Abstract
Parkinson’s disease is a progressive neurodegenerative movement disorder with a long latent phase and currently no disease-modifying treatments. Reliable predictive biomarkers that could transform efforts to develop neuroprotective treatments remain to be identified. Using UK Biobank, we investigated the predictive value of accelerometry in identifying prodromal Parkinson’s disease in the general population and compared this digital biomarker with models based on genetics, lifestyle, blood biochemistry or prodromal symptoms data. Machine learning models trained using accelerometry data achieved better test performance in distinguishing both clinically diagnosed Parkinson’s disease (n = 153) (area under precision recall curve (AUPRC) 0.14 ± 0.04) and prodromal Parkinson’s disease (n = 113) up to 7 years pre-diagnosis (AUPRC 0.07 ± 0.03) from the general population (n = 33,009) compared with all other modalities tested (genetics: AUPRC = 0.01 ± 0.00, P = 2.2 × 10−3; lifestyle: AUPRC = 0.03 ± 0.04, P = 2.5 × 10−3; blood biochemistry: AUPRC = 0.01 ± 0.00, P = 4.1 × 10−3; prodromal signs: AUPRC = 0.01 ± 0.00, P = 3.6 × 10−3). Accelerometry is a potentially important, low-cost screening tool for determining people at risk of developing Parkinson’s disease and identifying participants for clinical trials of neuroprotective treatments.
This is a preview of subscription content, access via your institution
Access options
Access Nature and 54 other Nature Portfolio journals
Get Nature+, our best-value online-access subscription
$29.99 / 30 days
cancel any time
Subscribe to this journal
Receive 12 print issues and online access
$209.00 per year
only $17.42 per issue
Buy this article
- Purchase on Springer Link
- Instant access to full article PDF
Prices may be subject to local taxes which are calculated during checkout





Similar content being viewed by others
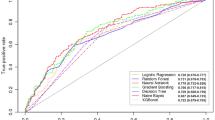
Data availability
DData from the UK Biobank (ukbiobank.ac.uk/) are available to researchers on application to the UK Biobank following the steps outlined here: https://www.ukbiobank.ac.uk/enable-your-research.
Code availability
Code that supports the findings of this study is available on GitHub https://github.com/aschalkamp/UKBBprodromalPD.
References
Fearnley, J. M. & Lees, A. J. Ageing and Parkinson’s disease: substantia nigra regional selectivity. Brain 114, 2283–2301 (1991).
Heinzel, S. et al. Update of the MDS research criteria for prodromal Parkinson’s disease. Mov. Disord. 34, 1464–1470 (2019).
Postuma, R. B. & Berg, D. Advances in markers of prodromal Parkinson disease. Nat. Rev. Neurol. 12, 622–634 (2016).
Postuma, R. B. & Berg, D. Prodromal Parkinson’s disease: the decade past, the decade to come. Mov. Disord. 34, 665–675 (2019).
Hustad, E. & Aasly, J. O. Clinical and imaging markers of prodromal Parkinson’s disease. Front. Neurol. 11, 395 (2020).
Fereshtehnejad, S. M. et al. Validation of the MDS research criteria for prodromal Parkinson’s disease: longitudinal assessment in a REM sleep behavior disorder (RBD) cohort. Mov. Disord. 32, 865–873 (2017).
Fereshtehnejad, S. M. et al. Evolution of prodromal Parkinson’s disease and dementia with Lewy bodies: a prospective study. Brain 142, 2051–2067 (2019).
Brognara, L., Palumbo, P., Grimm, B. & Palmerini, L. Assessing gait in Parkinsona’s disease using wearable motion sensors: a systematic review. Diseases 7, 18 (2019).
Dorsey, E. R. et al. Deep phenotyping of Parkinson’s disease. J. Parkinsons Dis. 10, 855–873 (2020).
Shah, V. V. et al. Digital biomarkers of mobility in Parkinson’s disease during daily living. J. Parkinsons Dis. 10, 1099–1111 (2020).
Schlachetzki, J. C. M. et al. Wearable sensors objectively measure gait parameters in Parkinson’s disease. PLoS ONE 12, e0183989 (2017).
Johansson, D., Malmgren, K. & Alt Murphy, M. Wearable sensors for clinical applications in epilepsy, Parkinson’s disease, and stroke: a mixed-methods systematic review. J. Neurol. 265, 1740–1752 (2018).
Del Din, S. et al. Gait analysis with wearables predicts conversion to Parkinson disease. Ann. Neurol. 86, 357–367 (2019).
Williamson, J. R., Telfer, B., Mullany, R. & Friedl, K. E. Detecting Parkinson’s disease from wrist-worn accelerometry in the U.K. Biobank. Sensors (Basel) 21, 2047 (2021).
Mirelman, A. et al. Arm swing as a potential new prodromal marker of Parkinson’s disease. Mov. Disord. 31, 1527–1534 (2016).
Bycroft, C. et al. The UK Biobank resource with deep phenotyping and genomic data. Nature 562, 203–209 (2018).
Doherty, A. et al. Large scale population assessment of physical activity using wrist worn accelerometers: the UK Biobank study. PLoS ONE 12, e0169649 (2017).
Parkinson’s UK (2017). The Incidence and Prevalence of Parkinson’s in the UK. Available at: https://www.parkinsons.org.uk/sites/default/files/2018-01/Prevalence%20%20Incidence%20Report%20Latest_Public_2.pdf (Accessed: June 2022).
Walmsley, R. et al. Reallocation of time between device-measured movement behaviours and risk of incident cardiovascular disease. Br. J. Sports Med. 56, 1008–1017 (2021).
Collins, G. S., Reitsma, J. B., Altman, D. G. & Moons, K. G. Transparent reporting of a multivariable prediction model for individual prognosis or diagnosis (TRIPOD): the TRIPOD Statement. BMC Med. 13, 1 (2015).
Davis, J. & Goadrich, M. The relationship between precision-recall and ROC curves. Proc. 23rd Int. Conf. Mach. Learn. 23, 233–240 (ACM, New York, 2006).
Darweesh, S. K. et al. Trajectories of prediagnostic functioning in Parkinson’s disease. Brain 140, 429–441 (2017).
Yang, Y. et al. Artificial intelligence-enabled detection and assessment of Parkinson’s disease using nocturnal breathing signals. Nat. Med. 28, 2207–2215 (2022).
Swaddiwudhipong, N. et al. Pre-diagnostic cognitive and functional impairment in multiple sporadic neurodegenerative diseases. Alzheimers Dement. 19, 1752–1763 (2023).
Chandrasekaran, R., Katthula, V. & Moustakas, E. Patterns of use and key predictors for the use of wearable health care devices by US adults: insights from a national survey. J. Med. Internet Res. 22, e22443 (2020).
Simon, D. A., Shachar, C. & Cohen, I. G. Unsettled liability issues for ‘prediagnostic’ wearables and health-related products. JAMA 328, 1391–1392 (2022).
Xu, S., Kim, J., Walter, J. R., Ghaffari, R. & Rogers, J. A. Translational gaps and opportunities for medical wearables in digital health. Sci. Transl. Med. 14, eabn6036 (2022).
The Parkinson Progression Marker Initiative The Parkinson Progression Marker Initiative (PPMI). Prog. Neurobiol. 95, 629–635 (2011).
The All of Us Research Program InvestigatorsThe ‘All of Us’ Research Program. N. Engl. J. Med. 381, 668–676 (2019).
Brigo, F., Matinella, A., Erro, R. & Tinazzi, M. [123I]FP-CIT SPECT (DaTSCAN) may be a useful tool to differentiate between Parkinson’s disease and vascular or drug-induced parkinsonisms: a meta-analysis. Eur. J. Neurol. 21, 1369–e90 (2014).
Wu, P. et al. Mapping ICD-10 and ICD-10-CM codes to phecodes: workflow development and initial evaluation. JMIR Med. Inform. 7, e14325 (2019).
NHS Digital (2018). NHS Read Browser. Available at: https://isd.digital.nhs.uk/trud/users/guest/filters/2/categories/9/items/8/releases (Accessed: July 2022).
Wu, Y. et al. Genome-wide association study of medication-use and associated disease in the UK Biobank. Nat. Commun. 10, 1891 (2019).
Willetts, M., Hollowell, S., Aslett, L., Holmes, C. & Doherty, A. Statistical machine learning of sleep and physical activity phenotypes from sensor data in 96,220 UK Biobank participants. Sci. Rep. 8, 7961 (2018).
Brandes, N., Linial, N. & Linial, M. PWAS: proteome-wide association study-linking genes and phenotypes by functional variation in proteins. Genome Biol. 21, 173 (2020).
Vallat, R. Pingouin: statistics in Python. J. Open Source Softw. 3, 1026 (2018).
Pedregosa, F. et al. Scikit-learn: machine learning in Python. J. Mach. Learn. Res. 12, 2825–2830 (2011).
Pölsterl, S. scikit-survival: a library for time-to-event analysis built on top of scikit-learn. J. Mach. Learn. Res. 21, 1–6 (2020).
Michael Waskom, et al. (2022). mwaskom/seaborn: v0.12.1 (Zenodo, October 2022) https://doi.org/10.5281/zenodo.7220902
Van Calster, B. et al. Calibration: the Achilles heel of predictive analytics. BMC Med. 17, 230 (2019).
Acknowledgements
We are grateful for the Advanced Research Computing at Cardiff. We are also grateful for the valuable comments of C. Webber and the input on survival modeling from V. Escott-Price. A.-K.S. is supported by a PhD studentship funded by the Welsh Government through Health and Care Research Wales (HS-20-11). C.S. is supported by the UK Dementia Research Institute funded by the Medical Research Council (MRC), Alzheimer’s Society and Alzheimer’s Research UK and by the Ser Cymru II programme (CU187) which is part-funded by Cardiff University and the European Regional Development Fund through the Welsh Government. K.P. is funded by an MRC Clinician-Scientist Fellowship (MR/P008593/1). N.H. has nothing to declare.
Author information
Authors and Affiliations
Contributions
A.-K.S. and C.S. participated in designing the study, topic definition and review of relevant studies. Machine learning models and statistical analyses were designed and implemented by A.-K.S. Figures and tables were done by A.-K.S. with the support of C.S. A.-K.S. wrote the first draft. A.-K.S., C.S., N.A.H. and K.J.P. contributed to subsequent versions of the manuscript. All authors critically reviewed the paper, all authors have a clear understanding of the content, results and conclusions of the study and agree to submit this manuscript for publication. The corresponding author (C.S.) declares that all authors listed meet the authorship criteria and that no other authors involved in this study are omitted. C.S. is ultimately responsible for this article.
Corresponding author
Ethics declarations
Competing interests
The authors declare no competing interests.
Peer review
Peer review information
Nature Medicine thanks Ronald Postuma, Bjoern Eskofier, Thomas Debray and Matthew Sperrin for their contribution to the peer review of this work. Primary Handling Editor: Lorenzo Righetto, in collaboration with the Nature Medicine team.
Additional information
Publisher’s note Springer Nature remains neutral with regard to jurisdictional claims in published maps and institutional affiliations.
Extended data
Extended Data Fig. 1 Overview of all logistic regression models.
We show with which sample sizes and which predictors each model is trained. With three different outcomes/cases and three different control groups, a total of nine different scenarios are modelled. We distinguish between baseline, modality-specific, and early and late combined models. For each model we show in brackets the number of predictors included.
Extended Data Fig. 2 Incidence and prevalence of Parkinson’s disease in UK Biobank.
The plots show (a) the cumulative number of Parkinson’s disease (PD) diagnoses expected and observed each year for the UK Biobank, (b) the percentage of people getting a diagnosis within a specific age-range, and (c) the proportion of people getting a new/incident PD diagnosis each year.
Extended Data Fig. 3 Feature importance for each modality for the prodromal vs matched unaffected controls models.
The most important, significant features across the five outer cross-validation splits are shown for the prodromal Parkinson’s disease (PD) versus matched unaffected controls models. For each feature we show the mean regression coefficient across the outer five cross-validation folds and the 95% CI corrected for multiple comparisons with the Bonferroni-correction. Features that increase the likelihood of getting PD are shown in red, whereas those that decrease the likelihood are shown in blue. If no features reached significance across folds, an empty plot is shown. Each plot shows the features of a model trained on a different feature set/modality: (a) genetics+family, (b) lifestyle, (c) blood, (d) prodromal symptoms, (e) all accelerometry, (f) combined.
Extended Data Fig. 4 Feature importance for each modality for the prodromal vs all unaffected controls models.
The most important, significant features across the five outer cross-validation splits are shown for the prodromal Parkinson’s disease (PD) versus matched unaffected controls models. For each feature we show the mean regression coefficient across the outer five cross-validation folds and the 95% CI corrected for multiple comparisons with the Bonferroni-correction. Features that increase the likelihood of getting PD are shown in red, whereas those that decrease the likelihood are shown in blue. Each plot shows the features of a model trained on a different feature set/modality: (a) genetics+family, (b) lifestyle, (c) blood, (d) prodromal symptoms, (e) all accelerometry, (f) combined.
Extended Data Fig. 5 Feature importance for each modality for the prodromal vs population models.
The most important, significant features across the five outer cross-validation splits are shown for the prodromal Parkinson’s disease (PD) versus matched unaffected controls models. For each feature we show the mean regression coefficient across the outer five cross-validation folds and the 95% CI corrected for multiple comparisons with the Bonferroni-correction. Features that increase the likelihood of getting PD are shown in red, whereas those that decrease the likelihood are shown in blue. If no features reached significance across folds, an empty plot is shown. Each plot shows the features of a model trained on a different feature set/modality: (a) genetics+family, (b) lifestyle, (c) blood, (d) prodromal symptoms, (e) all accelerometry, (f) combined.
Extended Data Fig. 6 Predicted probability on the test sets for each diagnosis group.
The boxplots show the mean predicted probability on the test set across the five outer folds for each diagnosis group with the 25% and 75% quartiles as the bounds of the box, and the Q3 + 1.5*IQR/ Q1 - 1.5*IQR as the whiskers. The individual data points for each subject are shown as overlayed dots. The predicted probabilities are also shown for the external data (not used for training or testing): for the prodromal model, the diagnosed Parkinson’s disease (PD) group is used and for the diagnosed model, the prodromal PD group is used. The dashed line indicates a potential 0.5 probability threshold to define the cut-off. This is shown for [left] the prodromal model and [right] the diagnosed model and for each modality-specific model: (a, b) genetics & family, (c, d) blood biochemistry, (e, f) lifestyle, (g–h) prodromal symptoms, (i, j) accelerometry, and (k, l) combined.
Extended Data Fig. 7 Slowness as the most informative feature across models.
The group comparisons with two-sided T-tests for the most informative and stable predictor, mean movement during epochs classified as ‘light’, are shown. The continuous feature is age, sex, and BMI corrected through coefficients learned from the unaffected control cohort. The boxplots show the mean as the centre, the 25% and 75% quartiles as the bounds of the box, and the Q3 + 1.5*IQR/ Q1 - 1.5*IQR as the whiskers. The yellow boxes show the number of subjects in each group. The p-values are shown when 0.05 Bonferroni-corrected significance is reached at 2.38×10−3.
Extended Data Fig. 8 Schematic figure of survival model.
The plot shows the survival function for one Parkinson’s disease (PD) prodromal subject and its matched unaffected control. The probability of not getting a diagnosis of PD is shown since the time of accelerometer data collection as estimated by the random survival forest trained on the matched unaffected control setting using all accelerometry features. The intersection of the survival function of the prodromal case with the chosen 0.5 probability threshold (black dashed line) is close to the real time of diagnosis (dashed orange line), meaning that the model correctly predicted the time of diagnosis for this subject.
Extended Data Fig. 9 Calibration of the prodromal models.
The calibration plots are shown for each single modality model and the combined model. We show this for all three control groups: (a) matched unaffected controls, (b) unmatched unaffected controls, and (c) general population. The curves show the mean predicted probability binned into ten bins against the true fraction of positives/prevalence. The dashed black line indicates perfect calibration.
Supplementary information
Supplementary Information
Supplementary Methods, legends for Tables 1–18, Figs. 1–20 and TRIPOD reporting checklist.
Supplementary Table 1
This excel file holds the data of all the supplementary tables as described in the Supplementary Information.
Rights and permissions
Springer Nature or its licensor (e.g. a society or other partner) holds exclusive rights to this article under a publishing agreement with the author(s) or other rightsholder(s); author self-archiving of the accepted manuscript version of this article is solely governed by the terms of such publishing agreement and applicable law.
About this article
Cite this article
Schalkamp, AK., Peall, K.J., Harrison, N.A. et al. Wearable movement-tracking data identify Parkinson’s disease years before clinical diagnosis. Nat Med 29, 2048–2056 (2023). https://doi.org/10.1038/s41591-023-02440-2
Received:
Accepted:
Published:
Issue Date:
DOI: https://doi.org/10.1038/s41591-023-02440-2
This article is cited by
-
Impaired 24-h activity patterns are associated with an increased risk of Alzheimer’s disease, Parkinson’s disease, and cognitive decline
Alzheimer's Research & Therapy (2024)
-
Machine learning in physical activity, sedentary, and sleep behavior research
Journal of Activity, Sedentary and Sleep Behaviors (2024)
-
Den Rauch riechen, bevor man das Feuer sieht – die oligosymptomatische Prodromalphase neurodegenerativer Erkrankungen
Der Nervenarzt (2024)
-
Artificial intelligence in neurology: opportunities, challenges, and policy implications
Journal of Neurology (2024)