 2) because of no clear beta peaks present in the power spectrum. PAC seemed to be more specific for the motor part of the STN than the beta power enhancement itself, mostly in cases in which a clear beta peak was not present – see Figs. 2 and 3 for a case-based example. The stimulation contact selection based on beta-HFO PAC corresponded to the contact localization provided by Lead-DBS reconstruction in 7 cases in the left STN and 13 cases in the right STN. For beta power, there were only 2 cases in the left STN and 5 cases in the right STN. Tested by McNemar’s chi-square, beta-HFO PAC is significantly more accurate than beta power (p = 0.0003). The concordance with the contact selection based on the clinical effect was even higher, although it was not significant compared to beta power, McNemar’s chi-square, p = 0.09 (discussed in the study limitation section), 12 cases in the left STN and 14 cases in the right STN for beta-HFO PAC, and 8 cases in the left STN and 11 cases in the right STN for beta power (Fig. 2).
Comparison of the best contact selection according to: electrode localization by lead-DBS (blue), PAC (orange), beta power (yellow), and HFOs (violet). The gray background shows the contact selected according to the clinical effect. Yellow beta power bars where a clear beta peak in the spectrum was not detectable are shown hatched, see also lower contact specificity in these cases. A – left STN (contact pairs L0-L1, L1-L2 and L2-L3), (B) – right STN (contact pairs R0-R1, R1-R2 and R2-R3). In the context of electrode localization, PAC identified the optimal stimulation contact for the left STN in 7 of 19 cases (subject 1, 2, 3, 6, 8, 11, 15) and for the right STN in 13 of 19 cases (subject 1, 2, 3, 6, 8, 9, 10, 11, 13, 14, 17, 18, 19). Specific beta peak identified optimal contact in 2 cases for the left STN (subject 11, 15) and in 5 cases for the right STN (subject 1, 8, 13, 14, 17). In the context of the concordance with the clinical effect, PAC identified the optimal contact in 12 cases for the left STN (subject 1, 2, 3, 5, 7, 10, 11, 13, 14, 15, 16, 17) and in 14 cases for the right STN (subject 1, 2, 3, 4, 7, 8, 9, 11, 13, 14, 16, 17, 18, 19). Specific beta peak identified contact in 8 cases for the left STN (subject 1, 5, 8, 10, 11, 13, 15, 17) and 11 for the right STN (subject 1, 4, 6, 7, 8, 10, 12, 13, 14, 15, 17).
Subject No. 7 (A) – electrode position reconstruction (orange – motor part, blue – associative part, yellow – limbic part of the STN), B – power spectrum, C – PAC. Beta power peak is sufficient for the right STN electrode but non-conclusive for the left STN, which is more severely affected. Here, the beta-HFO PAC clearly shows the bipolar pair L1-L2, which is best placed close to the motor part of the STN.
Beta-HFO PAC and structural connectivity
Structural connectivity was estimated using the Human Connectome Project21,22 for optimal and suboptimal contacts in each patient. This method has been described as having a predictive value similar to that of a patient-specific MRI tractography21. The activation of SMA in particular is thought to occur via the activation of fibers within the hyperdirect pathway and to have the highest importance for the DBS response in PD. In our study, the volume of tissue activated (VTA) created for contact pairs with the highest beta-HFO PAC (noted as optimal) were connected mainly to the supplementary motor area (SMA). VTA created for contact pairs with no or low beta-HFO PAC had structural connections to the SMA but also to other fronto-parietal structures – see Fig. 4. The activation of wide fronto-parietal areas is redundant and could also probably lead to the occurrence of adverse side effects.
Beta-HFO PAC and dopaminergic therapy
In a subgroup of 10 patients, we had the possibility to study the influence of dopaminergic therapy; recordings were repeated after chronic medication intake, in the medication “on” state. In the other cases, this could not be done for technical reasons or due to patient fatigue.
Concerning the beta power analysis of left side STN, 6 patients had no clear beta peak and 4 patients had partial or total suppression of the whole beta peak by medication. For the right STN, there were 3 patients with no clear beta peak and 7 patients with partial or total suppression of the whole beta peak by medication – see Table 3.
Beta-HFO PAC cluster size in the medication “on” state was significantly lower than the cluster size in the medication “off” state for the left STN (p = 0.004) and the right STN (p = 0.002); tested by the paired Wilcoxon signed-rank test – see Table 3, Fig. 5.
Discussion
We recorded and studied intracranial data from the STN in PD patients in the immediate postoperative period following DBS surgery via externalized electrodes before the system internalization. This kind of research is challenging for the patients and clinical system; on the other hand, despite the epoch of sensing neurostimulators, it has the potential to provide new and useful information, mainly in the field of HFOs and their important impact.
The role of beta-HFO PAC in the pathophysiology of PD and its hypokinetic symptomatology has been described, as has its potential role in predicting effective DBS lead contacts to treat motor symptoms15,23,24. It has been shown that the origins of excessive beta power and HFOs are close but do not exactly overlap. The PAC relationship maximum is spatially localized more closely to HFO origins24. Therefore, localizing the best clinical contact based on beta power and PAC can differ and aDBS based on these different biomarkers could have different outcomes. Further studies with more detailed topographic mapping, imaging methods, and visualization techniques have been recommended to resolve these questions. We have therefore implemented retrospective electro-clinical correlations and new visualization methods, including in particular a structural connectivity evaluation based on the Human Connectome Project atlas25.
In this study, we confirmed the occurrence of beta-HFO PAC phenomena in the STN with the maximum in the motor part of the STN. In our group of patients, the beta power evaluation was sufficient for the clinical contact selection in only approximately half of the cases. In some cases or sides, the beta power analysis was not conclusive; beta peaks were not clearly expressed, mainly where the lead location was not optimal. In these lead contacts, PAC was detectable and correlated with the best contact placed in the motor STN according to the Lead-DBS tool and clinical setting based on the effect on motor symptoms. According to these analyses – see Fig. 2, beta-HFO PAC seems to be a more precise marker for selecting the stimulation sweet spot26,27.
Beta-HFO PAC is linked with beta power hypersynchrony, which is known to be a correlate of parkinsonian main motor symptoms and the “off” state condition. Pathological beta power probably impedes pro-kinetic HFO patterns when the beta-HFO PAC is prominent24. Beta burst dynamics vary during PD “off” and “on” clinical states. During “off” states, they have a long duration and strong bilateral interhemispheric phasic coupling28. In our study, we were able to confirm the reduction of the beta-HFO PAC during the “on” medication state, similar to the well-known beta power decrease after levodopa intake (see Table 3 and Fig. 5). Therefore, this PAC could also serve as a potential future input signal for aDBS, probably more specific than sensing based on beta power alone.
We observed interindividual variability in the frequency range of the beta-HFO PAC as well as lateralization differences in individual subjects. It is known that there are variations even in individual beta frequency peaks29. The beta-HFO PAC was more dominant in the more affected STN and shifted to lower HFO frequencies (Table 2). Weaker coupling and HFOs in higher ranges probably correspond with better parkinsonian states and vice versa.
Effective DBS treatment and good clinical outcomes are related to specific MRI structural connectivity that can be evaluated using human connectome data without the need for additional imaging21. The importance of anatomical connections of the DBS electrodes to the area of SMA has been confirmed26. In our study, we have documented that the electrode contacts with the highest beta-HFO PAC have structural connectivity only to the SMA, in contrast to the other contacts that can influence much wider frontal and parietal regions. From this point of view again, beta-HFO PAC seems to be a sensitive marker for optimal structural connectivity contact in the motor part of the STN with potentially the best clinical outcomes in PD therapy.
The main limitation of this study is that the recordings in the majority of our subjects were performed from older four-ring contact electrodes, as the data collection started at a time when the new directional leads were not yet available in our country. Monopolar signals from leads were recorded against scalp references; they had to be recalculated into a bipolar montage to evaluate LFPs in STN. Such a technical limitation can be confusing when compared with a clinical stimulation setting that is usually monopolar. These comparisons are presented in Fig. 2, where one of two neighboring bipolar contacts can be selected as a good match to a monopolar clinical setting.
Another limitation is that beta-HFO PAC seems to be more sensitive than beta power according to the postoperative clinical setting, but not significantly. However, the statistically significant difference is present in the context of concordance with lead localization.
Unfortunately, we were not able to correlate the PAC changes to the clinical state improvement after dopaminergic medication intake, as we could not administer the full MDS-UPDRS scale. Patients were on the first day post-surgery, not verticalized, and transported from the postoperative intensive care unit. MDS-UPDRS scores obtained at other time periods are not representative, as they could be influenced by many factors.
In conclusion, we were able to confirm the occurrence of beta-HFO PAC in all subjects. The main difference from previous studies focused on this topic is the direct comparison of beta-HFO PAC to beta power for identifying the optimal clinical contact for clinical practice using various types of approaches. In a subgroup of the patients, we confirmed the dopaminergic reactivity of the PAC phenomena. Greater PAC clusters were detected in the more affected STN. Beta-HFO PAC is probably more specific for the motor part of the STN. It could be a more useful biomarker for the best stimulation contact selection and probably also serve as a future input signal for aDBS. Further studies are necessary to confirm these findings.
Methods
Surgical procedure
The stimulation leads were implanted bilaterally into the STN by stereotaxic MRI-guided technique, including intraoperative microrecording and stimulation. After the implantation of both electrodes, a CT study was performed under stereotactic conditions covering the entire length of the implanted electrodes.
Electrode contacts localization and connectivity
DBS electrode positions were reconstructed using Lead-DBS software (www.lead-dbs.org,30). Postoperative CT images were co-registered to preoperative MRI using advanced normalization tools (ANTs)31. Each step was manually checked in each patient. The structural connectivity from electrode contacts was estimated according to the anatomic connectome reconstruction based on the Human Connectome Project atlas, structural group connectome 32 Adult Diffusion MGH-USC HCP subjects GQI was used21,22,25. The seed areas were constructed as VTA by setting the stimulation parameters after the full course of clinical DBS optimization in each patient.
Subjects, experimental protocol, and recordings
PD patients with externalized DBS electrodes implanted to the STN bilaterally (n = 19, see Table 4) in the immediate post-operative period (second day after surgery) before the system internalization participated in the study. Patients did not express signs of dementia or major depression and did not have any other clinically relevant severe problems according to detailed preoperative neuropsychological examinations. All subjects were informed about the scientific nature of this study and signed informed consent forms. The study received the approval of the local ethics committees (the ethics board of Masaryk University and the ethics board of St. Anne’s hospital in Brno). Five-minute resting-state LFP EEG was recorded from the STN in the medication “off” state, after 12 h of dopaminergic therapy withdrawal. In a subgroup of the patients (n = 10), the recording was repeated 45 min after chronic dopaminergic medication intake. Simultaneously, 128-channel scalp EEG were recorded. A few scalp contacts had to be blinded in each patient because of the sterile covering of the externalized DBS electrode area. The scalp data were used only for referencing the intracerebral contacts in this work. Subjects reclined comfortably in the monitoring bed, in a Faraday-shielded room. They were instructed to remain calm, to keep their eyes fixed on the monitor, and to avoid unnecessary movements. The sampling rate was 5 kHz, the recordings were monopolar with the average reference of all scalp electrodes, except blinded contacts and the most peripheral ones with a higher risk of possible muscle artifacts (F9, F10, FT9, FT10, T9, T10, TP9, TP10, P9, P10, Nz, Iz). The data were recorded by amplifier M&I BioSDA09 (M&I Ltd., Czech Republic) with internal sampling frequency 25 kHz/24 bit. For the 5 kHz output sampling rate, the maximal useful frequency bandwidth is 0.01–2000 Hz.
Signal preprocessing
The LFPs data were processed off-line using the EEGLAB toolbox32 and MATLAB 2021a (The MathWorks, Inc, Natick, USA). The signal was first filtered to 1–500 Hz by a second-order Butterworth filter. The filtration was performed in forward and reverse directions for zero phase distortion. A bipolar montage was performed to exclude the volume conduction from other structures and to confirm the local origin of the potentials33,34. It created three bipolar signals for the left STN marked as L0-L1, L1-L2, L2-L3 and similarly three bipolar signals for the right STN marked as R0-R1, R1-R2, R2-R3. Bad signals were detected and marked manually by visual inspection of the data in SignalPlant software35 and excluded from the subsequent analysis.
Power analysis
For each bipolar signal (L0-L1, L1-L2, L2-L3 and R0-R1, R1-R2, R2-R3) the temporal fluctuations of beta power in the 12–30 Hz band and HFOs power in the 200–500 Hz band were computed by fast Fourier transform (FFT) in 10-second windows (Hamming) with 90% overlap and then averaged. To identify the dominant beta peak frequency, the power spectral density (PSD) estimate by periodogram was calculated with same window parameters and averaging.
Beta-HFO PAC
The PAC was calculated using the modulation index (MI) method36. The MI approach generates a complex valued composite signal, where the amplitude is created from the high frequency amplitude envelope values and the phase matched to the low frequency signal’s instantaneous phase. The composite signal creates a joint probability density function in the complex plane. If the average of the signal is non-zero, it shows that a particular amplitude and phase value co-occur in time. An MI value then corresponds to the absolute value of the average of the composite signal.
Whole PAC computation was adopted from the PAC toolbox for MATLAB37. It uses shuffled datasets to evaluate the statistical significance of the calculated MI values38,39. The high-frequency amplitude signal was shuffled to disrupt the time-ordering of values. This was achieved by segmenting the data, the number of which was set equal to the number of seconds. The boundaries of the segments were placed at random locations chosen with uniform probability throughout the signal. The segments were randomly reordered to create a shuffled signal. The shuffling retained the mean, variance, and power spectrum of the original signal, whereas the temporal relationship between amplitude values is removed. Discontinuities are introduced and there is evidence that this can introduce spurious PAC detection results38,39; however, the performance on artificial data was still deemed sufficient, presumably since the discontinuities are independently distributed in time. A population of 50 shuffled signals were created and compared to the original low-frequency signal in order to generate a distribution of PAC values using the modulation index (MI) measure. PAC in the top 5% of the distribution were deemed significant. The resulting values were corrected for multiple comparisons by the false discovery rate (FDR). Patient-specific PAC comodulograms were constructed with significant relationships only.
PAC was computed for the phase 1–50 Hz (step 2 Hz) and amplitude 1–500 Hz (step 5 Hz). Filtering to predefined frequency bins was achieved via a convolution with complex Morlet wavelets with width = 7 (number of cycles defining the Morlet mother wavelet). Particular frequency bins of beta and HFO where coupling was presented were subject-specific and also side-specific (see Fig. 3). Beta-HFO PAC cluster size (Table 2) was evaluated as an area of the significant PAC presence in the window of phase 12–30 Hz and amplitude 200–500 Hz. Whole individual PAC comodulograms were then pooled in spectral ranges to show group results as a median across patients (Figs. 1 and 5).
Results of the PAC and power analysis were compared with symptom severity lateralization, contact positions within the STN using Lead-DBS software (Figs. 2 and 3, Tables 1 and 2), connectivity estimation using the Human Connectome Project atlas25 (Fig. 4), and with the consequent clinical effect based on postoperative clinical testing of each contact stimulation and clinical setting with the maximal improvement of motor symptoms without side effects after a full course of DBS optimization. Finally, the effect of dopaminergic therapy on beta power and beta-HFO PAC was examined in a subgroup of patients (Table 3 and Fig. 5).
Reporting summary
Further information on research design is available in the Nature Research Reporting Summary linked to this article.
Data availability
The data supporting the findings of this study are not openly available. Anonymized data are, however, available from the corresponding author upon request.
Code availability
The underlying code for this study is available from the corresponding author upon request.
References
Kühn, A. A., Kupsch, A., Schneider, G.-H. & Brown, P. Reduction in subthalamic 8-35 Hz oscillatory activity correlates with clinical improvement in Parkinson’s disease. Eur. J. Neurosci. 23, 1956–1960 (2006).
Chen, C. C. et al. Complexity of subthalamic 13–35Hz oscillatory activity directly correlates with clinical impairment in patients with Parkinson’s disease. Exp. Neurol. 224, 234–240 (2010).
Oswal, A. et al. Deep brain stimulation modulates synchrony within spatially and spectrally distinct resting state networks in Parkinson’s disease. Brain 139, 1482–1496 (2016).
Steiner, L. A. et al. Subthalamic beta dynamics mirror Parkinsonian bradykinesia months after neurostimulator implantation. Mov. Disord. 32, 1183–1190 (2017).
Little, S. et al. Adaptive deep brain stimulation in advanced Parkinson disease. Ann. Neurol. 74, 449–457 (2013).
Rosa, M. et al. Adaptive deep brain stimulation in a freely moving parkinsonian patient. Mov. Disord. 30, 1003–1005 (2015).
Little, S. & Brown, P. Debugging adaptive deep brain stimulation for parkinson’s disease. Mov. Disord. 35, 555–561 (2020).
Pollo, C. et al. Directional deep brain stimulation: an intraoperative double-blind pilot study. Brain 137, 2015–2026 (2014).
Timmermann, L. et al. Multiple-source current steering in subthalamic nucleus deep brain stimulation for Parkinson’s disease (the VANTAGE study): a non-randomised, prospective, multicentre, open-label study. Lancet Neurol. 14, 693–701 (2015).
Giannicola, G. et al. The effects of levodopa and ongoing deep brain stimulation on subthalamic beta oscillations in Parkinson’s disease. Exp. Neurol. 226, 120–127 (2010).
Aleksovski, D., Miljkovic, D., Bravi, D. & Antonini, A. Disease progression in Parkinson subtypes: the PPMI dataset. Neurol. Sci. 39, 1971–1976 (2018).
Florin, E., Pfeifer, J., Visser-Vandewalle, V., Schnitzler, A. & Timmermann, L. Parkinson subtype-specific Granger-causal coupling and coherence frequency in the subthalamic area. Neuroscience 332, 170–180 (2016).
Castaño-Candamil, S. et al. Identifying controllable cortical neural markers with machine learning for adaptive deep brain stimulation in Parkinson’s disease. NeuroImage Clin. 28, 102376 (2020).
van Wijk, B. C. M., de Bie, R. M. A. & Beudel, M. A systematic review of local field potential physiomarkers in Parkinson’s disease: from clinical correlations to adaptive deep brain stimulation algorithms. J. Neurol. 270, 1162–1177 (2023).
López-Azcárate, J. et al. Coupling between beta and high-frequency activity in the human subthalamic nucleus may be a pathophysiological mechanism in parkinson’s disease. J. Neurosci. 30, 6667–6677 (2010).
van Wijk, B. C. M. et al. Subthalamic nucleus phase–amplitude coupling correlates with motor impairment in Parkinson’s disease. Clin. Neurophysiol. 127, 2010–2019 (2016).
Telkes, I. et al. Local field potentials of subthalamic nucleus contain electrophysiological footprints of motor subtypes of Parkinson’s disease. Proc. Natl. Acad. Sci. USA 115, E8567–E8576 (2018).
Meidahl, A. C. et al. Synchronised spiking activity underlies phase amplitude coupling in the subthalamic nucleus of Parkinson’s disease patients. Neurobiol. Dis. 127, 101–113 (2019).
Tinkhauser, G. et al. Directional local field potentials: a tool to optimize deep brain stimulation. Mov. Disord. 33, 159–164 (2018).
Akram, H. et al. Subthalamic deep brain stimulation sweet spots and hyperdirect cortical connectivity in Parkinson’s disease. NeuroImage 158, 332–345 (2017).
Horn, A. et al. Connectivity Predicts deep brain stimulation outcome in Parkinson disease. Ann. Neurol. 82, 67–78 (2017).
Horn, A. et al. Probabilistic conversion of neurosurgical DBS electrode coordinates into MNI space. NeuroImage 150, 395–404 (2017).
Yang, A. I., Vanegas, N., Lungu, C. & Zaghloul, K. A. Beta-coupled high-frequency activity and beta-locked neuronal spiking in the subthalamic nucleus of parkinson’s disease. J. Neurosci. 34, 12816–12827 (2014).
van Wijk, B. C. M. et al. Localization of beta and high-frequency oscillations within the subthalamic nucleus region. NeuroImage Clin. 16, 175–183 (2017).
Setsompop, K. et al. Pushing the limits of in vivo diffusion MRI for the Human Connectome Project. NeuroImage 80, 220–233 (2013).
Horn, A., Neumann, W.-J., Degen, K., Schneider, G.-H. & Kühn, A. A. Toward an electrophysiological “sweet spot” for deep brain stimulation in the subthalamic nucleus. Hum. Brain Mapp. 38, 3377–3390 (2017).
Nguyen, T. A. K. et al. Directional stimulation of subthalamic nucleus sweet spot predicts clinical efficacy: proof of concept. Brain Stimulat. 12, 1127–1134 (2019).
Tinkhauser, G. et al. Beta burst dynamics in Parkinson’s disease OFF and ON dopaminergic medication. Brain 140, 2968–2981 (2017).
Feldmann, L. K. et al. Toward therapeutic electrophysiology: beta-band suppression as a biomarker in chronic local field potential recordings. npj Parkinson’s Dis. 8, 44 (2022).
Horn, A. & Kühn, A. A. Lead-DBS: a toolbox for deep brain stimulation electrode localizations and visualizations. NeuroImage 107, 127–135 (2015).
Ewert, S. et al. Toward defining deep brain stimulation targets in MNI space: a subcortical atlas based on multimodal MRI, histology and structural connectivity. NeuroImage 170, 271–282 (2018).
Delorme, A. & Makeig, S. EEGLAB: an open source toolbox for analysis of single-trial EEG dynamics including independent component analysis. J. Neurosci. Methods 134, 9–21 (2004).
Wennberg, R. A. & Lozano, A. M. Intracranial volume conduction of cortical spikes and sleep potentials recorded with deep brain stimulating electrodes. Clin. Neurophysiol. 114, 1403–1418 (2003).
Wennberg, R. & Lozano, A. M. Restating the importance of bipolar recording in subcortical nuclei. Clin. Neurophysiol. 117, 474–475 (2006).
Plesinger, F., Jurco, J., Halamek, J. & Jurak, P. SignalPlant: an open signal processing software platform. Physiol. Meas. 37, N38–N48 (2016).
Canolty, R. T. et al. High gamma power is phase-locked to theta oscillations in human neocortex. Science 313, 1626–1628 (2006).
Onslow, A. C. E., Bogacz, R. & Jones, M. W. Quantifying phase–amplitude coupling in neuronal network oscillations. Prog. Biophys. Mol. Biol. 105, 49–57 (2011).
Kramer, M. A., Tort, A. B. L. & Kopell, N. J. Sharp edge artifacts and spurious coupling in EEG frequency comodulation measures. J. Neurosci. Methods 170, 352–357 (2008).
Aru, J. et al. Untangling cross-frequency coupling in neuroscience. Curr. Opin. Neurobiol. 31, 51–61 (2015).
Acknowledgements
This research was financially supported by Czech science foundation GAČR 21-25953 S and core facility MAFIL of CEITEC supported by the MEYS CR (LM2018129 Czech-BioImaging). Thanks to Anne Johnson for English language assistance and to Veronika Pulkrábková for patient organization.
Author information
Authors and Affiliations
Contributions
Martina Bočková – Conceptualization; Data curation; Funding acquisition; Investigation; Project administration; Resources; Writing - original draft. Martin Lamoš – Formal analysis; Investigation; Methodology; Software Visualization; Writing - review & editing. Jan Chrastina – Conceptualization; Project administration; Resources; Supervision; Validation; Writing - review & editing. Pavel Daniel – Data curation; Formal analysis; Writing - review & editing. Silvia Kupcová – Data curation; Project administration; Writing - review & editing. Ivo Říha – Formal analysis; Methodology; Writing - review & editing. Lucia Šmahovská – Project administration; Writing - review & editing. Marek Baláž – Supervision; Validation; Writing - review & editing. Ivan Rektor – Conceptualization; Funding acquisition; Investigation; Supervision; Validation; Writing - review & editing.
Corresponding author
Ethics declarations
Competing interests
The authors declare no competing interests.
Additional information
Publisher’s note Springer Nature remains neutral with regard to jurisdictional claims in published maps and institutional affiliations.
Supplementary information
Rights and permissions
Open Access This article is licensed under a Creative Commons Attribution 4.0 International License, which permits use, sharing, adaptation, distribution and reproduction in any medium or format, as long as you give appropriate credit to the original author(s) and the source, provide a link to the Creative Commons licence, and indicate if changes were made. The images or other third party material in this article are included in the article’s Creative Commons licence, unless indicated otherwise in a credit line to the material. If material is not included in the article’s Creative Commons licence and your intended use is not permitted by statutory regulation or exceeds the permitted use, you will need to obtain permission directly from the copyright holder. To view a copy of this licence, visit http://creativecommons.org/licenses/by/4.0/.
About this article
Cite this article
Bočková, M., Lamoš, M., Chrastina, J. et al. Coupling between beta band and high frequency oscillations as a clinically useful biomarker for DBS. npj Parkinsons Dis. 10, 40 (2024). https://doi.org/10.1038/s41531-024-00656-8
Received:
Accepted:
Published:
DOI: https://doi.org/10.1038/s41531-024-00656-8