Abstract
Observationally, coffee is inversely associated with type 2 diabetes mellitus (T2DM), depression and Alzheimer’s disease, but not ischemic heart disease (IHD). Coffee features as possibly protective in the 2015 Dietary Guidelines for Americans. Short-term trials suggest coffee has neutral effect on most glycemic traits, but raises lipids and adiponectin. To clarify we compared T2DM, depression, Alzheimer’s disease, and IHD and its risk factors by genetically predicted coffee consumption using two-sample Mendelian randomization applied to large extensively genotyped case-control and cross-sectional studies. Childhood cognition was used as a negative control outcome. Genetically predicted coffee consumption was not associated with T2DM (odds ratio (OR) 1.02, 95% confidence interval (CI) 0.76 to 1.36), depression (0.89, 95% CI 0.66 to 1.21), Alzheimer’s disease (1.17, 95% CI 0.96 to 1.43), IHD (0.96, 95% CI 0.80 to 1.14), lipids, glycemic traits, adiposity or adiponectin. Coffee was unrelated to childhood cognition. Consistent with observational studies, coffee was unrelated to IHD, and, as expected, childhood cognition. However, contrary to observational findings, coffee may not have beneficial effects on T2DM, depression or Alzheimer’s disease. These findings clarify the role of coffee with relevance to dietary guidelines and suggest interventions to prevent these complex chronic diseases should be sought elsewhere.
Similar content being viewed by others
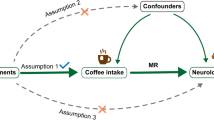
Introduction
Coffee is habitually consumed in Western societies. Adults in the United States and many European countries typically drink 2 to 3 cups of coffee a day1,2. With economic development coffee consumption is becoming more common in Asia including South Korea, China and India. Coffee is believed by the general public to have no benefits for type 2 diabetes mellitus (T2DM) or cognitive decline, but to increase the risk of cardiovascular disease (CVD)3. In contrast, coffee features in the latest 2015 Dietary Guidelines for Americans as something that might be healthy4. Observationally, coffee (both regular and decaffeinated) is monotonically associated with lower risk of type 2 diabetes mellitus (T2DM)5. Coffee is also associated with lower risk of depression6, as substantiated in a large prospective cohort study of older adults in the United States7, as well as of Alzheimer’s disease8. Coffee consumption is not clearly associated with ischemic heart disease (IHD)9, although moderate coffee drinking may be associated with slightly lower risk10. However, observational studies are open to biases from residual confounding by incompletely measured factors that may have major influences on lifestyle and health, such as socio-economic position and health status. Meta-analyses of randomized controlled trials (RCTs) suggest short-term coffee consumption raises triglycerides and low-density lipoprotein (LDL) cholesterol11. A small RCT found that short-term coffee consumption increased adiponectin12, which may relate to lower CVD risk13. Several RCTs showed short-term coffee consumption had no effect on fasting glucose, fasting insulin or insulin resistance12,14,15, although one RCT found it slightly increased glycosylated hemoglobin (HbA1c)16. The lack of evidence from long-term RCTs means that the effects of coffee on health are unclear, but are particularly important to establish in this window of opportunity before habitual coffee drinking extends to become the global norm.
In this situation, comparing health by genetically predicted coffee consumption, i.e., using Mendelian randomization (MR), may help clarify the causal effect of coffee on health by generating unbiased estimates from observational studies because MR is less prone to confounding and reverse causality17. To date, one MR study, using large cohort studies from Denmark, found no association of genetically predicted coffee consumption with T2DM or CVD risk factors including triglycerides, high-density lipoprotein (HDL) cholesterol, non-fasting glucose, waist circumference and body mass index (BMI)18. However, the study was underpowered to assess the effect of coffee on CVD risk factors and did not assess the effect on IHD. To clarify the role of coffee in health, we assessed the role of coffee consumption in T2DM, IHD, CVD risk factors (lipids, glycemic traits, adiposity and adiponectin), depression and Alzheimer’s disease using genetic determinants of coffee from genome-wide association studies (GWAS) applied to very large extensively genotyped case-control and cross-sectional studies. We used childhood cognition as a negative control outcome because coffee unlikely affects cognition in childhood, given coffee drinking usually becomes a habit after adolescence19.
Results
Genetically predicted coffee consumption
Table 1 shows ten single nucleotide polymorphisms (SNPs) were associated with habitual coffee consumption (number of cups of mainly regular-type coffee per day) at genome-wide significant (log10 Bayes Factor > 5.64 which approximates P < 5 × 10−8) in a GWAS of 129,788 coffee drinkers of mainly European descent (n = 121,824, 94%), mean age 54.0 years20. rs6968554 was excluded due to high linkage disequilibrium with rs4410790, giving 9 SNPs. rs17685 was not available for T2DM, lipids, so rs8565 was used instead because it was highly correlated with rs17685 (r2 = 0.845), in close proximity (distance within 25 kb of rs17685), had a similar allele frequency (HapMap CEU: rs8565 A (0.29) and rs17685 G (0.71)) and similar genetic association with IHD (Fig. 1). Four SNPs were related to body weight or lipids (rs6265, rs1260326, rs1481012 and rs7800944), so these were excluded for the analyses without known pleiotropy for T2DM, IHD and CVD risk factors. Three non-pleiotropic SNPs, which are known to be functionally relevant to coffee metabolism (rs4410790, rs2472297 and rs2470893)21,22, were included in the analyses of functionally relevant SNPs. rs2470893 and rs7800944 were not available for childhood cognition, so rs2472297 and rs14415, respectively, were used instead because they are highly correlated with the original SNPs (rs2472297: r2 = 0.694; rs14415: r2 = 0.816), in close proximity (rs2472297: distance within 10 kb of rs2470893; rs14415: distance within 100 kb of rs7800944) and had a similar allele frequency (HapMap CEU: rs2472297 T (0.25) and rs2470893 T (0.26); rs2286276 T (0.30) and rs7800944 T (0.29)).
Selection of single nucleotide polymorphisms for Mendelian randomization analysis of the association of coffee consumption with type 2 diabetes mellitus, ischemic heart disease, cardiovascular disease risk factors, depression and Alzheimer’s disease.
Abbreviations: HbA1c, glycosylated hemoglobin; HDL-cholesterol, high-density lipoprotein cholesterol; IHD, ischemic heart disease; LDL-cholesterol, low-density lipoprotein cholesterol; MR, Mendelian randomization; SD, standard deviation; SNP, single nucleotide polymorphisms; T2DM, type 2 diabetes mellitus; WHR, waist-hip ratio.
Table 2 shows genetically predicted coffee consumption was not clearly associated with T2DM, IHD, depression or Alzheimer’s disease both including and excluding SNPs with known pleiotropy. Most of the estimates were close to the null, particularly after excluding potentially pleiotropic SNPs, although the estimate for Alzheimer’s disease was in a positive direction. Coffee consumption was not clearly associated with most CVD risk factors (lipids, glycemic traits, BMI, WHR and adiponectin) particularly after excluding SNPs with known pleiotropy, although the estimates for LDL-cholesterol, BMI, WHR and adiponectin were in a positive direction. Coffee was unrelated to childhood cognition. An analysis using only the 3 functionally relevant SNPs gave a similar pattern of associations. Not using rs8565 as a replacement for rs17685 gave a very similar pattern of associations (data not shown). The associations remained similar after adjustment for multiple comparison (data not shown).
Discussion
Consistent with the previous smaller MR study using five SNPs for coffee18, we found little evidence of coffee being clearly related to T2DM or major CVD risk factors (HDL-cholesterol, LDL-cholesterol, triglycerides and BMI), although we cannot rule out the possibility of coffee raising LDL-cholesterol, BMI, WHR and adiponectin. Our study adds by replicating these findings in larger samples using more SNPs for coffee and showing coffee was also most likely unassociated with IHD and with glycemic traits, consistent with most12,14,15 but not all16 RCTs. This study also adds by showing coffee most likely unrelated to depression and Alzheimer’s disease, although we cannot exclude the possibility that coffee increases the risk of Alzheimer’s disease. Coffee was unrelated to childhood cognition as expected.
This large MR study taking advantage of publicly available ‘big data’ provides more precise estimates with greater statistical power because of the large sample sizes and less susceptibility to weak instrument bias from using 9 SNPs which reduces the possibility of false positives. Nonetheless, limitations exist. First, MR estimates could be confounded by population stratification23. We used genetic determinants of coffee from people of predominantly European ancestry (94%) and genetic associations with diseases or its risk factors from people almost exclusively of European ancestry with estimates adjusted for genomic control. In addition, genetic variants predicting coffee are not known to vary geographically within these populations20, unlike another beverage, milk, whose genetic determinant, lactase persistence, has a north-south gradient24. As such, our MR estimates are unlikely confounded by population stratification. Second, effects of genetic determinants of coffee via pathways other than through coffee intake may generate a bias (by violating the exclusion-restriction assumption)25. However, MR estimates with and without pleiotropic SNPs were fairly similar and we placed greater emphasis on the estimates without pleiotropic SNPs. We might have missed some pleiotropic effects because we could only identify known effects and current understanding of the underlying causal pathways. Nonetheless, 3 non-pleiotropic SNPs (rs4410790, rs2472297 and rs2470893) are known to be functionally relevant to coffee metabolism21,22. An analysis using only these SNPs gave broadly similar results. Third, the genetic variants for coffee were associated with number of cups of coffee per day among coffee drinkers, and the estimates would not relate to the effects of coffee if coffee drinking was uncommon in the samples with the outcomes26. However, the populations with the outcomes are from the United States or European countries27,28,29,30,31 where coffee drinking is typical1,2. Fourth, we cannot rule out the possibility of a non-linear effect of coffee, although that would require a more complex biological explanation. Fifth, the effect of coffee may vary by sex, given a cohort study found coffee consumption was associated with lower risk of cognitive decline in women but not in men32. Whether habitual coffee consumption affects health differently by age, sex or baseline coffee consumption could not be tested because genetic associations with coffee and with the outcomes were obtained from separate samples; however the effects of causal factors are generally consistent, although sex-specific mechanistic pathways are possible. Sixth, we used genetic variants for habitual coffee consumption among coffee drinkers. Whether the findings generalize to ever/never coffee drinkers remains elusive, although extrapolating associations from very infrequent coffee drinkers to never coffee drinkers may be reasonable. Seventh, given coffee drinking usually starts in adulthood, developmental canalization buffering the genetic effects as a compensatory mechanism is unlikely to affect interpretation of the MR estimates. Eighth, participants in the studies used may have taken medication for chronic diseases, although genetic associations with lipids33 and glycemic traits were based on participants not taking relevant medication34,35. However, medication use is unlikely to confound the association of genetic variants with the outcomes, because genetic variants are allocated at conception and precede medication use. Medication use might make the association of genetic variants with coffee consumption less precise. As such, medication use could bias the MR estimate away from the null, hence MR estimates are best interpreted as indicating direction rather than exact effects, particularly for estimates that differ from the null value36. Finally, since coffee consumption was not measured in the samples with the outcomes, two-sample MR generates approximate estimates by assuming the genetic associations for coffee are similar in the samples of genetic determinants of coffee and the outcomes26. Nonetheless, separate sample MR is more robust to chance findings than single-sample MR because it reduces the possibility of confounding by some cryptic data structure in the single sample37.
Unlike previous observational studies5, our study, as well as the previous smaller MR study18, did not find coffee consumption associated with lower risk of T2DM. Also, unlike some prospective cohort studies9,10, we found no association of coffee consumption with IHD. Such discrepancies might be partly explained by over-adjustment for potentially harmful mediators, such as BMI or lipids10, and the inevitable confounding in observational studies. For CVD risk factors, as in the other MR study18, we found little evidence of an association of coffee with HDL-cholesterol or triglycerides. The associations of coffee with LDL-cholesterol and adiponectin are directionally consistent with those found in RCTs11,12, but do not exclude no association. We also found no association of coffee with HbA1c, fasting glucose, fasting insulin, beta-cell function or insulin resistance, consistent with most12,14,15 but not all16 RCTs. In addition, trends in coffee consumption do not coincide with the changing patterns of IHD or T2DM, for example IHD declined38 but DM rose39 in the United States where coffee consumption was stable in the past decade40. Taken together, the overall lack of association of coffee with T2DM, IHD and many CVD risk factors are coherent within this study, and suggest that coffee has likely minor effects, if any, on these conditions.
Our MR study has some consistency with RCTs, although an MR study tests a causal pathway rather than an intervention41. Findings from MR give the lifetime effect of coffee and may be more relevant to the health implications of coffee than findings from RCTs evaluating the short-term effect of a coffee intervention42. Nonetheless, replication in a larger sample would be valuable. Our findings, using genetic variants for ‘regular’ coffee, i.e., coffee without decaffeination and/or filtration, do not exclude the possibility of coffee raising LDL cholesterol. Coffee has been thought to have cholesterol-raising effects due to the presence of diterpenes (cafestol and kahweol), and such effect is usually removed only when coffee is filtered43. Several SNPs functionally relevant to coffee regulate the cytochrome P-450 (CYP) enzyme, which may have implications for CVD risk44, but includes a large family of enzymes with different functions. The aryl hydrocarbon receptor (AHR) (rs4410790) regulates CYP1A2 (rs2472297). CYP1A2 is primarily responsible for metabolizing caffeine21 and CYP1A1 (rs2470893) metabolizes polycyclic aromatic hydrocarbons, another key ingredient of coffee22. CYP1A1/1A2/1B1 knockout mice have lower cholesterol45. Whether AHR is related to circulating cholesterol remains elusive; AHR knockout mice have higher hepatic triglycerides in response to high-fat diet46. However, SNPs from CYP1A1/2 have not featured in GWAS of CVD or diabetes27,28,29,47, consistent with the lack of association with these two conditions.
This study adds by showing no protective association of habitual coffee consumption with depression or Alzheimer’s disease, contrary to meta-analyses of observational studies where coffee is associated with lower risk6,8. These findings are consistent with null association of coffee with childhood cognition (control outcome). Observed associations of coffee with (particularly subjective measures of) mental health are prone to confounding by socioeconomic position and related attributes (diet and lifestyle), underlying physical health status, and reverse causality. However, the potentially positive association of coffee with Alzheimer’s disease does warrant further investigation. Coffee drinking habits may have changed over time; observationally increasing coffee consumption is associated with higher risk of mild cognitive impairment48, while constant moderate coffee consumption is associated with lower risk48. Hence, we cannot rule out the possibility that our finding was generated by increased coffee consumption as self-medication for cognitive lapses, although use of genetically predicted coffee consumption should reduce such ‘reverse causality’. Previous observational studies suggest coffee as a modifiable lifestyle factor that may be associated with lower risk of cognitive impairment/decline, although not across all studied cognitive domains49,50. In addition, cohort studies with more complete follow-up tended to observe weaker negative or positive associations of coffee with dementia51. Our MR findings raise a question as to the role of coffee in Alzheimer’s disease, which requires replication, so as to clarify the role of coffee as a potential intervention. Coffee consumption has been associated with smaller volume of the hippocampus and poor memory function52. EFCAB5 (rs9902453) is a newly identified SNP for coffee, downstream of SLC6A4, which encodes the serotonin transporter and could reduce circulating serotonin53, which might be related to Alzheimer’s disease54. Better understanding of whether and how serotonin regulation counteracts neurotoxicity reduction by caffeine induced blockage of adenosine A2 receptor55 or other non-caffeine components including chlorogenic acids that have been associated with lower risks of dementia56 would help clarify the etiology.
In summary, habitual coffee consumption may not have the beneficial effects on IHD, T2DM, most CVD risk factors, depression and Alzheimer’s disease suggested by observational studies, instead our study raises the possibility that coffee could increase the risk of Alzheimer’s disease and possibly have some unfavourable effects on lipids. This study demonstrates the pitfalls of formulating dietary recommendations based on observational evidence23 and emphasizes the importance of genetic validation of potential targets of intervention before making policy or testing interventions36.
Methods
Genetically predicted coffee consumption
Genetically predicted coffee consumption was based on single nucleotide polymorphisms (SNPs) of genome-wide significant (P < 5 × 10−8). Highly correlated SNPs (high linkage disequilibrium) (r2 > 0.8) were discarded based on larger P value with the correlations taken from SNP Annotation and Proxy Search (SNAP) (www.broadinstitute.org/mpg/snap/ldsearchpw.php) using the relevant catalog. SNPs potentially affecting an outcome directly rather than via coffee consumption (pleiotropic effects) were identified from Ensembl (Homo sapiens – phenotype) (http://grch37.ensembl.org/Homo_sapiens/Info/Index). Any SNP for coffee not available for an outcome was replaced with a highly correlated SNP (r2 > 0.8).
Genetically predicted T2DM, IHD, CVD risk factors, depression and Alzheimer’s disease
Genetic associations for T2DM were obtained from the DIAbetes Genetics Replication And Meta-analysis (DIAGRAM), a case (n = 34,840)-control (n = 114,981) study of T2DM mainly in people of European descent (n = 146,171, 98%), mean age 56.9 years, with genomic control and adjustment for study-specific covariates29. Data on coronary artery disease/myocardial infarction (MI) have been contributed by CARDIoGRAMplusC4D investigators and have been downloaded from www.CARDIOGRAMPLUSC4D.ORG. CARDIoGRAMplusC4D 1000 Genomes-based GWAS is a case (n = 60,801)-control (n = 123,504) study of IHD and MI in people of European (n = 143,485, 77%), South Asian (n = 25,557, 13%), East Asian (n = 11,323, 6%) and Hispanic or African American descent (~4%), adjusted for age and sex and corrected for genomic control47. CARDIoGRAMplusC4D Metabochip is a case (n = 63,746)-control (n = 130,681) study of IHD mainly in people of European descent (n = 176,892, 91%), mean age 57.4 years, adjusted for age and sex and corrected for genomic control27. When a SNP was not available in CARDIoGRAMplusC4D, genetic associations were obtained from CARDIoGRAM, a more extensively genotyped subset case (n = 22,233)-control (n = 64,762) study of IHD in people of European descent, mean age 58.1 years, with genetic associations similarly adjusted28. Genetic associations for lipids were obtained from the Global Lipids Genetics Consortium (GLGC) which has inverse normal transformed HDL-cholesterol, LDL-cholesterol and triglycerides for 188,577 people of European descent33. MAGIC concerns people mainly of European descent without diabetes and has glycosylated hemoglobin (HbA1c) (%) for 46,368 adults35, fasting glucose (mmol/L) for 133,010 and log-transformed fasting insulin for 108,55734 (or if not available, fasting glucose for 46,186 and fasting insulin for 38,238 based on the 2010 version57), homeostatic model assessment (HOMA) β-cell function for 36,466 and HOMA insulin resistance for 37,03757. Genetic associations for adiposity were obtained from the Genetic Investigation of Anthropometric Traits (GIANT) which has inverse normal transformed BMI (n = 322,154)58 and WHR (n = 210,088) for people of European descent59. Genetic associations for adiponectin were obtained from the ADIPOGen Consortium which includes 35,355 people mainly of European descent (n = 29,347, 83%)60. Genetic associations for depression were obtained from the Psychiatric GWAS Consortium (PGC), a case (n = 9,240)-control (n = 9,519) study of major depressive disorder in people of European descent, mean age 45.9 years30. Genetic associations for Alzheimer’s disease were obtained from the International Genomics of Alzheimer’s Project (IGAP), a case (n = 17,008)-control (n = 37,154) study of Alzheimer’s disease in people of European descent, mean age 71.4 years31.
Genetically predicted childhood cognition (control outcome)
Genetic associations for childhood cognition were obtained from the Social Science Genetic Association Consortium (SSGAC), which has cognition measured by general cognitive ability or intelligence quotient for 17,989 people of European descent61.
Statistical Analysis
Genetic associations with T2DM, IHD, CVD risk factors (lipids, glycemic traits, BMI, WHR, and adiponectin), depression, Alzheimer’s disease and childhood cognition (control outcome) were extracted based on the SNPs predicting habitual coffee consumption. Associations of coffee consumption with these outcomes were obtained using weighted generalized linear regression for correlated SNPs62, with a correlation matrix to account for correlation between genetic variants obtained from SNAP using the same catalog as used in the GWAS of the outcome62. Given the two IHD case-control studies overlap (57.5% of the cases and 40.1% of controls)47, we also combined their results for IHD accounting for this overlap using the Lin and Sullivan approach63. Estimates are shown with all genome-wide significant SNPs with potentially pleiotropic effects included and excluded. Estimates are also shown only for non-pleiotropic SNPs known to be functionally relevant to coffee metabolism21,22. As a sensitivity analysis, given the number of outcomes considered, adjustment was also made for multiple comparisons, using a Bonferroni corrected significance level of 0.002 (0.05/18) to account for testing 18 associations (coffee with four disease outcomes, 13 CVD risk factors and one control outcome).
The statistical analyses were conducted using Stata version 13.1 (StataCorp LP, College Station, TX) and R version 3.2.1 (R Foundation for Statistical Computing, Vienna, Austria).
Ethics approval
The methods were carried out in accordance with the approved guidelines. People of predominantly European descent were included in the study. Each study has been specifically approved by the Ethical Committees of the original studies and all the participants provided a written informed consent. This analysis of publicly available summary data does not require ethical approval.
Additional Information
How to cite this article: Kwok, M. K. et al. Habitual coffee consumption and risk of type 2 diabetes, ischemic heart disease, depression and Alzheimer’s disease: a Mendelian randomization study. Sci. Rep. 6, 36500; doi: 10.1038/srep36500 (2016).
Publisher’s note: Springer Nature remains neutral with regard to jurisdictional claims in published maps and institutional affiliations.
References
National Coffee Association. National Coffee Drinking Trends 2010, http://www.ncausa.org/Industry-Resources/Market-Research/National-Coffee-Drinking-Trends-Report (Date of access: April 28, 2016) (2010).
Michaud, D. S. et al. Coffee and tea intake and risk of brain tumors in the European Prospective Investigation into Cancer and Nutrition (EPIC) cohort study. Am J Clin Nutr 92, 1145–1150, doi: 10.3945/ajcn.2010.29876 (2010).
Institute for Scientific Information on Coffee. Can drinking coffee be healthy? Beliefs and behaviours across Europe, http://coffeeandhealth.org/wp-content/uploads/2016/01/Coffee-and-a-Healthy-Diet-European-Infographic-FINAL-1.pdf (2016). (Date of access: April 28, 2016).
United States Department of Health and Human Services & United States Department of Agriculture. 2015–2020 Dietary Guidelines for Americans. 8th Edition.http://health.gov/dietaryguidelines/2015/guidelines/ (Date of access: May 16, 2016) (2015).
Ding, M., Bhupathiraju, S. N., Chen, M., van Dam, R. M. & Hu, F. B. Caffeinated and decaffeinated coffee consumption and risk of type 2 diabetes: a systematic review and a dose-response meta-analysis. Diabetes Care 37, 569–586, doi: 10.2337/dc13-1203 (2014).
Liu, Q. P. et al. Habitual coffee consumption and risk of cognitive decline/dementia: A systematic review and meta-analysis of prospective cohort studies. Nutrition 32, 628–636, doi: 10.1016/j.nut.2015.11.015 (2016).
Guo, X. et al. Sweetened beverages, coffee, and tea and depression risk among older US adults. PLoS One 9, e94715, doi: 10.1371/journal.pone.0094715 (2014).
Grosso, G., Micek, A., Castellano, S., Pajak, A. & Galvano, F. Coffee, tea, caffeine and risk of depression: A systematic review and dose-response meta-analysis of observational studies. Mol Nutr Food Res 60, 223–234, doi: 10.1002/mnfr.201500620 (2016).
Wu, J. N. et al. Coffee consumption and risk of coronary heart diseases: a meta-analysis of 21 prospective cohort studies. Int J Cardiol 137, 216–225, doi: 10.1016/j.ijcard.2008.06.051 (2009).
Ding, M., Bhupathiraju, S. N., Satija, A., van Dam, R. M. & Hu, F. B. Long-term coffee consumption and risk of cardiovascular disease: a systematic review and a dose-response meta-analysis of prospective cohort studies. Circulation 129, 643–659, doi: 10.1161/CIRCULATIONAHA.113.005925 (2014).
Cai, L., Ma, D., Zhang, Y., Liu, Z. & Wang, P. The effect of coffee consumption on serum lipids: a meta-analysis of randomized controlled trials. Eur J Clin Nutr 66, 872–877, doi: 10.1038/ejcn.2012.68 (2012).
Wedick, N. M. et al. Effects of caffeinated and decaffeinated coffee on biological risk factors for type 2 diabetes: a randomized controlled trial. Nutr J 10, 93, doi: 10.1186/1475-2891-10-93 (2011).
Persson, J. et al. Sex-Specific Effects of Adiponectin on Carotid Intima-Media Thickness and Incident Cardiovascular Disease. J Am Heart Assoc 4, e001853, doi: 10.1161/JAHA.115.001853 (2015).
Ohnaka, K. et al. Effects of 16-week consumption of caffeinated and decaffeinated instant coffee on glucose metabolism in a randomized controlled trial. J Nutr Metab 2012, 207426, doi: 10.1155/2012/207426 (2012).
Correa, T. A. et al. Paper-filtered coffee increases cholesterol and inflammation biomarkers independent of roasting degree: a clinical trial. Nutrition 29, 977–981, doi: 10.1016/j.nut.2013.01.003 (2013).
Kempf, K. et al. Cardiometabolic effects of two coffee blends differing in content for major constituents in overweight adults: a randomized controlled trial. Eur J Nutr 54, 845–854, doi: 10.1007/s00394-014-0763-3 (2015).
Lawlor, D. A., Harbord, R. M., Sterne, J. A., Timpson, N. & Davey Smith, G. Mendelian randomization: using genes as instruments for making causal inferences in epidemiology. Stat Med 27, 1133–1163, doi: 10.1002/sim.3034 (2008).
Nordestgaard, A. T., Thomsen, M. & Nordestgaard, B. G. Coffee intake and risk of obesity, metabolic syndrome and type 2 diabetes: a Mendelian randomization study. Int J Epidemiol 44, 551–565, doi: 10.1093/ije/dyv083 (2015).
Drewnowski, A. & Rehm, C. D. Sources of Caffeine in Diets of US Children and Adults: Trends by Beverage Type and Purchase Location. Nutrients 8, doi: 10.3390/nu8030154 (2016).
The Coffee and Caffeine Genetics Consortium et al. Genome-wide meta-analysis identifies six novel loci associated with habitual coffee consumption. Mol Psychiatry 20, 647–656, doi: 10.1038/mp.2014.107 (2015).
Cornelis, M. C. et al. Genome-wide meta-analysis identifies regions on 7p21 (AHR) and 15q24 (CYP1A2) as determinants of habitual caffeine consumption. PLoS Genet 7, e1002033, doi: 10.1371/journal.pgen.1002033 (2011).
Amin, N. et al. Genome-wide association analysis of coffee drinking suggests association with CYP1A1/CYP1A2 and NRCAM. Mol Psychiatry 17, 1116–1129, doi: 10.1038/mp.2011.101 (2012).
Ebrahim, S. & Davey Smith, G. Mendelian randomization: can genetic epidemiology help redress the failures of observational epidemiology? Hum Genet 123, 15–33, doi: 10.1007/s00439-007-0448-6 (2008).
Mattar, R., de Campos Mazo, D. F. & Carrilho, F. J. Lactose intolerance: diagnosis, genetic, and clinical factors. Clin Exp Gastroenterol 5, 113–121, doi: 10.2147/CEG.S32368 (2012).
Haycock, P. C. et al. Best (but oft-forgotten) practices: the design, analysis, and interpretation of Mendelian randomization studies. Am J Clin Nutr 103, 965–978, doi: 10.3945/ajcn.115.118216 (2016).
Pierce, B. L. & Burgess, S. Efficient design for Mendelian randomization studies: subsample and 2-sample instrumental variable estimators. Am J Epidemiol 178, 1177–1184, doi: 10.1093/aje/kwt084 (2013).
CARDIoGRAMplusC4D Consortium et al. Large-scale association analysis identifies new risk loci for coronary artery disease. Nat Genet 45, 25–33, doi: 10.1038/ng.2480 (2013).
Schunkert, H. et al. Large-scale association analysis identifies 13 new susceptibility loci for coronary artery disease. Nat Genet 43, 333–338, doi: 10.1038/ng.784 (2011).
Morris, A. P. et al. Large-scale association analysis provides insights into the genetic architecture and pathophysiology of type 2 diabetes. Nat Genet 44, 981–990, doi: 10.1038/ng.2383 (2012).
Major Depressive Disorder Working Group of the Psychiatric Gwas Consortium et al. A mega-analysis of genome-wide association studies for major depressive disorder. Mol Psychiatry 18, 497–511, doi: 10.1038/mp.2012.21 (2013).
Lambert, J. C. et al. Meta-analysis of 74,046 individuals identifies 11 new susceptibility loci for Alzheimer’s disease. Nat Genet 45, 1452–1458, doi: 10.1038/ng.2802 (2013).
Ritchie, K. et al. The neuroprotective effects of caffeine: a prospective population study (the Three City Study). Neurology 69, 536–545, doi: 10.1212/01.wnl.0000266670.35219.0c (2007).
Global Lipids Genetics Consortium et al. Discovery and refinement of loci associated with lipid levels. Nat Genet 45, 1274–1283, doi: 10.1038/ng.2797 (2013).
Scott, R. A. et al. Large-scale association analyses identify new loci influencing glycemic traits and provide insight into the underlying biological pathways. Nat Genet 44, 991–1005, doi: 10.1038/ng.2385 (2012).
Soranzo, N. et al. Common variants at 10 genomic loci influence hemoglobin A(1)(C) levels via glycemic and nonglycemic pathways. Diabetes 59, 3229–3239, doi: 10.2337/db10-0502 (2010).
Schooling, C. M., Au Yeung, S. L. & Freeman, G. Mendelian randomization estimates may be inflated. J Am Coll Cardiol 61, 1931, doi: 10.1016/j.jacc.2012.12.049 (2013).
Burgess, S. et al. Using published data in Mendelian randomization: a blueprint for efficient identification of causal risk factors. Eur J Epidemiol 30, 543–552, doi: 10.1007/s10654-015-0011-z (2015).
Moran, A. E. et al. Variations in ischemic heart disease burden by age, country, and income: the Global Burden of Diseases, Injuries, and Risk Factors 2010 study. Glob Heart 9, 91–99, doi: 10.1016/j.gheart.2013.12.007 (2014).
Danaei, G. et al. National, regional, and global trends in fasting plasma glucose and diabetes prevalence since 1980: systematic analysis of health examination surveys and epidemiological studies with 370 country-years and 2.7 million participants. Lancet 378, 31–40, doi: 10.1016/S0140-6736(11)60679-X (2011).
Fulgoni, V. L. 3rd, Keast, D. R. & Lieberman, H. R. Trends in intake and sources of caffeine in the diets of US adults: 2001–2010. Am J Clin Nutr 101, 1081–1087, doi: 10.3945/ajcn.113.080077 (2015).
Schooling, C. M., Freeman, G. & Cowling, B. J. Mendelian randomization and estimation of treatment efficacy for chronic diseases. Am J Epidemiol 177, 1128–1133, doi: 10.1093/aje/kws344 (2013).
Davey Smith, G. & Ebrahim, S. Mendelian randomization: prospects, potentials, and limitations. Int J Epidemiol 33, 30–42, doi: 10.1093/ije/dyh132 (2004).
Strandhagen, E. & Thelle, D. S. Filtered coffee raises serum cholesterol: results from a controlled study. Eur J Clin Nutr 57, 1164–1168, doi: 10.1038/sj.ejcn.1601668 (2003).
Rowland, A. & Mangoni, A. A. Cytochrome P450 and ischemic heart disease: current concepts and future directions. Expert Opin Drug Metab Toxicol 10, 191–213, doi: 10.1517/17425255.2014.859675 (2014).
Dragin, N. et al. Phenotype of the Cyp1a1/1a2/1b1-/- triple-knockout mouse. Mol Pharmacol 73, 1844–1856, doi: 10.1124/mol.108.045658 (2008).
Wada, T. et al. Aryl Hydrocarbon Receptor Plays Protective Roles against High Fat Diet (HFD)-induced Hepatic Steatosis and the Subsequent Lipotoxicity via Direct Transcriptional Regulation of Socs3 Gene Expression. J Biol Chem 291, 7004–7016, doi: 10.1074/jbc.M115.693655 (2016).
Nikpay, M. et al. A comprehensive 1,000 Genomes-based genome-wide association meta-analysis of coronary artery disease. Nat Genet 47, 1121–1130, doi: 10.1038/ng.3396 (2015).
Solfrizzi, V. et al. Coffee Consumption Habits and the Risk of Mild Cognitive Impairment: The Italian Longitudinal Study on Aging. J Alzheimers Dis 47, 889–899, doi: 10.3233/JAD-150333 (2015).
Solfrizzi, V. et al. Lifestyle-related factors in predementia and dementia syndromes. Expert Rev Neurother 8, 133–158, doi: 10.1586/14737175.8.1.133 (2008).
Panza, F. et al. Coffee, tea, and caffeine consumption and prevention of late-life cognitive decline and dementia: a systematic review. J Nutr Health Aging 19, 313–328, doi: 10.1007/s12603-014-0563-8 (2015).
Santos, C., Costa, J., Santos, J., Vaz-Carneiro, A. & Lunet, N. Caffeine intake and dementia: systematic review and meta-analysis. J Alzheimers Dis 20 Suppl 1, S187–S204, doi: 10.3233/JAD-2010-091387 (2010).
Araujo, L. F. et al. Association of Coffee Consumption with MRI Markers and Cognitive Function: A Population-Based Study. J Alzheimers Dis, doi: 10.3233/JAD-160116 (2016).
Linder, A. E. et al. Vascular reactivity, 5-HT uptake, and blood pressure in the serotonin transporter knockout rat. Am J Physiol Heart Circ Physiol 294, H1745–H1752, doi: 10.1152/ajpheart.91415.2007 (2008).
Tajeddinn, W. et al. Association of Platelet Serotonin Levels in Alzheimer’s Disease with Clinical and Cerebrospinal Fluid Markers. J Alzheimers Dis, doi: 10.3233/JAD-160022 (2016).
Higdon, J. V. & Frei, B. Coffee and health: a review of recent human research. Crit Rev Food Sci Nutr 46, 101–123, doi: 10.1080/10408390500400009 (2006).
Oboh, G., Agunloye, O. M., Akinyemi, A. J., Ademiluyi, A. O. & Adefegha, S. A. Comparative study on the inhibitory effect of caffeic and chlorogenic acids on key enzymes linked to Alzheimer’s disease and some pro-oxidant induced oxidative stress in rats’ brain-in vitro. Neurochem Res 38, 413–419, doi: 10.1007/s11064-012-0935-6 (2013).
Dupuis, J. et al. New genetic loci implicated in fasting glucose homeostasis and their impact on type 2 diabetes risk. Nat Genet 42, 105–116, doi: 10.1038/ng.520 (2010).
Locke, A. E. et al. Genetic studies of body mass index yield new insights for obesity biology. Nature 518, 197–206, doi: 10.1038/nature14177 (2015).
Shungin, D. et al. New genetic loci link adipose and insulin biology to body fat distribution. Nature 518, 187–196, doi: 10.1038/nature14132 (2015).
Dastani, Z. et al. Novel loci for adiponectin levels and their influence on type 2 diabetes and metabolic traits: a multi-ethnic meta-analysis of 45,891 individuals. PLoS Genet 8, e1002607, doi: 10.1371/journal.pgen.1002607 (2012).
Benyamin, B. et al. Childhood intelligence is heritable, highly polygenic and associated with FNBP1L. Mol Psychiatry 19, 253–258, doi: 10.1038/mp.2012.184 (2014).
Burgess, S., Dudbridge, F. & Thompson, S. G. Combining information on multiple instrumental variables in Mendelian randomization: comparison of allele score and summarized data methods. Stat Med 35, 1880–1906, doi: 10.1002/sim.6835 (2016).
Han, B. et al. A general framework for meta-analyzing dependent studies with overlapping subjects in association mapping. Hum Mol Genet, 1–10, doi: 10.1093/hmg/ddw049 (2016).
Acknowledgements
The authors thank the Coffee and Caffeine Genetics Consortium, CARDIoGRAMplusC4D, DIAGRAM, GIANT, GLGC, ADIPOGen, MAGIC, PGC, IGAP and SSGAC Consortia for access to their GWAS data.
Author information
Authors and Affiliations
Contributions
M.K. Kwok performed the literature review, conducted data analysis, interpreted findings and drafted the manuscript. G.M. Leung interpreted findings and critically reviewed the manuscript. C.M. Schooling conceptualized ideas, designed and directed analytic strategy, interpreted findings, revised drafts of the manuscript critically and supervised the study from conception to completion.
Ethics declarations
Competing interests
The authors declare no competing financial interests.
Rights and permissions
This work is licensed under a Creative Commons Attribution 4.0 International License. The images or other third party material in this article are included in the article’s Creative Commons license, unless indicated otherwise in the credit line; if the material is not included under the Creative Commons license, users will need to obtain permission from the license holder to reproduce the material. To view a copy of this license, visit http://creativecommons.org/licenses/by/4.0/
About this article
Cite this article
Kwok, M., Leung, G. & Schooling, C. Habitual coffee consumption and risk of type 2 diabetes, ischemic heart disease, depression and Alzheimer’s disease: a Mendelian randomization study. Sci Rep 6, 36500 (2016). https://doi.org/10.1038/srep36500
Received:
Accepted:
Published:
DOI: https://doi.org/10.1038/srep36500
This article is cited by
-
Causal relationship from coffee consumption to diseases and mortality: a review of observational and Mendelian randomization studies including cardiometabolic diseases, cancer, gallstones and other diseases
European Journal of Nutrition (2022)
-
Dietary phytochemical index in relation to risk of glioma: a case-control study in Iranian adults
Nutrition Journal (2021)
-
Insulin resistance: a connecting link between Alzheimer’s disease and metabolic disorder
Metabolic Brain Disease (2021)
-
Dietary and policy priorities to reduce the global crises of obesity and diabetes
Nature Food (2020)
-
An atlas on risk factors for type 2 diabetes: a wide-angled Mendelian randomisation study
Diabetologia (2020)
Comments
By submitting a comment you agree to abide by our Terms and Community Guidelines. If you find something abusive or that does not comply with our terms or guidelines please flag it as inappropriate.