Abstract
Lightning strikes, snow, and wind are common causes of power system failures. Their frequency of occurrence varies depending on weather patterns and seasons. Here, we analyse the occurrence of thirty pre-defined daily weather patterns along with nearly 70,000 power system failures in the United Kingdom between 2010 and 2019 to investigate the predictability of weather-induced power outages. We identify high-risk weather patterns and pattern transitions for all seasons. Winter weather patterns characterized by high wind speeds and high precipitation volumes are responsible for many instances of power outages caused by wind, gale, and lightning strikes. Weather patterns with moderate to high snowfall are often linked to power outages caused by snow and ice. Because high-risk weather patterns can be forecast reliably with valuable lead time, clear links between specific patterns and transitions can significantly improve the preparedness of the United Kingdom and broader European distribution network operators for adverse weather conditions.
Similar content being viewed by others
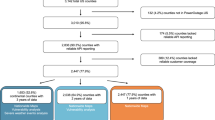
Introduction
Power outages caused by adverse weather conditions have negative impacts on economies and societies worldwide. Overall, adverse weather conditions are among the most common causes of power system faults and reliability problems and, in extreme cases, have caused the most widespread blackouts in history. One recent example from the United Kingdom developed after a lightning event in August 2019. As a result, part of the network was disconnected for a short period of time, but triggered a series of cascading effects that resulted in two large generators becoming disconnected from the network and the inability to meet demand. This caused widespread disruptions leaving over a million users without power for over 2 days and the overall costs are estimated to have exceeded £15M1. Power outages caused by weather phenomena (e.g., lightning strikes, snow and ice, wind and gale, flooding) include both high impact, low probability events like this one, but also low impact, high probability events, which evinces that power systems are vulnerable to an undesirably wide range of weather conditions. To reduce the impact of adverse weather conditions on power systems, the conditions in which weather-induced power outages may occur must be well understood so that system preparedness can be improved.
In previous works, the probability of occurrence of power outages caused by extreme weather was obtained from exposure and fragility modelling of individual system components. This approach represents the probability of failure of a given system component as a function of its level of exposure to weather variables over time, with applications to distinct extreme weather event categories (e.g., refs. 2,3). To this extent, some studies used information about representative extreme weather scenarios to determine the operating and damage statuses of specific power system components (e.g., refs. 4,5). Other pieces of research also investigated the effects of adverse weather on power system reliability and failure rates (e.g., refs. 6,7). Some works considered the spatiotemporal and inter-annual variability of weather for a cost-effective long-term planning of power systems with high shares of renewable energy (see refs. 8,9,10). However, none of these efforts considered that the occurrence of different types of weather-induced power system failures is related to specific large-scale atmospheric circulation types, which we refer to here as weather patterns.
Weather patterns have been widely used for over a decade as a way to isolate the most predictable components of atmospheric circulation (e.g., ref. 11). In turn, these patterns can be linked to their typical surface impacts both with the purpose of identifying relevant connections but also to exploit the predictability of the circulation patterns, which are generally easier to predict than the impacts themselves12,13,14. Promising results have been obtained from the use of such patterns in prediction at sub-seasonal scales—a few weeks ahead15,16,17.
To prepare for widespread power outages induced by extreme weather events, some efforts relied on weather forecasts, under the assumption they are sufficiently skilful (e.g., refs. 18,19). Other works identified the meteorological conditions which led to major power outages with the objective of providing reliable meteorological data for distribution network operators (DNOs) to implement effective adaptation strategies (e.g., ref. 20). In the UK,21 looked at relationships between wind gust speed and fault incidents caused by wind and gale, whereas22 performed spatiotemporal clustering of power system failures caused by distinct weather phenomena to look at the potential impacts of climate change on those failures. Nevertheless, none of these works looked at relationships between weather patterns and power system failures with the objective of identifying relevant trends to predict and prepare for power outages.
In this context, this paper utilizes an existing set of 30 Met Office daily weather pattern definitions23, derived with several applications in mind. Primarily, they are used within operational weather forecasting in order to identify the most likely weather patterns and pattern transitions within the forecast period, covering anything from a few days ahead, out to several weeks in advance23. However, the real utility of these forecasts becomes apparent once the weather patterns are related to specific impacts of interest, such as coastal flooding24, fluvial flooding25, lightning activity26, and flow from Iceland into UK airspace given the occurrence of a disruptive volcanic eruption27. The principle here is that once weather pattern characteristics are understood, in terms of their climatologies or impacts, it then becomes possible to interpret forecast output and describe likely consequences. Specifically related to this paper, the framework proposed by28 incorporated information from the Met Office weather patterns to perform a probabilistic impact assessment of lightning strikes on power systems. As well as the weather forecasting time-scales described above, these weather patterns are also proving useful in the interpretation of climate change projections, for example by assessing future changes to weather pattern occurrences29 and then relating these to changes in UK autumn precipitation30 and coastal flooding31.
With the objective of identifying relevant trends to reduce the impact of weather incidents on power systems, this article investigates the relationships between weather patterns and weather-induced power outages in the UK. Power system failures caused by distinct weather phenomena provided by the National Fault Interruption Reporting Scheme (NaFIRS) are examined against the set of 30 Met Office weather patterns using daily data collected between 2010 and 2019. Applications of this work are also discussed, including their use as a decision support tool in reliability and resilience enhancement investment, based on expected changes in the frequency of distinct weather-induced power outages in future climate scenarios.
Main text
Case study
In this section, the method incorporates information about power system failures provided by NaFIRS data from 1 January 2010 to 31 December 2019 in Great Britain (as mapped in Fig. 1) and weather patterns provided by the Met Office during the same period32. This information is used to compute the frequency of weather-induced outages by weather phenomenon, weather pattern, and season (Eq. (1) in the methodology section), then the strength of trends in weather-induced power outages and weather pattern transitions (Eqs. (5) and (6) in the methodology section). A breakdown of weather-induced power system failures per weather pattern in winter in the UK is shown in Fig. 2. Similarly, a breakdown of weather-induced power system failures per weather pattern in spring, summer, and fall in the UK is provided in Supplementary Figs. 2–4 of Supplementary Note 1.2.2.
In solid yellow: Southern Scotland (top region) and Northeast of England (middle and bottom regions). License area contours obtained from ref. 40.
The conditional probability of a next-day weather pattern for a given weather pattern and season is obtained from the updated daily historical weather pattern classifications provided by the Met Office32. These probabilities in winter are shown in Fig. 3 and represent daily weather pattern changes (including transitions and persistence) from 1950 to 2020, based on ref. 27. Similarly, the long-term probability of occurrence of a next-day weather pattern in spring, summer, and fall is shown in Supplementary Figs. 5–7 of Supplementary Note 1.3. This information is then used to calculate the probability of occurrence of a weather pattern over time (Eqs. (3) and (4)) in “Analysis of trends in power outages and changes in weather patterns”.
Note that the strength of trends in weather-induced power outages and weather pattern transitions is relative to the frequency of power outages attributed to a specific weather phenomenon by season. Thus, this work investigates the most frequent power outages caused by different weather phenomena by season (as listed in Table 1) to identify high-risk weather patterns and pattern transitions, regardless of their impact on supply interruption (in terms of customer interruptions, lost demand, and interruption duration). This choice is motivated by the better indication of mid- to long-term trends provided by a large sample size, considering that the majority of power system failures is expected to represent low impact, high probability events (as shown in Supplementary Note 1.2).
Frequency of weather-induced power system failures by weather pattern and season
In this section, only the top three causes of power system failures in winter are further investigated. This choice is motivated by the better indication of mid- to long-term trends provided by a large sample size and the fact that the top three causes of power outages per season represent roughly 95% of the total. Thereby, trends associated with lightning strikes (3020 occurrences), wind and gale (18,546 occurrences), and snow and ice (2198 occurrences) in winter are investigated.
The frequency of occurrence of a given weather pattern up to 2 weeks ahead of a power outage in winter shown in Fig. 4 indicate the main trends in power outages induced by different weather phenomena in the UK. The main trends in power outages induced by wind and gale (Fig. 4a) relate to weather patterns 26 (a very cyclonic north-westerly type) and 30 (a very cyclonic westerly type), as over 50% of outages occurred during or a few days after these weather patterns. Likewise, over 50% of outages caused by lightning strikes (Fig. 4b) occurred during or the day after weather pattern numbers 20 (cyclonic westerly), 23 (unbiased westerly), 26 (very cyclonic north-westerly), and 30 (very cyclonic westerly). In turn, the main trend in power outages induced by snow and ice (Fig. 4c) relates to weather pattern 27 (an anticyclonic easterly type), as nearly a third of outages occurred a few days after this weather pattern in winter. Similarly, the frequency of occurrence of a given weather pattern up to 2 weeks ahead of a power outage in spring, summer, and fall is shown in Supplementary Figs. 8–10 of Supplementary Discussion 1.5. The main trends in power outages induced by wind and gale, lightning strikes, and snow and ice in spring, summer, and fall are also discussed in Supplementary Discussion 1.4.
The 2-dimensional histograms show the frequency of occurrence of a given weather pattern up to 2 weeks ahead of the occurrence of a weather-induced power outage. The x-axis indicates the number of days ahead of an outage (day zero corresponds to the day when an outage occurs). The y-axis indicates the weather pattern reference number from 1 to 30. The colour scale indicates the frequency of occurrence of a given weather pattern as a function of the number of days ahead of an outage, with light yellow indicating high frequency values and dark blue indicating low frequency values for each individual subplot. a Top 1 winter: wind and gale (GB); b Top 2 winter: lightning strikes (GB); c Top 3 winter: snow and ice (GB); d Top 1 winter: wind and gale (NE); e Top 2 winter: lightning strikes (NE); f Top 3 winter: snow and ice (NE); g Top 1 winter: wind and gale (SS); h Top 2 winter: lightning strikes (SS); i: Top 3 winter: snow and ice (SS).
The GB NaFIRS dataset has the limitation that multiple locations could have reported faults related to the same event. This has the potential to lead to spuriously inflated connections between patterns and faults. To counteract this effect, two complementary shorter NaFIRS datasets corresponding to specific regions are included in the analysis, with the aim of reducing the effect of repeated faults: in the Northeast of England from 2004 to 2021 and in Southern Scotland from 2017 to 2022. A map of the corresponding DNO license areas is provided in Fig. 1.
Although DNOs are required to report power system failures to NaFIRS, they are not obliged to make their datasets publicly available. As the datasets used in this study were shared on a voluntary basis, the selection of time periods for this study is different across regions. Nevertheless, the effort of looking at different regions in this section is made to ensure that the trends obtained from the GB NaFIRS dataset are robust and any observed differences can be documented as sources of uncertainty in the results.
In the Northeast of England, the main trends in power outages induced by wind and gale (Fig. 4d) relate to weather patterns 20 (cyclonic westerly), 23 (unbiased westerly), 26 (very cyclonic north-westerly) and 30 (very cyclonic westerly), as over 50% of outages occurred during or a few days after these weather patterns. These results are similar to those shown in Fig. 4a, but with a higher contribution from weather patterns 20 and 23. Likewise, the majority of power outages caused by lightning strikes (Fig. 4e) occurred during or the day after weather pattern numbers >15, as in Fig. 4b. However, outages occurred during or the day after weather pattern numbers 19 (unbiased northerly), 20 (cyclonic westerly), 23 (unbiased westerly), 26 (very cyclonic north-westerly) and 30 (very cyclonic westerly) in nearly 50% of the cases. In turn, the main trend in power outages induced by snow and ice (Fig. 4f) relates to weather pattern 27 (anticyclonic easterly), as roughly a fifth of outages occurred a few days after this weather pattern in winter. This result agrees with Fig. 4c, but shows slightly different contributions from main and secondary weather patterns (e.g., weather patterns 19 (unbiased northerly) and 28 (southerly tracking low undercutting a cold pool over the UK)).
In Southern Scotland, the main trends in power outages induced by wind and gale (Fig. 4g) relate to weather patterns 20 (cyclonic westerly), 26 (very cyclonic south-westerly) and 30 (very cyclonic westerly), as roughly a third of outages occurred during or a few days after these weather patterns. These results are similar to those shown in Fig. 4a, but with a higher contribution from weather pattern 20. Likewise, over a third of outages caused by lightning strikes (Fig. 4h) occurred during or the day after weather pattern number 26 (very cyclonic south-westerly). This finding agrees with Fig. 4b, as over 10% of the outages induced by lightning strikes in Great Britain occurred during weather pattern number 26. In turn, the main trends in power outages induced by snow and ice (Fig. 4i) relate to weather patterns 26 (very cyclonic south-westerly) and 27 (anticyclonic easterly), as roughly a quarter of outages occurred during or a few days after these weather patterns in winter. This result partly agrees with Fig. 4c, as weather pattern number 27 is also in the main trends.
Analysis of trends in power outages and changes in weather patterns
In this section, the main trends associated with the outages described in “Frequency of weather-induced power system failures by weather pattern and season” are further evaluated in relation to the most likely proceeding weather pattern changes (including transitions and persistence). The Sankey diagrams in Fig. 5 show the breakdown of weather pattern transitions and persistence associated with the most common causes of weather-induced power outages in winter. Only the overall trends associated with weather-induced power outages in Great Britain are further investigated, given the similarities between the results shown in “Frequency of weather-induced power system failures by weather pattern and season”. This choice is also motivated by the larger sample size of fault incidents in the GB NaFIRS dataset compared to the regional datasets. Similarly, a breakdown of weather pattern transitions and persistence associated with the most common causes of weather-induced power outages in spring, summer, and fall is shown in Supplementary Figs. 11–13 of Supplementary Discussion 1.5.
The Sankey diagrams show the breakdown of weather pattern changes (including transitions and persistence) associated with the most common causes of weather-induced power outages. For improved readability, weather pattern numbers whose contributions are <5% are not shown in the Sankey diagrams. a Top 1 winter: wind and gale; b Top 2 winter: lightning strikes; c Top 3 winter: snow and ice.
The seasonal weather pattern transition / persistence matrix for winter (Fig. 3) shows that in most cases, the occurrence of a given weather pattern is most likely to persist the following day. However, there are also multiple other likely transitions which tend to be between similar types. For example, in winter a very common transition with a 17.65% probability is from weather pattern 20 (cyclonic westerly) to weather pattern 26 (cyclonic south-westerly) (Fig. 3), which represents the eastward movement of a large and deep low pressure system to the north of the UK. Although these represent different patterns, both would bring stormy and wet conditions, particularly to northern and western parts of the UK. Therefore, transitions between high-impact types, or the persistence of high-impact types is an important consideration when understanding the most likely weather patterns which lead to weather-induced power outages. Additionally, knowing that high-impact types tend to transition between each other or to have high persistence would improve the potential predictive skill of a pattern-conditioned forecasting system. For example, a forecasting system might have small error in forecasting the location of a weather system, but it could still project onto the high-risk patterns set (e.g., ref. 17).
The main trends in power outages induced by wind and gale (Fig. 5a) are represented by 26 → 26 (a persisting stormy south-westerly type) and 30 → 30 (a persisting stormy westerly type); note that the next-day weather pattern changes 20 → 20, 20 → 26, 20 → 30, 30 → 26, 23 → 20, 23 → 23, and 23 → 26 are also important—these also tend to represent weather patterns with an unsettled westerly, north-westerly or south-westerly flow over the UK. In turn, the main trends in power outages induced by lightning strikes (Fig. 5b) are represented by 20 → 20 (a persisting cyclonic westerly type), 23 → 23 (a persisting unbiased westerly type), 26 → 26 (a persisting cyclonic north-westerly type), and 30 → 30 (a persisting very cyclonic westerly type), which are all unstable westerly types with strong winds. For power outages induced by snow and ice (Fig. 5c), the main trends are represented by 24 → 24 (a persisting cyclonic northerly type), 20 → 26 (a transitioning west to north-westerly type), 27 → 27 (a persisting anticyclonic easterly type), 27 → 28 (a transitioning anticyclonic easterly to cyclonic south–easterly type), 28 → 28 (a persisting cyclonic south–easterly type), and 29 → 29 (a persisting cyclonic south-westerly type coming up against a cold pool of air) a few days ahead of an outage. These tend to represent cold unsettled northerly flows of blocking types with cold air establishing over the UK from continental Europe.
Discussions
The main trends identified in “Analysis of trends in power outages and changes in weather patterns” agree with the distinctive characteristics listed in Supplementary Table 1 (see Supplementary Note 1.1). In winter, weather patterns 20, 23, 26, and 30 are characterized by high wind speeds and above average temperatures, whereas weather patterns 20, 26, and 30 are also characterized by high precipitation volumes. These weather patterns represent the main trends in power outages caused by wind and gale and lightning strikes. Weather patterns 24, 26, 27, 28, and 29 are characterized by moderate to high snowfall, whereas weather patterns 24, 27, and 28 are colder than average and weather patterns 24, 26, 28, and 29 are also characterized by high precipitation volumes. Therefore, these weather patterns are expected to be among the main trends in power outages caused by snow and ice in winter (see also Supplementary Discussion 1.6 for expected effects in spring, summer, and fall).
The strong seasonality of weather patterns can partly explain why some of them are excluded for the key set of impact patterns. Note the similarities between the distinctive characteristics of certain weather patterns in Supplementary Table 1 and the differences in the results obtained in “Analysis of trends in power outages and changes in weather patterns” for distinct weather phenomena in winter (see also Supplementary Discussion 1.6 for expected effects in spring, summer, and fall). These discrepancies can be partly explained by the higher frequency of occurrence of some weather patterns across seasons (e.g., weather patterns 11 and 19, characterized by heavy snowfall, occur less often in winter). It is also important to emphasize that high weather pattern numbers occur more frequently in winter, which partly explains the higher frequency of power system failures in winter. Although high weather pattern numbers are less frequent across seasons, they likely bring failures with them.
Although the majority of events in the NaFIRS datasets did not result in major interruptions (see Supplementary Fig. 1 of Supplementary Note 1.2.1 and Supplementary Note 1.2 for more information), the analysis reveals important relationships between weather-induced power outages and high-risk weather patterns/pattern transitions. Furthermore, validation against past power outages induced by extreme weather events is provided in Supplementary Tables 2 and 3 of Supplementary Discussion 1.7. These insights are extremely valuable for DNOs to improve their preparedness to adverse weather conditions across Europe. Adopting this methodology can provide more comprehensive meteorological information to support reliability and resilience enhancement decisions over different timescales. In a short-term horizon, it can improve preparedness and responsiveness to adverse weather conditions with usage of reliable weather pattern forecast up to several weeks in advance. In a long-term horizon, it can improve power system planning decisions concerning reliability and resilience based on expected changes in the frequency of weather-induced power outages associated with high-risk weather patterns.
Currently, UK DNOs rely on information provided by Met Office weather warnings to watch weather forecasts and track weather fronts associated with severe weather conditions (e.g., high winds, heavy rain and snow). This information is derived from surface pressure charts provided up to 5 days ahead for Europe and the North East Atlantic, updated every 12 h for the next 3 days and once every day for days 4 and 533. Consequently, preemptive escalation under exceptional events has limited temporal resolution and is restricted to very few days before their expected occurrence. This methodology overcomes this limitation by incorporating comprehensive meteorological information derived from high-risk weather patterns with reliable forecasts up to several weeks in advance. By incorporating this methodology, DNOs can mobilise additional resources (e.g., emergency generators and mobile substations) and take advantage of different preventive strategies (e.g., grid reconfiguration and generation re-dispatch) implementable over longer time intervals before an outage occurs.
An important implication of this work is the potential for a development of a pattern-conditioned fault forecasting system. Firstly, because the results presented here highlight that in most cases there are relevant connections between each type of fault and a set of relevant weather patterns, that tend to persist or transition between each others with high probability. Secondly, because the set of weather patterns used here have been shown to have good levels of predictability up to a few weeks ahead23. Moreover, the Met Office already provides an operational forecasting product based on these patterns called Decider (https://www.metoffice.gov.uk/services/business-industry/energy/decider) which would facilitate the design and implementation of the derived forecasting tool. Another potential extension of this work would be to use future projections for the frequencies of occurrences of these patterns (e.g., ref. 29) to identify climate change induced trends in the risks of different fault types.
This work also evinces the great value of NaFIRS data for analysis of power system faults. Although they are not made publicly available by the UK DNOs at present, sharing them could lead to more in-depth assessments with the potential for translation into forecasting tools and early warning systems. Ultimately, findings would benefit general safety, asset protection, and maintenance scheduling in power grids, as well as reliability and resilience enhancement decisions.
Methods
Background
Weather-induced power system failures
In the event of a fault, distribution network operators (DNOs) in the UK are required to report to the National Fault Interruption Reporting Scheme (NaFIRS). For each incident, a vast amount of information must be provided for further analysis, including date, time, duration (total customer minutes lost), number of customer interruptions, maximum demand, direct cause, secondary contributing cause (if applicable), and equipment involved34. At present, however, DNOs are not required to make their NaFIRS data publicly available.
According to ref. 34, there are 11 direct causes of power system failures by weather phenomenon, including adverse weather conditions (high probability, low impact) and exceptional circumstances (low probability, high impact): lightning strikes, rain, snow and ice, ice, freezing fog and frost, wind and gale (excluding wind-borne materials), wind-borne materials, solar heat, flooding, condensation, and corrosion. This classification includes failures of power system components or groups of components which occur as a direct result of the corresponding weather phenomenon. Although power system failures can be attributed to a direct plus secondary contributing cause, the majority of occurrences (over 90% in the evaluated dataset) are attributed to a single (direct) cause.
Weather patterns
Each of the 30 Met Office weather pattern definitions from23 used in this article can be described as one of many atmospheric circulation types over a defined region. These circulation types differ in their characteristics from other weather patterns over the same region and vary on a daily basis. The defined regions of weather patterns can be continental in scale, with the ones used in this article being centred over the UK, but covering much of Europe and the North Atlantic area. These weather patterns were derived through k-means clustering of daily mean sea level pressure (MSLP) observations over an 154 year historical period (1850–2003) in order to produce a climatologically representative set of circulation types which affect the UK and surrounding European area. The weather patterns used are shown in Fig. 6 and are numbered according to their annual average occurrence between 1850 and 2003, from most frequent, 1, to least frequent, 30. The daily historical weather pattern classification was extended from 2003 to near present by objectively assigning daily global reanalysis fields of MSLP from the European Centre for Medium-Range Weather Forecasts’ (ECMWF) global reanalysis version 5 (ERA5,35) to the closest matching weather pattern type. The assignment methodology used is the same as in23 for forecast fields, whereby reanalysis and weather pattern pairings are based on those with the smallest area weighted sum-of-squares differences. Those data have been published by32 up to 2020 with the authors of this study extending it further to cover 2021 and 2022.
Weather pattern monthly frequency occurrences show that the lower numbered patterns are more likely to occur in the summer months and the higher numbered patterns are more likely to occur in the winter months. This seasonality relates to the intensity of each weather pattern’s respective cyclones (areas of low pressure) and anticyclones (areas of high pressure), which tend to be more pronounced in the higher numbered weather patterns. For example, weather pattern 30 represents a very cyclonic (stormy) westerly type over the UK, which would bring with it heavy rainfall and strong winds. Whereas, weather pattern 8 also represents a cyclonic westerly type over the UK, but the pressure gradient and cyclonicity is weaker leading to more “typical” / low impact weather conditions over the UK. In general, each weather pattern can be described according to its cyclonicity (i.e. anticyclonic, unbiased or cyclonic) and its dominant wind direction over the UK. Supplementary Table 1 summarizes each weather pattern’s distinctive characteristics over the UK in terms of its anomalies in wind speed, snowfall, and temperature for each observed season, using a 40-year weather pattern climatology dataset derived from ERA535 between 1981 and 2020. The seasonal breakdown considers that winter lasts from 21 December to 20 March, spring lasts from 21 March to 20 June, summer lasts from 21 June to 22 September, and fall lasts from 23 September to 20 December each year.
Weather patterns and weather-induced power system failures
The large spatial scale of these weather patterns allows them to be related to weather impacts across the whole domain which they cover. In the context of the energy sector, this analysis provides knowledge of the circulation types which lead to impacts on the grid. Past occurrences of power system failures caused by weather phenomena can be related to the large-scale driving atmospheric circulation (weather pattern) at the time and/or to transitions in weather patterns (chained persistence) leading up to power outages. For each power system failure cause attributed to adverse weather conditions, high-risk weather patterns and weather pattern transitions can be identified. The findings can be applied to a forecasting tool, whereby high probability forecasts for a high-risk weather pattern can be used as a signal toward an increased likelihood of faults on the energy network. Daily weather pattern forecasts also highlight the most likely changes in weather patterns (including transitions and persistence), with useful skill out to at least two weeks in advance23. These forecasts are based on the objective assignment of multiple ensemble members (forecast scenarios) to the closest matching weather pattern definition. It is noteworthy that these forecasts are probabilistic in nature to reflect the chaotic nature of the atmosphere and the increasing forecast uncertainties with lead time.
These results (in relation to the identified high-risk weather patterns) can also be applied to climate change projections29,30 as previously discussed in the Introduction. To this extent, any changing frequency in high-risk patterns out to the end of the century can be easily translated into changing risks for the energy sector across Europe. Thereby, information about weather patterns can bring benefits for the assessment of future climate responses, including analysis of changes in frequency and seasonality of high-risk weather patterns.
Frequency of weather-induced power system failures
Let Fxws be the frequency of occurrence of power outages caused by a specific weather phenomenon x during weather pattern w, w ∈ W, over time horizon Ts, representing season s, and fxwt be the number of failures attributed to x during weather pattern w and time interval t, t ∈ Ts. Then, Fxws is given by the sum of fxwt over the time horizon of the analysis, as in Eq. (1):
Let \({\overline{F}}_{s}\) be number of power outages caused by different weather phenomena during time horizon Ts. The contribution of Fxws to \({\overline{F}}_{s}\) is denoted by Rxs in Eq. (2):
Weather pattern transitions and persistence
Previous work by the authors investigated the climatology of weather pattern transitions and persistence (see ref. 27). Findings indicate that the probability of occurrence of a specific weather pattern over time is conditioned by the weather pattern in the previous day and season of the year. Thus, this section defines weather pattern transitions and persistence with the objective of investigating their relationships with specific weather-induced power outages.
Let wk be a unitary vector representing the occurrence of a weather pattern w in time interval k. Then, the probability of occurrence of wk, denoted by \(P\left({{{{{{{{\bf{w}}}}}}}}}_{{{{{{{{\bf{k}}}}}}}}}\right)\), is calculated with (3):
where \(P\left({{{{{{{{\bf{w}}}}}}}}}_{{{{{{{{\bf{k-1}}}}}}}}}\right)\) is a vector representing the probability of occurrence of a weather pattern in time interval k − 1 (i.e., previous day), denoted by wk-1, \(P\left({{{{{{{{\bf{w}}}}}}}}}_{{{{{{{{\bf{k}}}}}}}}}| {{{{{{{{\bf{w}}}}}}}}}_{{{{{{{{\bf{k-1}}}}}}}}}\right)\) is a vector representing the probability of occurrence of wk conditioned by wk-1, and T is the transpose operator. Note that, for past and present times, the elements of \(P\left({{{{{{{{\bf{w}}}}}}}}}_{{{{{{{{\bf{k-1}}}}}}}}}\right)\) are either 0 or 1, depending on the observed weather pattern in the previous day. In turn, for future times when wk-1 is unknown, the elements of \(P\left({{{{{{{{\bf{w}}}}}}}}}_{{{{{{{{\bf{k-1}}}}}}}}}\right)\) are in the interval \(\left[0,1\right]\), representing probabilities of a given weather pattern to occur. It is important to highlight that in this formulation it would be sensible to incorporate a dependence on time (either day of the year or season) if the probabilities of occurrence of patterns have strong seasonality.
Note that Eq. (3) can be generalized as Eq. (4) to calculate the probability of occurrence of wk as a function of the probability of occurrence of a weather pattern in time interval k − N (i.e., N days ahead of day k):
It is noteworthy that Eq. (3) is suitable for the analysis of weather phenomena with short duration (e.g., wind and gale, lightning strikes), whereas Eq. (4) is recommended for the analysis of weather phenomena with prolonged duration (e.g., snow and ice).
Trends in weather-induced power system failures and weather pattern transitions
After calculating Fxws in Eq. (1) and Rxs in Eq. (2), analysis proceeds with the weather phenomena which represent the highest causes of power system failures by season (highest values of Rxs). For each weather phenomenon x and season s, the highest values of Fxws calculated with Eq. (1) are identified and the corresponding vectors containing those weather patterns (i.e., the identified w as elements of wk-1) are obtained. Next, the probabilities of occurrence of those weather patterns are calculated with Eq. (3) and/or Eq. (4).
The strength of a trend relating a weather-induced power system failure x during wk to a specific transitioning or persistent weather pattern is then given by the contribution of wk-1 to Eq. (3) (or by the contributions of wk-1 to wk-N to Eq. (4)) over the time horizon of the analysis:
Extensions to other relevant periods of time
The methodology described in “Frequency of weather-induced power system failures–Trends in weather-induced power system failures and weather pattern transitions”, targeted at weather-induced power outage occurrences during weather pattern w, can also be extended to account for other periods of time. In this case, Eqs. (1)–(6) should be shifted in time to represent the frequency of power outages and probability of weather pattern changes (including transitions and persistence) a few days after weather pattern w (i.e., in time interval k − N, with N ≥ 1).
This extension is particularly relevant for power outages caused by weather phenomena with expected cumulative effects, such as snow and ice, freezing fog and frost, solar heat, and flooding5,36,37,38. For power outages caused by weather phenomena with immediate effects, however, “Frequency of weather-induced power system failures–Trends in weather-induced power system failures and weather pattern transitions” should be adopted, as in the case of lightning strikes, wind and gale, and rain15,19,28.
Data availability
The datasets used in this analysis are partly available on the internet. Weather pattern data up to 2020 are provided by https://doi.org/10.1594/PANGAEA.94289632. Power system failure datasets reported to NaFIRS are proprietary and cannot be made publicly available by the authors. For more information about NaFIRS, including definitions and reporting requirements for power system failures in the UK, refer to https://www.ofgem.gov.uk/sites/default/files/docs/2020/04/riio-ed1_regulatory_instructions_and_guidance_annex_f_-_interruptions.pdf34.
Code availability
The analysis was performed in MATLAB R2021a. Code used in the analysis is provided by https://doi.org/10.5281/zenodo.1047655339 without the datasets.
References
Ofgem, S. Investigation into 9 August 2019 Power Outage https://www.ofgem.gov.uk/publications/investigation-9-august-2019-power-outage (2019).
Bennett, J. A. et al. Extending energy system modelling to include extreme weather risks and application to hurricane events in Puerto Rico. Nat. Energy 6, 240–249 (2021).
Zhang, J., Bagtzoglou, Y., Zhu, J., Li, B. & Zhang, W. Fragility-based system performance assessment of critical power infrastructure. Reliab. Eng. Syst. Safety 232, 109065 (2023).
Yuan, W. et al. Robust optimization-based resilient distribution network planning against natural disasters. IEEE Trans. Smart Grid 7, 2817–2826 (2016).
Souto, L. et al. Power system resilience to floods: modeling, impact assessment, and mid-term mitigation strategies. Int. J. Electr. Power Energy Syst. 135, 107545 (2022).
Larsen, P. H., Lawson, M., LaCommare, K. H. & Eto, J. H. Severe weather, utility spending, and the long-term reliability of the U.S. power system. Energy 198, 117387 (2020).
Ren, H., Hou, Z. J., Ke, X., Huang, Q. & Makatov, Y. Analysis of weather and climate extremes impact on power system outage. In 2021 IEEE Power & Energy Society General Meeting 01–05 (PESGM, 2021).
Grams, C. M., Beerli, R., Pfenninger, S., Staffell, I. & Wernli, H. Balancing Europe’s wind-power output through spatial deployment informed by weather regimes. Nat. Clim. Change 7, 557–562 (2017).
Rodriguez, R. A., Becker, S. & Greiner, M. Cost-optimal design of a simplified, highly renewable pan-European electricity system. Energy 83, 658–668 (2015).
Zeyringer, M., Price, J., Fais, B., Li, P.-H. & Sharp, E. Designing low-carbon power systems for great Britain in 2050 that are robust to the spatiotemporal and inter-annual variability of weather. Nat. Energy 3, 395 –403 (2018).
Krishnamurthy, V. Predictability of weather and climate. Earth Space Sci. 6, 1043–1056 (2019).
Huth, R. et al. Classifications of atmospheric circulation patterns: recent advances and applications. Annal. New York Acad. Sci. 1146, 105–152 (2008).
Philipp, A. et al. Cost733cat–a database of weather and circulation type classifications. Phys. Chem. Earth Parts 35, 360–373 (2010).
Ghil, M., Groth, A., Kondrashov, D. & Robertson, A. W. In Sub-Seasonal to Seasonal Prediction 119–142 (Elsevier, 2019).
Mastrantonas, N., Magnusson, L., Pappenberger, F. & Matschullat, J. What do large-scale patterns teach us about extreme precipitation over the mediterranean at medium-and extended-range forecasts? Quart. J. Royal Meteorol. Soc. 148, 875–890 (2022).
Mariotti, A. et al. Windows of opportunity for skillful forecasts subseasonal to seasonal and beyond. Bullet. Am. Meteorol. Soc. 101, E608–E625 (2020).
Gonzalez, P. L. et al. Weather patterns in southeast Asia: enhancing high-impact weather subseasonal forecast skill. Quart. J. Royal Meteorol. Soc. 149, 19–39 (2023).
Wang, Y., Chen, C., Wang, J. & Baldick, R. Research on resilience of power systems under natural disasters-a review. IEEE Trans. Power Syst. 31, 1604–1613 (2016).
Sang, Y., Xue, J., Sahraei-Ardakani, M. & Ou, G. An integrated preventive operation framework for power systems during hurricanes. IEEE Syst. J. 14, 3245–3255 (2020).
Bonanno, R., Lacavalla, M. & Sperati, S. A new high-resolution meteorological reanalysis Italian dataset: Merida. Quart. J. Royal Meteorol. Soc. 145, 1756–1779 (2019).
Murray, K. & Bell, K. R. W. Wind related faults on the GB transmission network. In 2014 International Conference on Probabilistic Methods Applied to Power Systems 01–06 (PMAPS, 2014).
Morris, E. A., Bell, K. R. W. & Elders, I. M. Spatial and temporal clustering of fault events on the GB transmission network. In 2016 International Conference on Probabilistic Methods Applied to Power Systems 01–09 (PMAPS, 2016).
Neal, R., Fereday, D., Crocker, R. & Comer, R. E. A flexible approach to defining weather patterns and their application in weather forecasting over europe. Meteorol. Appl. 23, 389–400 (2016).
Neal, R. et al. Use of probabilistic medium to long range weather pattern forecasts for identifying periods with an increased likelihood of coastal flooding around the uk. Meteorol. Appl. 25, 534–547 (2018).
Richardson, D. et al. Linking weather patterns to regional extreme precipitation for highlighting potential flood events in medium to long range forecasts. Meteorol. Appl. 27, e1931 (2018).
Wilkinson, J. M. & Neal, R. Exploring relationships between weather patterns and observed lightning activity for Britain and Ireland. Quart. J. Royal Meteorol. Soc.147, 2772–2795 (2021).
Harrison, S. R. et al. Identifying weather patterns associated with increased volcanic ash risk within British isles airspace. Weather Forecast. 37, 1157 – 1168 (2022).
Souto, L., Taylor, P. C. & Wilkinson, J. Probabilistic impact assessment of lightning strikes on power systems incorporating lightning protection design and asset condition. Int. J. Electr. Power Energy Syst. 148, 108974 (2023).
Pope, J. O. et al. Investigation of future climate change over the British isles using weather patterns. Clim. Dyn. 58, 2405–2419 (2022).
Cotterill, D. F., Pope, J. O. & Stott, P. A. Future extension of the UK summer and its impact on autumn precipitation. Clim. Dyn. 60, 1801–1814 (2023).
Perks, R. J., Bernie, D., Lowe, J. & Neal, R. The influence of future weather pattern changes and projected sea-level rise on coastal flood impacts around the UK. Clim. Change 176, 25 (2023).
Neal, R. Daily Historical Weather Pattern Classifications for the UK and Surrounding European Area (1950 to 2020) https://doi.org/10.1594/PANGAEA.942896 (2022).
Office, M. Surface Pressure Charts https://www.metoffice.gov.uk/weather/maps-and-charts/surface-pressure (2023).
Ofgem, S. RIIO-ED1 Regulatory Instructions and Guidance: Annex F—Interruptions https://www.ofgem.gov.uk/sites/default/files/docs/2020/04/riio-ed1_regulatory_instructions_and_guidance_annex_f_-_interruptions.pdf (2020).
Hersbach, H. et al. The ERA5 global reanalysis. Quart. J. Royal Meteorol. Soc. 146, 1999–2049 (2020).
Farzaneh, M. & Chisholm, W. A. Effects of Ice and Snow on the Electrical Performance of Power Network Insulators 269–325 (Springer Dordrecht, 2008).
Hussain, M. M., Farokhi, S., McMeekin, S. G. & Farzaneh, M. Effect of cold fog on leakage current characteristics of polluted insulators. In 2015 International Conference on Condition Assessment Techniques in Electrical Systems 163–167 (CATCON, 2015).
Burillo, D. Effects of climate change in electric power infrastructures. In Power System Stability 2nd edn, Vol. 3 (eds. Kedu, K. E.) Ch. 5 (IntechOpen, 2018).
Souto, L. Generic MATLAB code to obtain trends in power system failures and weather patterns by season in the UK. Zenodo https://doi.org/10.5281/zenodo.10476553 (2024).
Operator, N. ESO Data Portal: GIS Boundaries for GB DNO License Areas—Dataset https://data.nationalgrideso.com/system/gis-boundaries-for-gb-dno-license-areas (2020).
Acknowledgements
This research was supported by the UK EPSRC under the auspices of the “Supergen Energy Networks Hub” project, grant number EP/S00078X/2. We would like to thank the Energy Networks Association and its member organizations for providing their NaFIRS data, as well as Northern Powergrid and Scottish Power Networks for providing their regional NaFIRS data. We would also like to thank Dr. Sam Harrison for the original code to extend the weather pattern record to the present and the reviewers for their valuable comments and suggestions which helped us to improve the quality of our manuscript.
Author information
Authors and Affiliations
Contributions
L.S. conceived and designed the study and performed the analysis. R.N. produced and provided the weather patterns. J.P. produced and provided the weather pattern transition matrices. L.S. and P.C.T. obtained the power system failure data sets. L.S., R.N., J.P., P.L.M.G., J.W., and P.C.T. contributed to discussing the methods and findings and writing and revising the paper.
Corresponding author
Ethics declarations
Competing interests
The authors declare no competing interests.
Peer review
Peer review information
Communications Earth & Environment thanks Jeffrey A. Bennett, Peter H. Larsen and Fabian Mockert for their contribution to the peer review of this work. Primary Handling Editors: Martina Grecequet. A peer review file is available.
Additional information
Publisher’s note Springer Nature remains neutral with regard to jurisdictional claims in published maps and institutional affiliations.
Supplementary information
Rights and permissions
Open Access This article is licensed under a Creative Commons Attribution 4.0 International License, which permits use, sharing, adaptation, distribution and reproduction in any medium or format, as long as you give appropriate credit to the original author(s) and the source, provide a link to the Creative Commons license, and indicate if changes were made. The images or other third party material in this article are included in the article’s Creative Commons license, unless indicated otherwise in a credit line to the material. If material is not included in the article’s Creative Commons license and your intended use is not permitted by statutory regulation or exceeds the permitted use, you will need to obtain permission directly from the copyright holder. To view a copy of this license, visit http://creativecommons.org/licenses/by/4.0/.
About this article
Cite this article
Souto, L., Neal, R., Pope, J.O. et al. Identification of weather patterns and transitions likely to cause power outages in the United Kingdom. Commun Earth Environ 5, 49 (2024). https://doi.org/10.1038/s43247-024-01217-w
Received:
Accepted:
Published:
DOI: https://doi.org/10.1038/s43247-024-01217-w
Comments
By submitting a comment you agree to abide by our Terms and Community Guidelines. If you find something abusive or that does not comply with our terms or guidelines please flag it as inappropriate.