Abstract
Small lakes and ponds occupy an enormous surface area of inland freshwater and represent an important terrestrial–water interface. Disturbances caused by extreme weather events can have substantial effects on these ecosystems. Here, we analysed the dynamics of nutrients and the entire plankton community in two flood events and afterwards, when quasi-stable conditions were established, to investigate the effect of such disturbances on a small forest pond. We show that floodings result in repeated washout of resident organisms and hundredfold increases in nutrient load. Despite this, the microbial community recovers to a predisturbance state within two weeks of flooding through four well-defined succession phases. Reassembly of phytoplankton and especially zooplankton takes up to two times longer and features repetitive and adaptive patterns. Release of dissolved nutrients from the pond is associated with inflow rates and community recovery, and returns to predisturbance levels before microbial compositions recover. Our findings shed light on the mechanisms underlying functional resilience of small waterbodies and are relevant to global change-induced increases in weather extremes.
This is a preview of subscription content, access via your institution
Access options
Access Nature and 54 other Nature Portfolio journals
Get Nature+, our best-value online-access subscription
$29.99 / 30 days
cancel any time
Subscribe to this journal
Receive 12 digital issues and online access to articles
$119.00 per year
only $9.92 per issue
Buy this article
- Purchase on Springer Link
- Instant access to full article PDF
Prices may be subject to local taxes which are calculated during checkout





Similar content being viewed by others
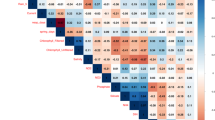
Data availability
The 16S rRNA and rDNA amplicon data from this study were deposited to NCBI under BioProject PRJNA547706, BioSamples SAMN11974970-11975031. Sequences of 23S rRNA genes obtained from clone libraries were submitted to NCBI under accession numbers MN565597–MN565664. The Silva SSU and LSU databases release 132 (https://www.arb-silva.de/download/archive/) were used for the sequence analysis and probe design. Source data are provided with this paper. Additional information can be obtained from the corresponding author upon request.
References
Battin, T. J. et al. Biophysical controls on organic carbon fluxes in fluvial networks. Nat. Geosci. 1, 95–100 (2008).
Tranvik, L. J. et al. Lakes and reservoirs as regulators of carbon cycling and climate. Limnol. Oceanogr. 54, 2298–2314 (2009).
Raymond, P. A. et al. Global carbon dioxide emissions from inland waters. Nature 503, 355–359 (2013).
Downing, J. A. Emerging global role of small lakes and ponds: little things mean a lot. Limnetica 29, 9–24 (2010).
Bastviken, D., Tranvik, L. J., Downing, J. A., Crill, P. M. & Enrich-Prast, A. Freshwater methane emissions offset the continental carbon sink. Science 331, 50–50 (2011).
Fairchild, G. W. & Velinsky, D. J. Effects of small ponds on stream water chemistry. Lake Reserv. Manag. 22, 321–330 (2006).
Yin, C. & Shan, B. Multipond systems: a sustainable way to control diffuse phosphorus pollution. AMBIO 30, 369–375 (2001).
Stanley, E. H. & Doyle, M. W. A geomorphic perspective on nutrient retention following dam removal: geomorphic models provide a means of predicting ecosystem responses to dam removal. BioScience 52, 693–701 (2002).
Downing, J. A., Cherrier, C. T. & Fulweiler, R. W. Low ratios of silica to dissolved nitrogen supplied to rivers arise from agriculture not reservoirs. Ecol. Lett. 19, 1414–1418 (2016).
Dickman, M. Some effects of lake renewal on phytoplankton productivity and species composition. Limnol. Oceanogr. 14, 660–666 (1969).
Madsen, H., Lawrence, D., Lang, M., Martinkova, M. & Kjeldsen, T. R. Review of trend analysis and climate change projections of extreme precipitation and floods in Europe. J. Hydrol. 519, 3634–3650 (2014).
Clark, J. M. et al. The importance of the relationship between scale and process in understanding long-term DOC dynamics. Sci. Total Environ. 408, 2768–2775 (2010).
Vystavna, Y., Hejzlar, J. & Kopáček, J. Long-term trends of phosphorus concentrations in an artificial lake: socio-economic and climate drivers. PLoS ONE 12, e0186917 (2017).
Reynolds, C. S. Phytoplankton assemblages and their periodicity in stratifying lake systems. Ecography 3, 141–159 (1980).
Sommer, U., Gliwicz, Z. M., Lampert, W. & Duncan, A. The PEG-model of seasonal succession of planktonic events in fresh waters. Arch. Hydrobiol. 106, 433–471 (1986).
Kundzewicz, Z. W. et al. Differences in flood hazard projections in Europe—their causes and consequences for decision making. Hydrol. Sci. J. 62, 1–14 (2017).
Arnell, N. W. & Gosling, S. N. The impacts of climate change on river flood risk at the global scale. Clim. Change 134, 387–401 (2016).
Hirabayashi, Y. et al. Global flood risk under climate change. Nat. Clim. Change 3, 816–821 (2013).
Lynch, L. M. et al. River channel connectivity shifts metabolite composition and dissolved organic matter chemistry. Nat. Commun. 10, 459 (2019).
Pimm, S. L. The complexity and stability of ecosystems. Nature 307, 321–326 (1984).
Shade, A. et al. Lake microbial communities are resilient after a whole-ecosystem disturbance. ISME J. 6, 2153–2167 (2012).
Holling, C. S. Resilience and stability of ecological systems. Annu. Rev. Ecol. Evol. Syst. 4, 1–23 (1973).
Holling, C. S. & Gunderson, L. H. in Panarchy Synopsis: Understanding Transformations in Human and Natural Systems (eds Gunderson, L. H. & Holling, C. S.) 25–62 (Island Press, 2002).
Gabaldón, C. et al. Repeated flood disturbance enhances rotifer dominance and diversity in a zooplankton community of a small dammed mountain pond. J. Limnol. 76, 13 (2016).
Porcal, P. & Kopáček, J. Photochemical degradation of dissolved organic matter reduces the availability of phosphorus for aquatic primary producers. Chemosphere 193, 1018–1026 (2018).
Macarthur, R. & Levins, R. The limiting similarity, convergence, and divergence of coexisting species. Am. Nat. 101, 377–385 (1967).
Newton, R. J., Kent, A. D., Triplett, E. W. & McMahon, K. D. Microbial community dynamics in a humic lake: differential persistence of common freshwater phylotypes. Environ. Microbiol. 8, 956–970 (2006).
Neuenschwander, S. M., Ghai, R., Pernthaler, J. & Salcher, M. M. Microdiversification in genome-streamlined ubiquitous freshwater Actinobacteria. ISME J. 12, 185–198 (2018).
Cabello-Yeves, P. J. et al. Reconstruction of diverse verrucomicrobial genomes from metagenome datasets of freshwater reservoirs. Front. Microbiol. 8, 2131 (2017).
Reznick, D., Bryant, M. J. & Bashey, F. r- and K-selection revisited: the role of population regulation in life-history evolution. Ecology 83, 1509–1520 (2002).
Mac Arthur, R. H. & Wilson, E. O. The Theory of Island Biogeography (Princeton Univ. Press, 1967).
Šimek, K. et al. A finely tuned symphony of factors modulates the microbial food web of a freshwater reservoir in spring. Limnol. Oceanogr. 59, 1477–1492 (2014).
Logue, J. B., Mouquet, N., Peter, H. & Hillebrand, H. Empirical approaches to metacommunities: a review and comparison with theory. Trends Ecol. Evol. 26, 482–491 (2011).
Shabarova, T. et al. Bacterial community structure and dissolved organic matter in repeatedly flooded subsurface karst water pools. FEMS Microbiol. Ecol. 89, 111–126 (2014).
Shabarova, T., Widmer, F. & Pernthaler, J. Mass effects meet species sorting: transformations of microbial assemblages in epiphreatic subsurface karst water pools. Environ. Microbiol. 15, 2476–2488 (2013).
Jones, S. E. et al. Typhoons initiate predictable change in aquatic bacterial communities. Limnol. Oceanogr. 53, 1319–1326 (2008).
Shade, A. et al. Fundamentals of microbial community resistance and resilience. Front. Microbiol. 3, 417 (2012).
Hahn, M. W. Isolation of strains belonging to the cosmopolitan Polynucleobacter necessarius cluster from freshwater habitats located in three climatic zones. Appl. Environ. Microb. 69, 5248–5254 (2003).
Salcher, M. M., Neuenschwander, S. M., Posch, T. & Pernthaler, J. The ecology of pelagic freshwater methylotrophs assessed by a high-resolution monitoring and isolation campaign. ISME J. 9, 2442–2453 (2015).
Vuono, D. C. et al. Disturbance and temporal partitioning of the activated sludge metacommunity. ISME J. 9, 425–435 (2014).
Shabarova, T. et al. Distribution and ecological preferences of the freshwater lineage LimA (genus Limnohabitans) revealed by a new double hybridization approach. Environ. Microbiol. 19, 1296–1309 (2017).
Hahn, M. W., Lang, E., Tarao, M. & Brandt, U. Polynucleobacter rarus sp. nov., a free-living planktonic bacterium isolated from an acidic lake. Int. J. Syst. Evol. Microbiol. 61, 781–787 (2011).
Hahn, M. W. et al. The passive yet successful way of planktonic life: genomic and experimental analysis of the ecology of a free-living Polynucleobacter population. PLoS ONE 7, e32772 (2012).
Pernthaler, J. Predation on prokaryotes in the water column and its ecological implications. Nat. Rev. Microbiol. 3, 537–546 (2005).
Sommer, U. et al. Beyond the plankton ecology group (Peg) model: mechanisms driving plankton succession. Annu. Rev. Ecol. Evol. Syst. 43, 429–448 (2012).
Šimek, K. et al. Bacterial prey food characteristics modulate community growth response of freshwater bacterivorous flagellates. Limnol. Oceanogr. 63, 484–502 (2018).
Posch, T. et al. Network of interactions between ciliates and phytoplankton during spring. Front. Microbiol. 6, 1289 (2015).
Geraldes, A. M. & Boavida, M.-J. Zooplankton assemblages in two reservoirs: one subjected to accentuated water level fluctuations, the other with more stable water levels. Aquat. Ecol. 41, 273–284 (2007).
Nilssen, J. P. & Wærvågen, S. B. Superficial ecosystem similarities vs autecological stripping: the ‘twin species’ Mesocyclops leuckarti (Claus) and Thermocyclops oithonoides (Sars)—seasonal habitat utilisation and life history traits. J. Limnol. 59, 79–102 (2000).
Cole, T. M. & Wells, S. A. CE-QUAL-W2: A Two-Dimensional, Laterally Averaged, Hydrodynamic and Water Quality Model, Version 4.1 (Department of Civil and Environmental Engineering, 2018).
Brussaard, C. P. D. Optimization of procedures for counting viruses by flow cytometry. Appl. Environ. Microb. 70, 1506–1513 (2004).
Porter, K. G. & Feig, Y. S. The use of DAPI for identifying and counting aquatic microflora. Limnol. Oceanogr. 25, 943–948 (1980).
Sherr, E. B. & Sherr, B. F. in Handbook of Methods in Aquatic Microbial Ecology (eds Kemp, P. F. et al.) 207–212 (Lewis Publishers, 1993).
Sherr, E. B. & Sherr, B. F. in Handbook of Methods in Aquatic Microbial Ecology (eds Kemp, P. F. et al.) 695–701 (Lewis Publishers, 1993).
Kasalický, V., Jezbera, J., Hahn, M. W. & Šimek, K. The diversity of the Limnohabitans genus, an important group of freshwater bacterioplankton, by characterization of 35 isolated strains. PLoS ONE 8, e58209 (2013).
Šimek, K. et al. Microbial food webs in hypertrophic fishponds: omnivorous ciliate taxa are major protistan bacterivores. Limnol. Oceanogr. 64, 2295–2309 (2019).
Lund, J. W. G., Kipling, C. & Le Cren, E. D. The inverted microscope method of estimating algal numbers and the statistical basis of estimations by counting. Hydrobiologia 11, 143–170 (1958).
Hillebrand, H., Dürselen, C. D., Kirschtel, D., Pollingher, U. & Zohary, T. Biovolume calculation for pelagic and benthic microalgae. J. Phycol. 35, 403–424 (1999).
Straškraba, M. & Hrbáček, J. Net-plankton cycle in slapy reservoir during 1958–1960. Hydrobiol. Stud. 1, 113–153 (1966).
Nercessian, O., Noyes, E., Kalyuzhnaya, M. G., Lidstrom, M. E. & Chistoserdova, L. Bacterial populations active in metabolism of C1 compounds in the sediment of Lake Washington, a freshwater lake. Appl. Environ. Microb. 71, 6885–6899 (2005).
Callahan, B. J. et al. DADA2: high-resolution sample inference from Illumina amplicon data. Nat. Methods 13, 581–583 (2016).
R Core Team. R: A Language and Environment for Statistical Computing (R Foundation for Statistical Computing, 2017).
Yilmaz, P. et al. The SILVA and ‘all-species living tree project (LTP)’ taxonomic frameworks. Nucleic Acids Res. 42, D643–D648 (2014).
Quast, C. et al. The SILVA ribosomal RNA gene database project: improved data processing and web-based tools. Nucleic Acids Res. 41, D590–D596 (2012).
Pruesse, E. et al. SILVA: a comprehensive online resource for quality checked and aligned ribosomal RNA sequence data compatible with ARB. Nucleic Acids Res. 35, 7188–7196 (2007).
Stamatakis, A. RAxML version 8: a tool for phylogenetic analysis and post-analysis of large phylogenies. Bioinformatics 30, 1312–1313 (2014).
Schöfl, G. reutils: talk to the NCBI EUtils. R version 0.2.3 https://CRAN.R-project.org/package=reutils (2016).
Pruesse, E., Peplies, J. & Glöckner, F. O. SINA: accurate high-throughput multiple sequence alignment of ribosomal RNA genes. Bioinformatics 28, 1823–1829 (2012).
Ludwig, W. et al. ARB: a software environment for sequence data. Nucleic Acids Res. 32, 1363–1371 (2004).
Fuchs, B. M., Glöckner, F. O., Wulf, J. & Amann, R. Unlabeled helper oligonucleotides increase the in situ accessibility to 16S rRNA of fluorescently labeled oligonucleotide probes. Appl. Environ. Microb. 66, 3603–3607 (2000).
Buckley, D. H. & Schmidt, T. M. Environmental factors influencing the distribution of rRNA from verrucomicrobia in soil. FEMS Microbiol. Ecol. 35, 105–112 (2001).
Yilmaz, L. S., Parnerkar, S. & Noguera, D. R. mathFISH, a web tool that uses thermodynamics-based mathematical models for in silico evaluation of oligonucleotide probes for fluorescence in situ hybridization. Appl. Environ. Microb. 77, 1118–1122 (2011).
Sekar, R. et al. An improved protocol for quantification of freshwater Actinobacteria by fluorescence in situ hybridization. Appl. Environ. Microb. 69, 2928–2935 (2003).
Lorenzen, C. J. Determination of chlorophyll and pheo-pigments: spectrophotometric equations 1. Limnol. Oceanogr. 12, 343–346 (1967).
Golterman, H. L. Methods for Chemical Analysis of Fresh Waters (F. A. Davis Company, 1969).
Murphy, J. & Riley, J. P. A modified single solution method for the determination of phosphate in natural waters. Anal. Chim. Acta 27, 31–36 (1962).
Kopáček, J. & Hejzlar, J. Semi-micro determination of total phosphorus in fresh waters with perchloric acid digestion. Int. J. Environ. Anal. Chem. 53, 173–183 (1993).
Oksanen, J. et al. vegan: community ecology package. R version 2.5–6 (2019); https://CRAN.R-project.org/package=vegan
Acknowledgements
We would like to thank all scientific and technical staff supporting the team during the exhausting but exciting sampling campaign, especially R. Malá, V. Kasalický and H. Kratochvílová. The study was supported by projects from the Czech Science Foundation project 20-23718Y awarded to T.S., project 13-00243S awarded to K.Š., project 19-00113S awarded to P.P. and projects 19-23469S and 20-12496X awarded to M.M.S. H.P.G. was supported by the German Federal Ministry of Education and Science (BMBF) in the frame of the BIBS project (TP2: 01LC1501G).
Author information
Authors and Affiliations
Contributions
T.S., M.M.S. and K.Š. designed the study, and collected and enumerated bacterial and protist samples. T.S. contributed to CARD-FISH analysis, performed bioinformatics analysis of amplicon sequences and statistical analyses of data, interpreted the data, designed the graphs and wrote the manuscript. M.M.S. designed the CARD-FISH probes, contributed to CARD-FISH analysis, prepared the phylogenetic trees and participated in data interpretation and manuscript writing. P.P. collected and analysed chemistry samples, and contributed to interpretation of data and manuscript revision. P.Z. and J.N. collected and analysed samples for phytoplankton and contributed to interpretation of data and manuscript revision. H.P.G. was responsible for the isolation of the nucleic acids and preparation of the amplicon libraries, and participated in data interpretation and manuscript writing. J.S. collected and analysed zooplankton data, and contributed to interpretation of data and manuscript revision. J.H. collected and analysed meteorological and hydrological data, calculated the transfer models and nutrient balances, and contributed to interpretation of data and manuscript revision. K.Š. analysed protist community-related parameters and contributed to interpretation of data and manuscript writing.
Corresponding author
Ethics declarations
Competing interests
The authors declare no competing interests.
Additional information
Peer review information Nature Microbiology thanks Diego Fontaneto and the other, anonymous, reviewer(s) for their contribution to the peer review of this work. Peer reviewer reports are available.
Publisher’s note Springer Nature remains neutral with regard to jurisdictional claims in published maps and institutional affiliations.
Extended data
Extended Data Fig. 1 Agglomerative hierarchical clustering (AHC) based on Bray–Curtis dissimilarity matrix of 16S rDNA amplicon data.
Dashed lines show the level of truncation. Sample names are composed of the date and the first letter of the sampling site (P – pond, S – stream). Samples from the pond are depicted in blue, samples from the stream in orange, samples collected during EREs are shown in red. a, AHC of pond samples. Coloured background areas highlight clusters, which defined four phases of the succession cycle: violet – ‘K’ conservation phase, orange – ‘Ω’ release phase, light green – ‘α’ reorganization phase, and aquamarine – ‘r’ exploitation phase. b, AHC of stream samples. c AHC of all stream and pond samples.
Extended Data Fig. 2 Mass balances of different chemical compounds in Jiřická Pond.
(a–g) Black circles represent values of compounds measured in the pond. Grey surfaces represent theoretical hydrological transport of compounds from the inlet (calculated with CE-QUAL-W2 Hydrodynamic and Water Quality Model using settings for conservative tracer). Dashed lines indicate two flood events observed during the sampling campaign. Precipitation events >3 mm d−1 are depicted in aquamarine above panel a. (a–c) Phosphorus fractions. TP, total phosphorus; DP, dissolved phosphorus; DRP, dissolved reactive phosphorus. (d–f) Nitrogen fractions. DN, dissolved nitrogen. g, Dissolved organic carbon (DOC). (h–n) Daily import and export rates of different compounds. Dotted lines separate three equal periods of 23 days each. Import to the pond is shown in orange, export from the pond in blue. (h–j) Phosphorus fractions. TP, total phosphorus; DP, dissolved phosphorus; DRP, dissolved reactive phosphorus. (k–m) Nitrogen fractions. DN, dissolved nitrogen. n, Dissolved organic carbon (DOC).
Extended Data Fig. 3 Highly abundant ASVs detectable only during conservation phase.
Type K, typical K-strategists. Circles connected with lines represent percentages of 16S rDNA amplicon reads, triangles represent percentages of 16S rRNA amplicons reads. Samples from the pond are depicted in blue, samples from the stream in orange. Background colours indicate different phases of the succession: violet – ‘K’ conservation phase, orange – ‘Ω’ collapse and release phase, light green – ‘α’ reorganization phase, and aquamarine – ‘r’ exploitation phase. Displayed taxonomy is based on naive Bayesian classifier method and trained Silva SSU database release 132 incorporated in the DADA2 pipeline; additionally, if available, 100% identity matches obtained with BLAST are shown in brackets, and CARD-FISH probes matching the ASVs (R-BT065 and opitu-346) are indicated in italics.
Extended Data Fig. 4 Highly abundant ASVs displaying highest read proportions in conservation phases and already detectable in exploitation phases.
Type K (r), fast recovering K-strategists. Circles connected with lines represent percentages of 16S rDNA amplicon reads, triangles represent percentages of 16S rRNA amplicons reads. Samples from the pond are depicted in blue, samples from the stream in orange. Background colours indicate different phases of the succession: violet – ‘K’ conservation phase, orange – ‘Ω’ collapse and release phase, light green – ‘α’ reorganization phase, and aquamarine – ‘r’ exploitation phase. Displayed taxonomy is based on naive Bayesian classifier method and trained Silva SSU database release 132 incorporated in the DADA2 pipeline; additionally, if available, 100% identity matches obtained with BLAST are shown in brackets, and CARD-FISH probes matching the ASVs (R-BT065 and opitu-346) are indicated in italics.
Extended Data Fig. 5 Highly abundant ASVs displaying highest read proportions in EREs or reorganization phases.
Circles connected with lines represent percentages of 16S rDNA amplicon reads, triangles represent percentages of 16S rRNA amplicons reads. Samples from the pond are depicted in blue, samples from the stream in orange. Background colours indicate different phases of the succession: violet – ‘K’ conservation phase, orange – ‘Ω’ collapse and release phase, light green – ‘α’ reorganization phase, and aquamarine – ‘r’ exploitation phase. Displayed taxonomy is based on naive Bayesian classifier method and trained Silva SSU database release 132 incorporated in the DADA2 pipeline; additionally, if available, 100% identity matches obtained with BLAST are shown in brackets, and CARD-FISH probes matching the ASVs (RBT-065 and opitu-346) are indicated in italics. a, Type Ω, ASVs displaying highest read proportions during the EREs (emigrants) that do not belong to the most common pond ASVs. b, Type α, ASVs displaying highest reads proportions during reorganization phase (inlet associated r-strategists).
Extended Data Fig. 6 Highly abundant omnipresent ASVs and ASVs displaying highest read proportions in exploitation phases.
Circles connected with lines represent percentages of 16S rDNA amplicon reads, triangles represent percentages of 16S rRNA amplicons reads. Samples from the pond are depicted in blue, samples from the stream in orange. Background colours indicate different phases of the succession: violet – ‘K’ conservation phase, orange – ‘Ω’ collapse and release phase, light green – ‘α’ reorganization phase, and aquamarine – ‘r’ exploitation phase. Displayed taxonomy is based on naive Bayesian classifier method and trained Silva SSU database release 132 incorporated in the DADA2 pipeline; additionally, if available, 100% identity matches obtained with BLAST are shown in brackets, and CARD-FISH probes matching the ASVs (R-BT065 and opitu-346) are indicated in italics. a: Type r, ASVs displaying highest reads proportions during exploitation phase (pond associated r-strategists). b: Type all-rounders, ASVs displaying comparable read proportions during all phases except EREs.
Extended Data Fig. 7 Changes in different size fractions of algae in the context of ciliate dynamics and contributions to the total bacterial loss.
a, b, Abundances and biovolumes of algal cells <600 µm3 and >600 µm3, respectively (that is, suitable or not suitable for ciliate grazing). c, Abundances of ciliates and the most dynamic fraction of algae <150 µm3 (represented mostly by small Chlamydomonas spp.). d, Total bacterial loss rate and HNF and ciliates grazing rates (GR). The dashed lines indicate the two EREs.
Extended Data Fig. 8 Hydrological, physical, chemical and microbial parameters measured in the stream and pond.
Extended version of Fig. 1. a, b, Precipitation (grey shade) in the watershed of Jiřícká Pond, stream flow rate (a) and water retention time at 0.5 m depth in the pond (b). c, d, Water temperature and pH. e, f, Concentrations of phosphorus fractions, TP – total phosphorus, DP – dissolved phosphorus, DRP – dissolved reactive phosphorus. g, h, Concentrations of nitrogen fractions, DN – dissolved nitrogen. i, j, Concentrations of dissolved organic carbon (DOC) and DOC size fractions. k, l, Concentrations of carboxylic acids (CA). m, n, Abundances of prokaryotic cells and virus-like particles. Prokaryotic abundances counted from two independent biological samples are presented as circles. Dashed lines indicate two flood events during the sampling campaign.
Extended Data Fig. 9 Bootstrapped maximum likelihood tree of 16S rRNA gene sequences of the closest relatives of the 50 most abundant ASVs detected in stream and pond samples and most abundant EREs genotypes.
The colours of the branches and ASVs correspond to the source: orange – stream, blue – pond, red – EREs, orange with red line – stream and EREs. ASVs are depicted as circles with different diameters representing the maximal percentages of corresponding reads, ASVs shared between pond and stream are shown as divided circles. Asterisks indicate ASVs with low identity values to the closest relatives (90–97%). Specific CARD-FISH probes are shown in italics. Bootstraps (100 repetitions) are indicated by different sized circles at the nodes, the scale bar applies to 20% sequence divergence.
Extended Data Fig. 10 Dynamics in stream microbial community.
a, Multidimensional scaling based on Bray–Curtis distances of bacterial community compositions in the stream samples (16S rRNA gene amplicons), Kruskal’s stress: 0.2; (start) and (end) indicate the first and last samples, respectively. The line connecting samples follows a chronological order. The diameters of the circles are proportional to the Pielou’s evenness of the samples (varying between 0.71 and 0.94). Orange circles indicate samples taken during the EREs. b, Pie charts depict the contribution of large taxonomical groups and follow the chronological order from left to right. The samples associated with EREs are highlighted with orange frames. *Gammaproteobacteria are shown without Betaproteobacteriales, which are presented as a separate group.
Supplementary information
Supplementary Information
Supplementary Figs. 1–8, Tables 1 and 2, Note and references.
Source data
Source Data Fig. 1
Statistical source data.
Source Data Fig. 2
Statistical source data.
Source Data Fig. 3
Statistical source data.
Source Data Fig. 4
Statistical source data.
Source Data Extended Data Fig. 1
Statistical source data.
Source Data Extended Data Fig. 2
Statistical source data.
Source Data Extended Data Fig. 3
Statistical source data.
Source Data Extended Data Fig. 4
Statistical source data.
Source Data Extended Data Fig. 5
Statistical source data.
Source Data Extended Data Fig. 6
Statistical source data.
Source Data Extended Data Fig. 7
Statistical source data.
Source Data Extended Data Fig. 8
Statistical source data.
Source Data Extended Data Fig. 10
Statistical source data.
Rights and permissions
About this article
Cite this article
Shabarova, T., Salcher, M.M., Porcal, P. et al. Recovery of freshwater microbial communities after extreme rain events is mediated by cyclic succession. Nat Microbiol 6, 479–488 (2021). https://doi.org/10.1038/s41564-020-00852-1
Received:
Accepted:
Published:
Issue Date:
DOI: https://doi.org/10.1038/s41564-020-00852-1
This article is cited by
-
Phenology and ecological role of aerobic anoxygenic phototrophs in freshwaters
Microbiome (2024)
-
Microbes and the water nexus
Nature Microbiology (2024)
-
High-resolution metagenomic reconstruction of the freshwater spring bloom
Microbiome (2023)
-
In the right place, at the right time: the integration of bacteria into the Plankton Ecology Group model
Microbiome (2023)
-
Environmental and Anthropogenic Factors Shape the Skin Bacterial Communities of a Semi-Arid Amphibian Species
Microbial Ecology (2023)