Abstract
We aimed to evaluate the association of four common polymorphisms (rs1800625, rs1800624, rs2070600 and rs184003) in receptor for advanced glycation end products (RAGE) gene to evaluate their epistatic influence on breast cancer risk in northeastern Han Chinese. This is a hospital-based case-control study involving 509 histologically-proven breast cancer patients and 504 cancer-free controls. The genotype and allele distributions of rs184003 differed significantly between patients and controls, even after the Bonferroni correction. Individuals carrying the rs184003 T allele exhibited 1.62-fold increased risk of breast cancer (odds ratio (OR) = 1.62; 95% confidence interval (95% CI): 1.26–2.08; P < 0.001) after adjusting for confounders. The frequency of haplotype T-T-G-T (alleles in order of rs1800625, rs1800624, rs2070600 and rs184003) was remarkably higher in patients than in controls (Simulated P = 0.001) and this haplotype was significantly associated with a 1.43-fold (95% CI: 1.01–2.01; P = 0.041) increase in adjusted risk of breast cancer. Further analysis indicated that there was synergistic interaction between rs184003 and rs2070600, whereas their joint information gain value was relatively small (0.27%). Taken together, although there was no suggestive evidence for the presence of epistasis in RAGE gene, our findings clearly demonstrate that rs184003 might play a predominant role in the development of breast cancer.
Similar content being viewed by others
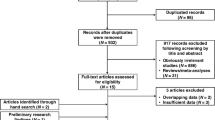
Introduction
Breast cancer is a complex disease that results from the inheritance of a number of susceptible genes1,2. Although exhaustive investigations from single-locus to genome-wide association studies have been conducted, to unravel the ultimate genetic underpinnings of breast cancer still remains a challengeable task. One potential obstacle facing global geneticists might be the overlook of epistasis3,4, which is defined as one locus masking the effect of another. In fact, epistasis is increasingly recognized as fundamentally important to better understanding the structure and function of genetic pathways and the evolutionary dynamics of complex genetic systems5. In such context, a promising data-mining technique multifactor dimensionality reduction (MDR) has been developed for collapsing high-dimensional genetic data into a single dimension and thus permitting interactions to be identified6,7. This technique is nonparametric and model-free in design and has been successfully applied to detect and characterize high-order gene-to-gene and gene-to-environment interactions in studies with relatively small samples8,9,10. To yield more information, we, in this study, sought to adopt the MDR technique to evaluate the epistatic influence of four well-characterized common polymorphisms (rs1800625, rs1800624, rs2070600 and rs184003) in the gene encoding receptor for advanced glycation end products (protein: RAGE; gene: RAGE) on the risk of having breast cancer in northeastern Han Chinese.
The selection of RAGE gene as a research candidate is biologically plausible. RAGE is a member of the immunoglobulin superfamily of cell surface receptors and its interaction with advanced glycation end products and other molecules plays a role in the pathogenesis of cancer progression and metastasis11. Converging evidence suggested that circulating RAGE level may be a novel clinical biomarker for many types of cancers, such as lung cancer, prostate cancer, colorectal cancer, breast cancer11,12,13,14,15. Experimental studies have demonstrated that to block RAGE signaling in mice can reduce the migration and invasivity of tumor cells and possibly cell proliferation and the production of tissue metalloproteinases16. Further studies that examined the genetic backgrounds of RAGE gene found that circulating RAGE level was largely determined by its genetic defects17,18,19. As RAGE genomic sequence is highly polymorphic, it is of interest to determine which genetic defects have a functional potential to affect the final bioavailability of RAGE and thus the development of breast cancer. On the basis of these observations, it is reasonable to speculate that RAGE gene might be a logical candidate involved in the underlying cause of breast cancer.
Results
Characteristics of study participants
As shown in Table 1, the mean age at enrollment was comparable between breast cancer patients and controls (P = 0.321). There were no differences between the two groups in terms of age of menarche (P = 0.915), menopausal status (P = 0.303) and BMI (P = 0.603). The proportions of histological grades of breast cancer were 5.22%, 50.50% and 44.28% for G1, G2 and G3, respectively.
Association of RAGE single polymorphisms with breast cancer
The observed genotype distributions of four examined polymorphisms in RAGE gene were consistent with the Hardy-Weinberg equilibrium in both breast cancer patients and controls (P > 0.05). The genotype distributions and allele frequencies of four polymorphisms in RAGE gene between patients and controls and their risk prediction for breast cancer are presented in Table 2. The genotype and allele distributions of rs184003 differed significantly between breast cancer patients and controls, even after the Bonferroni correction. The rs184003 TT genotype or T allele was overrepresented in patients relative to in controls and individuals carrying the rs184003 T allele exhibited a 1.62-fold increased risk of having breast cancer (OR = 1.62; 95% CI: 1.26–2.08; P < 0.001) compared with the GG genotype homozygotes after adjusting for age at enrollment, age of menarche, menopausal status and BMI. The power to reject the null hypothesis of no differences in the mutant allele frequencies of rs184003 between the two groups was 96.4%. In contrast, there were no significant differences for the genotype and allele distributions of the other three polymorphisms examined between the two groups (P > 0.05).
Association of RAGE haplotypes with breast cancer
The haplotypes are derived from four examined polymorphisms in RAGE gene and their frequencies (≥3%) are summarized in Table 3. The frequencies of haplotypes T-T-G-T (alleles in order of rs1800625, rs1800624, rs2070600 and rs184003) (Simulated P = 0.001) and T-T-A-T (Simulated P = 0.045) were remarkably higher in patients than in controls, whereas the frequency of haplotype T-A-G-G (Simulated P = 0.014) was remarkably lower. Accordingly, adjusted risk estimates indicated that compared with the most common haplytope T-T-G-G, whose frequencies were comparable between patients (32.44%) and controls (33.76%, Simulated P = 0.445), haplotype T-T-G-T was significantly associated with 1.43-fold increased risk for breast cancer (OR = 1.43; 95% CI: 1.01–2.01; P = 0.041) after adjusting for age at enrollment, age of menarche, menopausal status and BMI. The power to reject the null hypothesis of no differences in the frequencies of haplotype T-T-G-T between the two groups was 97.9%. In contrast, the risk estimates were nonsignificant for haplotypes T-A-G-G and T-A-G-G after adjustment for confounders.
Epistatic influence of RAGE polymorphisms on breast cancer
To evaluate the epistasis of four examined polymorphisms in RAGE gene on breast cancer risk, a promising data-mining analytical approach MDR was employed to identify and characterize the potential interactions of these polymorphisms (Table 4). For each best model, testing accuracy, cross-validation consistency and the permutated significance level are provided. There was no statistical significance for all derived one- to four-locus models. The overall best one-locus model was rs184003 with the maximal cross-validation consistency of 10 out of 10 and the testing accuracy of 0.559, secondary to that of the overall best two-locus model including rs184003 and rs2070600 with the testing accuracy of 0.567.
To visually inspect epistasis in RAGE gene, Figure 1 depicts the interaction graph of four examined polymorphisms according to the entropy measures. The independent main effect was largest for rs184003 with the information gain value of 1.10%. There was synergistic interaction as indicated by the red line between rs184003 and rs2070600, whereas their joint information gain value was relatively small (0.27%). Besides, the interaction of rs184003 with the other two polymorphisms was negative, suggesting the redundant effects.
Discussion
In this study, we focused on four well-characterized polymorphisms of RAGE gene and examined their epistatic influence on breast cancer risk in northeastern Han Chinese. Even with the data-mining MDR technique, we still failed to produce any suggestive evidence for the presence of epistasis in RAGE gene in predisposition to breast cancer. Instead, our findings clearly demonstrate that rs184003 might play a predominant role in the development of breast cancer. To our knowledge, there are currently no reports concerning the possible candidacy of RAGE gene in breast cancer among Chinese.
More recently, Hashemi and colleagues have examined three polymorphisms (rs1800625, rs1800624 and 63 bp Insertion/deletion) of RAGE gene among 71 breast cancer patients and 93 healthy controls of Middle Eastern ethnicity and they failed to demonstrate any association between these three polymorphisms and breast cancer27, which was likely due to the possible small effect of these polymorphisms on breast cancer risk and the relatively small sample sizes involved. Consistent with the finding of the present study, there was also no evident association between RAGE gene two promoter polymorphisms (rs1800625 and rs1800624) and breast cancer risk among 509 patients and 504 cancer-free controls. Besides this finding, we observed a significant, independent relationship between RAGE gene rs184003 and breast cancer risk in our single-locus analyses. Notably, the predominant role played by rs184003 was also implicated in our further haplotype and epistasis analyses.
Haplotype is defined as a combination of multiple alleles on one chromosome and so haplotype analysis could provide more information than single-locus analysis28. In this study, only haplotype T-T-G-T was identified to be associated with the significant risk of breast cancer after adjusting for confounders. This haplotype was composed of the risky T allele of rs184003 and other derived haplotypes possessing this allele were consistently overrepresented in breast cancer patients relative to controls and vice versa, suggesting a leading role of rs184003 in risk determination. To produce more information, we employed a promising data-mining MDR technique to explore the epistatic influence of RAGE genetic polymorphisms from statistical and graphic aspects. However, we failed to produce any suggestive evidence for the presence of epistasis in predisposition to breast cancer. Instead, the contributory role of rs184003 was observed to be offset by the other polymorphisms, which may in part explain the nonsignificant prediction of a majority of derived haplotypes for breast cancer. Nevertheless, because rs184003 is an intronic locus, it is unlikely to be functional, but might act as a surrogate for other linked functional loci in the regulatory regions of RAGE gene. Considering the fact that evolutionary history of linkage disequilibrium patterns will vary significantly in different ethnic populations20, there is a need to construct a database of breast cancer-susceptibility genes or polymorphisms in each ethnic group. These findings not only deepen our understandings that complex genetic interactions might account for disease susceptibility, but also yield strong evidence in favor of the logical candidacy of RAGE gene in the pathogenesis of breast cancer.
Several limitations of this study merit special consideration. First, the retrospective design of this study has inherent drawbacks and precludes causal inferences29. Second, this study of 1013 participants may be underpowered to demonstrate small risk effects. However, a sample size calculation enabled us to have more than 96% statistical power to detect significant differences between patients and controls. Third, only four polymorphisms were examined in this study and it is highly encouraged to incorporate other polymorphisms, especially the low-penetrance polymorphisms in RAGE gene or other candidate genes, such as genes from the renin-angiotensin system30,31. Fourth, the fact that our study subjects were of northeastern Han Chinese descent limited the generalizability of our findings, calling for further confirmation in other ethnic populations.
In summary, although there was no suggestive evidence for the presence of epistasis in RAGE gene, our findings clearly demonstrate that rs184003 might play a predominant role in the development of breast cancer. As breast cancer is a multifactorial complex trait, more emphases should be placed on the detection and characterization of multiple genetic and environmental interactions to predict high-risk individuals for the prevention and personalized treatment of breast cancer.
Methods
Study participants
All study participants were females of Han Chinese descent and they were local residents of Qiqihar city, Heilongjiang province, China. In total, there were 1013 unrelated participants in this study that were enrolled from two hospitals in Qiqihar city. Ethical approval was obtained from the ethics committee of Qiqihar Medical University and before entering this study all participants gave informed written consent according to the guidelines of the Declaration of Helsinki.
Of 1013 study participants, 509 were histologically-proven breast cancer patients and the remaining 504 participants formed the age-matched cancer-free controls.
From each participant, data on age (the onset age of breast cancer for patients), family history of cancers, age of menarche, menopausal status (yes or no), body weight and height (measured in light clothes and in bare feet) and the histological grade of breast cancer (from G1 to G3 for breast cancer patients only) were recorded at enrollment. Body mass index (BMI) was calculated as weight (in kilograms) divided by height (in meters) squared.
Polymorphism selection
In this study, the selection of four well-characterized common polymorphisms from RAGE gene is founded on their wide associations with vascular complications15,20,21. The four polymorphisms were rs1800625 or T-429C in promoter region, rs1800624 or T-374A in promoter region, rs2070600 or Gly82Ser in 3rd exon and rs184003 or G1704A in 7th intron.
Genotype determination
Genomic DNA was isolated from peripheral blood leukocytes by TIANamp Blood DNA Kit (Tiangen Biotect (Beijing) Co., China). The polymerase chain reaction - ligase detection reaction (PCR-LDR) method22 was used to determine the genotypes of four examined polymorphisms.
For each polymorphism, two specific probes were synthesized to discriminate specific bases with one common probe labeled with 6-carboxy-fluorescein (FAM) at the 3′ end and with horylated at the 5′ end. The multiplex ligation reaction was conducted in a reaction volume of 10 μl containing 2 μl of PCR product, 1 μl of 10 × Taq DNA ligase buffer, 1 μM of each discriminating probe and 5 U of Taq DNA ligase. The ligation parameters were 30 cycles of 94°C for 30 s and 56°C for 3 min. After the reaction, 1 μl of LDR reaction product was mixed with 1 μl of ROX passive reference and 1 μl of loading buffer before being denatured at 95°C for 3 minutes and chilled rapidly in ice water. The fluorescent products of the LDR were differentiated using an ABI 3730XL sequencer (Applied Biosystems, USA).
The accuracy of PCR-LDR method was validated by additionally genotyping 96 samples that were randomly selected from all study samples as internal references and the resultant genotypes showed no differences.
Statistical analysis
All recorded variables were presented as mean (standard deviation or SD) or percentage and were compared between patients and controls by the unpaired Student's t-test for continuous variables and by the Pearson χ2 test for categorical variables. Hardy-Weinberg equilibrium was tested at each locus on a contingency table of observed-versus-expected genotypic frequencies using the χ2 test. A two-tailed probability value of <0.05 was considered significant. The Bonferroni method was used to adjust the significant P values for multiple comparisons. Simple statistical analyses were conducted by the STATA software (StataCorp, Texas, USA, version 11.2 for Windows). Study power was estimated by the Power and Sample Size Calculations (PS) software (v3.0.7)23.
The genotypes of four examined polymorphisms in RAGE gene were explored by binary Logistical regression analyses under the assumptions of additive (major homozygotes versus heterozygotes versus minor homozygotes), dominant (major homozygotes versus heterozygotes plus minor homozygotes) and recessive (major homozygotes plus heterozygotes versus minor homozygotes) models of inheritance after adjusting for age at enrollment, age of menarche, menopausal status and BMI.
To evaluate the joint effects of four examined polymorphisms in RAGE gene, Haplo.Stats software (version 1.4.0) was employed to construct haplotypes between patients and controls. This software is operated in the R language (version 2.14, http://www.r-project.org). The haplo.em program, which computes the maximum likelihood estimates of haplotype probabilities using the progressive insertion algorithm which progressively inserts batches of loci into haplotypes of growing lengths, was used to estimate haplotype frequencies from four polymorphisms. To avoid chance findings, only common haplotypes with frequencies ≥3% in all study participants were analyzed. The other two programs, haplo.cc and haplo.glm, which are based on a generalized linear model and compute the regression of a trait on haplotypes and other covariates24, were used to calculate the adjusted odds ratios (ORs) and 95% confidence intervals (CIs) for each haplotype. P values were simulated based on 1000 replicates.
To evaluate the epistasis of four examined polymorphisms on breast cancer risk, an open-source MDR technique (version 3.0, www.epistasis.org)25,26 was adopted. All possible combinations of four polymorphisms were constructed by MDR constructive induction. The accuracy of each model was evaluated by a Bayes classifier in the context of 10-fold cross-validation. A single best model simultaneously has the maximal testing accuracy and cross-validation consistency. The cross-validation consistency is a measure of the number of times of 10 divisions of the dataset that the best model is extracted. Statistical significance was evaluated using a 1000-fold permutation test to compare observed testing accuracies with those expected under the null hypothesis of null association. Permutation testing corrects for multiple testing by repeating the entire analysis on 1000 datasets that are consistent with the null hypothesis.
Interaction entropy graph, which can visually inspect potential epistasis, was depicted to quantify the synergistic and non-synergistic interactions among polymorphisms. This graph is implemented in MDR software. In this graph, information gain values expressed as percentages in the nodes signify the independent main effect of the polymorphisms. The positive and negative information gain values (percentages) on the connected lines indicate synergistic interaction (the red or the orange line) and redundancy (lack of interaction, the green and the blue line) between the polymorphisms, respectively with the zero value indicating independence (the yellow line).
References
Warren Andersen, S. et al. The associations between a polygenic score, reproductive and menstrual risk factors and breast cancer risk. Breast Cancer Res Treat 140, 427–434 (2013).
Niu, W., Qi, Y., Gao, P. & Zhu, D. Association of TGFB1 -509 C > T polymorphism with breast cancer: evidence from a meta-analysis involving 23,579 subjects. Breast Cancer Res Treat 124, 243–249 (2010).
Sapkota, Y. et al. Assessing SNP-SNP interactions among DNA repair, modification and metabolism related pathway genes in breast cancer susceptibility. PLoS One 8, e64896 (2013).
Oestergaard, M. Z. et al. Interactions between genes involved in the antioxidant defence system and breast cancer risk. British journal of cancer 95, 525–531 (2006).
Phillips, P. C. Epistasis--the essential role of gene interactions in the structure and evolution of genetic systems. Nat Rev Genet 9, 855–867 (2008).
Moore, J. H. Computational analysis of gene-gene interactions using multifactor dimensionality reduction. Expert Rev Mol Diagn 4, 795–803 (2004).
Gui, J. et al. A Simple and Computationally Efficient Approach to Multifactor Dimensionality Reduction Analysis of Gene-Gene Interactions for Quantitative Traits. PLoS One 8, e66545 (2013).
Guo, S. et al. Synergistic association of PTGS2 and CYP2E1 genetic polymorphisms with lung cancer risk in northeastern Chinese. PLoS One 7, e39814 (2012).
Guo, S. et al. The relationship between XRCC1 and XRCC3 gene polymorphisms and lung cancer risk in northeastern Chinese. PLoS One 8, e56213 (2013).
Pan, H. et al. Contributory role of five common polymorphisms of RAGE and APE1 genes in lung cancer among Han Chinese. PLoS One 8, e69018 (2013).
Tesarova, P. et al. Receptor for advanced glycation end products (RAGE)--soluble form (sRAGE) and gene polymorphisms in patients with breast cancer. Cancer Invest 25, 720–725 (2007).
Jing, R., Cui, M., Wang, J. & Wang, H. Receptor for advanced glycation end products (RAGE) soluble form (sRAGE): a new biomarker for lung cancer. Neoplasma 57, 55–61 (2010).
Ishiguro, H. et al. Receptor for advanced glycation end products (RAGE) and its ligand, amphoterin are overexpressed and associated with prostate cancer development. The Prostate 64, 92–100 (2005).
Jiao, L. et al. Advanced glycation end products, soluble receptor for advanced glycation end products and risk of colorectal cancer. Cancer Epidemiol Biomarkers Prev 20, 1430–1438 (2011).
Zhang, S. et al. Polymorphisms of receptor for advanced glycation end products and risk of epithelial ovarian cancer in Chinese patients. Cellular physiology and biochemistry: international journal of experimental cellular physiology, biochemistry and pharmacology 31, 525–531 (2013).
Taguchi, A. et al. Blockade of RAGE-amphoterin signalling suppresses tumour growth and metastases. Nature 405, 354–360 (2000).
Catalano, M. et al. The Pro12Ala polymorphism of peroxisome proliferator-activated receptor-gamma2 gene is associated with plasma levels of soluble RAGE (Receptor for Advanced Glycation Endproducts) and the presence of peripheral arterial disease. Clinical biochemistry 41, 981–985 (2008).
Ng, Z. X., Kuppusamy, U. R., Iqbal, T. & Chua, K. H. Receptor for advanced glycation end-product (RAGE) gene polymorphism 2245G/A is associated with pro-inflammatory, oxidative-glycation markers and sRAGE in diabetic retinopathy. Gene 521, 227–233 (2013).
Cheng, D. T. et al. Systemic sRAGE is a Biomarker of Emphysema and Associated with AGER Genetic Variants in COPD Patients. Am J Respir Crit Care Med (2013).
Niu, W. et al. A meta-analysis of receptor for advanced glycation end products gene: four well-evaluated polymorphisms with diabetes mellitus. Molecular and cellular endocrinology 358, 9–17 (2012).
Forbes, J. M. et al. Receptor for advanced glycation end-products (RAGE) provides a link between genetic susceptibility and environmental factors in type 1 diabetes. Diabetologia 54, 1032–1042 (2011).
Khanna, M. et al. Multiplex PCR/LDR for detection of K-ras mutations in primary colon tumors. Oncogene 18, 27–38 (1999).
Neeper, M. et al. Cloning and expression of a cell surface receptor for advanced glycosylation end products of proteins. The Journal of biological chemistry 267, 14998–15004 (1992).
Stram, D. O. et al. Modeling and E-M estimation of haplotype-specific relative risks from genotype data for a case-control study of unrelated individuals. Hum Hered 55, 179–190 (2003).
Pattin, K. A. et al. A computationally efficient hypothesis testing method for epistasis analysis using multifactor dimensionality reduction. Genet Epidemiol 33, 87–94 (2009).
Hahn, L. W., Ritchie, M. D. & Moore, J. H. Multifactor dimensionality reduction software for detecting gene-gene and gene-environment interactions. Bioinformatics 19, 376–382 (2003).
Hashemi, M. et al. Genotyping of -374A/T, -429A/G and 63 bp Ins/del polymorphisms of RAGE by rapid one-step hexaprimer amplification refractory mutation system polymerase chain reaction in breast cancer patients. Nucleosides, nucleotides & nucleic acids 31, 401–410 (2012).
Ribas, G., Milne, R. L., Gonzalez-Neira, A. & Benitez, J. Haplotype patterns in cancer-related genes with long-range linkage disequilibrium: no evidence of association with breast cancer or positive selection. Eur J Hum Genet 16, 252–260 (2008).
Gu, M. et al. Strong association between two polymorphisms on 15q25.1 and lung cancer risk: a meta-analysis. PLoS One 7, e37970 (2012).
Fishchuk, L. E. & Gorovenko, N. G. Genetic polymorphisms of the renin-angiotensin system in breast cancer patients. Exp Oncol 35, 101–104 (2013).
Xi, B. et al. Association between polymorphisms of the renin-angiotensin system genes and breast cancer risk: a meta-analysis. Breast Cancer Res Treat 130, 561–568 (2011).
Acknowledgements
This study was financially supported by the Natural Science Foundation of Heilongjiang Province (41404115-2-13051 and C201320), the Project of Department of Education of Heilongjiang Province (Grant number: 12531780) and the Opening Project of Key Laboratory of Antitumor Nature Products R&D, College of Heilongjiang Province.
Author information
Authors and Affiliations
Contributions
H.P. and W.N. conceived and designed the experiments; H.P. and L.H. performed the experiments; W.N. analyzed the data; H.P., L.H. and B.W. contributed materials/analysis tools; H.P. and W.N. wrote the manuscript. All authors reviewed and approved the manuscript prior to submission.
Ethics declarations
Competing interests
The authors declare no competing financial interests.
Rights and permissions
This work is licensed under a Creative Commons Attribution-NonCommercial-NoDerivs 3.0 Unported License. To view a copy of this license, visit http://creativecommons.org/licenses/by-nc-nd/3.0/
About this article
Cite this article
Pan, H., He, L., Wang, B. et al. The relationship between RAGE gene four common polymorphisms and breast cancer risk in northeastern Han Chinese. Sci Rep 4, 4355 (2014). https://doi.org/10.1038/srep04355
Received:
Accepted:
Published:
DOI: https://doi.org/10.1038/srep04355
This article is cited by
-
RAGE and Its Ligands: Molecular Interplay Between Glycation, Inflammation, and Hallmarks of Cancer—a Review
Hormones and Cancer (2018)
-
Effect of RAGE gene polymorphisms and circulating sRAGE levels on susceptibility to gastric cancer: a case–control study
Cancer Cell International (2017)
-
Association of RAGE polymorphisms and cancer risk: a meta-analysis of 27 studies
Medical Oncology (2015)
Comments
By submitting a comment you agree to abide by our Terms and Community Guidelines. If you find something abusive or that does not comply with our terms or guidelines please flag it as inappropriate.