Abstract
Late-life depression (LLD) is a heterogenous mood disorder influenced by genetic factors. Cortical physiological processes such as cortical inhibition, facilitation, and plasticity may be markers of illness that are more strongly associated with genetic factors than the clinical phenotype. Thus, exploring the relationship between genetic factors and these physiological processes may help to characterize the biological mechanisms underlying LLD and improve diagnosis and treatment selection. Transcranial magnetic stimulation (TMS) combined with electromyography was used to measure short interval intracortical inhibition (SICI), cortical silent period (CSP), intracortical facilitation (ICF), and paired associative stimulation (PAS) in 79 participants with LLD. We used exploratory genome-wide association and gene-based analyses to assess for genetic correlations of these TMS measures. MARK4 (which encodes microtubule affinity-regulating kinase 4) and PPP1R37 (which encodes protein phosphatase 1 regulatory subunit 37) showed genome-wide significant association with SICI. EGFLAM (which encodes EGF-like fibronectin type III and laminin G domain) showed genome-wide significant association with CSP. No genes met genome-wide significant association with ICF or PAS. We observed genetic influences on cortical inhibition in older adults with LLD. Replication with larger sample sizes, exploration of clinical phenotype subgroups, and functional analysis of relevant genotypes is warranted to better characterize genetic influences on cortical physiology in LLD. This work is needed to determine whether cortical inhibition may serve as a biomarker to improve diagnostic precision and guide treatment selection in LLD.
Similar content being viewed by others
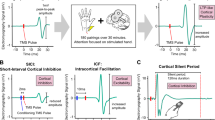
Introduction
Depression is a multifactorial disease influenced by both genetic and non-genetic factors [1]. In late-life, depression affects approximately 15% of older adults living in the community [2]. Aging and depression are both associated with changes in cortical physiology, such as cortical inhibition, excitation, and plasticity [3]. Exploring the relationship between genetic factors and cortical physiological processes could improve our understanding of biological mechanisms underlying late-life depression (LLD), leading to improved diagnosis and selection of treatment (i.e., biomarker-informed precision psychiatry).
Twin studies suggest that 16–55% of the variance in depressive symptoms in older adults may be due to genetic influences, and heritability may be greater with increased age [4, 5]. However, there has been limited success in identifying causal genetic loci for LLD [6]. In a meta-analysis, polymorphisms in apolipoprotein E (APOE), brain-derived neurotrophic factor (BDNF), and serotonin transporter (SLC6A4) genes were associated with LLD [6]. Genome-wide association studies (GWAS) of LLD have identified gene variants associated with cognitive decline and antidepressant response [7, 8]. However, environmental, epigenetic, and polygenic interactions make gene-depression signals difficult to detect and interpret [9].
Neural plasticity and cortical physiology may be potential pathways by which genes influence LLD. Transcranial magnetic stimulation (TMS) can be used to assess various neurophysiological measures. Short interval intracortical inhibition (SICI) measures the suppression of motor-evoked potential (MEP) amplitude when a suprathreshold TMS pulse is pre-conditioned by 1–6 ms with a subthreshold TMS pulse, which is indicative of GABAA inhibitory activity [10]. The cortical silent period (CSP) measures the duration of electromyography suppression when a suprathreshold TMS pulse is given during a sustained muscle contraction, which is indicative of GABAB inhibitory activity [11]. The resting motor threshold (RMT) is a general measure of neuronal excitability, which is influenced by ion channel conductivity [12]. Intracortical facilitation (ICF) measures relative MEP amplitude when a subthreshold TMS pulse precedes a suprathreshold TMS pulse by 10–15 ms, which is indicative of N-methyl-D-aspartate (NMDA) receptor-related excitatory activity [12]. Paired associative stimulation (PAS) refers to repeated median nerve stimulation preceding TMS stimulation and subsequent measurement of MEPs in a target muscle [13]. An interstimulus interval of approximately 25 ms causes an excitatory effect, and changes in subsequent MEP measurements indicate long-term potentiation (LTP) like cortical plasticity [13].
The interaction among depression, aging, and genetics in the pathophysiology of LLD remain unclear. Depression and advancing age have both been related to changes in cortical physiology. Attenuation in GABAA receptor-mediated cortical inhibition has been demonstrated in patients with LLD, healthy older adults, and younger adults with depression [3]. Decreases in cortical excitability and impaired LTP-like plasticity have been shown in healthy older adults and patients with major depressive disorder (MDD) [14,15,16]. Existing GWAS and candidate gene studies have assessed genetic correlations of various proxy phenotypes of aging, including longevity, age-associated diseases, and physiological characteristics, such as muscle strength and cognitive function [17]. Several genes have been associated with aging, including APOE, GRP78, and FOXO3A [17]. Aging-related genes may influence measures of cortical physiology, as changes in cortical physiology have been observed in healthy older adults [14,15,16].
Few existing studies have assessed the association of genetic polymorphisms with cortical physiological processes measured by TMS. The BDNF gene encodes a protein that has been implicated in neuronal survival, neuroplasticity, and synaptogenesis [18]. Substitution of valine to methionine at codon 66 (Val66Met) is the most common and well-researched single nucleotide polymorphism (SNP) of BDNF [18]. Multiple studies suggest that there are no differences in SICI, CSP, ICF, or RMT dependent on the Val66Met SNP in healthy participants, though this has not been explored in LLD [19,20,21,22]. A few studies report that an increase in post-PAS MEP amplitudes is diminished in those with the Val66Met SNP [23,24,25]. Even fewer studies have assessed the impact of other SNPs on TMS indices of cortical physiology, and none that are specific to LLD [26,27,28,29].
Thus, we conducted the first exploratory GWAS of TMS cortical physiological processes in LLD. Based on the literature reviewed above, we hypothesized that BDNF polymorphisms would be associated with cortical physiology. Second, based on extensive literature on the relationship between neurotransmitters and depression [1, 30], we hypothesized that variations in genes encoding for serotonin [31, 32], norepinephrine [33], dopamine [34, 35], GABA [36, 37], and glutamate receptors and transporters [36, 37] would be associated with cortical physiology changes in LLD.
Methods
Participants
Participants were recruited from the Toronto site of two multi-center clinical trials (ClinicalTrials.gov Identifiers: NCT00892047 and NCT02263248). Participants completed TMS and genetic assessment prior to receiving any treatment intervention in the trials. Informed consent was obtained from all subjects. As described in details previously [38, 39], the main inclusion criteria were: age ≥ 50 (although most participants were 60 years and older), current diagnosis of MDD as per the Diagnostic and Statistical Manual of Mental Disorders, Fourth Edition, and Montgomery-Åsberg Depression Rating Scale (MADRS) [40] score ≥15. Exclusion criteria were a diagnosis of dementia; Mini-Mental State Examination (MMSE) score ≤21; a diagnosis of bipolar disorder or a psychotic disorder; substance misuse; an unstable medical condition; and anticonvulsant use. The sample for this analysis comprised 79 participants whose data (clinical, TMS neurophysiology, and genetic) passed quality control.
TMS and electromyography measures
All TMS measures were conducted in accordance with international consensus guidelines [41]. TMS was delivered to the left motor cortex through a figure-of-eight coil (70 mm loop diameter) using two Magstim 200 stimulators (Magstim, Whitland, UK) connected by a Bistim module. Participants were asked to remain relaxed with their eyes open. Disposable 9 mm electrodes were attached to the abductor pollicis brevis muscle (active electrode) and the interphalangeal joint of the thumb (reference electrode) to measure surface electromyography (EMG). A ground electrode was positioned on the upper forearm. The figure-of-eight coil was held tangentially to the scalp over the left motor cortex “hot spot” to evoke maximum MEP strength, with the handle pointing 45 degrees from the midline to induce a posterior-to-anterior current flow. This position was marked on the scalp to ensure consistent coil placement.
RMT was defined as the lowest TMS intensity that elicited an MEP ≥ 50 μV in at least 5 of 10 consecutive trials. When assessing SICI, ICF, and PAS, a suprathreshold TMS test pulse was defined as the TMS intensity that evoked on average a ~1 mV peak-to-peak MEP amplitude. SICI and ICF were measured using paired-pulse TMS with a subthreshold conditioning stimulus (80% of RMT) followed by a test pulse with an interstimulus interval (ISI) of 2 ms (SICI) or 10 ms (ICF). Participants underwent 12 trials of test pulse alone (to determine the average unconditioned MEP amplitude), 12 trials of test pulse 2 ms after the conditioning pulse, and 12 trials of test pulse 10 ms after the conditioning pulse. SICI and ICF ratios were calculated as the average respective conditioned MEP amplitudes divided by the average unconditioned MEP amplitudes.
CSP was measured using a suprathreshold pulse (140% of RMT) delivered while participants maintained a voluntary isometric contraction of the abductor pollicis brevis muscle at approximately 20% of maximum contraction. The resulting silent period duration from MEP onset to return of EMG activity was averaged across 10 trials.
PAS was assessed using stimulation of the right median nerve paired with a suprathreshold TMS test pulse at an ISI of 25 ms. One hundred and eighty pairs of stimuli were delivered at 0.1 Hz. Twenty TMS pulses at 0.1 Hz were delivered before PAS and at 0-, 15-, 30-, and 60-minutes post-PAS, and resulting MEP amplitudes were measured. Ratios of average and maximum post-PAS MEP amplitudes to pre-PAS MEP amplitudes were calculated. Participants were asked to count the number of peripheral nerve stimulations on the target hand during PAS, as attention affects PAS-induced plasticity. This count was recorded randomly 8 times during the assessment of PAS and used to determine an inattention score.
Genotyping and gene-based analyses
Genome-wide genotyping was performed using the Illumina PsychArray Beadchip v1.3 endorsed by the Psychiatric Genomics Consortium for participants from both trials. We applied to samples from both trials the quality control (QC) procedure and imputation pipeline reported previously in the first trial [42]. In the second trial, QC was also performed following the standard protocol by Anderson et al. [43] and two participants were removed due to excessive heterozygosity and genotyping missingness. 3827 variants were removed due to missingness, and 266,057 variants with <1% minor allele frequency (MAF) were excluded. 301,495 variants and 34 participants in the second trial entered genome-wide imputation. According to the genipe pipeline, prephasing was first conducted using SHAPEIT2 and 1000 Genomes Phase 3 reference panel, followed by imputation using IMPUTE v2.2 in 5-Mb segments per chromosome [44,45,46,47]. The final data were imputed with an information threshold of ≥0.7, a completion rate of ≥0.95, and an imputation probability threshold ≥0.9, resulting in 5,067,675 SNPs after imputation. Out of the final imputed variants, 3,882,161 variants (76.6%) with a MAF ≥ 0.05 were used in the analyses described below. After checking the availability of genetic and TMS data, 45 participants from the first trial and 4,309,635 variants were merged with imputed samples from the second trial. The final merged data file contained 79 participants and 3,331,137 variants.
Genome-wide association analysis was conducted on the merged data using PLINK v2.0 [48]. We fitted a linear regression model for each of the phenotypes (SICI at 2 ms, ICF at 10 ms, maximum PAS ratio, average PAS ratio, RMT, and CSP), adjusted for age, MADRS baseline score, and the first three principal components of ancestry inference. Sensitivity analyses were conducted that included sex as an additional covariate. GWAS summary statistics were then utilized to conduct MAGMA gene-based analyses using FUMA with default parameters [49, 50]. Using the 1000 Genome Project Phase 3 (2504 individuals, approximately 84 million SNPs) as the reference panel, 3,331,137 SNPs were assigned to 18,580 genes within a 10 kb window [46]. The genome-wide significance level after correcting for the number of genes was 0.05/18,580 = 2.691 × 10−6. In MAGMA analyses implanted in FUMA, the gene-based Z-score is calculated based on a SNP-wide mean model and we used the Z-score to infer the direction of gene association. To check our hypothesis about the association between the BDNF gene and cortical physiological changes in LLD, we looked for BDNF among the MAGMA results. After MAGMA gene-based analyses, we conducted gene-set enrichment analysis for SICI and CSP, which had significantly associated genes. We used FUMA with default settings and background genes mapped by MAGMA to determine whether there was any overlap between the top 20 associated genes and pre-defined gene sets relevant to a specific phenotype.
Results
See Table 1 for the sociodemographic and clinical characteristics of the 79 participants included in the GWAS analyses and the subsequent gene-based analyses.
RMT
In the 79 participants with RMT data, among the mapped 18,580 genes, none of the 1154 nominally associated genes at the threshold of p < 0.05 reached genome-wide significance (Supplementary Fig. 1). The top association was the MRPS31 gene (Mitochondrial Ribosomal Protein S31, Z = 4.19, p = 1.41 × 10−5; Supplementary Table 1).
SICI
In the 78 participants with SICI data, MARK4 (Microtubule Affinity Regulating Kinase 4, Z = 4.77, p = 9.35 × 10−7) and PPP1R37 (Protein Phosphatase 1 Regulatory Subunit 37, Z = 4.71, p = 1.22 × 10−6) were positively associated with the SICI ratio with genome-wide significance (Fig. 1, Table 2). Among the independent SNPs assigned to MARK4 and PPP1R37, the top associated SNP was rs75918199 in chromosome 19 (β = 0.50 [0.30, 0.71], p = 8.66 × 10−6). 1122 genes were nominally associated with the phenotype (p < 0.05). Among the top 20 associated genes, the gene-set enrichment analysis showed that the GWAS catalog gene-set of Alzheimer’s disease or pleiotropy had 4 genes (GEMIN7, MARK4, PPP1R37, and NKPD1) overlapped (p = 1.74 × 10−7, adjusted-p = 3.16 × 10−4; Supplementary Table 5).
A Gene Manhattan plot. B Gene QQ (quantile-quantile) plot. Note: The dotted red line in (A) is the genome-wide significant threshold p = 2.691 × 10−6. MARK4 Microtubule Affinity Regulating Kinase 4, PPP1R37 Protein Phosphatase 1 Regulatory Subunit 37, GEMIN7 Gem Nuclear Organelle Associated Protein 7, C11orf21 Chromosome 11 Open Reading Frame 21, MARK2 Microtubule Affinity Regulating Kinase 1, RCOR2 REST Corepressor 2, TRA2B Transformer 2 Beta Homolog, NKPD1 NTPase KAP Family P-Loop Domain Containing 1, KRBA1 KRAB-A Domain Containing 1, TARDBP TAR DNA Binding Protein.
CSP
In 76 participants with CSP data, EGFLAM (EGF-like Fibronectin Type III and Laminin G Domains, Z = 4.94, p = 3.82 × 10−7) was positively associated with CSP with genome-wide significance (Fig. 2, Table 3). Among the independent SNPs assigned to EGFLAM, the top associated SNP was rs2561139 in chromosome 5 (β = −0.02 [−0.03, −0.01], p = 7.85 × 10−6). 1126 genes were nominally associated with the phenotype (p < 0.05). None of the top 20 associated genes were overrepresented in the pre-defined GWAS catalog reported gene sets.
A Gene Manhattan plot. B Gene QQ (quantile-quantile) plot. Note: The dotted red line in (A) is the genome-wide significant threshold p = 2.691 × 10−6. EGFLAM EGF Like - Fibronectin Type III and Laminin G Domains, FHL2 Four And A Half LIM Domains 2, SPTB Spectrin Beta Erythrocytic, PCK1 Phosphoenolpyruvate Carboxykinase 1, NOTCH4 Notch Receptor 4, AOX1 Aldehyde Oxidase 1, C17orf103 N-Acetyltransferase Domain Containing 1, GNG11 G Protein Subunit Gamma 11, TNFAIP6 TNF Alpha Induced Protein 6, FRYL FRY Like Transcription Coactivator.
ICF
In the 77 participants with ICF data, none of the 11,161 nominally associated genes reached genome-wide significance (Supplementary Fig. 2). The top association was SMIM17 gene (Small Integral Membrane Protein 17, Z = 4.30, p = 8.65 × 10−6; Supplementary Table 2).
Average PAS ratio
In the 63 participants with a PAS ratio, none of the 1067 nominally associated genes reached genome-wide significance (Supplementary Fig. 3). The top association was the TRIM58 gene (Tripartite Motif Containing 58, Z = 3.72, p = 9.95 × 10−5; Supplementary Table 3).
Maximum PAS ratio
In the 63 participants with a PAS ratio, none of the 1107 nominally associated genes reached genome-wide significance (Supplementary Fig. 4). The top association was the PRKAG3 gene (Protein Kinase AMP-Activated Non-Catalytic Subunit Gamma 3, Z = 3.99, p = 3.37 × 10−5; Supplementary Table 4).
Sensitivity Analyses
With sex as an additional covariate, PPP1R37 was not significantly associated with SICI (Z = 4.53, p = 2.90 × 10−6). MARK4 remained associated with SICI (Z = 4.58, p = 2.38 × 10−6) and EGFLAM remained associated with CSP (Z = 4.94, p = 3.99 × 10−7) with genome-wide significance. There were no other significant associations.
Discussion
We used exploratory GWAS and gene-based analyses to assess the association between genes and TMS measures of cortical inhibition, facilitation, or LTP-like plasticity in LLD. We had three key findings. First, MARK4 (which encodes microtubule affinity-regulating kinase 4) and PPP1R37 (which encodes protein phosphatase 1 regulatory subunit 37) showed genome-wide significant associations with SICI, an indirect measure of GABAA receptor-mediated cortical inhibition. Second, EGFLAM (which encodes EGF-like fibronectin type III and laminin G domains) showed genome-wide significant association with CSP, an indirect measure of GABAB receptor-mediated cortical inhibition. Third, no genes showed a genome-wide significant association with RMT, ICF, or PAS. These findings suggest there may be genetic influences on cortical inhibition in older adults with LLD. Further research is indicated to determine whether cortical inhibition may serve as a biomarker to improve diagnostic precision and treatment selection in this patient population.
SICI is believed to be an indirect measure of GABAA inhibitory activity [10]. MARK4 and PPP1R37 were both associated with decreased SICI in our sample. MARK4 encodes a protein that may be involved in regulation of microtubule networks in neurons [51]. Increased expression of MARK4 has been shown in Alzheimer’s disease (AD), and in vitro studies suggest MARK4 can potentiate tau aggregation [51]. Based on gene enrichment testing in FUMA, both MARK4 and PPP1R37 have been reported in “Alzheimer’s disease or HDL levels” in the GWAS catalog (see Supplementary Fig. 6). A de novo mutation of MARK4 has been associated with the production of abnormally phosphorylated tau [51]. In vivo studies in mice suggest tau expression may be associated with synaptic plasticity deficits [52]. Elevated tau has also been associated with depression, and recent literature suggests that LLD has a different distribution of tau compared to AD [53, 54]. In theory, tau aggregation could inhibit activity of GABAergic neurons, like its effects on hippocampal neurons in AD [55]. Furthermore, MARK4 has also been shown to be upregulated in ischemic axonal injury [56]. Cerebrovascular disease (CVD) may also contribute to LLD, and CVD has also been linked to AD [57, 58].
PPP1R37 encodes for a protein believed to inhibit phosphatase activity of protein phosphatase 1 (PP1) complexes [59]. Limited literature exists on the function of this protein. A transcriptome-wide analysis found an association of differential expression of PPP1R37 in hippocampal tissue with AD compared to controls [60]. Post-mortem studies of patients with AD suggest that PP1 is involved in regulation of tau dephosphorylation [61]. Experiments in mice suggest PP1 activity mediates effects of β-amyloid on synaptic plasticity in AD and PP1 has a role in long-term depression of neuronal activity [61]. Thus, variance in expression of PPP1R37 could lead to aberrant phosphorylation of neurotoxic aggregates, with subsequent effects on GABAergic function. Of note, PPP1R37 was not significantly associated with SICI in sensitivity analyses including sex as an additional covariate. Sex was not included as a covariate in the primary analyses due to limited sample size, however, there is one existing study that suggests biological males may have reduced SICI compared to females [62]. More studies are needed to characterize the role of sex in relation to depression and cortical physiology changes.
Existing literature reports a significant association between LLD and AD, with LLD being a risk factor or prodrome for AD [63]. Several biological mechanisms may overlap between depression and AD, including: inflammatory changes, CVD, decreased neurotrophic factors, and deposits of β-amyloid plaques [63]. The potential associations of both MARK4 and PPP1R37 with AD-related processes is supportive of a link between LLD and AD. The age-by-disease interaction hypothesis of LLD suggests age-related changes in gene expression in the brain may increase susceptibility to multiple neurodegenerative disorders simultaneously [64]. A previous study showed that deficits in cortical inhibition measured with SICI can occur with aging, independent of depression status [3]. Given that the present study is a cross-sectional analysis of cortical physiology in LLD and there is no age-matched control group, we cannot assess whether the potential effects of MARK4 and PPP1R37 are related to depression, aging, or both.
CSP is believed to be an indirect measure of GABAB inhibitory activity [11]. EGFLAM was associated with increased CSP in our sample. EGFLAM encodes a protein believed to enable calcium ion and glycosaminoglycan binding activity [65]. Previous GWAS studies suggest EGFLAM is associated with mathematical ability, educational attainment, and SSRI response [66,67,68]. There are no prior studies that have investigated the effect of EGFLAM on TMS cortical physiology measures. More studies are needed to clarify if EGFLAM is associated with cortical physiology.
Identifying predictors of response is important to optimize treatment selection for LLD. In a previous study, a combination of TMS measures of cortical excitability, with clinical and demographic data predicted response to venlafaxine treatment in LLD with 73% accuracy [69]. The combination of TMS measures with additional biomarkers, such as genetic data, may be useful for the development of models with higher accuracy. As cortical physiological processes are influenced by both neurostimulation and pharmacotherapy, further investigation to characterize the genetic determinants of cortical physiology in LLD may help identify genetic biomarkers of treatment response.
In our study, BDNF did not show a genome-wide significant association with SICI, CSP, ICF, RMT, or PAS. The association of BDNF genotype with TMS cortical physiology measures has been previously investigated. In a few studies in healthy participants, there were no differences in SICI, ICF, CSP, or RMT based on BDNF genotype [19,20,21,22]. In three small studies in young healthy participants (N = 18–32), there was an increase in post-PAS MEP amplitudes in those with the BDNF valine/valine genotype, but not in those with the Val66Met SNP [23,24,25]. One case-control study found a significant interaction between BDNF genotype and catechol-O-methyltransferase genotype in PAS response, suggesting there are likely polygenic determinants of LTP-like plasticity [70]. A case-control study of 23 participants with MDD matched to 23 healthy controls reported greater post-PAS MEP amplitudes in healthy controls than participants with depression, though there was no statistically significant effect of BDNF genotype on response to PAS [71]. There are also contradictory reports on the effect of the Val66Met SNP on TMS cortical physiology measures in response to interventions such as motor training, intermittent theta-burst stimulation, and transcranial direct current stimulation [19,20,21,22]. In light of our findings, based on lack of agreement in studies, more studies are needed to clarify whether BDNF is associated with cortical physiology in younger and older adults with and without depression.
A limited number of prior studies have assessed the association of other genes with TMS cortical physiology measures. A randomized crossover trial of 92 healthy participants found no effect of the SCN1A rs3812717 polymorphism on SICI, ICF, CSP, or RMT, however, the SCNIA genotype modulated the effect of carbamazepine on CSP [26]. A cross-sectional analysis of 77 healthy participants found two common TRPV1 SNPs do not have an effect on SICI, ICF, CSP, or RMT [27]. A small randomized crossover trial in children with Attention-deficit/hyperactivity disorder (ADHD) found that genetic variation in the dopamine transporter (DAT1) was not associated with baseline differences in SICI or ICF, but DAT1 genotype influenced the effect of stimulant administration on these measures [72]. A small exploratory study of eight healthy participants suggested allelic variation in the serotonin transporter promoter (5-HTTLPR) is associated with differential cortical excitability at baseline or in response to citalopram administration [28]. In addition, a case-control study in 24 participants with mild cognitive impairment compared to 24 age-matched controls found that APOE genotype was not correlated with response to PAS [29]. There are no prior studies that have investigated the effect of genes on TMS cortical physiology measures in LLD specifically. In our study, SCN1A, TRPV1, DAT1, and APOE did not show an association with TMS cortical physiology measures, and more studies are needed to clarify whether these genes are associated with cortical physiology processes.
Some strengths of our analysis include comprehensive clinical characterization of the patient sample and integration of several biological measures related to genome-wide genotyping and TMS neurophysiology. LLD is a heterogenous disorder in relation to pathophysiology, clinical phenotype, and intervention response. Recent literature suggests the importance of transdisciplinary approaches to research in psychiatry, as biological systems rarely act in isolation [73]. Comprehensive clinical and biological characterization of patients receiving treatment for LLD is needed given the complexity of depression and aging.
There were also several limitations in our analysis. First and foremost, our sample size was small, increasing the risk of type II error and potentially preventing us from finding true associations. Second, our results were specific to the motor cortex, and it is unclear how they would relate to other cortical regions. Future studies should assess genetic correlations of TMS measures in the dorsolateral prefrontal cortex directly, using TMS-EEG [74]. We were unable to assess for epistasis, that has been shown to have salient impacts on depression phenotypes [75, 76]. We were also unable to assess for causal associations of genes with the TMS processes, as this would require functional characterization of genotypes. As there is no control group in the analysis, we are unable to distinguish whether the identified SNPs are more relevant to depression or aging.
Furthermore, additional covariates that may impact cortical physiology, such as prescribed medications and comorbidities, were not accounted for in this analysis. Limited evidence suggests that single doses of specific serotonin reuptake inhibitors and norepinephrine reuptake inhibitors may have transient effects on cortical physiology [31,32,33]. Several studies suggest that administration of dopamine agonists and antagonists can modulate cortical inhibition, facilitation, and plasticity [34, 35, 77]. The impacts of other psychotropic classes, treatment duration, and medication interactions on cortical physiology have not been well-characterized. In addition, medical comorbidities are relatively common in older adults. Prior studies show that following stroke there may be reduced SICI, which may persist throughout the recovery course [78, 79]. The impact of other comorbidities, such as cerebrovascular disease or other neurological conditions, on cortical physiology is not well-defined. More studies are needed to elucidate the role of medications and comorbidities in cortical physiology.
In conclusion, our results suggest there may be genetic influences on cortical physiology in LLD. MARK4 and PPP1R37 (two genes that have been linked to AD), and EGFLAM, may influence cortical inhibition in LLD. Replication with larger sample sizes and functional analyses of relevant SNPs is needed to determine possible causal associations of genes with TMS measures. With an increasing number of treatment options available for LLD, it is important to characterize the influences of genetics on cortical neurophysiology. This could facilitate optimization of treatment selection and advancement of personalized medicine for LLD.
References
Shao X, Zhu G. Associations among monoamine neurotransmitter pathways, personality traits, and major depressive disorder. Front Psychiatry. 2020;11:381.
Blazer DG. Depression in late life: review and commentary. J Gerontol A Biol Sci Med Sci. 2003;58:M249–65.
Lissemore JI, Bhandari A, Mulsant BH, Lenze EJ, Reynolds CF, Karp JF, et al. Reduced GABAergic cortical inhibition in aging and depression. Neuropsychopharmacology 2018;43:2277–84.
Gatz M, Pederson NL, Plomin R, Nesselroade JR. Importance of shared genes and shared environments for symptoms of depression in older adults. J Abnorm Psychol. 1992;101:701–8.
Carmelli D, Swan GE, Kelly-Hayes M, Wolf PA, Reed T, Miller B. Longitudinal changes in the contribution of genetic and environmental influences to symptoms of depression in older male twins. Psychol Aging. 2000;15:505–10.
Tsang RSM, Mather KA, Sachdev PS, Reppermund S. Systematic review and meta-analysis of genetic studies of late-life depression. Neurosci Biobehav Rev. 2017;75:129–39.
Eyre HA, Eskin A, Nelson SF, St Cyr NM, Siddarth P, Baune BT, et al. Genomic predictors of remission to antidepressant treatment in geriatric depression using genome-wide expression analyses: a pilot study. Int J Geriatr Psychiatry. 2016;31:510–7.
Steffens DC, Garrett ME, Soldano KL, McQuoid DR, Ashley-Koch AE, Potter GG. Genome-wide screen to identify genetic loci associated with cognitive decline in late-life depression. Int Psychogeriatr. 2020;1–9.
Mullins N, Lewis CM. Genetics of depression: progress at last. Curr Psychiatry Rep. 2017;19:43.
Kujirai T, Caramia MD, Rothwell JC, Day BL, Thompson PD, Ferbert A, et al. Corticocortical inhibition in human motor cortex. J Physiol. 1993;471:501–19.
Cantello R, Gianelli M, Civardi C, Mutani R. Magnetic brain stimulation: the silent period after the motor evoked potential. Neurology 1992;42:1951–9.
Paulus W. State of the art: pharmacologic effects on cortical excitability measures tested by transcranial magnetic stimulation. Brain Stimul. 2008;1:151–63.
Wolters A, Sandbrink F, Schlottmann A, Kunesch E, Stefan K, Cohen LG, et al. A temporally asymmetric Hebbian rule governing plasticity in the human motor cortex. J Neurophysiol. 2003;89:2339–45.
Cantone M, Bramanti A, Lanza G, Pennisi M, Bramanti P, Pennisi G, et al. Cortical plasticity in depression. ASN Neuro. 2017;9:1–15.
Cueva AS, Galhardoni R, Cury RG, Parravano DC, Correa G, Araujo H, et al. Normative data of cortical excitability measurements obtained by transcranial magnetic stimulation in healthy subjects. Neurophysiol Clin. 2016;46:43–51.
Tecchio F, Zappasodi F, Pasqualetti P, Gennaro LD, Pellicciari MC, Ercolani M, et al. Age dependence of primary motor cortex plasticity induced by paired associative stimulation. Clin Neurophysiol. 2008;119:675–82.
Melzer D, Pilling LC, Ferrucci L. The genetics of human ageing. Nat Rev Genet. 2020;21:88–101.
Levy MJF, Boulle F, Steinbusch HW, van den Hove DLA, Kenis G, Lanfumey L. Neurotrophic factors and neuroplasticity pathways in the pathophysiology and treatment of depression. Psychopharmacology. 2018;235:2195–220.
Morin-Moncet O, Latulipe-Loiselle A, Therrien-Blanchet JM, Theoret H. BDNF Val66Met polymorphism is associated with altered activity-dependent modulation of short-interval intracortical inhibition in bilateral M1. PLoS ONE. 2018;13:e0197505.
Lee M, Kim SE, Kim WS, Lee J, Yoo HK, Park KD, et al. Interaction of motor training and intermittent theta burst stimulation in modulating motor cortical plasticity: influence of BDNF Val66Met polymorphism. Chen R, editor PLoS ONE. 2013;8:e57690.
Di Lazzaro V, Manganelli F, Dileone M, Notturno F, Esposito M, Capasso M, et al. The effects of prolonged cathodal direct current stimulation on the excitatory and inhibitory circuits of the ipsilateral and contralateral motor cortex. J Neural Transm. 2012;119:1499–506.
Frazer A, Williams J, Spittles M, Rantalainen T, Kidgell D. Anodal transcranial direct current stimulation of the motor cortex increases cortical voluntary activation and neural plasticity. Muscle Nerve. 2016;54:903–13.
Cirillo J, Hughes J, Ridding M, Thomas PQ, Semmler JG. Differential modulation of motor cortex excitability in BDNF Met allele carriers following experimentally induced and use-dependent plasticity. Eur J Neurosci. 2012;36:2640–9.
Cheeran B, Talelli P, Mori F, Koch G, Suppa A, Edwards M, et al. A common polymorphism in the brain-derived neurotrophic factor gene (BDNF) modulates human cortical plasticity and the response to rTMS. J Physiol. 2008;586:5717–25.
Missitzi J, Gentner R, Geladas N, Politis P, Karandreas N, Classen J, et al. Plasticity in human motor cortex is in part genetically determined. J Physiol. 2011;589:297–306.
Menzler K, Hermsen A, Balkenhol K, Duddek C, Bugiel H, Bauer S, et al. A common SCN1A splice-site polymorphism modifies the effect of carbamazepine on cortical excitability-a pharmacogenetic transcranial magnetic stimulation study. Epilepsia 2014;55:362–9.
Mori F, Ribolsi M, Kusayanagi H, Monteleone F, Mantovani V, Buttari F, et al. TRPV1 channels regulate cortical excitability in humans. J Neurosci. 2012;32:873–9.
Eichhammer P, Langguth B, Wiegand R, Kharraz A, Frick U, Hajak G. Allelic variation in the serotonin transporter promoter affects neuromodulatory effects of a selective serotonin transporter reuptake inhibitor (SSRI). Psychopharmacology 2003;166:294–7.
Lahr J, Peter J, Minkova L, Lauer E, Reis J, Heimbach B, et al. No difference in paired associative stimulation induced cortical neuroplasticity between patients with mild cognitive impairment and elderly controls. Clin Neurophysiol. 2016;127:1254–60.
Duman RS, Sanacora G, Krystal JH. Altered connectivity in depression: GABA and glutamate neurotransmitter deficits and reversal by novel treatments. Neuron 2019;102:75–90.
Robol E, Fiaschi A, Manganotti P. Effects of citalopram on the excitability of the human motor cortex: a paired magnetic stimulation study. J Neurol Sci. 2004;221:41–6.
Batsikadze G, Paulus W, Kuo MF, Nitsche MA. Effect of serotonin on paired associative stimulation-induced plasticity in the human motor cortex. Neuropsychopharmacology 2013;38:2260–7.
Herwig U, Bräuer K, Connemann B, Spitzer M, Schönfeldt-Lecuona C. Intracortical excitability is modulated by a norepinephrine-reuptake inhibitor as measured with paired-pulse transcranial magnetic stimulation. Psychopharmacology. 2002;164:228–32.
Fresnoza S, Stiksrud E, Klinker F, Liebetanz D, Paulus W, Kuo MF, et al. Dosage-dependent effect of dopamine D2 receptor activation on motor cortex plasticity in humans. J Neurosci. 2014;34:10701–9.
Thirugnanasambandam N, Grundey J, Paulus W, Nitsche MA. Dose-dependent nonlinear effect of l-DOPA on paired associative stimulation-induced neuroplasticity in humans. J Neurosci. 2011;31:5294–9.
Ziemann U. Pharmacology of TMS. Suppl Clin Neurophysiol. 2003;56:226–31.
Cohen Kadosh K, Krause B, King AJ, Near J, Cohen Kadosh R. Linking GABA and glutamate levels to cognitive skill acquisition during development. Hum Brain Mapp. 2015;36:4334–45.
Lenze EJ, Mulsant BH, Blumberger DM, Karp JF, Newcomer JW, Anderson SJ, et al. Efficacy, safety, and tolerability of augmentation pharmacotherapy with aripiprazole for treatment-resistant depression in late life: a randomised, double-blind, placebo-controlled trial. Lancet 2015;386:2404–12.
Lin C, Karim HT, Pecina M, Aizenstein HJ, Lenze EJ, Blumberger DM, et al. Low-dose augmentation with buprenorphine increases emotional reactivity but not reward activity in treatment resistant mid- and late-life depression. Neuroimage Clin. 2019;21:101679.
Montgomery SA, Asberg M. A new depression scale designed to be sensitive to change. Br J Psychiatry. 1979;134:382–9.
Chipchase L, Schabrun S, Cohen L, Hodges P, Ridding M, Rothwell J, et al. A checklist for assessing the methodological quality of studies using transcranial magnetic stimulation to study the motor system: an international consensus study. Clin Neurophysiol. 2012;123:1698–704.
Marshe VS, Maciukiewicz M, Hauschild AC, Islam F, Qin L, Tiwari AK, et al. Genome-wide analysis suggests the importance of vascular processes and neuroinflammation in late-life antidepressant response. Transl Psychiatry. 2021;11:127.
Anderson CA, Pettersson FH, Clarke GM, Cardon LR, Morris AP, Zondervan KT. Data quality control in genetic case-control association studies. Nat Protoc. 2010;5:1564–73.
Lemieux Perreault LP, Legault MA, Asselin G, Dubé MP. genipe: an automated genome-wide imputation pipeline with automatic reporting and statistical tools. Bioinformatics 2016;32:3661–3.
van Leeuwen EM, Kanterakis A, Deelen P, Kattenberg MV, Genome of the Netherlands Consortium, Slagboom PE, et al. Population-specific genotype imputations using minimac or IMPUTE2. Nat Protoc. 2015;10:1285–96.
Auton A, Abecasis GR, Altshuler DM, Durbin RM, Abecasis GR, Bentley DR, et al. A global reference for human genetic variation. Nature 2015;526:68–74.
Loh PR, Danecek P, Palamara PF, Fuchsberger C, Reshef AY, Finucane KH, et al. Reference-based phasing using the Haplotype Reference Consortium panel. Nat Genet. 2016;48:1443–8.
Purcell S, Neale B, Todd-Brown K, Thomas L, Ferreira MAR, Bender D, et al. PLINK: a tool set for whole-genome association and population-based linkage analyses. Am J Hum Genet. 2007;81:559–75.
Leeuw CA, de, Mooij JM, Heskes T, Posthuma D. MAGMA: generalized gene-set analysis of GWAS data. PLOS Comput Biol. 2015;11:e1004219.
Watanabe K, Taskesen E, van Bochoven A, Posthuma D. Functional mapping and annotation of genetic associations with FUMA. Nat Commun. 2017;8:1826.
Oba T, Saito T, Asada A, Shimizu S, Iijima KM, Ando K. Microtubule affinity–regulating kinase 4 with an Alzheimer’s disease-related mutation promotes tau accumulation and exacerbates neurodegeneration. J Biol Chem. 2020;295:17138–47.
Biundo F, Del Prete D, Zhang H, Arancio O, D’Adamio L. A role for tau in learning, memory and synaptic plasticity. Sci Rep. 2018;8:3184.
Babulal GM, Roe CM, Stout SH, Rajasekar G, Wisch JK, Benzinger TLS, et al. Depression is associated with tau and not amyloid positron emission tomography in cognitively normal adults. J Alzheimers Dis. 2020;74:1045–55.
Loureiro JC, Stella F, Pais MV, Radanovic M, Canineu PR, Joaquim HPG, et al. Cognitive impairment in remitted late-life depression is not associated with Alzheimer’s disease-related CSF biomarkers. J Affect Disord. 2020;272:409–16.
Jouanne M, Rault S, Voisin-Chiret AS. Tau protein aggregation in Alzheimer’s disease: an attractive target for the development of novel therapeutic agents. Eur J Med Chem. 2017;139:153–67.
Hayden EY, Putman J, Nunez S, Shin WS, Oberoi M, Charreton M, et al. Ischemic axonal injury up-regulates MARK4 in cortical neurons and primes tau phosphorylation and aggregation. Acta Neuropathol Commun. 2019;7:135.
Alexopoulos GS Mechanisms and treatment of late-life depression. Transl Psychiatry. 2019;9:188.
Attems J, Jellinger KA. The overlap between vascular disease and Alzheimer’s disease - lessons from pathology. BMC Med. 2014;12:206.
PPP1R37 protein phosphatase 1 regulatory subunit 37 [Homo sapiens (human)] - Gene - NCBI. [cited 2022 Feb 25]. Available from: https://www.ncbi.nlm.nih.gov/gene/284352.
Liu N, Xu J, Liu H, Zhang S, Li M, Zhou Y, et al. Hippocampal transcriptome-wide association study and neurobiological pathway analysis for Alzheimer’s disease. PLoS Genet. 2021;17:e1009363.
Braithwaite SP, Stock JB, Lombroso PJ, Nairn AC. Protein phosphatases and Alzheimer’s disease. Prog Mol Biol Transl Sci. 2012;106:343–79.
Shibuya K, Park SB, Geevasinga N, Huynh W, Simon NG, Menon P, et al. Threshold tracking transcranial magnetic stimulation: Effects of age and gender on motor cortical function. Clin Neurophysiol. 2016;127:2355–61.
Byers AL, Yaffe K. Depression and risk of developing dementia. Nat Rev Neurol. 2011;7:323–31.
McKinney BC, Sibille E. The age-by-disease interaction hypothesis of late-life depression. Am J Geriatr Psychiatry. 2013;21. https://doi.org/10.1016/j.jagp.2013.01.053.
EGFLAM EGF like, fibronectin type III and laminin G domains [Homo sapiens (human)] - Gene - NCBI [Internet]. [cited 2022 Nov 18]. Available from: https://www.ncbi.nlm.nih.gov/gene/133584#summary.
Adkins DE, Clark SL, Åberg K, Hettema JM, Bukszár J, McClay JL, et al. Genome-wide pharmacogenomic study of citalopram-induced side effects in STAR*D. Transl Psychiatry. 2012;2:e129.
Lee JJ, Wedow R, Okbay A, Kong E, Maghzian O, Zacher M, et al. Gene discovery and polygenic prediction from a genome-wide association study of educational attainment in 1.1 million individuals. Nat Genet. 2018;50:1112–21.
Okbay A, Wu Y, Wang N, Jayashankar H, Bennett M, Nehzati SM, et al. Polygenic prediction of educational attainment within and between families from genome-wide association analyses in 3 million individuals. Nat Genet. 2022;54:437–49.
Lissemore JI, Mulsant BH, Bonner AJ, Butters MA, Chen R, Downar J, et al. Transcranial magnetic stimulation indices of cortical excitability enhance the prediction of response to pharmacotherapy in late-life depression. Biol Psychiatry: Cogn Neurosci Neuroimaging. 2022;7:265–75.
Witte AV, Kürten J, Jansen S, Schirmacher A, Brand E, Sommer J, et al. Interaction of BDNF and COMT polymorphisms on paired-associative stimulation-induced cortical plasticity. J Neurosci. 2012;32:4553–61.
Player MJ, Taylor JL, Weickert CS, Alonzo A, Sachdev P, Martin D, et al. Neuroplasticity in depressed individuals compared with healthy controls. Neuropsychopharmacology 2013;38:2101–8.
Gilbert DL, Wang Z, Sallee FR, Ridel KR, Merhar S, Zhang J, et al. Dopamine transporter genotype influences the physiological response to medication in ADHD. Brain 2006;129:2038–46.
Reynolds CF III, Weissman MM. Transdisciplinary science and research training in psychiatry: a robust approach to innovation. JAMA Psychiatry. 2022;79:839–40.
Tremblay S, Rogasch NC, Premoli I, Blumberger DM, Casarotto S, Chen R, et al. Clinical utility and prospective of TMS-EEG. Clin Neurophysiol. 2019;130:802–44.
Tam V, Patel N, Turcotte M, Bossé Y, Paré G, Meyre D. Benefits and limitations of genome-wide association studies. Nat Rev Genet. 2019;20:467–84.
Penner-Goeke S, Binder EB. Epigenetics and depression. Dialogues Clin Neurosci. 2019;21:397–405.
Nitsche MA, Kuo MF, Grosch J, Bergner C, Monte-Silva K, Paulus W. D1-receptor impact on neuroplasticity in humans. J Neurosci. 2009;29:2648–53.
Hummel FC, Steven B, Hoppe J, Heise K, Thomalla G, Cohen LG, et al. Deficient intracortical inhibition (SICI) during movement preparation after chronic stroke. Neurology 2009;72:1766–72.
Ding Q, Triggs WJ, Kamath SM, Patten C. Short intracortical inhibition during voluntary movement reveals persistent impairment post-stroke. Front Neurol. 2019;9:1105.
Acknowledgements
We acknowledge the CAMH Biobank and Molecular Core Family for preparing and genotyping the data. Analyses were performed on the CAMH Specialized Computing Cluster (SCC). The SCC is funded by The Canada Foundation for Innovation, Research Hospital Fund. This work was also funded by the National Institute of Mental Health (R01 MH083660, P30 MH90333, R01 MH083648, R01 MH083643, R34 MH101371, R01 DA025931), the UPMC Endowment in Geriatric Psychiatry, the Taylor Family Institute for Innovative Psychiatric Research, the Washington University Institute of Clinical and Translational Sciences grant (UL1 TR000448), and the Campbell Family Mental Health Research Institute at the Centre for Addiction and Mental Health, Toronto.
Author information
Authors and Affiliations
Contributions
RAW, XM, DJM, and DMB conceived and designed the study. All authors contributed to acquisition, analysis, or interpretation of data. RAW, XM, DJM, and DMB were involved in drafting the manuscript. All authors contributed to critical revisions of the manuscript. XM completed statistical analysis. DJM and DMB provided supervision.
Corresponding author
Ethics declarations
Competing interests
None for RAW, XM, SSME, VSM, JIL, and DJM. TKR has received research support from Brain Canada, Brain and Behavior Research Foundation, BrightFocus Foundation, Canada Foundation for Innovation, Canada Research Chair, Canadian Institutes of Health Research, Centre for Aging and Brain Health Innovation, National Institutes of Health, Ontario Ministry of Health and Long-Term Care, Ontario Ministry of Research and Innovation, and the Weston Brain Institute. Dr. Rajji also received for an investigator-initiated study in-kind equipment support from Newronika, and in-kind research online accounts from Scientific Brain Training Pro, and participated in 2021 and 2022 in an advisory activity for Biogen Canada Inc. Dr. Rajji is also an inventor on the United States Provisional Patent No. 17/396,030 that describes cell-based assays and kits for assessing serum cholinergic receptor activity. BHM is supported in part by the Labatt Family Chair in Biology of Depression in Late-Life Adults at the University of Toronto. He currently receives or has received during the past five years research support from Brain Canada, the Canadian Institutes of Health Research, the CAMH Foundation, the Patient-Centered Outcomes Research Institute (PCORI), the US National Institute of Health (NIH), Capital Solution Design LLC (software used in a study founded by CAMH Foundation), and HAPPYneuron (software used in a study founded by Brain Canada). Within the past five years, he has been an unpaid consultant to Myriad Neuroscience. JFK receipt of medication supplies for investigator-initiated studies from Pfizer and Indivior. Service on scientific advisory board of Aifred Health with potential for equity. He has served as a scientific advisor for Biogen. CFR has received research support from the NIH, PCORI, the Center for Medicare and Medicaid Services, the American Foundation for Suicide Prevention, the Brain and Behavior Research Foundation, and the Commonwealth of Pennsylvania. Bristol Meyers Squib and Pfizer have provided pharmaceutical supplies for his NIH sponsored research. EJL receives research support from the US National Institute of Health (NIH), the Patient-Centered Outcomes Research Institute (PCORI), McKnight Brain Research Foundation, Takeda, Lundbeck, and consulting fees (past) from Janssen and Jazz Pharmaceuticals. ZJD has received research and equipment in-kind support for an investigator-initiated study through Brainsway Inc and Magventure Inc and industry-initiated trials through Magnus Inc. He also currently serves on the scientific advisory board for Brainsway Inc. His work has been supported by the National Institutes of Mental Health (NIMH), the Canadian Institutes of Health Research (CIHR), Brain Canada and the Temerty Family, Grant and Kreutzcamp Family Foundations. DMB receives research support from the Canadian Institutes of Health Research (CIHR), National Institutes of Health – US (NIH), Weston Brain Institute, Brain Canada and the Temerty Family through the CAMH Foundation and the Campbell Research Institute. He received research support and in-kind equipment support for an investigator-initiated study from Brainsway Ltd. and he is the site principal investigator for three sponsor-initiated studies for Brainsway Ltd. He receives in-kind equipment support from Magventure for two investigator-initiated studies. He received medication supplies for an investigator-initiated trial from Indivior. He has participated in an advisory board for Janssen.
Additional information
Publisher’s note Springer Nature remains neutral with regard to jurisdictional claims in published maps and institutional affiliations.
Rights and permissions
Open Access This article is licensed under a Creative Commons Attribution 4.0 International License, which permits use, sharing, adaptation, distribution and reproduction in any medium or format, as long as you give appropriate credit to the original author(s) and the source, provide a link to the Creative Commons license, and indicate if changes were made. The images or other third party material in this article are included in the article’s Creative Commons license, unless indicated otherwise in a credit line to the material. If material is not included in the article’s Creative Commons license and your intended use is not permitted by statutory regulation or exceeds the permitted use, you will need to obtain permission directly from the copyright holder. To view a copy of this license, visit http://creativecommons.org/licenses/by/4.0/.
About this article
Cite this article
Wathra, R.A., Men, X., Elsheikh, S.S.M. et al. Exploratory genome-wide analyses of cortical inhibition, facilitation, and plasticity in late-life depression. Transl Psychiatry 13, 234 (2023). https://doi.org/10.1038/s41398-023-02532-0
Received:
Revised:
Accepted:
Published:
DOI: https://doi.org/10.1038/s41398-023-02532-0