Abstract
Environmental and community factors may influence the development or course of depression and sleep problems. We evaluated the association of unconventional natural gas development (UNGD) with depression symptoms and disordered sleep diagnoses using the Patient Health Questionnaire-8 and electronic health record data among Geisinger adult primary care patients in Pennsylvania. Participants received a retrospective metric for UNGD at their residence (very low, low, medium, and high) that incorporated dates and durations of well development, distance from patient homes to wells, and well characteristics. Analyses included 4,762 participants with no (62%), mild (23%), moderate (10%), and moderately severe or severe (5%) depression symptoms in 2014–2015 and 3,868 disordered sleep diagnoses between 2009–2015. We observed associations between living closer to more and bigger wells and depression symptoms, but not disordered sleep diagnoses in models weighted to account for sampling design and participation. High UNGD (vs. very low) was associated with depression symptoms in an adjusted negative binomial model (exponentiated coefficient = 1.18, 95% confidence interval [CI]: 1.04–1.34). High and low UNGD (vs. very low) were associated with depression symptoms (vs. none) in an adjusted multinomial logistic model. Our findings suggest that UNGD may be associated with adverse mental health in Pennsylvania.
Similar content being viewed by others
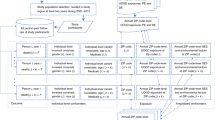
Introduction
Unconventional natural gas development (UNGD) is a long-lasting industrial process with potential environmental and social impacts, including noise, light, vibration, truck traffic, air, water, and soil pollution, social disruption, crime, and stress and anxiety related to these features as well as rapid industrial development1,2,3. UNGD involves pad preparation, drilling, stimulation (“fracking”), and production1. Operators in Pennsylvania had drilled 9,669 wells in the Marcellus shale by the end of 20154 and Pennsylvania led the country in shale gas production in 20165.
Growth in energy production has resulted in both local economic benefits and concern about potential health consequences. Economists have reported inconsistent effects on property values6 and increased employment and increased wages in counties with UNGD7,8, but the permanency of these benefits remains uncertain9,10. Public health researchers have found associations between UNGD and adverse birth outcomes11,12,13,14, asthma exacerbations15, and self-reported health problems or symptoms16,17,18, all outcomes with environmental and social risk factors. No prior epidemiologic study, however, has considered clinically-diagnosed sleep problems or a mental health outcome measured via a validated scale19. We considered these outcomes as important to evaluate in relation to UNGD given the biologically plausible relationship with UNGD and the significant societal costs of these outcomes. Major depressive disorder cost $210.5 billion and accounted for 3.7% of total U.S. disability-adjusted life years in 201020,21, and over one-third of U.S. adults did not meet recommended sleep durations in 201422.
Evidence suggests that depression and sleep problems may co-occur23,24 and that UNGD could influence these outcomes via several pathways (Fig. 1). Individuals living near UNGD have reported reduced life satisfaction, feelings of disempowerment, social stress, negative psychological states, and disruption in sense of place (i.e., meaning and attachments that residents have for their community)25,26,27,28,29,30,31. A growing body of evidence also links particulate air pollution, an environmental hazard associated with UNGD, to depression and anxiety32,33. Further, nighttime noise and light pollution can disrupt sleep, with potential consequences for mental health34,35,36,37.
Hypothesized relationships between unconventional natural gas development (UNGD), associated physical and psychological exposures, disordered sleep and depression symptoms measured via the eight-item Patient Health Questionnaire-8 (PHQ-8) depression scale. The solid rectangle identifies factors measured in this study and the dashed rectangle identifies unmeasured factors.
Here, we evaluated the association of UNGD in the Marcellus shale in Pennsylvania with depression symptoms and disordered sleep diagnoses, measured via questionnaire and electronic health record (EHR) data, respectively. We also explored effect modification of the UNGD-depression symptoms association by antidepressant medication use under the hypothesis that those not receiving treatment may respond more strongly to UNGD exposure.
Results
Of the 4,932 subjects in the study, 170 did not answer any PHQ-8 questions, 2,976 had no significant depression symptoms, 1,075 had mild depression symptoms, 454 had moderate depression symptoms, and 257 had moderately severe/severe depression symptoms in 2014–2015 (Table 1). Participants with more severe depression symptoms, compared to those with no or less severe symptoms, were more likely to be female, on Medical Assistance, take antidepressants, and have heavy alcohol use (all p < 0.01). We identified 8,578 disordered sleep diagnoses between January 2009 and June 2015 using EHR data among 1,699 of the 4,932 study subjects. The remaining study subjects did not have disordered sleep diagnoses using our criteria. After randomly selecting one disordered sleep diagnosis per person per year, we included 3,868 disordered sleep diagnoses over 6 years. Participants with at least one disordered sleep diagnosis, compared to those with none, were more likely to be female and to be older (both p < 0.05).
By December 31, 2014, companies had drilled 8807 unconventional wells in the Pennsylvania Marcellus shale4. In unadjusted truncated-weighted multinomial logistic regression models, the high and low groups of the UNGD activity index (vs. very low) were associated with increased odds of mild depression symptoms (vs. none, odds ratios [95% confidence interval] = 1.49 [1.11–1.99] and 1.52 [1.13–2.04], respectively). We also observed associations between the high and low groups of the UNGD activity index (vs. very low) and mild depression symptoms (vs. none) in an adjusted multinomial logistic model (Table 2) and the number of depression symptoms (continuous variable) in an adjusted negative binomial model (Table 3). There was no significant association between the medium UNGD activity group (vs. very low) and depression symptoms in either model. In the adjusted multilevel model for the longitudinal disordered sleep outcome, UNGD was not associated with disordered sleep diagnoses between 2009–2015 (Table 4) or in analyses restricted to diagnoses in 2014–2015 (see Supplementary Table S3). We also observed no association between UNGD and encounters that resulted in both a disordered sleep diagnosis and sleep-related medication order (see Supplementary Table S4).
When we added a cross-product between UNGD and antidepressant medication use to the model we found no evidence of multiplicative interaction, the p-value from the Wald test of the cross-product was 0.14 and 0.12 in the multinomial logistic and negative binomial models, respectively. In the sensitivity analysis to evaluate the influence of weighting on associations, we observed stronger associations between UNGD and depression symptoms using full weights, and no association between UNGD and depression symptoms using no weights (Tables S5 and S6). Additionally, results did not change when we limited the multinomial logistic models to participants that completed all 8 questions on the PHQ-8 (Table S7).
Discussion
In this study of nearly 5,000 adults in Pennsylvania in 2015, we observed an association between living closer to more and bigger UNGD wells and more depression symptoms as measured by the PHQ-8. Antidepressant use did not appear to act as an effect modifier. We found no evidence of an association between UNGD and disordered sleep diagnoses. To our knowledge, this study represents the first to evaluate associations between UNGD and mental health and sleep using a validated instrument and clinical diagnoses.
Our results align with prior qualitative studies finding mental distress among members of UNGD communities19. For example, in an analysis of letters to the editor about UNGD in a newspaper in Pennsylvania, stress was a major theme2, and a sample of people living near UNGD reported stress as their most common symptom38. In Texas, Maguire and colleagues used county-level Behavioral Risk Factor Surveillance System data and found an association between UNGD and reduced life satisfaction among women and an increased number of poor mental health days among both sexes26. Perceived changes in quality of life30,39,40, health effects29, or resource loss25, as well as feelings of disempowerment31,41, a disrupted sense of place25,27, and a loss of community cohesion10,25 could potentially explain our observed association between UNGD activity and depression symptoms.
In a previous study, we found an association between UNGD and nasal and sinus, migraine, and fatigue symptoms18. These outcomes may co-occur or lie on the pathway between UNGD and mental health outcomes and sleep disturbances42,43. In addition, the findings could have relevance to our prior reported associations of UNGD with asthma exacerbations15; as stress could be a plausible mediator between both UNGD and depression symptoms and UNGD and asthma exacerbations44,45.
UNGD could also influence mental health via an air, noise, or light pollution pathway. Our model incorporated all phases of UNGD (from well pad development to production), each of which releases air pollutants from truck traffic, diesel powered machinery, and fugitive emissions1. Short- and long-term exposure to air pollution has been associated with depression symptoms. For example, in a study in Korea that evaluated long-term exposure, a 10 µg/m3 increase in PM2.5 over the prior year was associated with 1.47 times the risk of a diagnosis of major depression disorder33. Air pollution may increase pro-inflammatory markers in the blood46 and overall oxidative stress47, both factors previously associated with depression48,49. Nighttime noise and light can affect sleep duration and quality, which in turn could lead to adverse mental health outcomes34,35,36,37. For example, Orban et al. reported an association between 24-hour traffic noise and high depressive symptoms, an association that was stronger among participants reporting insomnia at baseline50.
More extreme environmental exposures, like those resulting from disasters, have been associated with adverse mental health outcomes51. Telephone interviews after the Deepwater Horizon oil spill revealed increased depressive symptoms and mental distress among women with physical exposure to the event52. Oil and gas wastewater injection into class II injection wells in Oklahoma has triggered felt earthquakes over the past decade53. A recent study reported an association between earthquakes with magnitude greater than four and increased Google search episodes for anxiety54. UNGD, a human-made industry with several resulting individual and community exposures in Pennsylvania, could perhaps result in a similar mental health impacts.
This study had several strengths, in particular its large sample size. It assessed depression symptoms with a validated questionnaire and scale, a strength because EHR data may not capture depression and its symptoms well55. In addition, prior population-based studies have reported high sensitivity and specificity using PHQ-8 scores ≥ 10 to identify individuals with major depression56,57. A small portion of participants did not answer all PHQ-8 questions, but we observed no difference in results when stratifying analyses by participants with complete and incomplete questionnaires. Our study questionnaire did not mention UNGD, which should have reduced the possibility of over-reporting of symptoms among participants around higher UNGD activity (i.e., same source bias). Additionally, the UNGD metric captured the time-varying nature of well development and incorporated distance to multiple wells and size and phase of wells in the activity metric. The metric, however, did not allow us to determine which, if any, of the hypothesized pathways in Fig. 1 may account for the association between UNGD and depression symptoms.
This study had additional limitations. Exposure assignments likely included some misclassification for two reasons. First, we used patient address at the time of questionnaire mailing to assign the UNGD activity metric. Previous work has shown, however, that the Geisinger population exhibits residential stability with just 4% of the population moving >16 km from their original address over a 3-year period11. Second, we succeeded in geocoding at the address-level for 89.1% of the sample. Associations did not change appreciably when we restricted analyses to this population.
Responders tended to be sicker than the general population because the original survey was designed to oversample patients with nasal and sinus symptoms18,58. This could limit the generalizability of our results as sicker individuals may represent vulnerable populations who might more readily develop UNGD-related depression or sleep problems59 or exhibit a stronger response to psychosocial stressors60, air61 or noise pollution62. Further, individual factors including age, race/ethnicity, sex, and underlying health may affect survey response63. While we used survey weights to account for the survey design and non-response, differences may still have existed between the weighted population and the source population. A previous study of pediatric patients suggested that the use of International Classification of Diseases, 9th Revision (ICD-9) codes to identify sleep disorders likely underestimates the true prevalence64. Our use of ICD-9 codes and medication orders to identify these conditions likely also led to under-ascertainment. Participants may have treated disordered sleep over-the-counter; only a portion of individuals with sleep problems seek medical attention and only a subset of care-seekers receives a diagnosis or medication65. Future studies could consider identifying disordered sleep in clinical notes or by questionnaire66. We lacked biologic measures of stress (e.g., cortisol) as well as information on survey responders’ attitudes about their community generally or about UNGD specifically, factors that could have influenced their psychological and physical response to the development25,27,67. We did not know if survey responders had signed a lease with a drilling company. Leaseholders receive economic benefit from UNGD, making them more supportive of UNGD and possibly less likely to experience adverse psychological outcomes as a result68. Lastly, we did not make air quality, light, vibration, traffic, or noise level measurements, so cannot evaluate which, if any, environmental hazards were present at higher levels in the highest UNGD activity group.
In conclusion, we combined information from a mailed questionnaire, EHR data, and a time-varying measure of residential proximity to more and bigger UNGD wells to conduct the most comprehensive study to date on the potential mental health and sleep consequences of UNGD. We found an association between UNGD and depression symptoms but not with disordered sleep diagnoses. Individuals require access to mental health services with clinicians trained to screen, monitor, and treat psychological problems among populations potentially affected by UNGD19,69. At the same time, further research is required to disentangle the multifactorial pathways through which UNGD may influence mental health. Our findings should be interpreted in the context of prior reports of associations of UNGD with other health outcomes and suggest the need to incorporate potential mental health consequences of UNGD in risk-benefit calculations.
Methods
Study area
We conducted this study among Geisinger’s adult patients58, located in over 40 counties in central and northeast Pennsylvania in a region with a range of UNGD activity. Geisinger’s primary care population is representative of the general population of the region based on distributions of age, sex, and race/ethnicity11. Geisinger has had a fully-operational EHR installed since 2005. All Geisinger patients had the option to opt out of all research, but less than 0.1% did so at the time of the study. Those that did not opt out were informed that their EHR data could be used for research.
Survey design and study population
Our study population came from a cohort originally designed to study the epidemiology of chronic rhinosinusitis and related nasal and sinus symptoms18,58. The survey design oversampled racial/ethnic minorities and people more likely to have nasal and sinus symptoms. In April 2014, a baseline questionnaire, a cover letter that explained the study, and a $1 bill as incentive was sent to 23,700 adults 18 years of age and older. The cover letter explained that study participation was voluntary and that if participants did not return the questionnaire they would not be included; by returning the questionnaire participants provided informed consent. Of the 23,700 letters sent, 7,847 participants responded (response rate = 33.1%)58. Six months later, a follow-up questionnaire, which included the eight-item Patient Health Questionnaire-8 (PHQ-8), was sent to all responders of the baseline questionnaire, of whom 4,966 responded (secondary response rate = 63.3%). Follow-up questionnaires were received from November 2014 to May 2015 (median of November 12, 2014). Using previously described methods70, we geocoded study subjects to their residential address listed in the EHR, 89.1% to street address, 3.1% to ZIP + 4, and 7.7% to ZIP code centroid. After excluding respondents living outside Pennsylvania (n = 34), the analysis consisted of 4,932 participants. All study protocols were reviewed and carried out in accordance with guidelines approved by the Geisinger Institutional Review Board.
Outcome ascertainment
Depression symptoms
The PHQ-8 asked individuals to report symptoms in the prior two weeks, for example, “how often have you been bothered by feeling down, depressed, hopeless?”56. Each question on the PHQ-8 has response options: “not at all”, “several days”, “more than half the days”, or “nearly every day”, scored as 0–3 respectively. Over 90% of participants answered all eight questions, for whom we calculated their total score by summing scores from the eight questions57. For participants who answered fewer than eight questions, we calculated their total score as a pro-rated sum using the formula: (sum of answered questions × 8)/(number of questions answered). Of participants who answered 1–7 questions, 81% answered 7 questions. We defined current depression symptoms using the total PHQ-8 score based on previously established categories56, but combined the two most severe groups because few participants had a “severe” total score. Scores were categorized into 0 to <5, no significant depression symptoms; 5 to <10, mild depression symptoms; 10 to <15, moderate depression symptoms; and 15 to 24, moderately severe/severe depression symptoms57. We excluded participants who did not answer any PHQ-8 questions (n = 170) from statistical analyses.
Disordered sleep diagnoses
Disordered sleep diagnoses (case-events) among the study population were identified in Geisinger’s EHR from January 2009 to June 2015. We identified encounters (98% outpatient) in the EHR that were accompanied by ICD-9 codes for disordered sleep (see Supplementary Table S1). We also identified orders for disordered sleep medications, using drug class “hypnotics” as well as drug subclass and name. We included all medications in the drug subclass antihistamine hypnotics, selective melatonin receptor agonists, hypnotics – tricyclic agents, and orexin receptor antagonists. In the subclass non-barbiturate hypnotics, we included all medications except midazolam hydrochloride, which is more often used for procedural sedation. We considered either an appropriate medication order or an encounter with a disordered sleep ICD-9 code as a disordered sleep outcome. We only retained disordered sleep diagnoses from when the participant was 18 years of age or older and randomly selected one disordered sleep diagnosis per participant per year so that study subjects with many encounters for sleep disorders would not unduly contribute (see Supplementary Figure S1).
For control dates, we identified all their dates of contact with the health system, excluded contact dates within one year of a disordered sleep diagnosis, and randomly selected one encounter date per year per participant. Control dates were frequency-matched to cases on age category (i.e., 18–44, 45–61, 62–74, 75+ years), sex, and year. We used encounter dates, rather than patients, to match as controls because of the time-varying nature of UNGD and many covariates.
Well data and activity metric assignment
We compiled well data from the Pennsylvania Department of Environmental Protection, the Pennsylvania Department of Conservation and Natural Resources, and SkyTruth, as described previously11,15,18. These data, collected for all unconventional natural gas wells in Pennsylvania from 2005–2015, included: latitude and longitude; dates of well pad construction, drilling, stimulation, and production; total well depth; and volume of natural gas produced biannually or annually.
We assigned UNGD activity for the four phases of well development (pad preparation, drilling, stimulation, and production) to each study subject (in the depression symptom analysis) or index date (in the disordered sleep analysis) using metrics that incorporated distances from participant residence to wells, and the density and size of wells, as in prior studies11,15,18. The metric has the potential to incorporate a variety UNGD-related hazards that exist on different temporal and spatial scales (e.g., regional air pollutants, local noise, truck traffic, activities that may lead to stress)71. We calculated the metric for each phase of well development:
where d was the date of return of the questionnaire, n was the number of wells in the given phase, mij2 was the squared-distance (meters) between well i and participant j, and si was 1 for the pad production and drilling phases, total well depth (meters) of well i for the stimulation phase, and daily natural gas production volume (m3) of well i for the production phase. For the depression symptom analysis, for each phase of development, the metric was summed for the 14 days prior (t = d − 14) to the date of the returned follow-up questionnaire (d). We chose 14 days prior to the survey return because the PHQ-8 ascertained depression symptoms over the past two weeks. For the disordered sleep analysis, we summed the UNGD activity for the three months prior to the date of the sleep disorder diagnosis (t = d − 90). In both analyses, we z-transformed the activity metrics for each of the four phases of development, summed the transformed values, and calculated quartiles of the sums to create a composite UNGD metric that represented very low, low, medium, and high residential proximity to more and bigger UNGD wells.
Covariates
Using the EHR and questionnaire, we created covariates for potential confounding variables: race/ethnicity; sex; Medical Assistance (a means-tested program used as a surrogate for family socioeconomic status)72; age at time of questionnaire return, disordered sleep diagnosis, or control date; smoking and alcohol use status; and body mass index. Antidepressant medication use in the month prior to survey return was ascertained with medication orders based on drug group (e.g., antidepressants), class (e.g., selective serotonin reuptake inhibitors [SSRIs]), sub-class, and name. We evaluated antidepressant use as an effect modifier; we hypothesized that antidepressant medication could attenuate associations of UNGD with depression symptoms. Time-varying-covariates (all but race/ethnicity and sex) were assigned before the date of questionnaire return (for the depression symptom analysis) or before the disordered sleep diagnosis or comparison date (for the disordered sleep analysis). Based on the participants’ geocoded coordinates, we assigned them to a community using a mixed definition of place (township, borough, or census tract in cities)70. For each community, we used the 2006–2010 American Community Survey to calculate community socioeconomic deprivation73. We used public water supplier service areas from the Pennsylvania Department of Environmental Protection to assign residential water source (municipal water or ground water)74. Patients residing in homes outside the public water supplier service area were assumed to use ground water.
Statistical analysis
We employed sampling weights to estimate unbiased measures of association while accounting for the survey stratified sampling design, the response rate to the baseline questionnaire, and loss to follow-up from the baseline to the follow-up questionnaires (see Supplementary Table S2). Because one weight was much larger than the others, we truncated the largest weight to the next largest for our primary analyses75.
To build models, we first included the UNGD variable representing residential proximity to more and bigger wells (quartiles: very low, low, medium, high), and then added potential confounding variables identified a priori: race/ethnicity (non-Hispanic White, non-Hispanic Black, Hispanic), sex (male, female), Medical Assistance (no, yes), age (years), smoking status (never, former, current), alcohol use status (heavy [based on the Centers for Disease Control definition of heavy drinking as 8 or more drinks per week for females and 15 or more drinks for males76]; vs. not heavy [which included no alcohol use]), body mass index from the EHR at the visit closest to questionnaire return (BMI, kg/m2), community socioeconomic deprivation, and water source (municipal water, well water). We centered the continuous covariates (age, BMI, and community socioeconomic deprivation) and included them as linear and quadratic terms to allow for non-linearity. We did not include community type (i.e., township, borough, or city) in final models because it may lie on the causal pathway between UNGD and sleep and mental health. We used a 2-sided type 1 error rate of 0.05 for significance testing and Stata version 11.2 (StataCorp Inc.) and R version 3.2.2 (R Foundation for Statistical Computing) for analyses.
We fit multinomial logistic models to estimate the association UNGD with each level of depression symptoms (mild, moderate, moderately severe/severe) compared to no depression symptoms (reference outcome). We also evaluated the association of UNGD with depression symptoms using negative binomial regression, which treated the PHQ-8 score as a continuous outcome, allowing us to evaluate associations between UNGD and the continuous burden of depression symptoms, rather than with the screening tool’s categories77. To assess the association of proximity to more and bigger UNGD wells with disordered sleep diagnoses, we fit a survey-weighted generalized estimating equations model, to account for multiple diagnoses within participants. Because sleep diagnoses spanned 2009–2015 but most UNGD began after 2010 and attitudes towards UNGD may have changed over time, in a secondary analysis we restricted the sleep analysis to diagnoses between January 2014 and June 2015. To test a definition with a higher positive predicative value for sleep disorder, we also ran a model where a case had to have both a diagnostic code for a sleep disorder and a sleep-related medication order.
We hypothesized reduced susceptibility to stressors like UNGD among participants taking antidepressants78,79. To test this, we evaluated effect modification by antidepressant use by including cross-products of the UNGD variables and antidepressant medication use to our final depression symptom models. We used a Wald test to evaluate the significance of the cross-products.
In a sensitivity analysis, we evaluated the influence of our sample weights by examining associations of UNGD with depression symptoms among all subjects using the final multinomial logistic and negative binomial models without weights and with full and truncated weights. In theory, weighted models will provide less precise, but more unbiased estimates than unweighted models80, our rationale for using truncated weighted models as the primary analysis.
Data Availability
Data on unconventional natural gas development in Pennsylvania are publicly available from the Pennsylvania Department of Environmental Protection, the Pennsylvania Department of Conservation and Natural Resources at http://www.dcnr.state.pa.us/topogeo/econresource/oilandgas/resrefs/wis_home/. The health data that were used in this study are protected health information and subject to many restrictions. Data may be available from the corresponding author upon reasonable request and with specific required agreements in place.
References
Adgate, J. L., Goldstein, B. D. & McKenzie, L. M. Potential public health hazards, exposures and health effects from unconventional natural gas development. Environ Sci Technol 48, 8307–8320, https://doi.org/10.1021/es404621d (2014).
Powers, M. et al. Popular epidemiology and “fracking”: citizens’ concerns regarding the economic, environmental, health and social impacts of unconventional natural gas drilling operations. J Community Health 40, 534–541 (2015).
James, A. & Smith, B. There will be blood: Crime rates in shale-rich US counties. J Environ Econ Manage 84, 125–152 (2017).
Pennsylvania Department of Environmental Protection. Oil and gas locations – unconventional, http://data-padep-1.opendata.arcgis.com/datasets?q=Oil%20&%20Gas.
Energy Information Agency. Natural Gas Gross Withdrawals and Production, https://www.eia.gov/dnav/ng/ng_prod_sum_a_EPG0_FGS_mmcf_a.htm (2018).
Muehlenbachs, L., Spiller, E. & Timmins, C. The housing market impacts of shale gas development. Am Econ Rev 105, 3633–3659 (2015).
Feyrer, J., Mansur, E. T. & Sacerdote, B. Geographic dispersion of economic shocks: Evidence from the fracking revolution. Am Econ Rev 107, 1313–1334 (2017).
Maniloff, P. & Mastromonaco, R. The local employment impacts of fracking: A national study. Resour Energy Econ 49, 62–85, https://doi.org/10.1016/j.reseneeco.2017.04.005 (2017).
Jacobsen, G. D. & Parker, D. P. The economic aftermath of resource booms: evidence from boomtowns in the American West. Econ J 126, 1092–1128 (2016).
Jacquet, J. & Kay, D. L. The Unconventional Boomtown: Updating the impact model to fit new spatial and temporal scales. J Rural Stud 9 (2014).
Casey, J. A. et al. Unconventional natural gas development and birth outcomes in Pennsylvania, USA. Epidemiology 27, 163–172, https://doi.org/10.1097/EDE.0000000000000387 (2016).
Currie, J., Greenstone, M. & Meckel, K. Hydraulic fracturing and infant health: New evidence from Pennsylvania. Sci Adv 3, e1603021 (2017).
McKenzie, L. M. et al. Birth outcomes and maternal residential proximity to natural gas development in rural Colorado. Environ Health Perspect 122, 412–417, https://doi.org/10.1289/ehp.1306722 (2014).
Whitworth, K. W., Marshall, A. K. & Symanski, E. Maternal residential proximity to unconventional gas development and perinatal outcomes among a diverse urban population in Texas. PLoS One 12, e0180966, https://doi.org/10.1371/journal.pone.0180966 (2017).
Rasmussen, S. G. et al. Association between unconventional natural gas development in the Marcellus Shale and asthma exacerbations. JAMA Intern Med 176, 1334–1343, https://doi.org/10.1001/jamainternmed.2016.2436 (2016).
Rabinowitz, P. M. et al. Proximity to natural gas wells and reported health status: results of a household survey in Washington County, Pennsylvania. Environ Health Perspect 123, 21–26, https://doi.org/10.1289/ehp.1307732 (2015).
Saberi, P., Propert, K. J., Powers, M., Emmett, E. & Green-McKenzie, J. Field survey of health perception and complaints of Pennsylvania residents in the Marcellus Shale region. Int J Environ Res Publ Health 11, 6517–6527 (2014).
Tustin, A. W. et al. Associations between unconventional natural gas development and nasal and sinus, migraine headache, and fatigue symptoms in Pennsylvania. Environ Health Perspect 125, 189–197, https://doi.org/10.1289/EHP281 (2017).
Hirsch, J. K. et al. Psychosocial impact of fracking: a review of the literature on the mental health consequences of hydraulic fracturing. Int J Ment Health Addict 16, 1–15 (2018).
Murray, C. J. et al. The state of US health, 1990–2010: burden of diseases, injuries, and risk factors. JAMA 310, 591–606 (2013).
Greenberg, P. E., Fournier, A.-A., Sisitsky, T., Pike, C. T. & Kessler, R. C. The economic burden of adults with major depressive disorder in the United States (2005 and 2010). J Clin Psychiatry 76, 155–162 (2015).
Liu, Y. Prevalence of healthy sleep duration among adults—United States, 2014. MMWR Morb Mortal Wkly Rep 65 (2016).
Riemann, D. Insomnia and comorbid psychiatric disorders. Sleep Med 8(Suppl 4), S15–20, https://doi.org/10.1016/S1389-9457(08)70004-2 (2007).
Riemann, D. & Voderholzer, U. Primary insomnia: a risk factor to develop depression? J Affect Disord 76, 255–259 (2003).
Lai, P.-H., Lyons, K. D., Gudergan, S. P. & Grimstad, S. Understanding the psychological impact of unconventional gas developments in affected communities. Energ Policy 101, 492–501 (2017).
Maguire, K. & Winters, J. V. Energy boom and gloom? Local effects of oil and natural gas drilling on subjective well-being. Growth Change 48, 590–610, https://doi.org/10.1111/grow.12204 (2016).
Sangaramoorthy, T. et al. Place-based perceptions of the impacts of fracking along the Marcellus Shale. Soc Sci Med 151, 27–37, https://doi.org/10.1016/j.socscimed.2016.01.002 (2016).
Thomas, M., Partridge, T., Harthorn, B. H. & Pidgeon, N. Deliberating the perceived risks, benefits, and societal implications of shale gas and oil extraction by hydraulic fracturing in the US and UK. Nat Energy 2, https://doi.org/10.1038/nenergy.2017.54 (2017).
Evensen, D. & Stedman, R. Beliefs about impacts matter little for attitudes on shale gas development. Energ Policy 109, 10–21 (2017).
Perry, S. L. Using ethnography to monitor the community health implications of onshore unconventional oil and gas developments: examples from Pennsylvania’s Marcellus Shale. New Solut 23, 33–53, https://doi.org/10.2190/NS.23.1.d (2013).
Willow, A. J., Zak, R., Vilaplana, D. & Sheeley, D. The contested landscape of unconventional energy development: a report from Ohio’s shale gas country. J Environ Stud Sci 4, 56–64 (2014).
Power, M. C. et al. The relation between past exposure to fine particulate air pollution and prevalent anxiety: observational cohort study. BMJ 350, h1111, https://doi.org/10.1136/bmj.h1111 (2015).
Kim, K. N. et al. Long-term fine particulate matter exposure and major depressive disorder in a community-based urban cohort. Environ Health Perspect 124, 1547–1553, https://doi.org/10.1289/EHP192 (2016).
Evans, G. W., Hygge, S. & Bullinger, M. Chronic noise and psychological stress. Psychol Sci, 333–338 (1995).
Navara, K. J. & Nelson, R. J. The dark side of light at night: physiological, epidemiological, and ecological consequences. J Pineal Res 43, 215–224 (2007).
Haines, M. M., Stansfeld, S. A., Job, R. F., Berglund, B. & Head, J. A follow-up study of effects of chronic aircraft noise exposure on child stress responses and cognition. Int J Epidemiol 30, 839–845 (2001).
Werner, A. K., Vink, S., Watt, K. & Jagals, P. Environmental health impacts of unconventional natural gas development: A review of the current strength of evidence. Sci Total Environ 505C, 1127–1141, https://doi.org/10.1016/j.scitotenv.2014.10.084 (2015).
Ferrar, K. J. et al. Assessment and longitudinal analysis of health impacts and stressors perceived to result from unconventional shale gas development in the Marcellus Shale region. Int J Occup Environ Health 19, 104–112, https://doi.org/10.1179/2049396713Y.0000000024 (2013).
Fernando, F. N. & Cooley, D. R. Attitudes toward shale oil development in western North Dakota: the role of place based community values in attitude formation. J Rural Stud 46, 132–146 (2016).
Fisher, M. P., Mayer, A., Vollet, K., Hill, E. L. & Haynes, E. N. Psychosocial implications of unconventional natural gas development: Quality of life in Ohio’s Guernsey and Noble Counties. J Environ Psychol 55, 90–98, https://doi.org/10.1016/j.jenvp.2017.12.008 (2018).
McDermott‐Levy, R. & Garcia, V. Health concerns of northeastern Pennsylvania residents living in an unconventional oil and gas development county. Public Health Nurs 33, 502–510 (2016).
Antonaci, F. et al. Migraine and psychiatric comorbidity: a review of clinical findings. J Headache Pain 12, 115–125, https://doi.org/10.1007/s10194-010-0282-4 (2011).
Demyttenaere, K., De Fruyt, J. & Stahl, S. M. The many faces of fatigue in major depressive disorder. Int J Neuropsychopharmacol 8, 93–105, https://doi.org/10.1017/S1461145704004729 (2005).
Chen, E. & Miller, G. E. Stress and inflammation in exacerbations of asthma. Brain Behav Immun 21, 993–999, https://doi.org/10.1016/j.bbi.2007.03.009 (2007).
Yonas, M. A., Lange, N. E. & Celedon, J. C. Psychosocial stress and asthma morbidity. Curr Opin Allergy Clin Immunol 12, 202–210, https://doi.org/10.1097/ACI.0b013e32835090c9 (2012).
Thompson, A. M. et al. Baseline repeated measures from controlled human exposure studies: associations between ambient air pollution exposure and the systemic inflammatory biomarkers IL-6 and fibrinogen. Environ Health Perspect 118, 120 (2010).
Kelly, F. J. Oxidative stress: its role in air pollution and adverse health effects. Occup Environ Med 60, 612–616 (2003).
Miller, G. E., Stetler, C. A., Carney, R. M., Freedland, K. E. & Banks, W. A. Clinical depression and inflammatory risk markers for coronary heart disease. Am J Cardiol 90, 1279–1283 (2002).
Ng, F., Berk, M., Dean, O. & Bush, A. I. Oxidative stress in psychiatric disorders: evidence base and therapeutic implications. Int J Neuropsychopharmacol 11, 851–876 (2008).
Orban, E. et al. Residential road traffic noise and high depressive symptoms after five years of follow-up: results from the Heinz Nixdorf Recall Study. Environ Health Perspect 124, 578–585, https://doi.org/10.1289/ehp.1409400 (2016).
North, C. S. & Pfefferbaum, B. Mental health response to community disasters: a systematic review. JAMA 310, 507–518, https://doi.org/10.1001/jama.2013.107799 (2013).
Rung, A. L. et al. Depression, mental distress, and domestic conflict among Louisiana women exposed to the Deepwater Horizon oil spill in the WaTCH study. Environ Health Perspect 124, 1429 (2016).
Weingarten, M., Ge, S., Godt, J. W., Bekins, B. A. & Rubinstein, J. L. Induced Seismicity. High-rate injection is associated with the increase in U.S. mid-continent seismicity. Science 348, 1336–1340, https://doi.org/10.1126/science.aab1345 (2015).
Casey, J. A., Goldman-Mellor, S. & Catalano, R. Association between Oklahoma earthquakes and anxiety-related Google search episodes. Under review (2018).
Adler, N. E. & Stead, W. W. Patients in context–EHR capture of social and behavioral determinants of health. N Engl J Med 372, 698–701, https://doi.org/10.1056/NEJMp1413945 (2015).
Kroenke, K. & Spitzer, R. L. The PHQ-9: a new depression diagnostic and severity measure. Psychiatr Ann 32, 509–515 (2002).
Kroenke, K. et al. The PHQ-8 as a measure of current depression in the general population. J Affect Disord 114, 163–173 (2009).
Hirsch, A. G. et al. Nasal and sinus symptoms and chronic rhinosinusitis in a population-based sample. Allergy 72, 274–281, https://doi.org/10.1111/all.13042 (2017).
Mulsant, B. H., Ganguli, M. & Seaberg, E. C. The relationship between self‐rated health and depressive symptoms in an epidemiological sample of community‐dwelling older adults. J Am Geriatr Soc 45, 954–958 (1997).
Schneiderman, N., Ironson, G. & Siegel, S. D. Stress and health: psychological, behavioral, and biological determinants. Annu Rev Clin Psychol 1, 607–628 (2005).
Sacks, J. D. et al. Particulate matter-induced health effects: who is susceptible? Environ Health Perspect 119, 446–454, https://doi.org/10.1289/ehp.1002255 (2011).
WHO (World Health Organization). Night Noise Guidelines For Europe, http://www.euro.who.int/__data/assets/pdf_file/0017/43316/E92845.pdf (2009).
Waston, N. & Wooden, M. In Methodology of Longitudinal Surveys (ed. Peter Lynn) (John Wiley & Sons, Incorporated, 2009).
Meltzer, L. J., Johnson, C., Crosette, J., Ramos, M. & Mindell, J. A. Prevalence of diagnosed sleep disorders in pediatric primary care practices. Pediatrics 125, e1410–e1418 (2010).
Ford, E. S. et al. Trends in outpatient visits for insomnia, sleep apnea, and prescriptions for sleep medications among US ddults: findings from the National Ambulatory Medical Care Survey 1999–2010. Sleep 37, 1283–1293, https://doi.org/10.5665/sleep.3914 (2014).
Gundlapalli, A. V. et al. Validating a strategy for psychosocial phenotyping using a large corpus of clinical text. J Am Med Inform Assoc 20, e355 (2013).
Mayer, A. Risk and benefits in a fracking boom: Evidence from Colorado. The Extractive Industries and Society 3, 744–753 (2016).
Kriesky, J., Goldstein, B. D., Zell, K. & Beach, S. Differing opinions about natural gas drilling in two adjacent counties with different levels of drilling activity. Energ Policy 58, 228–236 (2013).
Korfmacher, K. S., Jones, W. A., Malone, S. L. & Vinci, L. F. Public health and high volume hydraulic fracturing. New Solut 23, 13–31 (2013).
Schwartz, B. S. et al. Body mass index and the built and social environments in children and adolescents using electronic health records. Am J Prev Med 41, e17–e28, https://doi.org/10.1016/J.Amepre.2011.06.038 (2011).
Koehler, K. et al. Exposure assessment using secondary data sources in unconventional natural gas development and health studies. Environ Sci Technol 52, 6061–6069, https://doi.org/10.1021/acs.est.8b00507 (2018).
Casey, J. A. et al. Measures of SES for Electronic Health Record-based Research. Am J Prev Med 54, 430–439, https://doi.org/10.1016/j.amepre.2017.10.004 (2018).
Liu, A. Y., Curriero, F. C., Glass, T. A., Stewart, W. F. & Schwartz, B. S. Associations of the burden of coal abandoned mine lands with three dimensions of community context in Pennsylvania. ISRN Public Health 2012, 1–11, https://doi.org/10.5402/2012/251201 (2012).
Pennsylvania Department of Health. Public Water Systems, http://www.health.pa.gov/My%20Health/Environmental%20Health/Environmental%20Public%20Health%20Tracking/Pages/Metadata-for-Drinking-Water-Quality.aspx#.WqBg6pMbPGJ (2015).
Potter, F. In American Statistical Association Proceedings of the Survey Research Methods Section 453–458 (1988).
Esser, M. B. et al. Prevalence of alcohol dependence among US adult drinkers, 2009-2011. Prev Chronic Dis 11, E206, https://doi.org/10.5888/pcd11.140329 (2014).
Gries, C. J. et al. Predictors of symptoms of posttraumatic stress and depression in family members after patient death in the ICU. Chest 137, 280–287, https://doi.org/10.1378/chest.09-1291 (2010).
Kim, K.-H., Lee, S.-M., Paik, J.-W. & Kim, N.-S. The effects of continuous antidepressant treatment during the first 6 months on relapse or recurrence of depression. J Affect Disord 132, 121–129 (2011).
Kornstein, S. G., Bose, A., Li, D., Saikali, K. G. & Gandhi, C. Escitalopram maintenance treatment for prevention of recurrent depression: a randomized, placebo-controlled trial. J Clin Psychiatry 67, 1767–1775 (2006).
Pike, G. R. Using weighting adjustments to compensate for survey nonresponse. Res High Educ 49, 153–171 (2008).
Acknowledgements
We thank Sara Rasmussen for her contributions to the work (Johns Hopkins Bloomberg School of Public Health); Joseph J. DeWalle, BS (Geisinger) for patient geocoding; Aaron Tustin (JHSPH) for assistance with survey weights; and Karen Bandeen-Roche, PhD (JHSPH) for assistance in approach to analysis and interpretation of results. This research was funded by National Institutes of Health U19 AI106683 (PI Robert Schleimer), R21 ES023675 (PI Brian Schwartz), K99 ES027023 (Joan Casey), and the Degenstein Foundation. No funders had input into the study design, conduct, data collection or analysis, or manuscript preparation.
Author information
Authors and Affiliations
Contributions
J.A.C., H.C.W., A.G.H., J.P., and B.S.S. were involved in conceptualizing the study. B.S.S. and A.G.H. were involved in collection of the health data. J.A.C. and B.S.S. developed and implemented the exposure metric. J.P. analyzed the data. J.A.C. prepared Figure 1. J.A.C. and B.S.S. wrote the manuscript. J.A.C., H.C.W., A.G.H., J.P., and B.S.S. supplied feedback and revised the manuscript.
Corresponding author
Ethics declarations
Competing Interests
Drs Casey, Wilcox, Hirsch and Pollak declare they have no actual or potential competing financial interests. Dr. Schwartz is a Fellow of the Post Carbon Institute (PCI), serving as an informal advisor on climate, energy, and health issues. He receives no payment for this role. His research is entirely independent of PCI, and is not motivated, reviewed, or funded by PCI.
Additional information
Publisher's note: Springer Nature remains neutral with regard to jurisdictional claims in published maps and institutional affiliations.
Electronic supplementary material
Rights and permissions
Open Access This article is licensed under a Creative Commons Attribution 4.0 International License, which permits use, sharing, adaptation, distribution and reproduction in any medium or format, as long as you give appropriate credit to the original author(s) and the source, provide a link to the Creative Commons license, and indicate if changes were made. The images or other third party material in this article are included in the article’s Creative Commons license, unless indicated otherwise in a credit line to the material. If material is not included in the article’s Creative Commons license and your intended use is not permitted by statutory regulation or exceeds the permitted use, you will need to obtain permission directly from the copyright holder. To view a copy of this license, visit http://creativecommons.org/licenses/by/4.0/.
About this article
Cite this article
Casey, J.A., Wilcox, H.C., Hirsch, A.G. et al. Associations of unconventional natural gas development with depression symptoms and disordered sleep in Pennsylvania. Sci Rep 8, 11375 (2018). https://doi.org/10.1038/s41598-018-29747-2
Received:
Accepted:
Published:
DOI: https://doi.org/10.1038/s41598-018-29747-2
This article is cited by
-
The Environment and Headache: a Narrative Review
Current Environmental Health Reports (2024)
-
Ethical Implications of the Impact of Fracking on Brain Health
Neuroethics (2024)
-
Historic redlining and the siting of oil and gas wells in the United States
Journal of Exposure Science & Environmental Epidemiology (2023)
-
Prevalence of ANCA-associated vasculitis amid natural gas drilling sites in West Virginia
Journal of Nephrology (2022)
-
Assessing Exposure to Unconventional Oil and Gas Development: Strengths, Challenges, and Implications for Epidemiologic Research
Current Environmental Health Reports (2022)
Comments
By submitting a comment you agree to abide by our Terms and Community Guidelines. If you find something abusive or that does not comply with our terms or guidelines please flag it as inappropriate.