Abstract
Ki-67 assessment is a key step in the diagnosis of neuroendocrine neoplasms (NENs) from all anatomic locations. Several challenges exist related to quantifying the Ki-67 proliferation index due to lack of method standardization and inter-reader variability. The application of digital pathology coupled with machine learning has been shown to be highly accurate and reproducible for the evaluation of Ki-67 in NENs. We systematically reviewed all published studies on the subject of Ki-67 assessment in pancreatic NENs (PanNENs) employing digital image analysis (DIA). The most common advantages of DIA were improvement in the standardization and reliability of Ki-67 evaluation, as well as its speed and practicality, compared to the current gold standard approach of manual counts from captured images, which is cumbersome and time consuming. The main limitations were attributed to higher costs, lack of widespread availability (as of yet), operator qualification and training issues (if it is not done by pathologists), and most importantly, the drawback of image algorithms counting contaminating non-neoplastic cells and other signals like hemosiderin. However, solutions are rapidly developing for all of these challenging issues. A comparative meta-analysis for DIA versus manual counting shows very high concordance (global coefficient of concordance: 0.94, 95% CI: 0.83–0.98) between these two modalities. These findings support the widespread adoption of validated DIA methods for Ki-67 assessment in PanNENs, provided that measures are in place to ensure counting of only tumor cells either by software modifications or education of non-pathologist operators, as well as selection of standard regions of interest for analysis. NENs, being cellular and monotonous neoplasms, are naturally more amenable to Ki-67 assessment. However, lessons of this review may be applicable to other neoplasms where proliferation activity has become an integral part of theranostic evaluation including breast, brain, and hematolymphoid neoplasms.
Similar content being viewed by others
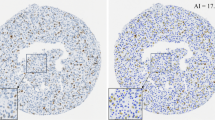
Introduction
The world health organization (WHO) released in 2019 a consensus document entitled “Recommendations on digital interventions for health system strengthening”, acknowledging that artificial intelligence (AI) and digital technologies can offer limitless possibilities to advance health management and achievements (https://apps.who.int/iris/handle/10665/311980, last access 10/30/2021). Indeed, AI-based technologies are emerging in every medical field, especially in radiology and pathology1,2,3. In pathology, AI-based systems utilize machine- and/or deep- learning models to assist pathologists in analyzing digital images to perform different tasks, including screening for rare events, quantification, diagnosing lesions, and prognostication1,4,5. Digital pathology, which includes the digitizing of glass slides to generate whole slide images, facilitates the application of AI in pathology6,7,8. A key benefit of employing AI-based systems in pathology is to provide reliable, objective and reproducible results, thereby reducing inter- and intra-pathologist variability and enabling automation to augment routine practice1,6,7,8.
In this context, digital image analysis (DIA) has been utilized to evaluate neuroendocrine neoplasms (NENs). Among well-differentiated neuroendocrine tumors (NETs), grading is based on the assessment of mitotic rate and the proliferation index determined by Ki-67 immunostaining9,10,11. Currently, the WHO classification of some NENs specifies that Ki-67 should be assessed by manual counting on a printed image including at least 500 neoplastic cells from the regions of highest labeling (hotspots)9,10. Recently, different DIA-based systems have been developed to assist pathologists with this important task (Fig. 1), which has implications for the clinical management of patients with NENs. To date, the majority of studies on this topic were performed on NENs of the gastro-entero-pancreatic system.
This is a particularly illustrative case due to the presence of a lymphocytic infiltrate at the tumor periphery, which represents a potential source of bias for Ki67 assessment with digital systems. A A pancreatic neuroendocrine tumor, G2, is shown. (Hematoxylin-eosin, 10x original magnification); B the digitalized system can count all cells present in a specific field, also on hematoxylin-eosin slides; C, D modern systems can select a specific area for the Ki-67 count: in this example, the field with lymphocytes has been excluded from the count, reducing potential important biases in tumor grading (Ki67 immunohistochemistry, 10x original magnification).
The aim of our study was to systematically review all published studies that compared manual Ki-67 assessment in pancreatic NENs (PanNENs) with DIA-based determination, highlighting the benefits and drawbacks of each approach. A comparative meta-analysis is also undertaken of manual counting versus DIA for PanNENs.
Materials and methods
This systematic review adhered to the MOOSE guidelines12 and PRISMA statement13. Studies were considered eligible for inclusion if they reported original data on DIA-based assessment of Ki-67 in PanNENs. Both neuroendocrine tumors (PanNETs) and carcinomas (PanNECs) were included. For the comparative meta-analysis of manual counting vs. DIA, we considered all manuscripts reporting an analytical comparison between these two modalities used in the assessment of Ki-67. In the case of duplicate cohorts, the largest and then most recent was selected. Exclusion criteria included no definitive histological diagnosis of PanNEN, and in vitro or animal studies.
Data sources and literature search strategy
Two investigators (CL, PA) independently searched PubMed, Embase and SCOPUS databases up until 30/06/2021. The search strategy included combinations of the following keywords: #1 “digital”[Title/Abstract] AND “pathology”[Title/Abstract]; #2 “image”[Title/Abstract] AND “analysis”[Title/Abstract]; #3 “artificial intelligence”[Title/Abstract] OR “AI”[Title/Abstract] OR “machine learning”[Title/Abstract] OR “deep learning”[Title/Abstract] OR “automated”[Title/Abstract] OR “semiautomated”[Title/Abstract] OR “algorithm*“[Title/Abstract] OR “neural network”[Title/Abstract] OR “computer-aid”[Title/Abstract] OR “computer-aided”[Title/Abstract] OR “image analysis”[Title/Abstract] OR “digital pathology”[Title/Abstract] OR “WSI”[Title/Abstract] OR “whole slide”[Title/Abstract] OR “digital”[Title/Abstract]; #4 #1 OR #2 OR #3; #5 “neuroendocrine”[Title/Abstract] OR “carcinoid”[Title/Abstract] OR “medullary”[Title/Abstract]; #6 #4 AND #5; #7 “artificial intelligence”[MeSH Terms]; #8 “Neuroendocrine Tumors”[MeSH Terms] OR “carcinoma, neuroendocrine”[MeSH Terms] OR “Gastro-enteropancreatic neuroendocrine tumor” [Supplementary Concept] OR “Carcinoid Tumor”[MeSH Terms]; #9 #7 AND #8; #10 #6 OR #9.
Study selection and data extraction
Following the aforementioned search strategy, duplicates were removed and then two reviewers (CL, PA) independently screened titles and abstracts of all potentially eligible articles. These two authors applied eligibility criteria and reviewed the full texts of included studies. A final list of articles was subsequently established for both the systematic review and comparative meta-analysis by consensus with a third independent author (AE). Two authors were involved in extracting data in a preset Excel database: one (CL) extracted data from the selected articles; the other (AS) independently validated the extracted data. For each article, we extracted the following information: authors; year of publication; country study originated from; number of cases; patient demographics; type of analyzed material; tumor grading; as well as methods for manual counting and DIA. For the comparative meta-analysis, the primary outcome was the coefficient of agreement between manual counting vs. DIA in the assessment of Ki-67 in PanNENs.
Data synthesis, quality, and publication bias assessment
The comparative meta-analysis was conducted using Comprehensive Meta-Analysis v2 software (Biostat; Englewood, NJ, USA). Furthermore, the Newcastle–Ottawa Scale (NOS) was used to assess study quality, following existing guidelines14,15. Finally, we investigated publication bias by visual inspection of funnel plots and with the Egger bias test16.
Results
Search results
The search yielded a total of 4286 potential eligible studies. Following in-depth screening based on title/abstract, only 56 (1.3%) of these studies were retrieved for further analysis. Of them, 22 were considered eligible for the systematic review17,18,19,20,21,22,23,24,25,26,27,28,29,30,31,32,33,34,35,36,37,38, and 4 for the correlation meta-analysis (Supplementary Fig. 1)25,27,28,34.
Study and patient characteristics
The most important features from the extracted data are summarized in Table 1. Overall, the selected studies reported data on a total of 752 PanNENs. The majority of the investigated cohorts (59.1%) were from the USA, with the remaining composed of European patients (27.3%) and mixed cohorts including Asian patients (13.6%). There was an almost equal distribution of male (50.5%) and female (49.5%) patients. Regarding tumor grading (G), the majority of cases were G1 (55.3%), followed by G2 (40.6%) and G3 (4.1%). The type of specimen material analyzed varied, including surgical resection specimens, biopsies, and cytology cell blocks. The majority of the studies (54.5%) did not report specific data on the type of specimens analyzed. The reported procedures used for manual counting and the specific DIA technologies adopted in the assessment of Ki-67 in PanNENs are summarized in Table 1.
Advantages and limitations of DIA-based systems in the assessment of Ki-67
The key advantages and limitations of DIA-based systems in the assessment of Ki-67 in PanNENs are summarized in Table 2. The most commonly described advantages of DIA were improved reproducibility and reliability, as well as reduced time required for Ki-67 assessment. The most common limitations of DIA were counting non-neoplastic (“contaminants”) cells (e.g., lymphocytes), the higher cost compared with manual counting, and the potential delay in diagnosis, which was dependent on some procedures or technician availability.
Comparative meta-analysis, quality and publication bias assessment
Overall, for the comparative meta-analysis of 4 studies including 238 patients with PanNEN were selected25,27,28,34. The pooled correlation estimate was 0.94 (95%CI: 0.83–0.98; I2 = 24.15%), indicating a high correlation between manual (reference value) and digital count. The heterogeneity was low (i.e., I2 < 50%), reinforcing the reliability of these results. The quality of the studies did not represent risk of bias (mean score of the Newcastle–Ottawa Scale: 8). Furthermore, no publication bias emerged (Egger’s test = 1.42; p = 0.90). The fail-safe number was 660, a value that indicates strong statistical significance of our results based on existing guidelines15,16.
Discussion
The Ki-67 proliferative index is critical in the pathologic assessment of PanNEN, and has important clinical implications for patient management. The adoption of international recommendations released by the WHO classification of tumors and the European neuroendocrine tumor society (ENETS) for assessing Ki-67 has improved the standardization of methodologies for this task9,39. However, given the persistence of interlaboratory and interobserver discrepancies, as well as potential inconsistencies with different scoring systems, accurately grading PanNENs remains a challenge for pathologists, especially in the G1-G2 and G2-G3 transition areas for PanNETs. Multiple factors affecting the interpretation of the Ki-67 index include the use of different antibody clones and staining protocols, tissue section thickness, tumor cell density, and difficulty distinguishing tumor from non-tumor cells. According to Adsay, “to count or not to count is not the question, but rather how to count”40. Manually counting camera captured or printed images is generally favored over eyeballing. Further, more recently DIA has proven to be an acceptable method for Ki-67 assessment. In this study, we reviewed all published investigations that employed DIA for Ki-67 determination in PanNENs, highlighting some of the advantages and limitations of utilizing this technology. Furthermore, by comparing the coefficient of correlation between manual counting and DIA by means of a comparative meta-analysis, we demonstrated a high value of consistency (0.94, 95%CI: 0.83–0.98) between these two approaches.
The advantages derived from utilizing DIA include more reproducible results, higher accuracy, and reduced time to evaluate Ki-67 in PanNENs1,6,7,8. Current guidelines for assessing Ki-67 recommended manual counting from a printed image that includes at least 500 neoplastic cells from tumor hotspots. While still time consuming, this manual method does promote standardization that helps reduce interobserver variability24. However, for grade transitions between G1 and G2 (3% of Ki-67) and between G2 and G3 (20% of Ki-67), there were still discrepancies with manual counting from a printed image. The use of DIA for Ki-67 determination resulted in greater consistency in grading of all PanNEN cases, particularly for those cases belonging to the aforementioned gray transition areas G1-G2 and G2-G3. However, it should be noted that even when using DIA one can obtain different results depending on the selection of hotspots and by altering the number of cells counted. Access to DIA allows rapid counting of more cells, and that alone can push a tumor from G2 to G1 or G3 to G2, whereas counting fewer cells in the same hotspot can achieve the reverse41.
DIA assistance with grading PanNEN is of particular benefit in fine needle aspiration (FNA) cytology samples. Guidelines established using histological samples have been extrapolated to grading PanNENs in cytology material (e.g., cell blocks) procured by FNA. Several studies have demonstrated that Ki-67 assessment by manual counting of tumor cells in cell blocks can result in under-grading of these neoplasms when compared with matched surgical resection specimens36,42, with discrepancies more often observed in G2 cases20,29. Intriguingly, Abi-Raad and colleagues demonstrated that counting hotspots instead of the complete cell block can provide a higher concordance with surgical specimens, especially for FNA samples that contain ≥ 1000 cells43. A different perspective was provided by Satturwar and colleagues who investigated the potential role of augmented reality microscopy (ARM) for Ki-67 assessment in cytology specimens36. ARM, which is basically a modified microscope associated with an attached computer unit, enables real-time image analysis using a traditional light microscope and glass slides, without the need to first photograph or digitize slides36,44,45. If coupled with image analysis software, ARM allows quantifying immunohistochemical stains including Ki-67, and can also be combined with elaborate AI-based algorithms to perform more complex tasks44,45,46. Like other DIA methods, ARM has significant potential for improving PanNEN grading on cell block material36.
Currently, DIA for Ki-67 assessment has some limitations that may need to be addressed if counting in practice is to be performed with this approach. The most commonly reported drawback is the risk of counting dividing non-neoplastic “contaminating” cells (e.g., endothelial cells, lymphocytes), thereby erroneously increasing the overall tumor grade. Other brown-pigmented signals (hemosiderin and hematoidin) also cause this over-counting phenomenon. Such issues are enhanced in NEC, especially due to the effect of artefacts (e.g., smeared chromatin material, nuclear molding in small cell NEC) on DIA. However, these problems can be overcome by having pathologists directly annotate regions of interest to be scored, with the intent of excluding contaminating cells from being counted. Further studies that specifically address these challenges in PanNEC are needed. This issue becomes particularly important if non-pathologist personnel such as trainees and technicians are used as key operators. Of note, more sophisticated AI-algorithms are being developed that only count neoplastic cells47,48,49 and become more operator-independent. One potential solution that also has been employed is to utilize double-stained slides (e.g., Ki-67 and synaptophysin) with deep learning algorithms to improve the accuracy of Ki-67 index quantification50,51,52,53. More recently, some investigators have shown that they are able to predict Ki-67 positive cells directly from H&E images using AI-based methods51. Another important pending issue that needs to be addressed for improving Ki-67 assessment in PanNENs is related to standardizing hotspot size and number to be evaluated41,54. Hotspots are defined as tumor areas with higher Ki-67 nuclear staining. It has been shown that the greater the hotspot size, the lower the Ki-67 count, highlighting the importance of standardizing this parameter for reliable evaluation34,41. Furthermore, not only is the size of the hotspot difficult to define, but so is the shape55. Most pathologists and algorithms define a hotspot as a circular shape; however, there is no biological evidence to support this notion. Another important factor to consider is the number of hotspots when determining the Ki-67 index. Training operators not to select a geographic region that may lead to hyper-selection of positive cells in a given hotspot region is also important, which erroneously creates higher “percentage” positivity. However, all of the above shortcomings are relatively easy to address with proper training and application of improved AI software.
Despite the clear advantages of DIA for determination of the Ki-67 labeling index, scoring with this digital modality is still subject to the fundamental limitation that applies to any cut-off in a continuous variable: it can be changed randomly, as it was for PanNETs in 2017 when it was moved from 2 to 3 for Grade 19. Moreover, any cut-off of a continuous variable can be shown to have value, but the actual grading is inherently arbitrary41. Indeed, how best to employ K-i67 as a reliable prognosticator of PanNETs has been a study in progress. For example, in 2017 the cut-off was clarified such that cases with an index less than 3.0 (including 2.99), which were previously unclear as to which grade this belonged, now clearly included Grade 19. Naturally, as in any grading and staging system that assesses a continuous variable, the Ki-67 index-based system is imperfect54. For example, it can be expected that cases with 2.99 (now in G1) and 3.0 (now in G2) will be similar in biological behavior. Nevertheless, DIA will help standardize the process, not only offering more reproducible results in daily clinical practice, but also allowing for better comparison between studies that aim to fine-tune this grading system. For example, there have been proposals to move the G1/G2 cut-off to 5%; but it is difficult to verify the results of these proposals due to variation in counting methods. Fundamentally, the reality is that even with more accurate analysis provided by DIA, a G2 tumor with a Ki67 of 4% will still be more likely to behave in an indolent fashion than a G2 tumor with a Ki67 of 19%. Thus, the issue of a continuous variable, which is a complex concept involving statistical and biological sciences56, enhances the need for accurate Ki-67 quantification and may ultimately be more important than the actual grade score. Finally, a significant limitation of DIA for widespread adoption has been the accessibility of this technology due to cost and maintenance. However, as whole slide scanners and digital cameras (and related software) become more widely available, the adaptation of facilities to perform DIA for Ki-67 counting is becoming increasingly feasible and amenable to employ57. Another issue to be considered is the need to better integrate Ki-67 counting by DIA into routine workflow24,58.
In this review, we chose to focus on PanNENs. However, the topic of manual vs. digital pathology scoring of Ki-67 is also certainly of importance for NENs at many other anatomic sites54, as well as for other neoplasms in which DIA-based systems are being leveraged to assess biomarkers. In 2015, Joseph et al. studying a cohort of 48 lung carcinoids, demonstrated an overall similarity of manual counting vs. DIA; although Ki-67 estimation resulted in slightly higher results than manual counting59. Of note, a more recent analysis by Swarts et al. comparing the use of manual analysis vs. DIA (in-house Leica Qwin program) in a cohort of 201 lung tumors, described a substantial equivalence of both methodologies60. It is also worth noting that Ki-67 assessment may be of importance in other tumor types. For example, in 2020 Hida et al. compared the use of manual analysis vs. DIA (Visiopharm software) for proliferative index evaluation in a total of 413 cases of breast cancer, showing a high value of correlation (coefficient of correlation = 0.82, p < 0.0001) between both methods61. Alataki et al. corroborated these findings, demonstrating a high correlation in Ki-67 assessment between manual and DIA in both surgical breast resections and biopsies62.
An important question is whether the comparison of Ki-67 assessment between manual vs. DIA-based systems influences clinical management and prognostication. Among all selected manuscripts, only four provided data on this specific topic20,23,25,30. Goodell et al. demonstrated significant reliability in predicting nodal and distant metastasis of PanNETs with the ventana image analysis system (VIAS), with the highest specificity (94% in their cohort) demonstrated when analyzing 10 consecutive and randomly selected fields20. Similarly, van Velthuysen et al. investigating the performance of manual vs. digital (ImageJ) Ki-67 scoring in a cohort of 73 PanNENs, showed that tumor grading correlated with survival irrespective of the way Ki-67 was assessed23. Similar results were replicated by Kroneman et al.25. and Conemans et al.30, showing substantial similarities in terms of prognostication between manual vs. DIA scoring of Ki-67. It is important to note that only four studies in the literature provided data on this fundamental topic. Moreover, all of these studies were conducted prior to the introduction of the 2017 grading system. Thus, further studies on larger cohorts and based on current grading methods are needed. We advocate that DIA-based systems could provide a more standardized method, guaranteeing a more reliable basis for prognostic stratification.
In summary, this systematic review and comparative meta-analysis demonstrates that the advantages outweigh the limitations of using DIA to assess Ki-67 in PanNENs. We advocate that the next logical step for more broadly adopting DIA in pathology practice would be to further explore the relationship between hotspot parameters (number, size, and shape) and the Ki-67 index with patient outcome. Currently, most studies use manual counting from captured images as the gold standard; however, the ultimate validation will naturally come from prognostic correlation. Based upon current evidence provided by peer-reviewed literature, DIA appears to offer pathologists higher reliability and reproducibility than manual counting for grading PanNENs. The overall findings of this review, therefore, support widespread adoption of carefully optimized and validated DIA-based methods for this important diagnostic task in clinical practice. Lessons learned from the application of DIA to the PanNEN model can also be extrapolated to different tumors in other organ systems, such as breast carcinoma in which Ki-67 quantification is increasingly becoming a key driver for patient management.
Data availability
All data/information are available in the manuscript and in the supplementary material.
References
Luchini C., Pea A., Scarpa A. Artificial intelligence in oncology: current applications and future perspectives. Br. J. Cancer https://doi.org/10.1038/s41416-021-01633-1 (2021).
Benzekry, S. Artificial Intelligence and Mechanistic Modeling for Clinical Decision Making in Oncology. Clin. Pharm. Ther. 108, 471–486 (2020).
Huynh, E. et al. Artificial intelligence in radiation oncology. Nat. Rev. Clin. Oncol. 17, 771–781 (2020).
Niazi, M. K. K., Parwani, A. V. & Gurcan, M. N. Digital pathology and artificial intelligence. Lancet. Oncol. 20, e253–e261 (2019).
Harrison, J. H. et al. Introduction to artificial intelligence and machine learning for pathology. Arch. Pathol. Lab. Med. 145, 1228–1254 (2021).
Cohen, S., Levenson, R. & Pantanowitz, L. Artificial intelligence in pathology. Am. J. Pathol. 191, 1670–1672 (2021).
Cheng, J. Y., Abel, J. T., Ugj, B., McClintock, D. S. & Pantanowitz, L. Challenges in the development, deployment, and regulation of artificial intelligence in anatomic pathology. Am. J. Pathol. 191, 1684–1692 (2021).
Tizhoosh, H. R. & I Pantanowitz, L. Artificial intelligence and digital pathology: challenges and opportunities. J. Pathol. Inf. 14, 9 (2018).
Lloyd R. V., Osamura R. Y., Kloppel G., Rosai J. WHO Classification of Tumours of Endocrine Organs 4th edn. Lyon, IARC Press, 2017.
WHO Classification of Tumours Editorial Board. Digestive System Tumours 5th edn. IARC Press, Lyon, 2019.
van Velthuysen, M. L. et al. Grading of neuroendocrine neoplasms: mitoses and ki-67 are both essentials. Neuroendocrinology 100, 221–227 (2014).
Stroup, D. F. et al. Meta-analysis of observational studies in epidemiology: a proposal for reporting. Meta-analysis of observational studies in epidemiology (MOOSE) group. JAMA 283, 2008–2012 (2000).
Liberati, A. et al. The PRISMA statement for reporting systematic reviews and meta-analyses of studies that evaluate healthcare interventions: explanation and elaboration. BMJ 339, b2700 (2009).
Luchini, C., Stubbs, B., Solmi, M. & Veronese, N. Assessing the quality of studies in meta-analyses: advantages and limitations of the Newcastle Ottawa scale. World J. Meta-Anal. 5, 80–84 (2017).
Luchini, C. et al. Assessing the quality of studies in meta-research: Review/guidelines on the most important quality assessment tools. Pharm. Stat. 20, 185–195 (2021).
Egger, M., Davey Smith, G., Schneider, M. & Minder, C. Bias in meta-analysis detected by a simple, graphical test. BMJ 315, 629–634 (1997).
Bagci, P. et al. Comparative analysis of different counting methodologies for Ki-67 in pancreatic neuroendocrine tumors. Lab. Invest. 92, 441A (2012).
Remes, S. M., Tuominen, V. J., Helin, H., Isola, J. & Arola, J. Grading of neuroendocrine tumors with Ki-67 requires high-quality assessment practices. Am. J. Surg. Pathol. 36, 1359–1363 (2012).
Fung, A., Cohen, C., Kavuri, S., Gao, X. & Reid, M. Measurement of interobserver variability in calculating MIB1 labeling index by counting tumor cells in well differentiated neuroendocrine tumors (WDNETs) of the pancreas and gastrointestinal tract: a cytologic analysis of 22 cases. J. Am. Soc. Cytopathol. 1, S95 (2012).
Goodell, P. P., Krasinskas, A. M., Davison, J. M. & Hartman, D. J. Comparison of methods for proliferative index analysis for grading pancreatic well-differentiated neuroendocrine tumors. Am. J. Clin. Pathol. 137, 576–582 (2012).
Tang, L. H., Gonen, M., Hedvat, C., Modlin, I. M. & Klimstra, D. S. Objective quantification of the Ki67 proliferative index in neuroendocrine tumors of the gastroenteropancreatic system: a comparison of digital image analysis with manual methods. Am. J. Surg. Pathol. 36, 1761–1770 (2012).
Cimic, A., Johnsen, A. E., Harrison, W., Sirintrapun, J. S. & Mott, R. T. KI67 by image analysis and phosphohistone H3 are objective methods in grading pancreatic neuroendocrine tumors. Lab. Invest. 94, 397A (2013).
van Velthuysen, M. et al. Reliability of proliferation assessment by Ki-67 expression in neuroendocrine neoplasms: eyeballing or image analysis? Neuroendocrinology 100, 288–292 (2014).
Reid, M. D. et al. Calculation of the Ki67 index in pancreatic neuroendocrine tumors: a comparative analysis of four counting methodologies. Mod. Pathol. 28, 686–694 (2015).
Kroneman, T. N. et al. Comparison of three Ki-67 index quantification methods and clinical significance in pancreatic neuroendocrine tumors. Endocr. Pathol. 26, 255–262 (2015).
Mejias, L., Bhalla, A., Salem, N., Thomas, S. & Shidham, V. Evaluation of KI-67 (MIB-1) labeling index with dual-color immunocytochemistry (KI-67 with LCA) for grading of pancreatic neuroendocrine tumors. Lab. Invest. 95, 520A (2015).
Neely, C. et al. A comparison of automated digital image analysis (DIA) and manual count of camera-captured images in calculating Ki67 proliferation index (PI) in cytologic samples from pancreatic neuroendocrine neoplasms (PanNENs). Lab. Invest. 96, 111A (2015).
Burdette, E. et al. A comparison of manual counting with camera captured images and digital image analysis for KI-67 proliferative index assessment in pancreatic neuroendocrine tumors. Lab. Invest. 96, 510A (2015).
Jin, M. et al. Grading pancreatic neuroendocrine neoplasms by Ki-67 staining on cytology cell blocks: manual count and digital image analysis of 58 cases. J. Am. Soc. Cytopathol. 5, 286–295 (2016).
Conemans, E. et al. Prognostic value of WHO grade in pancreatic neuro-endocrine tumors in Multiple Endocrine Neoplasia type 1: Results from the DutchMEN1 Study Group. Pancreatology 17, 766–772 (2017).
Niazi, M. K. K. et al. Identifying tumor in pancreatic neuroendocrine neoplasms from Ki67 images using transfer learning. PLoS One 13, e0195621 (2018).
Dere, Y., Ozkaraca, O., Cetin, G. & Dere, O. Evaluation of an image-based automated detection system in detecting Ki67 proliferation index and correlation with the traditional eye-ball method in gastroenteropancreatic neuroendocrine tumors. J. Coll. Physicians. Surg. Pak. 29, 137–140 (2019).
Sajjan, S., Yang, Y., Mansoor, N. & Lee, L. Low incidence mitotic activity best detected by manual count as compared to whole slide imaging digital computer assessed counting: Lessons learned. Mod. Pathol. 32, 3 (2019).
Owens, R. et al. Comparison of different anti-Ki67 antibody clones and hot-spot sizes for assessing proliferative index and grading in pancreatic neuroendocrine tumours using manual and image analysis. Histopathology 77, 646–658 (2020).
Saadeh, H., Abdullah, N., Erashdi, M., Sughayer, M. & Al-Kadi, O. Histopathologist-level quantification of Ki-67 immunoexpression in gastroenteropancreatic neuroendocrine tumors using semiautomated method. J. Med. Imag. 7, 012704 (2020).
Satturwar, S. P. et al. Ki-67 proliferation index in neuroendocrine tumors: Can augmented reality microscopy with image analysis improve scoring? Cancer Cytopathol. 128, 535–544 (2020).
Lea, D. et al. Digital image analysis of the proliferation markers Ki67 and phosphohistone H3 in gastroenteropancreatic neuroendocrine neoplasms: accuracy of grading compared with routine manual hot spot evaluation of the Ki67 index. Appl. Immunohistochem. Mol. Morphol. 29, 499–505 (2021).
Boukhar, S., Gosse, M., Bellizzi, A. & Rajan, K. D. A. Ki-67 proliferation index assessment in gastroenteropancreatic neuroendocrine tumors by digital image analysis with stringent case and hotspot level concordance requirements. Am. J. Clin. Pathol. 156, 607–619 (2021).
O’Toole, D., Kianmanesh, R. & Enets, C. M. Consensus guidelines for the management of patients with digestive neuroendocrine tumors: an update. Neuroendocrinology 103, 117–118 (2016).
Adsay, V. Ki67 labeling index in neuroendocrine tumors of the gastrointestinal and pancreatobiliary tract: to count or not to count is not the question, but rather how to count. Am. J. Surg. Pathol. 36, 1743–1746 (2012).
Volynskaya, Z., Mete, O., Pakbaz, S., Al-Ghamdi, D. & Asa, S. L. Ki67 quantitative interpretation: insights using image analysis. J. Pathol. Inf. 10, 8 (2019).
Farrell, J. M., Pang, J. C., Kim, G. E. & Tabatabai, Z. L. Pancreatic neuroendocrine tumors: accurate grading with Ki-67 index on fine-needle aspiration specimens using the WHO 2010/ENETS criteria. Cancer Cytopathol. 122, 770–778 (2014).
Abi-Raad, R. et al. Grading pancreatic neuroendocrine tumors by Ki-67 index evaluated on fine-needle aspiration cell block material. Am. J. Clin. Pathol. 153, 74–81 (2020).
Chen, P. C., Gadepalli, K. & MacDonald, R. An augmented reality microscope with real-time artificial intelligence integration for cancer diagnosis. Nat. Med. 25, 1453–1457 (2019).
Razavian, N. Augmented reality microscopes for cancer histopathology. Nat. Med. 25, 1334–1336 (2019).
Ghosh, A. et al. The potential of artificial intelligence to detect lymphovascular invasion in testicular cancer. Cancers 16, 13 (2021).
Dov D., et al. Hybrid human-machine learning approach for screening prostate biopsies can improve clinical efficiency without compromising diagnostic accuracy. Arch. Pathol. Lab. Med. https://doi.org/10.5858/arpa.2020-0850-OA (2021).
D’Alfonso, T. M. et al. Multi-magnification-based machine learning as an ancillary tool for the pathologic assessment of shaved margins for breast carcinoma lumpectomy specimens. Mod. Pathol. 34, 1487–1494 (2021).
Chen, S. et al. Clinical use of a machine learning histopathological image signature in diagnosis and survival prediction of clear cell renal cell carcinoma. Int. J. Cancer 148, 780–790 (2021).
Govind, D. et al. Improving the accuracy of gastrointestinal neuroendocrine tumor grading with deep learning. Sci. Rep. 10, 11064 (2020).
Liu, Y. et al. Predict Ki-67 positive cells in H&E-stained images using deep learning independently from IHC-stained images. Front. Mol. Biosci. 7, 183 (2020).
Matsukuma, K. et al. Synaptophysin-Ki67 double stain: a novel technique that improves interobserver agreement in the grading of well-differentiated gastrointestinal neuroendocrine tumors. Mod. Pathol. 30, 620–629 (2017).
Hacking, S. M. et al. Potential pitfalls in diagnostic digital image analysis: experience with Ki-67 and PHH3 in gastrointestinal neuroendocrine tumors. Pathol. Res. Pr. 216, 152753 (2020).
Cree, I. A. et al. Counting mitoses: SI(ze) matters! Mod. Pathol. 34, 1651–1657 (2021).
Khan Niazi, M. K., Yearsley, M. M., Zhou, X., Frankel, W. L. & Gurcan, M. N. Perceptual clustering for automatic hotspot detection from Ki-67-stained neuroendocrine tumour images. J. Microsc. 256, 213–225 (2014).
Altman, D. G. & Royston, P. The cost of dichotomising continuous variables. BMJ 332, 1080 (2006).
Snead, D. R. et al. Validation of digital pathology imaging for primary histopathological diagnosis. Histopathology 68, 1063–1072 (2016).
Volynskaya, Z. et al. Integrated pathology informatics enables high-quality personalized and precision medicine: digital pathology and beyond. Arch. Pathol. Lab. Med. 142, 369–382 (2018).
Joseph, M. G. et al. Usefulness of Ki-67, mitoses, and tumor size for predicting metastasis in carcinoid tumors of the lung: a study of 48 cases at a tertiary care centre in Canada. Lung. Cancer Int. 2015, 545601 (2015).
Swarts, D. R. et al. Limited additive value of the Ki-67 proliferative index on patient survival in World Health Organization-classified pulmonary carcinoids. Histopathology 70, 412–422 (2017).
Hida, A. I. et al. Automated assessment of Ki-67 in breast cancer: the utility of digital image analysis using virtual triple staining and whole slide imaging. Histopathology 77, 471–480 (2020).
Alataki, A., Zabaglo, L., Tovey, H., Dodson, A. & Dowsett, M. A simple digital image analysis system for automated Ki67 assessment in primary breast cancer. Histopathology 79, 200–209 (2021).
Acknowledgements
The authors thank Dr. Enrico Cavallo for his support.
Funding
This study is supported by Associazione Italiana Ricerca sul Cancro (AIRC IG n. 26343); Fondazione Cariverona: Oncology Biobank Project “Antonio Schiavi” (prot. 203885/2017); Fondazione Italiana Malattie Pancreas (FIMP-Ministero Salute J38D19000690001)
Author information
Authors and Affiliations
Contributions
C.L., L.P., V.A., S.L.A., P.A., I.G., A.N., N.V., M.K.N., M.N.G., A.E., I.A.C., A.S.: study conception and design; C.L., L.P., P.A., I.G., A.N., N.V., A.E., I.A.C., A.S.: systematic review and meta-analysis; all authors: data interpretation and discussion; C.L., L.P., V.A., S.L.A., P.A., M.K.N., M.N.G., A.E., I.A.C., A.S.: paper writing; all authors: final editing and approval of the present version.
Corresponding authors
Ethics declarations
Competing interests
The authors declare no competing interests.
Additional information
Publisher’s note Springer Nature remains neutral with regard to jurisdictional claims in published maps and institutional affiliations.
Supplementary information
Rights and permissions
Open Access This article is licensed under a Creative Commons Attribution 4.0 International License, which permits use, sharing, adaptation, distribution and reproduction in any medium or format, as long as you give appropriate credit to the original author(s) and the source, provide a link to the Creative Commons license, and indicate if changes were made. The images or other third party material in this article are included in the article’s Creative Commons license, unless indicated otherwise in a credit line to the material. If material is not included in the article’s Creative Commons license and your intended use is not permitted by statutory regulation or exceeds the permitted use, you will need to obtain permission directly from the copyright holder. To view a copy of this license, visit http://creativecommons.org/licenses/by/4.0/.
About this article
Cite this article
Luchini, C., Pantanowitz, L., Adsay, V. et al. Ki-67 assessment of pancreatic neuroendocrine neoplasms: Systematic review and meta-analysis of manual vs. digital pathology scoring. Mod Pathol 35, 712–720 (2022). https://doi.org/10.1038/s41379-022-01055-1
Received:
Revised:
Accepted:
Published:
Issue Date:
DOI: https://doi.org/10.1038/s41379-022-01055-1
This article is cited by
-
Establishment, characterization, and biobanking of 36 pancreatic cancer organoids: prediction of metastasis in resectable pancreatic cancer
Cellular Oncology (2024)
-
Digital counting of tissue cells for molecular analysis: the QuANTUM pipeline
Virchows Archiv (2024)
-
Exploration of Digital Image Analysis for Ki67 Quantification in the Grading of Medullary Thyroid Carcinoma: A Pilot Study with 85 Cases
Head and Neck Pathology (2023)
-
The Driver Role of Pathologists in Endocrine Oncology: What Clinicians Seek in Pathology Reports
Endocrine Pathology (2023)