Abstract
Worldwide, primary liver cancer is the third leading cause of cancer-related death. Hepatocellular carcinoma (HCC) accounts for the majority of primary liver cancers. Recent studies have shown that circular RNAs (circRNAs) that interact with microRNAs (miRNAs) are involved in the occurrence and development of various tumours. Transcriptional profile analysis was used to analyse expression of circRNAs in HCC in this study. The top ten upregulated circRNAs were selected and validated by quantitative reverse transcription polymerase chain reaction (qRT-PCR) in another 34 HCC patients. MiRNAs and mRNAs downstream of these circRNAs were explored through database analysis, and finally, the competitive endogenous RNA (ceRNA) networks were constructed for 5 selected circRNAs. We identified 9658 differentially expressed circRNAs by transcriptional profile analysis. QRT-PCR was performed to validate the top ten upregulated circRNAs, and five circRNAs were selected for further analysis. The miRNAs and mRNAs downstream of these five circRNAs were predicted to construct ceRNA network diagrams. Further analysis revealed five circRNA–miRNA–mRNA axes that correlate negatively with HCC prognosis. Numerous differentially expressed circRNAs exist in HCC, and they can regulate the biological behaviour of HCC through ceRNA networks. Bioinformatics analysis showed that ceRNA regulatory axes involved in HCC have high diagnostic and prognostic value and deserve further exploration.
Similar content being viewed by others
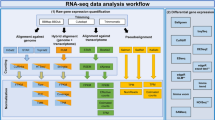
Introduction
According to the statistics of world cancer data in 2020, primary hepatic carcinoma (PHC) is the sixth most common cancer in the world and the third cause of cancer-related death, with approximately 906,000 new cases every year, resulting in 830,000 deaths1. Compared with statistical results in 2018, the incidence rate and case fatality rate of PHC have shown a gradual increase2. There are three types of PHC: HCC, intrahepatic cholangiocarcinoma (ICC), and mixed type. HCC accounts for the highest proportion (75–85%), followed by ICC (10–15%). There are significant differences in pathophysiological mechanism, biological behaviour, treatment regimen and prognosis among the three types of PHC1,3. In addition, the prevalence of HCC varies greatly geographically, with the majority of cases occurring in Asia. Many patients with liver cancer have no obvious specific symptoms in early stages, and liver cancer is often discovered incidentally during liver disease follow-up or when combined with liver ultrasound and AFP testing4. When symptoms appear, the liver cancer is most often at an advanced stage, and the opportunity for radical surgical treatment is lost. There are several treatment options for patients with advanced disease, but the tumour metastasis and recurrence rates are high5. Hence, there is an urgent need to explore new biomarkers to diagnose HCC early and improve the prognosis of these patients.
Recent advances in high-throughput sequencing technology and bioinformatics tools have led to an increasing number of noncoding RNAs (ncRNAs) being found to play an important role in human biological process, especially in the occurrence and development of cancer6. NcRNAs include microRNAs (miRNAs), long noncoding RNAs (lncRNAs), and circRNAs7. Among these ncRNAs, miRNAs function in post-transcriptional regulation by binding to miRNA response elements (MREs) in target mRNAs. An increasing number of studies have shown that target genes containing common MREs can compete for the same miRNAs. Therefore, in 2011, Salmena et al. proposed the ceRNA hypothesis8, which has attracted widespread attention from researchers and stated that coding and ncRNA molecules with common MREs can compete for miRNA binding at these loci and thus indirectly reciprocally regulate expression by acting as miRNA sponges9. CircRNAs are a novel class of noncoding ceRNAs and have a circular structure formed by the covalent closure of precursor messenger RNAs (pre-mRNAs) through backsplicing10. CircRNAs have no 5′ cap or 3′ tail and are thus not easily degraded by ribonucleases. In addition, they are stable and highly conserved in tissues and cells. Therefore, circRNAs have been widely studied11,12.
In this study, we used a combined strategy of gene microarray analysis and computational biology to investigate novel circRNAs and their potential mechanisms in HCC. A flowchart summarizing the current work is shown in Fig. 1. First, we screened for differentially expressed circRNAs in liver cancer tissues by transcriptional profile analysis and confirmed their expression using qRT‒qPCR. To determine whether circRNAs play roles as ceRNAs in HCC, we identified their sponged and target miRNAs to construct circRNA–miRNA‒mRNA networks. Then, we intersected the mRNAs in the ceRNA networks with upregulated genes in the GEPIA database to verify expression of these mRNAs. Finally, we constructed ceRNA network maps based on ten circRNAs and their respective potential interacting miRNAs/mRNAs to better investigate their potential mechanisms of action in HCC.
Methods
Collection of tissue specimens
A total of 34 HCC patients were included in this study. The clinical data of 34 patients are shown in Table 1. Tumour tissue samples and paired adjacent normal tissue samples from these patients were obtained during surgical treatment at the Department of General Surgery, Nantong Third People’s Hospital affiliated with Nantong University, China. The samples were frozened in liquid nitrogen immediately after resection and stored at − 80 °C before use. This study was approved by the Ethics Committee of Nantong Third People’s Hospital, and an informed consent form was signed by every participant. The ethics approval number is EL2021012.
Transcriptional profile analysis
We commissioned CapitalBio Technology (Beijing, China) to perform transcriptional profile analysis. The specific methods were as follows: CapitalBio Technology human circular RNA chip v2 expression profile chip was used to detect the total RNA of samples. In vitro amplification and fluorescence labelling were performed using an Ambion WT Expression kit.
CircRNA data analysis
The CapitalBio Human CircRNA Array v2.1 was designed with 4 identical arrays per slide (4 × 180 K format), detects human gene expression, using AG-GE-WL11-01-2010 and AG-GEDL00-01-2010. Post-transcriptional profile analysis was performed by CapitalBio Technology with the following steps. The image after hybridization scanning were preprocessed and converted into quantifiable values by Feature Extraction software. The output of this process is raw data file,which was imported into Gene Spring software, and the grouping and other parameter information were recorded. The “Percentile 75” method of GeneSpring GX software was used for normalization (the ratio was obtained by dividing the 75th digit value of each chip’s own expression data). The value generated after log2 was taken, comments were added, and the data were finally gathered into a total data table that contained the raw and normalized signal values for all samples on the chip, along with the corresponding annotated information. Cluster analysis and graphical presentation were performed using Cluster 3.0 software. Based on the grouping information, differential expression comparisons were performed, and differentially expressed genes were obtained.
Gene ontology (GO) and Kyoto Encyclopedia of Genes and Genomes (KEGG) enrichment analyses
We performed Gene Ontology (GO) and Kyoto Encyclopedia of Genes and Genomes (KEGG) enrichment analysis on 278 differentially expressed target mRNAs. Functional enrichment of DE genes, using gene sets from GO and KEGG, was determined with Fisher’s exact test, as implemented in the clusterProfiler Bioconductor package13.
Validation of candidate circRNAs using quantitative reverse transcription polymerase chain reaction (qRT-PCR)
Total RNA was extracted from the tissue samples using RNAiso Plus (Takara, Beijing, China) according to the manufacturer’s protocol. Total RNA was reverse transcribed complementary DNA (cDNA) using PrimeScript RT Master Mix (Takara), which was used as a template for determining relative expression of circRNAs with TB Green Premix Ex Taq II (Takara), and the results were normalized to GAPDH as a control. The PCR primers used are listed in Table 2.
Prediction of circRNA–miRNA‒mRNA networks
CircRNA–miRNA‒mRNA interactions were predicted by commonly used target gene prediction databases, and networks were constructed by Cytoscape_v3.9.1 software. The CircInteractome (https://circinteractome.nia.nih.gov/), miRDB (http://mirdb.org/), starBase (https://starbase.sysu.edu.cn/) and TargetScan (https://www.targetscan.org/vert_80/) databases were used for specific predictions. For each circRNA, we show the overlapping miRNAs in CircInteractome and starBase. The mRNAs of each target miRNA were predicted by determining the overlap among miRDB, starBase and TargetScan. If there were more than 20 overlapping mRNAs, we screened the top 20 mRNAs based on the miRDB database’s Target Score.
Selected circRNA–miRNA–mRNA axes
We determined the overlap between all the target mRNAs in the ceRNA networks, with the GEPIA (http://gepia.cancer-pku.cn/) database, and obtained 14 upregulated differentially expressed genes in HCC. Then, we further screened the differentially expressed genes through the UALCAN (http://ualcan.path.uab.edu/) and KM Plotter (http://kmplot.com/analysis/) databases and finally obtained 4 highly expressed differentially expressed genes that correlated negatively with survival. A mechanistic diagram of the ceRNAs was constructed according to the circRNA–miRNA–mRNA action mechanisms.
Statistical analysis
The circRNA–miRNA‒mRNA ceRNA network diagrams were generated by Cytoscape v3.9.1. GraphPad Prism 8.0 and SPSS 25.0 were used for data analysis. All data are expressed as the mean ± standard deviation (SD) values. Statistical significance was defined as P < 0.05 (Supplementary information).
Ethics approval and consent to participate
All patients were informed of the purpose of the study and signed informed consents, and the collection of specimens was approved by the ethics committee of Nantong Third People’s Hospital (EL2021012). All methods were carried out in accordance with relevant guidelines and regulations.
Results
Differentially expressed circRNAs in HCC tissues were analysed by transcriptional profile analysis
We used transcriptional profile analysis to analyse differentially expressed circRNAs in cancer and noncancerous tissues of five HCC patients. The raw output data of differential expression analysis have uploaded as supplementary information. As shown in a volcano plot, differentially expressed circRNAs were screened with log2 |(fold change)| >1 and P<0.05 as the criteria (Fig. 2A). A total of 175,875 circRNAs were identified in tissue samples by transcriptional profile analysis; most of these circRNAs are approximately 100–500 nucleotides in length (Fig. 2B). Among the 175,875 circRNAs, 9658 were significantly differentially expressed: 3862 significantly upregulated and 5796 significantly downregulated (Fig. 2C). In addition, we classified all differentially expressed circRNAs according to their chromosomal locations. The circRNAs on all chromosomes were differentially expressed (Fig. 2D). A heatmap shows these significantly differentially expressed circRNAs (Fig. 2E), and a Circos plot clearly demonstrates the degree of the differentially expressed gene based on chromosomal location (Fig. 2F).
Differentially expressed circRNAs in hepatocellular carcinoma tissues were analysed by transcriptional profile analysis. (A) The volcano plot shows the expression profiles in the two groups. The horizontal line represents fold change (log 2). The vertical line represents P value (−log10). The red dots in the figure represent the significantly upregulated circRNAs, and the green dots in the figure represent the significantly downregulated circRNAs. (B) The length distribution of circRNAs. (C) The numbers of total circRNAs and differentially expressed circRNAs. (D) The distribution of circRNAs on human chromosomes. (E) Hierarchical clustering demonstrated these significant differentially expressed circRNAs. (F) The Circos plot clearly shows the degree of differences in gene expression based on the chromosomal location of the genes.
Validation of differentially expressed genes and prediction of downstream target miRNAs
We selected the top 10 circRNAs with the largest difference according to the log2FC ranking at P < 0.05. To validate the transcriptional profile analysis results of these 10 circRNAs, their expression levels in cancer and noncancerous tissues from 34 HCC patients were verified by qRT-PCR. Hsa_circ_0060361 and hsa_circ_0001135 expression levels were too low to be verified. The results for the remaining 8 circRNAs (6 upregulated and 2 downregulated) were generally consistent with the transcriptional profile analysis results (Fig. 3A). Hsa_circ_0002514 did not show a statistically significant difference because its basic expression was too low. Then, we selected the remaining five statistically significant circRNAs, hsa_circ_0079875, hsa_circ0091580, hsa_circ0091581, hsa_circ0004788 and hsa_circ_0059730, for further analysis. We subsequently analysed these remaining circRNAs with StarBase and CircInteractome (taking the intersection of the results from the two databases to predict the downstream miRNAs of the five circRNAs) (Fig. 3B, C, D, E and F). We used the overlap among three databases, miRDB, TargetScan and StarBase, to predict the downstream mRNAs of these miRNAs (Fig. 3G).
Differential expressed gene validation and downstream target miRNA prediction. (A) CircRNA expression was verified by qRT-PCR. The top ten circRNAs with upregulated expression were identified by RNA-seq, and qRT-PCR was used to evaluate their expression in 34 HCC patients, two of which had low RNA expression and are not shown in the figure (B, C, D, E and F) Prediction of target miRNAs downstream of the five differentially expressed circRNAs (G). The mRNA targeted by each target miRNA was predicted by taking the intersection of the results from the miRDB, StarBase and TargetScan databases.
CeRNA network diagram visualization
We predicted the downstream mRNAs of the miRNAs by taking the intersection of the results from miRDB, TargetScan and StarBase. The overlapping mRNAs were screened by the target score in miRDB, and 20 downstream mRNAs of each miRNA were selected to construct ceRNA network diagrams (Fig. 4A, B, C, D and E).
GO term and KEGG pathway enrichment analyses of mRNAs in the ceRNA network
GO enrichment analysis includes three categories: cellular component, biological process, and molecular function. In cellular component analysis, the three most enriched terms were neuronal cell body, Golgi apparatus subcompartment and apical part of cell. In biological process analysis, negative regulation of neurogenesis, negative regulation of nervous system development and limbic system development were the three most enriched terms. In molecular function analysis, transcription coregulator binding, SMAD binding and apolipoprotein binding were the most prominent terms (Fig. 5A). We constructed a GO network by intersecting the first five components of the GO enrichment analysis with mRNAs (Fig. 5B). KEGG pathway enrichment analysis showed the functions of the mRNAs in the ceRNA network, microRNAs in cancer, human papillomavirus infection and human T-cell leukaemia virus 1 infection (Fig. 5C). We constructed a KEGG network by intersecting the first five components of KEGG enrichment analysis with mRNA (Fig. 5D).
Selected circRNA–miRNA–mRNA axes
A total of 2207 differentially expressed mRNAs in HCC patients, of which 1482 were upregulated and 725 downregulated, were identified in the GEPIA database. We intersected the upregulated mRNAs in GEPIA with all the mRNAs in our ceRNA network diagrams (Fig. 6A) and obtained 14 candicates. Analysis of relative expression was performed by collating the original data from the GEPIA database (Fig. 6B). Further screening of expression and survival correlations was performed with both the UALCAN and KM Plotter databases, and four differentially expressed genes with high expression and a negative correlation with survival were ultimately obtained: ADSL, AP3B1, MAPRE1, and TRNP1 (Fig. 6C, D, E and F).
Screening of differentially expressed genes. (A) Intersection of upregulated mRNAs with the miRNA-targeted mRNAs in HCC from the GEPIA database. (B) Relative expression of 14 upregulated mRNAs in the GEPIA database in HCC and adjacent paracancerous tissues. (C) Expression of differentially expressed gene ADSL in HCC (UALCAN) and its correlation with prognosis (UALCAN and KM Plotter). (D) Expression (UALCAN) and prognostic correlation (UALCAN and KM Plotter) of differentially expressed gene AP3B1 in HCC. (E) Expression (UALCAN) and prognostic correlation of the differentially expressed gene MAPRE1 in HCC (UALCAN and KM Plotter). (E) Expression of the differentially expressed gene TRNP1 in HCC (UALCAN) and its correlation with prognosis (UALCAN and KM Plotter).
Based on the above results, we identified 5 circRNA–miRNA–mRNA mechanistic axes: hsa_circ_0091581/miR-1179/AP3B1, hsa_circ_0059730/miR-516a-5p/ADSL, hsa_circ_0059730/miR-378g/TRNP1, hsa_circ_0059730/miR-515-5p/MAPRE1, and hsa_circ_0091580/miR-1179/AP3B1. Finally, we established a schematic diagram based on the roles of the circRNA–miRNA–mRNA axes, as shown in Fig. 7.
Mechanism of action by which the circRNA–miRNA–mRNA axes affect patient prognosis. The five circRNA–miRNA–mRNA mechanistic axes: hsa_circ_0091581/miR-1179/AP3B1, hsa_circ_0059730/miR-516a-5p/ADSL, hsa_circ_0059730/miR-378g/TRNP1, hsa_circ_0059730/miR-515-5p/MAPRE1, and hsa_circ_0091580/miR-1179/AP3B1.
Discussion
Increasing evidence indicates that circRNAs are dysregulated in HCC and can regulate gene expression at the transcriptional or post-transcriptional level, mediating immune escape, drug resistance, proliferation, invasion, metastasis and various malignant biological behaviours of HCC cells14. Moreover, circRNAs have high potential as biomarkers for early diagnosis and prognostic assessment15,16. These characteristics are verified by the detectability of circRNA in human plasma, saliva, urine and other body fluids17. Using transcriptional profile analysis, we found numerous circRNAs (175,875), 9658 of which were dysregulated in HCC and distributed across every human chromosome. Research has confirmed that humans with Down syndrome are at low risk of developing solid tumours due to amplification of several tumour-suppressor genes on human chromosome 21 (HSA21)18. Based on this, Peng et al. found that circPTTG1IP derived from HSA21 can inhibit development of HCC19. This means that we can infer the possible biological function of circRNA based on the distribution of circRNA chromosomes. Most circRNAs are 100–500 nucleotides long, that is, small molecules. CircRNAs are widely distributed and expressed in all organs and play different roles in various diseases. Many valuable circRNAs deserve exploration.
An increasing number of experiments have demonstrated that circRNAs can promote development of HCC by regulating various signals involved in the cell cycle, proliferation, differentiation, cell survival and apoptosis, leading to abnormal activation of several molecules in signal transduction pathways20. Studies have confirmed that circRNAs can act via the AKT/ERK21, Wnt/β-catenin22, nuclear factor-kappa B23, hedgehog24, c-Met25, JAK2/STAT326 and other signalling pathways and are involved in the malignant biological behaviours of HCC. Recent studies have shown that circRNAs not only exert biological functions through the above signalling pathways but also affect the biological processes of HCC through other pathways. For instance, Liu et al. found that the circRNA cIARS regulates ferroptosis in HCC cells by interacting with the RNA-binding protein ALKBH527, Zhang et al. demonstrated that cancer cell-derived exosomal circUHRF1 induces natural killer cell exhaustion and may cause resistance to anti-PD1 therapy in HCC28, and Li et al.29 discovered that circRPN2 inhibits aerobic glycolysis and metastasis in HCC. Moreover, Cao et al.30 verified that hsa_circ_0003410 promotes HCC progression by increasing the ratio of M2/M1 macrophages through the miR-139-3p/CCL5 axis, Liu et al. found that EIF4A3-induced circTOLLIP promotes progression of HCC via the miR-516a-5p/PBX3/EMT pathway, and Wu et al.31 confirmed that methyltransferase-like 3-mediated m6A methylation of hsacirc_0058493 accelerates HCC progression by binding to YTH domain-containing protein 1.
In our study, 5 circRNAs, 15 miRNAs, and 278 mRNAs were identified through transcriptional profile analysis, and 5 ceRNA network maps were constructed of highly expressed circRNAs. A total of 278 mRNAs were further screened, and 4 highly expressed mRNAs with prognostic value were selected. We found that circRNAs can influence the prognosis of patients through ceRNA networks. MAPRE1 promotes cell cycle progression in HCC cells by interacting with CDK232. Adenylosuccinate lyase (ADSL), a key enzyme involved in the de novo purine synthesis pathway, is a potential drug target in HCC33. At present, there is no research on AP3B1 and RNP1 in liver cancer, which is worth exploring. In addition, we combined bioinformatics analysis and qRT-PCR results to obtain five circRNA–miRNA–mRNA mechanistic axes that correlate negatively with HCC prognosis (hsa_circ_0091581/miR-1179/AP3B1, hsa_circ_0059730/miR-516a-5p/ADSL, hsa_circ_0059730/miR-378g/TRNP1, hsa_circ_0059730/miR-515-5p/MAPRE1, and hsa_circ_0091580/miR-1179/AP3B1). To date, there has been no research on these five circRNA–miRNA–mRNA mechanistic axes, which are worthy of further exploration. We also performed GO and KEGG enrichment analyses of the 278 mRNAs in the ceRNA network to accurately study the function of these ceRNAs.
Studies on the detailed mechanisms of ceRNA networks and their relationships with HCC are still in their initial stages. Despite increasing interest in exploiting circRNAs as ceRNAs in HCC, little attention has been given to interactions in ceRNA regulatory networks mediated by circRNAs in HCC. Thus, there is a need to investigate the properties, roles and mechanisms of ceRNAs in different stages of HCC. To date, studies of ncRNAs that act as ceRNAs in HCC have mainly involved overexpression and knockdown experiments in cells and animals. However, other factors, such as the subcellular location and abundance of ceRNA components, interactions with RNA-binding proteins, RNA editing, and ceRNA affinity, also affect ceRNA activity. Whether the results of overexpression assays can truly reflect the spontaneous action mechanisms of ceRNAs during carcinogenesis in liver cancer patients remains unknown. Therefore, more animal experiments and clinical trials should be performed for validation. Furthermore, most identified ceRNA interactions show a single binding partner, though ceRNAs crosstalk occurs in large interconnected networks. In addition to direct interactions via shared miRNAs, secondary and indirect interactions may significantly affect ceRNA regulation34. Our bioinformatics analysis showed that each circRNA targets several miRNAs; for example, hsa_circ_0091580 targets 64 miRNAs, of which three miRNAs overlapped between two databases. In addition, both hsa_circ_0091580 and hsa_circ_0091581 target miR-1179. According to our study, a circRNA may target several miRNAs at the same time, and we are exploring whether there is a correlation between these miRNAs. Moreover, several circRNAs may also act together on a single miRNA, and whether several circRNAs interact with each other is unknown. Whether there is a correlation between individuals within the ceRNA network will be our next research focus.
However, our study also has some limitations. The results of this study are based on bioinformatic predictions and require further experimental and clinical verification. In addition, data from available datasets are limited and insufficient for prognostic analysis because some circRNAs and miRNAs have not been adequately studied in HCC. We expect that more studies with larger sample sizes will allow us to extend and refine our conclusions.
Conclusion
In conclusion, by transcriptional profile analysis and integration of data from various databases, we successfully constructed a ceRNA network containing 5 circRNAs, 15 miRNAs, and 278 mRNAs. CeRNA networks contribute to progression of HCC through signal transduction, overall regulation of biological functions, and other pathways. Bioinformatics analysis and qRT-PCR results were combined to obtain five circRNA–miRNA–mRNA mechanistic axes correlating negatively with HCC prognosis, which have high diagnostic and prognostic value and deserve further exploration. Our findings are expected to provide potential biomarkers and therapeutic targets for HCC management.
Data availability
All the data are reliable and all the reagents and devices described in the article are commercially available. The transcriptional profile analysis data was uploaded to the GEO database (https://www.ncbi.nlm.nih.gov/geo/query/acc.cgi?) at the NCBI website under accession number GSE242797. We have uploaded the raw output data of differential expression analysis as a supplementary file. The private access token before this manuscript is published is opmpiaugtfivpgf. After the manuscript is approved, we will immediately apply for data publication.
Abbreviations
- circRNAs:
-
Circular RNAs
- ceRNAs:
-
Competing endogenous RNAs
- HCC:
-
Hepatocellular carcinoma
- qRT-PCR:
-
Quantitative reverse transcription polymerase chain reactio
- ncRNAs:
-
Noncoding RNA
- miRNAs:
-
MicroRNAs
- lncRNAs:
-
Long noncoding RNAs
- MREs:
-
MiRNA response elements
- pre-mRNAs:
-
Precursor messenger RNAs
- PKR:
-
Protein kinase R
- GO:
-
Gene Ontology
- KEGG:
-
Kyoto Encyclopedia of Genes and Genomes
- cDNA:
-
Complementary DNA
- SD:
-
Standard deviation
- HSA21:
-
Human chromosome 21
- ADSL:
-
Adenylosuccinate lyase
References
Sung, H. et al. Global cancer statistics 2020: GLOBOCAN estimates of incidence and mortality worldwide for 36 cancers in 185 countries. CA Cancer J. Clin. 71(3), 209–249 (2021).
Bray, F. et al. Global cancer statistics 2018: GLOBOCAN estimates of incidence and mortality worldwide for 36 cancers in 185 countries. CA Cancer J. Clin. 68(6), 394–424 (2018).
Anwanwan, D. et al. Challenges in liver cancer and possible treatment approaches. Biochim. Biophys. Acta Rev. Cancer 1873(1), 188314 (2020).
Gilles, H., Garbutt, T. & Landrum, J. Hepatocellular carcinoma. Crit. Care Nurs. Clin. N. Am. 34(3), 289–301 (2022).
Ju, L. et al. Circular RNA hsa_circ_0051040 promotes hepatocellular carcinoma progression by sponging miR-569 and regulating ITGAV expression. Cells 11(22), 3571 (2022).
Chen, L. et al. The bioinformatics toolbox for circRNA discovery and analysis. Brief. Bioinform. 22(2), 1706–1728 (2021).
Mao, M. et al. Role of exosomal competitive endogenous RNA (ceRNA) in diagnosis and treatment of malignant tumors. Bioengineered 13(5), 12156–12168 (2022).
Sun, J. et al. A novel lncRNA ARST represses glioma progression by inhibiting ALDOA-mediated actin cytoskeleton integrity. J. Exp. Clin. Cancer Res. 40(1), 187 (2021).
Qi, X. et al. Prognostic roles of ceRNA network-based signatures in gastrointestinal cancers. Front. Oncol. 12, 921194 (2022).
He, Y. et al. Circular RNA-related CeRNA network and prognostic signature for patients with oral squamous cell carcinoma. Front. Pharmacol. 13, 949713 (2022).
Memczak, S. et al. Circular RNAs are a large class of animal RNAs with regulatory potency. Nature 495(7441), 333–338 (2013).
Li, S. et al. RNA-Seq profiling of circular RNAs during development of hindgut in rat embryos with ethylenethiourea-induced anorectal malformations. Front. Genet. 12, 605015 (2021).
Kanehisa, M. et al. KEGG for taxonomy-based analysis of pathways and genomes. Nucleic Acids Res. 51(D1), D587-d592 (2023).
Huang, Z. et al. The mechanism and clinical significance of circular RNAs in hepatocellular carcinoma. Front. Oncol. 11, 714665 (2021).
Xiong, D. et al. The latest overview of circRNA in the progression, diagnosis, prognosis, treatment, and drug resistance of hepatocellular carcinoma. Front. Oncol. 10, 608257 (2020).
Xu, H. et al. RNA-Seq profiling of circular RNAs in human colorectal cancer liver metastasis and the potential biomarkers. Mol. Cancer 18(1), 8 (2019).
Kristensen, L., Jakobsen, T., Hager, H. et al. The emerging roles of circRNAs in cancer and oncology (2021).
Nižetić, D. & Groet, J. Tumorigenesis in Down’s syndrome: Big lessons from a small chromosome. Nat. Rev. Cancer 12(10), 721–732 (2012).
Peng, R. et al. Down-regulation of circPTTG1IP induces hepatocellular carcinoma development via miR-16-5p/RNF125/JAK1 axis. Cancer Lett. 543, 215778 (2022).
Alqahtani, A. et al. Hepatocellular carcinoma: Molecular mechanisms and targeted therapies. Medicina 55(9), 526 (2019).
Liu, Y. M. et al. CircCCNB1 silencing acting as a miR-106b-5p sponge inhibited GPM6A expression to promote HCC progression by enhancing DYNC1I1 expression and activating the AKT/ERK signaling pathway. Int. J. Biol. Sci. 18(2), 637–651 (2022).
Li, D. et al. CircMTO1 suppresses hepatocellular carcinoma progression via the miR-541-5p/ZIC1 axis by regulating Wnt/β-catenin signaling pathway and epithelial-to-mesenchymal transition. Cell Death Dis. 13(1), 12 (2021).
Zhou, Y. et al. Cancer-associated fibroblast exosomes promote chemoresistance to cisplatin in hepatocellular carcinoma through circZFR targeting signal transducers and activators of transcription (STAT3)/ nuclear factor -kappa B (NF-κB) pathway. Bioengineered 13(3), 4786–4797 (2022).
Wang, L. et al. Circ_0036412 affects the proliferation and cell cycle of hepatocellular carcinoma via hedgehog signaling pathway. J. Transl. Med. 20(1), 154 (2022).
Liu, Y., Xiao, X., Wang, J. et al. Silencing CircEIF3I/miR-526b-5p axis epigenetically targets HGF/c-Met signal to hinder the malignant growth, metastasis and angiogenesis of hepatocellular carcinoma. Biochem. Genet. (2022).
Zhao, Z. et al. CircSOD2 induced epigenetic alteration drives hepatocellular carcinoma progression through activating JAK2/STAT3 signaling pathway. J. Exp. Clin. Cancer Res. 39(1), 259 (2020).
Liu, Z. et al. Circular RNA cIARS regulates ferroptosis in HCC cells through interacting with RNA binding protein ALKBH5. Cell Death Discov. 6, 72 (2020).
Zhang, P. F. et al. Cancer cell-derived exosomal circUHRF1 induces natural killer cell exhaustion and may cause resistance to anti-PD1 therapy in hepatocellular carcinoma. Mol. Cancer 19(1), 110 (2020).
Li, J. et al. CircRPN2 inhibits aerobic glycolysis and metastasis in hepatocellular carcinoma. Cancer Res. 82(6), 1055–1069 (2022).
Cao, P. et al. hsa_circ_0003410 promotes hepatocellular carcinoma progression by increasing the ratio of M2/M1 macrophages through the miR-139-3p/CCL5 axis. Cancer Sci. 113(2), 634–647 (2022).
Wu, A. et al. Methyltransferase-Like 3-mediated m6A methylation of Hsa_circ_0058493 accelerates hepatocellular carcinoma progression by binding to YTH domain-containing protein 1. Front. Cell Dev. Biol. 9, 762588 (2021).
Liang, X. H. et al. MAPRE1 promotes cell cycle progression of hepatocellular carcinoma cells by interacting with CDK2. Cell Biol. Int. 44(11), 2326–2333 (2020).
Jiang, T. et al. Targeting the de novo purine synthesis pathway through adenylosuccinate lyase depletion impairs liver cancer growth by perturbing mitochondrial function. Hepatology 74(1), 233–247 (2021).
Shi, Y. et al. The role of ceRNA-mediated diagnosis and therapy in hepatocellular carcinoma. Hereditas 158(1), 44 (2021).
Acknowledgements
We would like to thank the Animal Center of Nantong University and the Institute of Liver Diseases of Nantong Third People’s Hospital for providing the experimental platform.
Funding
This study was supported by grants from Science and Technology Bureau (MS22018007), Six Peak Talents in Jiangsu Province (YY-177), Project of Jiangsu Province Youth Medical Talent Development (QNRC2016400) and Project of Nantong Youth Medical Talent Development (No.05), and Youth Fund of Natural Science Foundation of Jiangsu Province (BK20200965), Scientific Research Fund of Nantong Health Commission (MB2020037), Health Bureau of Nantong City (MB2021057). The funding source had no involvement in study design; in the collection, analysis and interpretation of data; in the writing of the report; and in the decision to submit the paper for publication.
Author information
Authors and Affiliations
Contributions
Y.L. performed the main part of the study and wrote original draft. Z.D. performed the main part of the study. W.C. provided technical support and analyzed the data. L.C., L.J., W.C. contributed to part of the experiments. Z.B. and X.L. designed the study, performed the main part of the study and guided the manuscript writing. Specimens from human hepatocellular carcinoma tumour tissue samples and paired adjacent normal tissue were obtained with written informed consent. All data were anonymized, and no information was traceable to any individual donor.
Corresponding authors
Ethics declarations
Competing interests
The authors declare no competing interests.
Additional information
Publisher's note
Springer Nature remains neutral with regard to jurisdictional claims in published maps and institutional affiliations.
Supplementary Information
Rights and permissions
Open Access This article is licensed under a Creative Commons Attribution 4.0 International License, which permits use, sharing, adaptation, distribution and reproduction in any medium or format, as long as you give appropriate credit to the original author(s) and the source, provide a link to the Creative Commons licence, and indicate if changes were made. The images or other third party material in this article are included in the article's Creative Commons licence, unless indicated otherwise in a credit line to the material. If material is not included in the article's Creative Commons licence and your intended use is not permitted by statutory regulation or exceeds the permitted use, you will need to obtain permission directly from the copyright holder. To view a copy of this licence, visit http://creativecommons.org/licenses/by/4.0/.
About this article
Cite this article
Liu, Y., Dong, Z., Chen, W. et al. Construction of a ceRNA regulatory network to explore potential pathogenesis mechanisms involved in human hepatocellular carcinoma. Sci Rep 13, 22058 (2023). https://doi.org/10.1038/s41598-023-47374-4
Received:
Accepted:
Published:
DOI: https://doi.org/10.1038/s41598-023-47374-4
Comments
By submitting a comment you agree to abide by our Terms and Community Guidelines. If you find something abusive or that does not comply with our terms or guidelines please flag it as inappropriate.