Abstract
The in vitro differentiation of insulin-producing beta-like cells can model aspects of human pancreatic development. Here, we generate 95,308 single-cell transcriptomes and reconstruct a lineage tree of the entire differentiation process from human embryonic stem cells to beta-like cells to study temporally regulated genes during differentiation. We identify so-called ‘switch genes’ at the branch point of endocrine/non-endocrine cell fate choice, revealing insights into the mechanisms of differentiation-promoting reagents, such as NOTCH and ROCKII inhibitors, and providing improved differentiation protocols. Over 20% of all detectable genes are activated multiple times during differentiation, even though their enhancer activation is usually unimodal, indicating extensive gene reuse driven by different enhancers. We also identify a stage-specific enhancer at the TCF7L2 locus for diabetes, uncovered by genome-wide association studies, that drives a transient wave of gene expression in pancreatic progenitors. Finally, we develop a web app to visualize gene expression on the lineage tree, providing a comprehensive single-cell data resource for researchers studying islet biology and diabetes.
This is a preview of subscription content, access via your institution
Access options
Access Nature and 54 other Nature Portfolio journals
Get Nature+, our best-value online-access subscription
$29.99 / 30 days
cancel any time
Subscribe to this journal
Receive 12 digital issues and online access to articles
$119.00 per year
only $9.92 per issue
Buy this article
- Purchase on Springer Link
- Instant access to full article PDF
Prices may be subject to local taxes which are calculated during checkout







Similar content being viewed by others
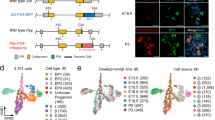
Data availability
Raw and processed Drop-seq data are accessible at the Gene Expression Omnibus under accession GSE143783.The web app to visualize gene expression on the lineage tree is available at http://hiview.case.edu/public/BetaCellHub/differentiation.php.
GWAS data were downloaded from the GWAS catalogue66 (https://www.ebi.ac.uk/gwas/). The list of TFs was obtained from the TF prediction database67 (http://www.transcriptionfactor.org/). The list of cell surface proteins was downloaded from a previous study57. All lists are available in Supplementary Data 3.
All other data that support the findings of this study are available from the corresponding author on reasonable request. Source data are provided with this paper.
Code availability
All code used in this study is available from the GitHub repository: https://github.com/JinLabBioinfo/LineageTreeBuilder/.
References
D’Amour, K. A. et al. Production of pancreatic hormone-expressing endocrine cells from human embryonic stem cells. Nat. Biotechnol. 24, 1392–1401 (2006).
Kroon, E. et al. Pancreatic endoderm derived from human embryonic stem cells generates glucose-responsive insulin-secreting cells in vivo. Nat. Biotechnol. 26, 443–452 (2008).
Kelly, O. G. et al. Cell-surface markers for the isolation of pancreatic cell types derived from human embryonic stem cells. Nat. Biotechnol. 29, 750–756 (2011).
Pagliuca, F. W. et al. Generation of functional human pancreatic beta cells in vitro. Cell 159, 428–439 (2014).
Rezania, A. et al. Reversal of diabetes with insulin-producing cells derived in vitro from human pluripotent stem cells. Nat. Biotechnol. 32, 1121–1133 (2014).
Osafune, K. et al. Marked differences in differentiation propensity among human embryonic stem cell lines. Nat. Biotechnol. 26, 313–315 (2008).
Bruin, J. E. et al. Characterization of polyhormonal insulin-producing cells derived in vitro from human embryonic stem cells. Stem Cell Res. 12, 194–208 (2014).
Nostro, M. C. et al. Stage-specific signaling through TGF-β family members and WNT regulates patterning and pancreatic specification of human pluripotent stem cells. Development 138, 861–871 (2011).
Sharon, N. et al. Wnt signaling separates the progenitor and endocrine compartments during pancreas development. Cell Rep. 27, 2281–2291 (2019).
Veres, A. et al. Charting cellular identity during human in vitro beta-cell differentiation. Nature 569, 368–373 (2019).
Macosko, E. Z. et al. Highly parallel genome-wide expression profiling of individual cells using nanoliter droplets. Cell 161, 1202–1214 (2015).
Xue, R. et al. A rising star in pancreatic diseases: pancreatic stellate cells. Front. Physiol. 9, 754 (2018).
Fang, Z. et al. Single-cell heterogeneity analysis and CRISPR screen identify key beta-cell-specific disease genes. Cell Rep. 26, 3132–3144 (2019).
Stuart, T. et al. Comprehensive integration of single-cell data. Cell 177, 1888–1902 (2019).
Hrvatin, S. et al. Differentiated human stem cells resemble fetal, not adult, beta cells. Proc. Natl Acad. Sci. USA 111, 3038–3043 (2014).
Rezania, A. et al. Maturation of human embryonic stem cell-derived pancreatic progenitors into functional islets capable of treating pre-existing diabetes in mice. Diabetes 61, 2016–2029 (2012).
Riedel, M. J. et al. Immunohistochemical characterisation of cells co-producing insulin and glucagon in the developing human pancreas. Diabetologia 55, 372–381 (2012).
Hu He, K. H. et al. In vivo conditional Pax4 overexpression in mature islet beta cells prevents stress-induced hyperglycemia in mice. Diabetes 60, 1705–1715 (2011).
Saelens, W., Cannoodt, R., Todorov, H. & Saeys, Y. A comparison of single-cell trajectory inference methods. Nat. Biotechnol. 37, 547–554 (2019).
Cao, J. et al. The single-cell transcriptional landscape of mammalian organogenesis. Nature 566, 496–502 (2019).
Georgia, S., Soliz, R., Li, M., Zhang, P. & Bhushan, A. p57 and Hes1 coordinate cell cycle exit with self-renewal of pancreatic progenitors. Dev. Biol. 298, 22–31 (2006).
Baron, M. et al. A single-cell transcriptomic map of the human and mouse pancreas reveals inter- and intra-cell population structure. Cell Syst. 3, 346–360 e344 (2016).
Segerstolpe, A. et al. Single-cell transcriptome profiling of human pancreatic islets in health and type 2 diabetes. Cell Metab. 24, 593–607 (2016).
Lawlor, N. et al. Single-cell transcriptomes identify human islet cell signatures and reveal cell-type-specific expression changes in type 2 diabetes. Genome Res. 27, 208–222 (2017).
Chiou, J. et al. Single-cell chromatin accessibility reveals pancreatic islet cell-type- and state-specific regulatory programs of diabetes risk. Preprint at bioRxiv https://doi.org/10.1101/693671 (2019).
Chen, Q. & Hu, G. Post-transcriptional regulation of the pluripotent state. Curr. Opin. Genet. Dev. 46, 15–23 (2017).
Wang, A. et al. Epigenetic priming of enhancers predicts developmental competence of hESC-derived endodermal lineage intermediates. Cell Stem Cell 16, 386–399 (2015).
Alvarez-Dominguez, J. R. et al. Circadian entrainment triggers maturation of human in vitro islets. Cell Stem Cell 26, 108–122 (2020).
Mahajan, A. et al. Fine-mapping type 2 diabetes loci to single-variant resolution using high-density imputation and islet-specific epigenome maps. Nat. Genet. 50, 1505–1513 (2018).
Segre, A. V. et al. Common inherited variation in mitochondrial genes is not enriched for associations with type 2 diabetes or related glycemic traits. PLoS Genet. 6, e1001058 (2010).
Perez-Alcantara, M. et al. Patterns of differential gene expression in a cellular model of human islet development, and relationship to type 2 diabetes predisposition. Diabetologia 61, 1614–1622 (2018).
Plaisier, C. L. et al. Causal mechanistic regulatory network for glioblastoma deciphered using systems genetics network analysis. Cell Syst. 3, 172–186 (2016).
van Gurp, L. et al. A transcriptomic roadmap to alpha- and beta-cell differentiation in the embryonic pancreas. Development 146, dev173716 (2019).
Krentz, N. A. J. et al. Single-cell transcriptome profiling of mouse and hESC-derived pancreatic progenitors. Stem Cell Rep. 11, 1551–1564 (2018).
Byrnes, L. E. et al. Lineage dynamics of murine pancreatic development at single-cell resolution. Nat. Commun. 9, 3922 (2018).
Scavuzzo, M. A. et al. Endocrine lineage biases arise in temporally distinct endocrine progenitors during pancreatic morphogenesis. Nat. Commun. 9, 3356 (2018).
Velazco-Cruz, L. et al. Acquisition of dynamic function in human stem cell-derived beta cells. Stem Cell Rep. 12, 351–365 (2019).
Murtaugh, L. C., Stanger, B. Z., Kwan, K. M. & Melton, D. A. Notch signaling controls multiple steps of pancreatic differentiation. Proc. Natl Acad. Sci. USA 100, 14920–14925 (2003).
Apelqvist, A. et al. Notch signalling controls pancreatic cell differentiation. Nature 400, 877–881 (1999).
Jensen, J. et al. Control of endodermal endocrine development by Hes-1. Nat. Genet. 24, 36–44 (2000).
Ghazizadeh, Z. et al. ROCKII inhibition promotes the maturation of human pancreatic beta-like cells. Nat. Commun. 8, 298 (2017).
Hogrebe, N. J., Augsornworawat, P., Maxwell, K. G., Velazco-Cruz, L. & Millman, J. R. Targeting the cytoskeleton to direct pancreatic differentiation of human pluripotent stem cells. Nat. Biotechnol. 38, 460–470 (2020).
Tsuchiya, M. et al. Cholesterol biosynthesis pathway intermediates and inhibitors regulate glucose-stimulated insulin secretion and secretory granule formation in pancreatic beta cells. Endocrinology 151, 4705–4716 (2010).
Xia, F. et al. Inhibition of cholesterol biosynthesis impairs insulin secretion and voltage-gated calcium channel function in pancreatic beta cells. Endocrinology 149, 5136–5145 (2008).
Bailey, K. A. et al. Evidence of non-pancreatic beta cell-dependent roles of Tcf7l2 in the regulation of glucose metabolism in mice. Hum. Mol. Genet 24, 1646–1654 (2015).
Jin, T. Current understanding on role of the Wnt signaling pathway effector TCF7L2 in glucose homeostasis. Endocr. Rev. 37, 254–277 (2016).
Sokol, S. Y. Maintaining embryonic stem cell pluripotency with Wnt signaling. Development 138, 4341–4350 (2011).
Lyssenko, V. et al. Mechanisms by which common variants in the TCF7L2 gene increase risk of type 2 diabetes. J. Clin. Invest. 117, 2155–2163 (2007).
Viñuela, A. et al. Genetic variant effects on gene expression in human pancreatic islets and their implications for T2D. Nat. Commun. 11, 4912 (2020).
Ikeda, H. et al. Involvement of Rho/Rho kinase pathway in regulation of apoptosis in rat hepatic stellate cells. Am. J. Physiol. Gastrointest. Liver Physiol. 285, G880–G886 (2003).
Genuth, N. R. & Barna, M. The discovery of ribosome heterogeneity and its implications for gene regulation and organismal life. Mol. Cell 71, 364–374 (2018).
Gerst, J. E. Pimp My Ribosome: ribosomal protein paralogs specify translational control. Trends Genet. 34, 832–845 (2018).
Fulco, C. P. et al. Activity-by-contact model of enhancer–promoter regulation from thousands of CRISPR perturbations. Nat. Genet. 51, 1664–1669 (2019).
Lu, L. et al. Robust Hi-C maps of enhancer–promoter interactions reveal the function of non-coding genome in neural development and diseases. Mol. Cell 79, 521–534 (2020).
Gu, B. et al. Transcription-coupled changes in nuclear mobility of mammalian cis-regulatory elements. Science 359, 1050–1055 (2018).
Labun, K. et al. CHOPCHOP v3: expanding the CRISPR web toolbox beyond genome editing. Nucleic Acids Res. 47, W171–W174 (2019).
da Cunha, J. P. C. et al. Bioinformatics construction of the human cell surfaceome. Proc. Natl Acad. Sci. USA 106, 16752–16757 (2009).
Lun, A. T., Bach, K. & Marioni, J. C. Pooling across cells to normalize single-cell RNA-sequencing data with many zero counts. Genome Biol. 17, 75 (2016).
Subramanian, A. et al. Gene-set enrichment analysis: a knowledge-based approach for interpreting genome-wide expression profiles. Proc. Natl Acad. Sci. USA 102, 15545–15550 (2005).
Wolf, F. A. et al. PAGA: graph abstraction reconciles clustering with trajectory inference through a topology preserving map of single cells. Genome Biol. 20, 59 (2019).
Charrad, M., Ghazzali, N., Boiteau, V. & Niknafs, A. NbClust: an R Package for determining the relevant number of clusters in a data set. J. Stat. Softw. 61, 1–36 (2014).
Buniello, A. et al. The NHGRI-EBI GWAS catalog of published genome-wide association studies, targeted arrays and summary statistics 2019. Nucleic Acids Res. 47, D1005–D1012 (2019).
Kulakovskiy, I. V. et al. HOCOMOCO: towards a complete collection of transcription factor-binding models for human and mouse via large-scale ChIP–seq analysis. Nucleic Acids Res. 46, D252–D259 (2018).
Zhang, Y. et al. Model-based analysis of ChIP–seq (MACS). Genome Biol. 9, R137 (2008).
Bailey, T. L. et al. MEME SUITE: tools for motif discovery and searching. Nucleic Acids Res. 37, W202–W208 (2009).
MacArthur, J. et al. The new NHGRI-EBI catalog of published genome-wide association studies (GWAS Catalog). Nucleic Acids Res. 45, D896–D901 (2017).
Wilson, D., Charoensawan, V., Kummerfeld, S. K. & Teichmann, S. A. DBD—taxonomically broad transcription factor predictions: new content and functionality. Nucleic Acids Res. 36, D88–D92 (2008).
Acknowledgements
This work was supported by grants from the National Institutes of Health (NIH; R01DK113185 to Y.L. and R01HG009658 to F.J.) and Mt. Sinai Health Care Foundation (OSA510114 to Y.L. and OSA510113 to F.J.), and a pilot award from the Clinical and Translational Science Collaborative at Case Western Reserve University (VSN639001 to Y.L.). A.G. is supported by a NIH MSTP training grant (T32 GM007250) and a Functional Genomics Training Program grant (T32 GM135081). We also gratefully acknowledge the insightful discussions with M. Scavuzzo, X. Liu, S. Zhang, L. Lu and L. Wang.
Author information
Authors and Affiliations
Contributions
Y.L. and F.J. conceived the project. C.W., J.X. and H.L. performed the experiments. J.C., A.G., S.L., K.L. and L.K. contributed to the experiments. C.W. and J.X. carried out the data analysis. C.W., X.J., F.J. and Y.L. wrote the manuscript.
Corresponding authors
Ethics declarations
Competing interests
The authors declare no competing interests.
Additional information
Peer review information Primary Handling Editors: Elena Bellafante; Christoph Schmitt.
Publisher’s note Springer Nature remains neutral with regard to jurisdictional claims in published maps and institutional affiliations.
Extended data
Extended Data Fig. 1 Schematics of experimental design and benchmark of differentiated cell maturity.
a, On the top is the timeline of the directed beta-cell differentiation process that takes 31 days encompassing 7 stages. Each stage recapitulates an in vivo developmental stage respectively. The key chemicals added at different time points are listed. Cells from each of the 12 time points are collected for Drop-seq experiments. For instance, S1_D1 stands for stage 1 day 1. b, Glucose stimulated insulin secretion (GSIS) assay for differentiated Stage7 cells. Three rounds of glucose challenges were performed before final treatment with KCl (detail in Methods). n=3 biologically independent replicates. Error bars show +/- 1 standard error of mean (SEM). One-sided Student’s t-test was performed. *** indicates p-value<0.01. The exact p-values (multiple test unadjusted) for 1st, 2nd and 3rd glucose challenge are 1.4*10-4; 2.8*10-3 and 3.2*10-4 respectively. c, CCA-based co-embedding analysis between the differentiated cells and the primary islet dataset. d, Cell type specific marker genes visualized on co-embedded UMAP. Color intensity indicates relative expression level (Z score). e, Bubble plot of endocrine and non-endocrine signature genes in different populations (Extended for Fig. 1f). Color intensity indicates the Z score of averaged expression levels; bubble size indicates the percentage of expressing cells.
Extended Data Fig. 2 Unsupervised clustering of sub-populations from Stage 4 to Stage 7.
a, Two step clustering for S4, S5, S6, S7 respectively. For each stage, a low-resolution clustering for all cells is shown on the left, where two big populations, endocrine (green) and non-endocrine (grey), are clearly separated. On the right are the secondary high-resolution sub-clusters for non-endocrine (right top of each stage) and endocrine population (right bottom of each stage) respectively. Below the tSNEs is the endocrine/non-endocrine population summary for each stage (b) Endocrine marker genes examination (NEUROD1, CPE and CHGA) on first low-resolution tSNE map for each stage. Color intensity indicates relative expression level (z score). c, Summary of the sub-populations of stage 7. d-g, Marker gene examinations on stage 7 endocrine tSNE plot. Color intensity indicates relative expression level (z score) with INS for beta-like population (d); GCG for alpha-like population (e); EC-like population markers (f); and proliferation markers (g).
Extended Data Fig. 3 Comparison of trajectory inference algorithms.
a-b, Global lineage inference connecting cells from all 12 time points in the same UMAP embedding, using the method in this manuscript in (a) and using Monocle3 in (b). Note that starting from H1/S0, Monocle3 created a branch directly to Stage 2 Day1 (S2_D1), bypassing the time points in Stage 1. c, Differentially expressed genes among H1(ES), S1_D2 and S2_D1. Color intensity indicates the expression level. TPM: transcripts per million UMI. d-g, Our method and Monocle3 inferred the consistent local differentiation trajectories. Local trajectory inference of S4 and S5 starting from Branch point 1 (BP1), using our method is shown in (d) and using Monocle3 in (e). Local trajectory inference of alpha-like and beta-like lineages starting from branch point 2 (BP2), using our method is shown in (f) and using Monocle3 in (g). h, Side-by-side comparisons of switch genes at BP1 in Fig. 3b between on our trajectories (Left panels) and on Monocle3 trajectories (Right panels). i, Similar to (g), side-by-side comparison between our method and Monocle3 for BP2 switch genes reported in Extended Data Fig. 8b).
Extended Data Fig. 4 Lineage specific gene modules on full developmental tree.
a, 64 gene modules extended for Fig. 2a. Each gene module contains a couple of hundreds of genes that share a similar expression pattern of time & lineage specificity on the full developmental tree. Three representative genes in each module are shown. For full list see Supplementary Data 3. ME: meso-endoderm; DE: definitive endoderm; FG: foregut. PL: PSC-like; DL: Duct-like; EC: enterochromaffin cells; βL: beta-like; αL: α-like. b, Expression of islet cell type specific marker genes are illustrated on the full tree. The color intensity indicates the relative expression level. INS: beta cell marker; GCG: alpha cell marker; SST: delta cell marker; PPY: gamma cell marker and GHRL: epsilon cell marker (c) Expression of representative cell cycle genes are illustrated on the full tree. Color intensity indicates the relative expression level.
Extended Data Fig. 5 Examples of time dependent dynamic genes on β-like specific lineage.
a, Characterization of genes along β-like specific lineage highlighted in Fig. 1b. Left pie-chart: Summary of genes in each category. Out of all the genes, 11876 genes with detectable expression level. 9117 genes show time dependent expression pattern. Right histogram: histogram showing the number of genes with different variance across time. Dashed line indicates 2-fold relative expression change along trajectory. b-f, Show the examples of time dependent dynamic genes from ES cell to beta cell lineage. b, shows the cell cycle genes. c, shows the developmental genes (d) shows classic beta cell signature genes. e, f, shows calcium channel and sodium channel genes respectively. The color intensity indicates the relative expression level (z score).
Extended Data Fig. 6 Time dependent TF motif analysis on β-like specific lineage.
a, Summary of the motif analysis flow. 769 transcription factors show time dependent expression pattern. 195 TFs out of those have reported DNA binding motif matrix that can be used for motif enrichment analysis. 85 out of 195 shows motif enrichment at least in one of the 7 stage H3K27ac peaks genome wide. For these 85 TFs, the single cell expression level was aggregated into each stage as a pseudo-bulk RNA expression data; TF motif density among enhancers from each stage of H3K27ac can be then correlated to the TF expression. We defined positive correlation when r >0.3 (Pearson’s correlation coefficient), and defined negative correlation when r < -0.3. 48 TFs show positive correlation between expression pattern and motif density pattern. 24 TFs show negative correlation. 13 TFs with no significant correlation. b, The details of all correlated 72 TFs. For each TF, Top panel shows the relative expression change along the pseudo-time computed from single-cell RNA data. Bottom shows the TF motif enrichment on genome-wide H3K27ac peaks across 7 stages. Stage labels are shown in the middle, shared by top and bottom panels. Green: positive correlation; Red: Negative correlation.
Extended Data Fig. 7 Time dependent dynamic GWAS genes on β-like specific lineage.
a, Heatmap showing top time dependent dynamic T2D/glycemic GWAS genes from ES cell to beta cell lineage highlighted in Fig. 1b. The color intensity indicates the relative expression level. The left color bar indicates the traits associated with a certain gene. Gene loci associated with both T2D and glycemic traits are highlighted in bold with asterisk. On the right: bubble plot showing the expression level in human primary beta cells. The size of the bubble indicates the percentage of cells with detectable expression. Bubble color indicates the averaged expression level. Full list of dynamic T2D/glycemic GWAS genes in Supplementary Data 3. b, MAGENTA enrichment for stage-specific gene groups (defined in Fig. 2b) by T2D GWAS 99% credible variants. The significance of enrichment of each gene group was measured by nominal p-value after randomly selecting the same number of genes in the genome. (For more details, see Methods). Multiple tests are adjusted by qvalue.
Extended Data Fig. 8 Molecular profiling of lineage specification between α-like and β-like lineages.
a, PCA analysis of pooled populations for the bifurcation of β-like and α-like lineages, including S5-I, S6-E, S7-H for α-like and S5-I, S6-D, S7-I for β-like, respectively. α-like in yellow, β-like in green, cells of BP2 (S5I) is in grey. b, Heatmap showing β-like vs α-like lineage switch genes. 282 genes are specifically up-regulated in β-like lineage. 58 genes are specifically up-regulated in α-like lineage. c, Fold change between primary β vs. primary α cells computed from human islet Drop-Seq for each gene in (b). d, GSEA functional enrichment in β-like and α-like lineage genes respectively. Color intensity indicates enrichment score (One-sided binomial test, adjusted q value). Gene numbers of all enriched functional terms are shown. The exact q values for the presented 8 terms (top-down) are: 2.6*10-2, 1.1*10-15, 9.6*10-9, 3.8*10-9, 7*10-3, 1.9*10-5, 4.9*10-5, 4.2*10-5. e, The β-like and α-like lineage switch transcription factors. f, Fold change between primary β vs. primary α cells computed from human islet Drop-Seq for each lineage transcription factor in (e).
Extended Data Fig. 9 Assessing the effects of ROCKII inhibition on the endocrine differentiation and protocol optimization.
a, The tSNE plots of S5 ~ S7 cells differentiated with or without ROCKII inhibitor (Ro.In). Green: endocrine cells; grey: non-endocrine cells. b, Heatmap of 6872 unimodal time-dependent genes on ES to beta-like lineage on two protocols. c, Marker genes visualized on lineage tree of both standard and Ro.In+ protocol. The expression patterns of marker genes are consistent in two protocols. NEUROG3 for endocrine precursors; CHGA, CHGB, CPE, PAX6, NEUROD1 for endocrine cells. FOXA2, PDX1, INSM1, INS for β-like cells. GCG, ARX for α-like cells. SST, HHEX for delta-like cells. d, PCA analysis for three populations: primary β cell (Grey); β-like cell in standard protocol(S7-I); β-like cell in Ro-in+ protocol(S7R-F). e, Hormone gene transcripts proportion. Top panel is insulin transcripts proportion across 6 populations: islet β; β-like in standard protocol; β-like in Ro-in+ protocol; islet α; α-like in standard protocol; α-like in Ro-in+ protocol. Bottom panel is glucagon proportion across those 6 cell populations. (n= 3953, 421, 1676, 5700, 732, 655 single cells for islet-β, β-like, β-like-Ro.In+, islet-α, α-like, α-like-Ro.In+ respectively). Boxplots are shown within the violin plots, where the middle line is the median, the lower and upper hinges correspond to the first and third quartiles, the upper whisker extends from the hinge to the largest value no further than 1.5×IQR from the hinge, the lower whisker extends from the hinge to the smallest value at most 1.5×IQR of the hinge (IQR is the inter-quartile range) (f) Schematics of six differentiation protocols with or without ROCKII inhibition at different stages. g, Visualization of ROCK2 expression in the lineage tree. h, Flow cytometry data showing the yield of pancreatic progenitors (NKX6.1+/PDX1+), endocrine cells (NKX2.2+ CHGA+) and β-like cells (C-peptide+/NKX6-1+) at the end of stage 4, Stage 5, and Stage 6 with different protocols in (f). The standard protocol without ROCKII inhibition (condition 6) was included as control.
Extended Data Fig. 10 H3K27ac ChIP-seq on the promoters and distal enhancers of double wave genes.
a, Density heatmap displaying the H3K27ac on TSSs of double wave genes across 7 stages. ChIP-seq signal is plotted as reads per million per base pair in TSS ± 5kb region (b) Density heatmap displaying the H3K27ac on putative distal enhancers within 250kb from TSSs of double wave genes across 7 stages. ChIP-seq signal is plotted as reads per million per base pair in enhancer peak center ± 5kb region. c, Eight T2D GWAS fine-mapping signals at TCF7L2 loci reported by Mahajan et al. Black vertical lines in the top tracks are 99% credible variants in each signal; red lines are the leading SNPs.
Supplementary information
Supplementary Information
Supplementary Figs. 1–8 and Tables 1 and 2
Supplementary Data 1
Supplementary Data 1
Supplementary Data 2
Supplementary Data 2
Supplementary Data 3
Supplementary Data 3
Supplementary Data 4
Supplementary Data 4
Supplementary Data 5
Supplementary Data 5
Source data
Source Data Fig. 7
Unprocessed gel for Fig. 7d.
Rights and permissions
About this article
Cite this article
Weng, C., Xi, J., Li, H. et al. Single-cell lineage analysis reveals extensive multimodal transcriptional control during directed beta-cell differentiation. Nat Metab 2, 1443–1458 (2020). https://doi.org/10.1038/s42255-020-00314-2
Received:
Accepted:
Published:
Issue Date:
DOI: https://doi.org/10.1038/s42255-020-00314-2
This article is cited by
-
Comparative and integrative single cell analysis reveals new insights into the transcriptional immaturity of stem cell-derived β cells
BMC Genomics (2024)
-
FGF7 enhances the expression of ACE2 in human islet organoids aggravating SARS-CoV-2 infection
Signal Transduction and Targeted Therapy (2024)
-
Chromosome 20p11.2 deletions cause congenital hyperinsulinism via the loss of FOXA2 or its regulatory elements
European Journal of Human Genetics (2024)
-
Single-nucleus multi-omics of human stem cell-derived islets identifies deficiencies in lineage specification
Nature Cell Biology (2023)
-
Protocol development to further differentiate and transition stem cell-derived pancreatic progenitors from a monolayer into endocrine cells in suspension culture
Scientific Reports (2023)