Abstract
Neurodevelopmental disorders are constantly increasing on a global scale. Some elements like heavy metals are known to be neurotoxic. In this cross-sectional study we assessed the neurobehavioral effect of the exposure to trace elements including lead, mercury, cadmium, manganese, arsenic and selenium and their interactions among 299 schoolchildren residing in the heavily polluted Taranto area in Italy. Whole blood, urine and hair were collected for metal analyses, while the Child Behavior Checklist and the Social Responsiveness Scale, administered to the main teacher and the mothers were considered to identify behavioral problems in children. Blood lead mainly influenced social problems, aggressive behavior, externalizing and total problems. Urinary arsenic showed an impact on anxiety and depression, somatic problems, attention problems and rule breaking behavior. A significant interaction between lead and arsenic was observed, with a synergistic effect of the two metals increasing the risk of attention problems, aggressive behavior, externalizing problems and total problems. Overall, we were able to test that higher blood lead, urinary arsenic concentrations and their interaction increase the risk of neurobehavioral problems. This is in line with the U.S. Environmental Protection Agency’s priority list of hazardous substances where arsenic and lead are ranked as first and second respectively.
Similar content being viewed by others
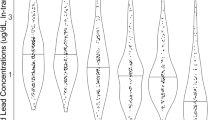
Introduction
Neurodevelopmental disorders including autism, Attention Deficit Hyperactivity Disorder (ADHD), learning disabilities and behavior, affect 10–15% of the births1 and are constantly increasing on a global scale2. Similar results were observed among US children3. In two Regions of Central Italy, about 1.3% of the Italian elementary and middle-school children were reported to suffer from severe ADHD4. According to the statistics on the incidence of autism from the U.S. Centers for Disease Control and Prevention (CDC), one child every 6–8 suffers from autistic spectrum disorders in the USA5. A comparison in temporal trends showed that the prevalence of diagnosed autism has risen dramatically in the U.S over the last several decades, mostly since the 1980s, and continues to trend upward. Average prevalence in Asia, Europe and North America varies between 1 and 2%6. These data have shown a tenfold increase in the last 40 years about 3% of developmental disabilities may be a consequence of environmental exposure to neurotoxicants and another 25% of the interaction between environmental hazards and individual genetic predisposition7,8. Environmental toxicants show increasing trends that correlate positively to the rise in autism9. Neurotoxic elements including lead (Pb), mercury (Hg), cadmium (Cd), manganese (Mn) and arsenic (As) are known being able to cross the placenta through sequence variations which can affect protein metabolizing and transporting metals10 and to cross the blood–brain barrier and interfere with neurons11,12 while selenium (Se) is a potentially protective agent for metabolic defense against oxidative stress, although its margin of safety is rather narrow13,14. Several studies on children environmentally exposed to these elements have shown impacts on attention and behavior and school performance15,16,17,18,19,20,21,22,23,24,25,26. Few works exploring behavioral effects of the exposure to metal mixture have found an increased harmful effect27,28,29,30. However, due to the complexity of the problem more evidences are needed about the synergistic effect of the exposure to metals on neurobehavior. The exact neurotoxicological mechanism through which a mixture of metals including Pb, Hg, Cd, Mn and As can lead to behavioral problems is still unclear and there is the need of more investigation and evidences on how metal mixtures and the interactions among the chemicals can influence behavior31.
In this study we assessed the neurobehavioral effect of the exposure to some elements including Pb, Hg, Cd, Mn, As and Se among 6–11 years old children residing in the heavily polluted Taranto area in Italy considering the interactions among the elements that showed a significant association with the behavioral outcomes.
Results
Table 1 shows the overall socio-demographic characteristics of participants, grouped also by the area of residence. Two of the neighborhoods closer to the industrial site (Tamburi and Paolo VI) were characterized by a lower percentage of high-level socio-economic status (SES) (1.3% and 0% respectively, Chi-squared p-value < 0.001), a higher frequency of passive smoking exposure (26.9% and 11.1% respectively, Chi-squared p-value < 0.001) and lower Intelligence Quotient (IQ) (median IQ equal to 94 and 102 respectively, compared to 110 in the neighborhoods of Statte and Talsano, Kruskall–Wallis p-value < 0.001) Children residing in Tamburi also showed lower cognitive stimulation at home (median (1Q-3Q) HOME total score equal to 5.0 (4.0, 6.0), Kruskall-Wallis p-value < 0.001), were slightly older and had a higher percentage of females than children living farther from the source.
Metal concentrations showed a low inter-correlation (Table S1 of the Supplementary Material) with the highest value equal to 0.276 corresponding to the correlation between urinary As (UAs) and hair Hg (HHg).
After checking the assumption of a linear relationship through cubic splines (data not shown), we observed a negative trend of metal concentrations at increasing distance from the source of exposure (Table 2). Only HHg and blood Se (BSe) showed a positive association.
A poor agreement was found between parents and teachers evaluation (ICC ranging from 0.06 for anxiety problems to 0.36 for conduct problems) while the skewness and kurtosis highlighted a non-normal distribution of the lower level empirically-based syndrome and the DSM oriented scales (skewness and kurtosis above 0.5 and 3 respectively for all these outcomes). Tables 3 and 4 show median and 1st–3rd quartiles interval of the neurobehavioral scores including the CBCL empirically based and DSM oriented scales reported by parents and teachers respectively. Higher scores indicate higher levels of problems in an area meaning better performance in the more distant areas while lower scores indicate fewer problems. The p-value of the Kruskal–Wallis is also reported.
We found significant differences among the areas of residence for social problems, externalizing problems, oppositional defiant problems and the SRS total score reported by parents while for the teachers report there was a significant difference in all the scores apart from the thought problems, attention problems, affective problems, ADHD problems and oppositional defiant problems. Overall, we are able to see higher score values for the areas at closer distance from the emission source compared to those at greater distance.
Multicollinearity analysis was performed when multivariable models were applied: low VIF were observed for all the models fitted for each outcome (all VIF < 5). The marginal effects of the neurotoxic elements and 95% CI of the censored regression for the CBCL sub-scales are shown in Table 5 (only significant results were displayed). Significant positive association were found between blood Pb (BPb) and social problems and aggressive behavior scales while UAs has a greater impact on the anxious depressed, somatic complaints, attention problems and rule breaking behavior scales. The estimated effects of each models were at the net of sex, age, SES, distance from the source, IQ, exposure to passive smoking and HOME total score. In Table 5 are also reported the results of linear mixed effect models. In this case only BPb has a statistically significant impact on the externalizing and total problems scales of the CBCL, while UAs has a marginally significant association with total problems and the SRS total score. In this case the models were adjusted for the same covariates as the previous ones, but the random effects allowed to consider the nested structure of the data (subjects, schools and areas of residence). Overall, we were able to test that higher BPb or UAs concentrations are associated with increased neurobehavioral problems. All the other elements did not appear to have a statistically significant association with children neurobehavior. Semipartial correlations applied to multivariable models confirmed the results obtained and displayed in Table 5 and 6 showing higher R2 for BPb when considering the social problems, aggressive behavior, externalizing and total problems as dependent variables while UAs significantly increased the explained amount of variability of anxious depressed, somatic complaints, attention problems and rule breaking behavior (the proportion of explained variance associated to BPb and UAs was greater than 7% in all these cases).
We finally introduced interaction terms between pairs of metals but only BPb and UAs showed a synergistic effect on child behavior. Figure 1 shows the effects of the interaction between BPb and UAs on aggressive behavior and attention problems. The first column of Fig. 1 shows the associations of both BPb (first row) and UAs (second row) with attention problems: for both metals we can see higher values of the β parameter at higher metal concentrations, moreover the effect becomes significant when at higher concentration levels of the interacting element. Specifically, BPb has a significant positive association with attention problems when UAs reaches its higher concentration levels (the black solid line corresponding to β = 0 is outside the CI when As is at the 75th and 90th percentiles). The same is for UAs where we can see a similar trend that reaches a significant association with attention problems even at lower BPb levels: the β parameter is significantly different from 0 starting from a concentration level of BPb corresponding to the median value. We can observe similar trends for aggressive behavior with a significant association with Pb, while As reaches statistical significance only for simultaneous very high Pb exposure (corresponding to the BPb 90th percentile). The results for anxiety and depression, somatic complaints, social problems and rule breaking behavior, showing association respectively with Pb and As, are reported in Figs. S1 and S2 of Supplementary Material.
BPb and UAs interaction association with aggressive behavior and attention problems. Association between BPb and UAs interaction and aggressive behavior (first column) and attention problems (second column). In each box the change of the β parameter is represented as a function of BPb (first row) or UAs (second row) concentration. Each line shows how the β coefficient varies across the different levels of the interacting metal (UAs for the first row and BPb for the second row).
When considering the externalizing problems, the total problems and the SRS total T score and adding the interaction term between BPb and UAs (Table 6), a significant conditional effect of both Pb and As is also observed for increasing UAs and BPb concentration respectively. BPb reached significance in the association with externalizing and total problems when UAs was at least equal to its median value. UAs showed a significant association with externalizing problems when BPb was as high as the 90th percentile, with total problems when BPb was equal or greater than the 60th percentile and with SRS total T score when BPb reached the 75th percentile.
We represented the effect of the interaction between BPb and UAs on CBCL total problems score in Fig. 2, to provide a better representation of how the association between the outcome and the BPb and UAs changed at increasing levels of the respective interacting element. A clear pattern of an increased metal impact on behavioral total problems at higher levels of both the element of interest and the interacting metal can be appreciated. The results for externalizing problems and SRS total T score are shown in Figs. S1 and S2 respectively of Supplementary Material.
BPb and UAs interaction association with total problems. BPb and UAs association with CBCL total problems when an interaction term between the two metal concentrations is included in the linear mixed effects model. Blue color corresponds to low level of Pb or As concentrations while red corresponds to high concentration levels.
Discussion
The aim of this study was to explore the association between the exposure to elements potentially neurotoxic and the neurobehavioral outcomes. We were able to identify Pb and As and their interaction as important risk factors in increasing the likelihood of neurobehavioral problems among the children residing in the province of Taranto.
Increased hyperactivity and psychopathological traits, impairment of social behavior and higher risk of autism were detected in the neighborhoods of Tamburi and Paolo VI, which are located at closer distance from the industrial emission. We were able to find major differences among the areas of residence considering the teacher reports compared to the parent ones. Along with a decrease of the neurobehavioral scores at higher distance from the plant (meaning fewer behavioral problems in the areas at greater distance) we observed negative trends also for understudied element concentrations but not for BSe and HHg. However, the first element is known to have a protective effect on neurodevelopment while the second can be confounded by fish intake; the lack of this information is a limitation of the current study.
When looking at the association between the biomarkers and the neurobehavioral outcomes in a multivariable model we were able to find significant association with BPb and UAs even adjusting for SES and the distance from the plant. BPb mainly influenced social problems, aggressive behavior, externalizing and total problems. UAs showed an impact on anxiety, depression, somatic problems, attention problems and rule breaking behavior beside a significant association with autism (measured through the SRS T total score). This is in line with the U.S. Environmental Protection Agency’s (EPA) priority list of hazardous substances where As and Pb are ranked as first and second respectively.
Because of the absence of multicollinearity among metals, the skewed distribution as well as the multilevel structure of the dependent variables we opted for the application of linear mixed effect models and censored regressions instead of more recent environmental mixture models like Weighted Quantile Sum (WQS) regression32 or Bayesian Kernel Machine Regression (BKMR)33. This choice did not allow us to estimate an overall effect of metal mixture as in WQS or BKMR, however we were still able to find the association between each chemical and the outcomes at the net of the exposure to the other elements and to account for interactions among metals. Through the inclusion of an interaction term between pairs of metals we were able to observe a multiplicative effect of Pb and As on child behavior. In particular a synergistic effect of the two chemicals was highlighted in the association with attention problems, aggressive behavior, externalizing and total problems. The mechanism of neurotoxicity of these two elements are different, as they involve the inhibition of some cellular elements and the rising of apoptotic factors causing the neuronal cell death. However, the mechanism through which the mixture of metals causes neuronal damage is still unclear and needs further exploration31.
Through this and the previous work Lucchini et al. 2019, we were able to observe an association of Pb on both behavior and cognition. These adverse effects of Pb were also showed by other studies34,35 and were associated to alterations in DNA and chromosomal integrity, interaction with proteins36 and alteration to cellular redox status37.
The biomonitoring measurement did not show elevated levels compared to the available standards or to other similar community studies38,39,40,41,42,43,44,45,46,47,48,49,50,51,52. Nevertheless, we were able to find a significant association between the exposure and the outcomes. This result highlights the importance of considering the possible interactions among pollutants that can amplify the effects of the exposure even at low levels as already pointed out by previous studies that showed how co-occurring metal exposure may contribute to a greater risk of autism29 and behavioral outcomes27,28,30. However, our data mainly reflect current exposure, and cannot exclude previous higher exposure levels that may have occurred in the past years when the plant was at higher production levels than today.
This is a complementary analysis to the previous study38 where we found an association between the exposures to neurotoxic elements and neurocognitive abilities among children residing in the Taranto area. In this study we considered the same population but focusing on neurobehavioral aspects. This study also highlights how disadvantaged areas are at increased risk of neurobehavioral problems besides the risk of lower neurocognitive abilities as already pointed out in Lucchini et al. 2019.
We observed a poor agreement between parent and teacher evaluation, however even if there was no available tendency to lie measure, thanks to the availability of both the parent and teacher reports we had more robust assessment of children neurobehavioral problems because of the different perception and expectation of the two persons being interviewed. Since teacher reports can only amplify the effect of chemicals on behavior within the same sex24, the double report from both teachers and parents can avoid to incur in this situation.
The study has also some limitation: we were not able to account for potential selection bias which may have been generated for the low participation rate; due to the cross-sectional nature of the study we cannot attribute a causative role to the neurotoxic elements on child behavior; the biomonitoring covered only current exposure excluding prenatal and postnatal periods which are considered vulnerable exposure windows, in particular the lack of information about previous exposure during the higher production levels of the plant is a limit in identifying the impact that this chemicals can have on the considered outcomes; UAs is a marker of short term exposure, the unavailability of As in hair or nails which are biomarkers of longer-term burden, is a limitation; we could also not consider the effect that concurrent exposure to other factors such as organic compounds can have on neurodevelopment; although we adjusted for several confounders there still was missing information like parental relations; finally the sample size was probably not sufficient to entirely detect the association of metals and their interactions on neurobehavior.
In this work we found that As and Pb can affect school-children behavior by increasing the risk of neurobehavioral problems and autism and have a synergistic effect on some child behavioral problems. Through this study we highlighted the importance of considering the interactions among pollutants since it can amplify the effect of the exposure even at low levels. Further work is needed to address the neurological effect of the exposure to mixtures of metals considering their interactions.
Methods
Study area and population
The study area, the sample selection and the recruitment strategies were already described in previous works38,53. Briefly, the study area is the city of Taranto, South Italy, where a wide industrial pole including one of the largest steel producers in Europe operates since many decades, causing emission of toxic elements and many other chemical compounds in a wide surrounding area. 12 primary schools located in the 5 sub-areas by incremental distance from the industrial pole were selected by the enrollment procedures.
A total of 700 children-parent pairs were invited to participate on voluntary bases to the study. Children from 6 to 11 years-old were enrolled through the public school system according to a community-based participatory approach that involved the community of Taranto. The aims and methodology of the study were explained through ad hoc meetings and the availability of brochures for teachers, parents and children. 432 subjects agreed to participate to the study showing a 62% participation rate. Informed consent was obtained from all parents. Subjects who agreed to participate filled in a screening questionnaire for the evaluation of inclusion criteria which were being born and raised in the target study areas, and having carried the entire pregnancy period of the mother in the same area at the time of recruitment, and the exclusion criteria: familiarity of neurodegenerative diseases, diagnosis/treatments for neurological and psychiatric illnesses, hepatic or biliary diseases, dysmetabolic diseases, endocrine disorders, kidney disorders, previous total parenteral nutrition and uncorrected visual defects. Overall 299 children matched the inclusion criteria reaching the minimum sample size estimated by power calculation where the effect of the 6 metals was considered on cognitive outcomes hypothesizing around 1.4% explanation of the outcome variability for each element based on a previous study50 and allowing for interactions and multiple testing but not considering the strata; in particular, for each school an average of 27 students were recruited ranging from a minimum of 16 to a maximum of 56. The study design, the information about the study aims and the forms for informed consent had been reviewed and approved by the ethical committees of the local Public Health Agency of Brindisi. All methods were performed in accordance with the relevant guidelines and regulations.
Elements biomonitoring
Whole blood, urine and hair were collected in the schools on a day before the neuropsychological testing. High Resolution-Inductively Coupled Plasma Mass Spectrometry (HR-ICP-MS) (ElementII, Thermo Scientific, Bremen, Germany) was used to measures in whole BPb and BSe, in UAs and urinary Cd (UCd), and in hair Mn (HMn)54,55. In addition, HHg was quantified by thermal decomposition amalgamation atomic absorption spectrometry (TDA-AAS) using a Direct Mercury Analyzer (DMA-80 TRICELL, FKV, Bergamo, Italy)56. Blood and urine were refrigerated at − 20 °C. Both analytical procedures have been extensively described in previous papers, including the quality control aspects, in Ruggieri et al. 2016 and Alimonti et al. 2015 for HR-ICP-MS and Domanico et al. 2017 for DMA analysis.
Psychological assessment, adult survey about the child
The neuropsychological assessment was conducted by four neuropsychologists who performed periodical intercalibration of testing procedure and scoring. The Child Behavior Checklist (CBCL), administered to the main teacher and to the mother57 was considered to identify problem behavior in children. This test measures eight empirically-based syndrome scales: Aggressive Behavior, Anxious/Depressed, Attention Problems, Rule-Breaking Behavior, Somatic Complaints, Social Problems, Thought Problems, Withdrawn/Depressed. All these scales can be combined in the Internalizing problems and the Externalizing problem scores which can be summarized in turn in the Total problem score. The Diagnostic and Statistical Manual of Mental Disorders (DSM) oriented scales were also considered: Affective Problems, Anxiety Problems, Somatic Problems, ADHD Problems, Oppositional Defiant Problems, Conduct Problems. The Social Responsiveness Scale (SRS)58 was administered to measure autistic traits as observed by the main teacher and by the mother through a 65 questions survey. For both CBCL and SRS scores a lower value means fewer behavioral problems.
Psychological assessment, child performance
The Wechsler Intelligence Scale for Children edition IV (WISC-IV)59 (the Italian version of the most recent WISC-V was not yet available) for children’s cognitive assessment was also taken by the participants.
Sociodemographic and lifestyle data
Sociodemographic data including age, sex, SES, area of residence and distance from the source were collected from participants through the administration of questionnaires to parents. Parental age, level of education, occupational level and work-related stress perception were considered to categorize each subject in three education and occupational levels (low, medium, high) through whose combination a SES variable with as many levels was built60. Distance from the source was measured as the distance from the closest point of the polygon delimiting the industrial site responsible of the major amount of the emissions. Lifestyle information was also collected like exposure to passive smoking at home and cognitive stimulation via the Home Observation for Measurement of the Environment (HOME) questionnaire61.
Statistical analysis
Descriptive statistics were reported for all the variables of interest for the overall sample size and stratified by area of residence (Tamburi, Statte, Paolo VI, Taranto, Talsano). Frequencies and proportions were used for categorical variables, median and interquartile range for continuous variables. Preliminary comparison between the study sub-sites was performed with non-parametric Kruskal–Wallis rank-sum test. The correlation among metal concentrations was measured through the Spearman correlation coefficient.
Biomarker statistics were reported as in Lucchini et al.38: median, 1st and 3rd quartiles were shown alongside the linear regression coefficient and 95% confidence intervals (CI) related to the association between the distance to the emission source and the biomarkers after having log-transformed the metal concentrations to obtain a normal distribution and after having applied cubic splines to the distance from the source to asses a linear trend.
The homogeneity between parent and teacher scores was assessed through the intraclass correlation coefficient (ICC). After checking for skewness and kurtosis of each neurobehavioral outcome, the association between exposure to multiple elements and the combined syndrome scales (internalizing, externalizing and total problems) and SRS scores was tested through a linear mixed effects model allowing to consider both parent and teacher scores with participants, school and area of residence considered as random effects with a nested structure. A censored regression62 was applied to the lower level empirically-based syndrome and the DSM oriented scales because of the censored and skewed data (the score is included between 50 and 100 and there was a mass point at the lower limit). Robust estimates of the standard errors clustered by subject were considered allowing to include both scores obtained from the questionnaire filled out by parents and teachers. For the censored regression we reported the marginal effect (the effects on the actual bounded dependent variables) at the mean value of the covariates since significance did not change both for lower or higher values. Multicollinearity was assessed in multivariable models through the variance inflation factor (VIF) while semipartial correlation was estimated to assess which variable explained the higher proportion of variance for each outcome. An interaction term between pairs of metal was introduced (as the product of the two considered metals) in the linear mixed effect models and the censored regressions. The marginal effect of each element at increasing level of the interacting chemical was shown for both models. In particular for the linear mixed effects model we reported the total effect of each metal on the considered outcome at different level of concentrations of the interacting element. In the case of the censored regression, since the parameter not only changes at different levels of the variable with which it interacts (due to the interaction term) but also with the variation of the variable with which it is associated (when considering the marginal effects), we represented the β parameters with their 95% CI as a dependent variable of the metal with which it was associated and at different levels of the interacting element.
We included in the analyses the values < LOD (Limit of Detection) (these were 1.6% of the results, only for UCd) to not loose statistical power.
All models were adjusted for sex, age, SES, distance from the exposure source, child IQ, exposure to passive smoking and the total HOME score as potential confounders. All statistical tests were two sided and assumed a 5% significance level after applying Hochberg adjusted p-values to account for multiple testing for Kruskal Wallis test, while Tukey adjustment was considered for marginal effects. All analyses were performed with R 4.0.263 and Stata 1564.
Ethics approval and consent to participate
This study obtained the approval of the Ethical Committee of Local Public Health (ASL, Azienda Sanitaria Locale) of Brindisi, with the jurisdiction on Taranto province.
Data availability
The datasets generated during and analysed during the current study are available from the corresponding author on reasonable request.
Abbreviations
- ADHD:
-
Attention Deficit Hyperactivity Disorder
- CDC:
-
Centers for Disease Control and Prevention
- Pb:
-
Lead
- Hg:
-
Mercury
- Cd:
-
Cadmium
- Mn:
-
Manganese
- As:
-
Arsenic
- Se:
-
Selenium
- HR-ICP-MS:
-
High resolution-inductively coupled plasma mass spectrometry
- BPb:
-
Blood lead
- BSe:
-
Blood selenium
- UAs:
-
Urine arsenic
- UCd:
-
Urine cadmium
- HMn:
-
Hair manganese
- HHg:
-
Hair mercury
- TDA-AAS:
-
Thermal decomposition amalgamation atomic absorption spectrometry
- CBCL:
-
Child Behavior Checklist
- DSM:
-
Statistical Manual of Mental Disorders
- SRS:
-
Social Responsiveness Scale
- WISC-IV:
-
Wechsler Intelligence Scale for Children edition IV
- SES:
-
Socio-economic status
- CI:
-
Confidence intervals
- LOD:
-
Limit of detection
- EPA:
-
Environmental Protection Agency’s
References
Bloom, B., Cohen, R. A. & Freeman, G. Summary health statistics for U.S. children: National Health Interview Survey, 2009. Vital Health Stat. 10, 1–82 (2010).
Grandjean, P. & Landrigan, P. J. Neurobehavioural effects of developmental toxicity. Lancet Neurol. 13, 330–338. https://doi.org/10.1016/S1474-4422(13)70278-3 (2014).
Zablotsky, B. et al. Prevalence and trends of developmental disabilities among children in the United States: 2009–2017. Pediatrics https://doi.org/10.1542/peds.2019-0811 (2019).
Donfrancesco, R. et al. Prevalence of severe ADHD: An epidemiological study in the Italian regions of Tuscany and Latium. Epidemiol. Psychiatr. Sci. 24, 525–533. https://doi.org/10.1017/S2045796014000523 (2015).
Christensen, D. L. et al. Prevalence and characteristics of autism spectrum disorder among children aged 8 years—Autism and developmental disabilities monitoring network, 11 sites, United States, 2012. MMWR Surveill. Summ. 65, 1–23. https://doi.org/10.15585/mmwr.ss6503a1 (2016).
CDC. Data & Statistics (Center for Disease Control and Prevention, US Department of Health & Human Services, 2016).
Toxicology, N. R. C. C. O. D. Scientific Frontiers in Developmental Toxicology and Risk Assessment (National Academies Press, Berlin, 2000).
Landrigan, P. J., Lambertini, L. & Birnbaum, L. S. A research strategy to discover the environmental causes of autism and neurodevelopmental disabilities. Environ. Health Perspect. 120, a258–a260. https://doi.org/10.1289/ehp.1104285 (2012).
Nevison, C. D. A comparison of temporal trends in United States autism prevalence to trends in suspected environmental factors. Environ. Health 13, 73. https://doi.org/10.1186/1476-069X-13-73 (2014).
Gundacker, C. et al. Genetics of the human placenta: Implications for toxicokinetics. Arch. Toxicol. 90, 2563–2581. https://doi.org/10.1007/s00204-016-1816-6 (2016).
Gil, F. & Pla, A. Biomarkers as biological indicators of xenobiotic exposure. J. Appl. Toxicol. 21, 245–255 (2001).
Ruggieri, F., Majorani, C., Domanico, F. & Alimonti, A. Mercury in children: Current state on exposure through human biomonitoring studies. Int. J. Environ. Res. Public Health. https://doi.org/10.3390/ijerph14050519 (2017).
Amoros, R. et al. Selenium status during pregnancy: Influential factors and effects on neuropsychological development among Spanish infants. Sci. Total Environ. 610–611, 741–749. https://doi.org/10.1016/j.scitotenv.2017.08.042 (2017).
Vinceti, M. et al. Selenium neurotoxicity in humans: Bridging laboratory and epidemiologic studies. Toxicol. Lett. 230, 295–303. https://doi.org/10.1016/j.toxlet.2013.11.016 (2014).
Farias, A. C. et al. Manganese in children with attention-deficit/hyperactivity disorder: Relationship with methylphenidate exposure. J. Child Adolesc. Psychopharmacol. 20, 113–118. https://doi.org/10.1089/cap.2009.0073 (2010).
Bhang, S. Y. et al. Relationship between blood manganese levels and children’s attention, cognition, behavior, and academic performance—A nationwide cross-sectional study. Environ. Res. 126, 9–16. https://doi.org/10.1016/j.envres.2013.05.006 (2013).
Nigg, J. T., Nikolas, M., Mark Knottnerus, G., Cavanagh, K. & Friderici, K. Confirmation and extension of association of blood lead with attention-deficit/hyperactivity disorder (ADHD) and ADHD symptom domains at population-typical exposure levels. J. Child Psychol. Psychiatry 51, 58–65. https://doi.org/10.1111/j.1469-7610.2009.02135.x (2010).
Nicolescu, R. et al. Environmental exposure to lead, but not other neurotoxic metals, relates to core elements of ADHD in Romanian children: Performance and questionnaire data. Environ. Res. 110, 476–483. https://doi.org/10.1016/j.envres.2010.04.002 (2010).
Braun, J. M. et al. Association of environmental toxicants and conduct disorder in US children: NHANES 2001–2004. Environ. Health Perspect. 116, 956–962. https://doi.org/10.1289/ehp.11177 (2008).
Gump, B. B. et al. Background lead and mercury exposures: Psychological and behavioral problems in children. Environ. Res. 158, 576–582. https://doi.org/10.1016/j.envres.2017.06.033 (2017).
Horton, M. K. et al. Dentine biomarkers of prenatal and early childhood exposure to manganese, zinc and lead and childhood behavior. Environ. Int. 121, 148–158. https://doi.org/10.1016/j.envint.2018.08.045 (2018).
Roy, A. et al. Association between arsenic exposure and behavior among first-graders from Torreón, Mexico. Environ. Res. 111, 670–676. https://doi.org/10.1016/j.envres.2011.03.003 (2011).
Arbuckle, T. E., Davis, K., Boylan, K., Fisher, M. & Fu, J. Bisphenol A, phthalates and lead and learning and behavioral problems in Canadian children 6–11 years of age: CHMS 2007–2009. Neurotoxicology 54, 89–98. https://doi.org/10.1016/j.neuro.2016.03.014 (2016).
Barg, G. et al. Association of low lead levels with behavioral problems and executive function deficits in schoolers from Montevideo, Uruguay. Int. J. Environ. Res. Public Health. https://doi.org/10.3390/ijerph15122735 (2018).
Roy, A. et al. Lead exposure and behavior among young children in Chennai, India. Environ. Health Perspect. 117, 1607–1611. https://doi.org/10.1289/ehp.0900625 (2009).
Khan, K. et al. Manganese exposure from drinking water and children’s academic achievement. Neurotoxicology 33, 91–97. https://doi.org/10.1016/j.neuro.2011.12.002 (2012).
Sanders, A. P., Claus Henn, B. & Wright, R. O. Perinatal and childhood exposure to cadmium, manganese, and metal mixtures and effects on cognition and behavior: A review of recent literature. Curr. Environ. Health Rep. 2, 284–294. https://doi.org/10.1007/s40572-015-0058-8 (2015).
von Stackelberg, K., Guzy, E., Chu, T. & Claus Henn, B. Exposure to mixtures of metals and neurodevelopmental outcomes: A multidisciplinary review using an adverse outcome pathway framework. Risk Anal. 35, 971–1016. https://doi.org/10.1111/risa.12425 (2015).
Dickerson, A. S. et al. Autism spectrum disorder prevalence and associations with air concentrations of lead, mercury, and arsenic. Environ. Monit. Assess. 188, 407. https://doi.org/10.1007/s10661-016-5405-1 (2016).
Doherty, B. T. et al. Periconceptional and prenatal exposure to metal mixtures in relation to behavioral development at 3 years of age. Environ. Epidemiol. 4, e0106. https://doi.org/10.1097/EE9.0000000000000106 (2020).
Karri, V., Schuhmacher, M. & Kumar, V. Heavy metals (Pb, Cd, As and MeHg) as risk factors for cognitive dysfunction: A general review of metal mixture mechanism in brain. Environ. Toxicol. Pharmacol. 48, 203–213. https://doi.org/10.1016/j.etap.2016.09.016 (2016).
Czarnota, J., Gennings, C. & Wheeler, D. C. Assessment of weighted quantile sum regression for modeling chemical mixtures and cancer risk. Cancer Inf. 14, 159–171. https://doi.org/10.4137/CIN.S17295 (2015).
Bobb, J. F. et al. Bayesian kernel machine regression for estimating the health effects of multi-pollutant mixtures. Biostatistics 16, 493–508. https://doi.org/10.1093/biostatistics/kxu058 (2015).
Ramírez Ortega, D. et al. Cognitive impairment induced by lead exposure during lifespan: mechanisms of lead neurotoxicity. Toxics. https://doi.org/10.3390/toxics9020023 (2021).
Glass, T. A. et al. Neighborhood psychosocial hazards and the association of cumulative lead dose with cognitive function in older adults. Am. J. Epidemiol. 169, 683–692. https://doi.org/10.1093/aje/kwn390 (2009).
Pottier, G. et al. Lead exposure induces telomere instability in human cells. PLoS ONE 8, e67501. https://doi.org/10.1371/journal.pone.0067501 (2013).
Kasperczyk, S., Dobrakowski, M., Kasperczyk, A., Ostałowska, A. & Birkner, E. The administration of N-acetylcysteine reduces oxidative stress and regulates glutathione metabolism in the blood cells of workers exposed to lead. Clin. Toxicol. (Phila) 51, 480–486. https://doi.org/10.3109/15563650.2013.802797 (2013).
Lucchini, R. G. et al. Neurocognitive impact of metal exposure and social stressors among schoolchildren in Taranto, Italy. Environ. Health 18, 67. https://doi.org/10.1186/s12940-019-0505-3 (2019).
Schulz, C., Angerer, J., Ewers, U., Heudorf, U. & Wilhelm, M. Revised and new reference values for environmental pollutants in urine or blood of children in Germany derived from the German environmental survey on children 2003–2006 (GerES IV). Int. J. Hyg. Environ. Health 212, 637–647. https://doi.org/10.1016/j.ijheh.2009.05.003 (2009).
NHANES. Updated Tables. Fourth National Report on Human Exposure to Environmental Chemicals: CDC National Health and Nutrition Examination Survey (2017).
Davis, M. A. et al. Rice consumption and urinary arsenic concentrations in U.S. children. Environ. Health Perspect. 120, 1418–1424. https://doi.org/10.1289/ehp.1205014 (2012).
Riederer, A. M., Belova, A., George, B. J. & Anastas, P. T. Urinary cadmium in the 1999–2008 U.S. National Health and Nutrition Examination Survey (NHANES). Environ. Sci. Technol. 47, 1137–1147. https://doi.org/10.1021/es303556n (2013).
Carneiro, M. F. et al. Assessment of trace elements in scalp hair of a young urban population in Brazil. Biol. Trace Elem. Res. 143, 815–824. https://doi.org/10.1007/s12011-010-8947-z (2011).
Benes, B., Sladká, J., Spevácková, V. & Smid, J. Determination of normal concentration levels of Cd, Cr, Cu, Hg, Pb, Se and Zn in hair of the child population in the Czech Republic. Cent. Eur. J. Public Health 11, 184–186 (2003).
Smolders, R. et al. Interpreting biomarker data from the COPHES/DEMOCOPHES twin projects: Using external exposure data to understand biomarker differences among countries. Environ. Res. 141, 86–95. https://doi.org/10.1016/j.envres.2014.08.016 (2015).
Molina-Villalba, I. et al. Biomonitoring of arsenic, cadmium, lead, manganese and mercury in urine and hair of children living near mining and industrial areas. Chemosphere 124, 83–91. https://doi.org/10.1016/j.chemosphere.2014.11.016 (2015).
Fréry, N., Vandentorren, S., Etchevers, A. & Fillol, C. Highlights of recent studies and future plans for the French human biomonitoring (HBM) programme. Int. J. Hyg. Environ. Health 215, 127–132. https://doi.org/10.1016/j.ijheh.2011.08.008 (2012).
Pino, A. et al. Human biomonitoring data analysis for metals in an Italian adolescents cohort: An exposome approach. Environ. Res. 159, 344–354. https://doi.org/10.1016/j.envres.2017.08.012 (2017).
Tatsuta, N. et al. Total mercury levels in hair of children aged 7years before and after the Great East Japan Earthquake. Sci. Total Environ. 596–597, 207–211. https://doi.org/10.1016/j.scitotenv.2017.04.110 (2017).
Lucchini, R. G. et al. Inverse association of intellectual function with very low blood lead but not with manganese exposure in Italian adolescents. Environ. Res. 118, 65–71. https://doi.org/10.1016/j.envres.2012.08.003 (2012).
Pino, A., Amato, A., Alimonti, A., Mattei, D. & Bocca, B. Human biomonitoring for metals in Italian urban adolescents: Data from Latium Region. Int. J. Hyg. Environ Health 215, 185–190. https://doi.org/10.1016/j.ijheh.2011.07.015 (2012).
Eastman, R. R., Jursa, T. P., Benedetti, C., Lucchini, R. G. & Smith, D. R. Hair as a biomarker of environmental manganese exposure. Environ. Sci. Technol. 47, 1629–1637. https://doi.org/10.1021/es3035297 (2013).
Bansal, E. et al. Metabolic outcomes in southern italian preadolescents residing near an industrial complex: The role of residential location and socioeconomic status. Int. J. Environ. Res. Public Health. https://doi.org/10.3390/ijerph16112036 (2019).
Ruggieri, F., Alimonti, A. & Bocca, B. Full validation and accreditation of a method to support human biomonitoring studies for trace and ultra-trace elements. TrAC, Trends Anal. Chem. 80, 471–485 (2016).
Alimonti, A., Bocca, B. & Ruggieri, F. Reference method for the determination of chemical elements in human biological matrices: analytical performances and uncertainty of data (2015).
Domanico, F. et al. Determination of mercury in hair: Comparison between gold amalgamation-atomic absorption spectrometry and mass spectrometry. J. Trace Elem. Med. Biol. 43, 3–8. https://doi.org/10.1016/j.jtemb.2016.09.008 (2017).
Achenbach, T. Manual for the Child Behavior Checklist/4–18 and 1991 Profile (University of Vermont, 1991).
Constantino, J. N. & Gruber, C. P. Social Responsiveness Scale (SRS) (Western Psychological Services, 2005).
Wechsler, D. Wechsler Intelligence Scale for Children - Fourth Edition (WISC-IV) (Pearson, 2003).
Cesana, G. C., de Vito, G., Ferrario, M., Sega, R. & Mocarelli, P. Trends of smoking habits in northern Italy (1986–1990). The WHO MONICA Project in Area Brianza, Italy. MONICA Area Brianza Research Group. Eur. J. Epidemiol. 11, 251–258 (1995).
Bradley, R. H., Caldwell, B. M., Rock, S. L., Hamrick, H. M. & Harris, P. Home observation for measurement of the environment: Development of a home inventory for use with families having children 6 to 10 years old. Contemp. Educ. Psychol. 13, 58–71. https://doi.org/10.1016/0361-476X(88)90006-9 (1988).
Tobin, J. Estimation of relationships for limited dependent variables. Econometrica 26, 24–36 (1958).
Team, R. D. C. (R Foundation for Statistical Computing, Vienna, Austria, 2013).
Stata Statistical Software. Release 15 (StataCorp LLC, 2017).
Acknowledgements
We would like to thank all the participating children and their families, the teachers and school authorities of Taranto, for their outstanding contribution to this study.
Funding
The study presented herein was performed with the support of the project funded by the Italian Ministry of Health: CCM 2013 “Biomonitoring and toxicity of pollutants in the territory of Taranto, Italy”. It was also funded by the National Institute of Environmental Health Sciences (NIH/NIEHS) [R01ES019222, R56ES019222] and Università degli Studi di Milano [UNBSCLE 9015].
Author information
Authors and Affiliations
Contributions
S.R.; manuscript drafting, data analysis and interpretation. G.C.; data acquisition, data analysis and interpretation. S.C.; data analysis and interpretation. M.C.; study design and coordination. C.F., G.F., A.G., C.M., M.O., M.P., F.P., A.P., O.S. and S.Z.; data acquisition. S.G.; study design and coordination, data analysis and interpretation. A.P.; data acquisition. D.P.; data analysis and interpretation. A.A.; study design and coordination. R.G.L.; study design and coordination, data analysis and interpretation. All authors have read and approved the final manuscript.
Corresponding author
Ethics declarations
Competing interests
The authors declare no competing interests.
Additional information
Publisher's note
Springer Nature remains neutral with regard to jurisdictional claims in published maps and institutional affiliations.
Supplementary Information
Rights and permissions
Open Access This article is licensed under a Creative Commons Attribution 4.0 International License, which permits use, sharing, adaptation, distribution and reproduction in any medium or format, as long as you give appropriate credit to the original author(s) and the source, provide a link to the Creative Commons licence, and indicate if changes were made. The images or other third party material in this article are included in the article's Creative Commons licence, unless indicated otherwise in a credit line to the material. If material is not included in the article's Creative Commons licence and your intended use is not permitted by statutory regulation or exceeds the permitted use, you will need to obtain permission directly from the copyright holder. To view a copy of this licence, visit http://creativecommons.org/licenses/by/4.0/.
About this article
Cite this article
Renzetti, S., Cagna, G., Calza, S. et al. The effects of the exposure to neurotoxic elements on Italian schoolchildren behavior. Sci Rep 11, 9898 (2021). https://doi.org/10.1038/s41598-021-88969-z
Received:
Accepted:
Published:
DOI: https://doi.org/10.1038/s41598-021-88969-z
This article is cited by
-
Early childhood exposure to environmental phenols and parabens, phthalates, organophosphate pesticides, and trace elements in association with attention deficit hyperactivity disorder (ADHD) symptoms in the CHARGE study
Environmental Health (2024)
-
Natural radioisotopes and chemical elements causing carcinogenic risks in soils of urban green spaces in the Metropolitan Region of Recife, Pernambuco, Brazil?
Journal of Radioanalytical and Nuclear Chemistry (2023)
-
Ameliorative effect of Brassica oleracea var. Italica extract on oxidative damage of arsenic in the rat’s brain: biochemical, pathological, and behavioral aspects
Metabolic Brain Disease (2023)
-
Biomonitoring of urinary metals in athletes according to particulate matter air pollution before and after exercise
Environmental Science and Pollution Research (2022)
Comments
By submitting a comment you agree to abide by our Terms and Community Guidelines. If you find something abusive or that does not comply with our terms or guidelines please flag it as inappropriate.