Abstract
Machine learning interatomic potentials (MLIPs) describe the interactions between atoms in materials and molecules by learning them from a reference database generated by ab initio calculations. MLIPs can accurately and efficiently predict such interactions and have been applied to various fields of physical science. However, high-performance MLIPs rely on a large amount of labelled data, which are costly to obtain by ab initio calculations. Here we propose a geometric structure learning framework that leverages unlabelled configurations to improve the performance of MLIPs. Our framework consists of two stages: first, using classical molecular dynamics simulations to generate unlabelled configurations of the target molecular system; and second, applying geometry-enhanced self-supervised learning techniques, including masking, denoising and contrastive learning, to capture structural information. We evaluate our framework on various benchmarks ranging from small molecule datasets to complex periodic molecular systems with more types of elements. We show that our method significantly improves the accuracy and generalization of MLIPs with only a few additional computational costs and is compatible with different invariant or equivariant graph neural network architectures. Our method enhances MLIPs and advances the simulations of molecular systems.
This is a preview of subscription content, access via your institution
Access options
Access Nature and 54 other Nature Portfolio journals
Get Nature+, our best-value online-access subscription
$29.99 / 30 days
cancel any time
Subscribe to this journal
Receive 12 digital issues and online access to articles
$119.00 per year
only $9.92 per issue
Buy this article
- Purchase on Springer Link
- Instant access to full article PDF
Prices may be subject to local taxes which are calculated during checkout



Similar content being viewed by others
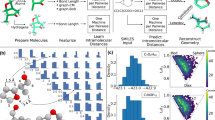
Data availability
The data used for pretraining and downstream tasks are available in the figshare database: https://doi.org/10.6084/m9.figshare.25314649 (ref. 48).
Code availability
The source code of the GPIP framework is available at GitHub: https://github.com/cuitaoyong/GPIP (ref. 49).
References
Hospital, A., Goñi, J. R., Orozco, M. & Gelpí, J. L. Molecular dynamics simulations: advances and applications. Adv. Appl. Bioinform. Chem. 19, 37–47 (2015).
Senftle, T. P. et al. The ReaxFF reactive force-field: development, applications and future directions. npj Comput. Mater. 2, 1–14 (2016).
Karplus, M. & Petsko, G. A. Molecular dynamics simulations in biology. Nature 347, 631–639 (1990).
Yao, N., Chen, X., Fu, Z.-H. & Zhang, Q. Applying classical, ab initio, and machine-learning molecular dynamics simulations to the liquid electrolyte for rechargeable batteries. Chem. Rev. 122, 10970–11021 (2022).
Kaminski, G. A., Friesner, R. A., Tirado-Rives, J. & Jorgensen, W. L. Evaluation and reparametrization of the OPLS-AA force field for proteins via comparison with accurate quantum chemical calculations on peptides. J. Phys. Chem. B 105, 6474–6487 (2001).
Car, R. & Parrinello, M. Unified approach for molecular dynamics and density-functional theory. Phys. Rev. Lett. 55, 2471 (1985).
Butler, K. T., Davies, D. W., Cartwright, H., Isayev, O. & Walsh, A. Machine learning for molecular and materials science. Nature 559, 547–555 (2018).
Noé, F., Tkatchenko, A., Müller, K.-R. & Clementi, C. Machine learning for molecular simulation. Ann. Rev. Phys. Chem. 71, 361–390 (2020).
Unke, O. T. et al. Machine learning force fields. Chem. Rev. 121, 10142–10186 (2021).
Gilmer, J., Schoenholz, S. S., Riley, P. F., Vinyals, O. & Dahl, G. E. Neural message passing for quantum chemistry. In Proc. 34th International Conference on Machine Learning (eds Precup, D. & Teh, Y.W.) 1263–1272 (PMLR, 2017).
Schütt, K. et al. SchNet: a continuous-filter convolutional neural network for modeling quantum interactions. In Proc. 31st International Conference on Neural Information Processing Systems (eds von Luxburg, U. & Guyon, I.) 992–1002 (Curran, 2017).
Gasteiger, J., Groß, J. & Günnemann, S. Directional message passing for molecular graphs. Paper presented at the ICLR 2020 The Eighth International Conference on Learning Representations (2020); https://openreview.net/pdf?id=B1eWbxStPH
Liu, Y. et al. Spherical message passing for 3D molecular graphs. Paper presented at the ICLR 2022 The Tenth International Conference on Learning Representations (2022); https://openreview.net/pdf?id=givsRXsOt9r
Thomas, N. et al. Tensor field networks: Rotation-and translation-equivariant neural networks for 3d point clouds. Preprint at https://doi.org/10.48550/arXiv.1802.08219 (2018).
Batzner, S. et al. E(3)-equivariant graph neural networks for data-efficient and accurate interatomic potentials. Nat. Commun. 13, 2453 (2022).
Satorras, V. G., Hoogeboom, E. & Welling, M. E(n) equivariant graph neural networks. In Proc. of the 38th International Conference on Machine Learning (eds Meila, M. & Zhang, T.) 9323–9332 (PMLR, 2021).
Schütt, K., Unke, O. & Gastegger, M. Equivariant message passing for the prediction of tensorial properties and molecular spectra. In Proc. of the 38th International Conference on Machine Learning (eds Meila, M. & Zhang, T.) 9377–9388 (PMLR, 2021).
Gasteiger, J., Becker, F. & Günnemann, S. GemNet: universal directional graph neural networks for molecules. In Advances in Neural Information Processing Systems 34 (eds Ranzato, M. et al.) 6790–6802 (2021).
Veličković, P. et al. Deep Graph Infomax. Paper presented at ICLR 2019 The Seventh International Conference on Learning Representations (2019); https://openreview.net/forum?id=rklz9iAcKQ
Hassani, K. & Khasahmadi, A. H. Contrastive multi-view representation learning on graphs. In Proc. of the 37th International Conference on Machine Learning (eds Daumé III, H. & Singh, A.) 4116–4126 (PMLR, 2020).
Qiu, J. et al. GCC: Graph contrastive coding for graph neural network pre-training. In KDD '20: Proceedings of the 26th ACM SIGKDD International Conference on Knowledge Discovery & Data Mining 1150–1160 (ACM, 2020).
Hu, W. et al. Strategies for pre-training graph neural networks. Paper presented at ICLR 2020 The Eighth International Conference on Learning Representations (2020); https://openreview.net/forum?id=HJlWWJSFDH
Wang, Y., Wang, J., Cao, Z. & Barati Farimani, A. Molecular contrastive learning of representations via graph neural networks. Nat. Mach. Intell. 4, 279–287 (2022).
Zhou, G. et al. Uni-mol: a universal 3D molecular representation learning framework. Paper presented at ICLR 2023 The Eleventh International Conference on Learning Representations (2023); https://openreview.net/forum?id=6K2RM6wVqKu
Zhang, D. et al. Dpa-1: Pretraining of attention-based deep potential model for molecular simulation. Preprint at https://doi.org/10.48550/arXiv.2208.08236 (2022).
Wang, Y., Xu, C., Li, Z. & Farimani, A. B. Denoise pre-training on non-equilibrium molecules for accurate and transferable neural potentials. J Chem. Theory Comput. 19, 5077–5087 (2023).
Chanussot, L. et al. Open catalyst 2020 (OC20) dataset and community challenges. ACS Catal. 11, 6059–6072 (2021).
Smith, J. S., Isayev, O. & Roitberg, A. E. ANI-1: an extensible neural network potential with DFT accuracy at force field computational cost. Chem. Sci. 8, 3192–3203 (2017).
Gardner, J. L., Baker, K. T. & Deringer, V. L. Synthetic pre-training for neural-network interatomic potentials. Mach. Learn. Sci. Technol. 5, 015003 (2024).
Stärk, H. et al. 3D Infomax improves GNNs for molecular property prediction. In Proc. of the 39th International Conference on Machine Learning (eds Kamalika, C. et al.) 20479–20502 (PMLR, 2022).
Rappé, A. K., Casewit, C. J., Colwell, K., Goddard III, W. A. & Skiff, W. M. UFF, a full periodic table force field for molecular mechanics and molecular dynamics simulations. J. Am. Chem. Soc. 114, 10024–10035 (1992).
He, K. et al. Masked autoencoders are scalable vision learners. In 2022 IEEE/CVF Conference on Computer Vision and Pattern Recognition (CVPR) ( ) 15979–15988 (2022).
Hou, Z. et al. GraphMAE: Self-supervised masked graph autoencoders. In KDD '22: Proc. of the 28th ACM SIGKDD International Conference on Knowledge Discovery & Data Mining (ed. Zhang, A.) 594–604 (ACM, 2022).
Vincent, P., Larochelle, H., Bengio, Y. & Manzagol, P.-A. Extracting and composing robust features with denoising autoencoders. In ICML '08: Proc. of the 25th International Conference on Machine Learning 1096–1103 (ACM, 2008).
Chmiela, S. et al. Machine learning of accurate energy-conserving molecular force fields. Sci. Adv. 3, e1603015 (2017).
Ramakrishnan, R., Dral, P. O., Rupp, M. & Von Lilienfeld, O. A. Quantum chemistry structures and properties of 134 kilo molecules. Sci. Data 1, 1–7 (2014).
Fu, X. et al. Forces are not enough: benchmark and critical evaluation for machine learning force fields with molecular simulations. Preprint at https://arxiv.org/abs/2210.07237 (2023).
Zhang, L., Wang, H., Car, R. & E, W. Phase diagram of a deep potential water model. Phys. Rev. Lett. 126, 236001 (2021).
Staacke, C. G. et al. On the role of long-range electrostatics in machine-learned interatomic potentials for complex battery materials. ACS Appl. Energy Mater. 4, 12562–12569 (2021).
Mondal, A., Kussainova, D., Yue, S. & Panagiotopoulos, A. Z. Modeling chemical reactions in alkali carbonate–hydroxide electrolytes with deep learning potentials. J. Chem. Theory Comput. 19, 4584–4595 (2023).
Anstine, D. M. & Isayev, O. Machine learning interatomic potentials and long-range physics. J. Phys. Chem. A 127, 2417–2431 (2023).
McInnes, L., Healy, J., Saul, N. & Großberger, L. UMAP: Uniform Manifold Approximation and Projection. J. Open Source Softw. 3, 861 (2018).
Thompson, A. P. et al. LAMMPS - a flexible simulation tool for particle-based materials modeling at the atomic, meso, and continuum scales. Comput. Phys. Commun. 271, 108171 (2022).
Jorgensen, W. L., Chandrasekhar, J., Madura, J. D., Impey, R. W. & Klein, M. L. Comparison of simple potential functions for simulating liquid water. J. Chem. Phys. 79, 926–935 (1983).
Perdew, J. P., Burke, K. & Ernzerhof, M. Generalized gradient approximation made simple. Phys. Rev. Lett. 77, 3865 (1996).
Blöchl, P. E. Projector augmented-wave method. Phys. Rev. B 50, 17953 (1994).
Loshchilov, I. & Hutter, F. Decoupled weight decay regularization. Paper presented at ICLR 2017 The Fifth International Conference on Learning Representations (2017); https://openreview.net/pdf?id=Bkg6RiCqY7
Cui, T. et al. GPIP dataset. figsharehttps://doi.org/10.6084/m9.figshare.25314649 (2024).
Cui, T. et al. cuitaoyong/GPIP: v1.0.0. Zenodo https://doi.org/10.5281/zenodo.10693481 (2024).
Acknowledgements
This work was supported by the National Key R&D Programme of China (Grant No. 2022ZD0160101). M.S. was partially supported by Shanghai Committee of Science and Technology, China (Grant No. 23QD1400900). T.C. and C.T. did this work during their internship at Shanghai Artificial Intelligence Laboratory.
Author information
Authors and Affiliations
Contributions
M.S. and S.Z. conceived the idea and led the research. T.C. developed the codes and trained the models. C.T. generated datasets and performed experiments and analyses. Y.L. and X.G. contributed technical ideas for datasets and experiments. L.B., Y.D. and W.O. contributed technical ideas for self-supervised methods. T.C., C.T., M.S. and S.Z. wrote the paper. All authors discussed the results and reviewed the manuscript.
Corresponding authors
Ethics declarations
Competing interests
The authors declare no competing interests.
Peer review
Peer review information
Nature Machine Intelligence thanks Liang Hong and the other, anonymous, reviewer(s) for their contribution to the peer review of this work.
Additional information
Publisher’s note Springer Nature remains neutral with regard to jurisdictional claims in published maps and institutional affiliations.
Supplementary information
Supplementary Information
Supplementary Figs. 1–4, Tables 1–8 and refs. 1–4.
Source Data Fig. 2
Statistical source data.
Source Data Fig. 3
Statistical source data.
Rights and permissions
Springer Nature or its licensor (e.g. a society or other partner) holds exclusive rights to this article under a publishing agreement with the author(s) or other rightsholder(s); author self-archiving of the accepted manuscript version of this article is solely governed by the terms of such publishing agreement and applicable law.
About this article
Cite this article
Cui, T., Tang, C., Su, M. et al. Geometry-enhanced pretraining on interatomic potentials. Nat Mach Intell 6, 428–436 (2024). https://doi.org/10.1038/s42256-024-00818-6
Received:
Accepted:
Published:
Issue Date:
DOI: https://doi.org/10.1038/s42256-024-00818-6