Abstract
Leiomyosarcoma (LMS) is a soft tissue tumor with a significant degree of morphologic and molecular heterogeneity. We used integrative molecular profiling to discover and characterize molecular subtypes of LMS. Gene expression profiling was performed on 51 LMS samples. Unsupervised clustering showed three reproducible LMS clusters. Array comparative genomic hybridization (aCGH) was performed on 20 LMS samples and showed that the molecular subtypes defined by gene expression showed distinct genomic changes. Tumors from the ‘muscle-enriched’ cluster showed significantly increased copy number changes (P=0.04). A majority of the muscle-enriched cases showed loss at 16q24, which contains Fanconi anemia, complementation group A, known to have an important role in DNA repair, and loss at 1p36, which contains PRDM16, of which loss promotes muscle differentiation. Immunohistochemistry (IHC) was performed on LMS tissue microarrays (n=377) for five markers with high levels of messenger RNA in the muscle-enriched cluster (ACTG2, CASQ2, SLMAP, CFL2 and MYLK) and showed significantly correlated expression of the five proteins (all pairwise P<0.005). Expression of the five markers was associated with improved disease-specific survival in a multivariate Cox regression analysis (P<0.04). In this analysis that combined gene expression profiling, aCGH and IHC, we characterized distinct molecular LMS subtypes, provided insight into their pathogenesis, and identified prognostic biomarkers.
This is a preview of subscription content, access via your institution
Access options
Subscribe to this journal
Receive 50 print issues and online access
$259.00 per year
only $5.18 per issue
Buy this article
- Purchase on Springer Link
- Instant access to full article PDF
Prices may be subject to local taxes which are calculated during checkout



Similar content being viewed by others
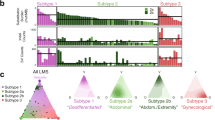
Accession codes
References
Abbas AK, Cotran RS, Fausto N, Kumar V, Perkins JA, Robbins SL . (2005). Robbins and Cotran Pathologic Basis of Disease [print/digital], Vol xv, 7th edn. Elsevier/Saunders: Philadelphia, 1525, pp.
Ackerman LV, Rosai J . (2004). Rosai and Ackerman's Surgical Pathology [print], 9th edn. Mosby: Edinburgh; New York.
Alizadeh AA, Eisen MB, Davis RE, Ma C, Lossos IS, Rosenwald A et al. (2000). Distinct types of diffuse large B-cell lymphoma identified by gene expression profiling. Nature 403: 503–511.
Baird K, Davis S, Antonescu CR, Harper UL, Walker RL, Chen Y et al. (2005). Gene expression profiling of human sarcomas: insights into sarcoma biology. Cancer Res 65: 9226–9235.
Beck AH, Espinosa I, Edris B, Li R, Montgomery K, Zhu S et al. (2009a). The macrophage colony-stimulating factor 1 response signature in breast carcinoma. Clin Cancer Res 15: 778–787.
Beck AH, West RB, van de Rijn M . (2009b). Gene expression profiling for the investigation of soft tissue sarcoma pathogenesis and the identification of diagnostic, prognostic, and predictive biomarkers. Virchows Arch (e-pub ahead of print 2 May 2009; PMID: 19412622).
Bernards R, Weinberg RA . (2002). A progression puzzle. Nature 418: 823.
Borden EC, Baker LH, Bell RS, Bramwell V, Demetri GD, Eisenberg BL et al. (2003). Soft tissue sarcomas of adults: state of the translational science. Clin Cancer Res 9: 1941–1956.
Bullinger L, Dohner K, Bair E, Frohling S, Schlenk RF, Tibshirani R et al. (2004). Use of gene-expression profiling to identify prognostic subclasses in adult acute myeloid leukemia. N Engl J Med 350: 1605–1616.
Edgar R, Domrachev M, Lash AE . (2002). Gene expression omnibus: NCBI gene expression and hybridization array data repository. Nucleic Acids Res 30: 207–210.
Espinosa I, Beck AH, Lee CH, Zhu S, Montgomery KD, Marinelli RJ et al. (2009). Coordinate expression of colony-stimulating factor-1 and colony-stimulating factor-1-related proteins is associated with poor prognosis in gynecological and nongynecological leiomyosarcoma. Am J Pathol 174: 2347–2356.
Fletcher CDM, Unni KK, Mertens F, World Health Organization, International Agency for Research on Cancer. Pathology and Genetics of Tumours of Soft Tissue and Bone. IARC Press: Lyon, 427 pp.
Francis P, Namlos HM, Muller C, Eden P, Fernebro J, Berner JM et al. (2007). Diagnostic and prognostic gene expression signatures in 177 soft tissue sarcomas: hypoxia-induced transcription profile signifies metastatic potential. BMC Genomics 8: 73.
Helman LJ, Meltzer P . (2003). Mechanisms of sarcoma development. Nat Rev Cancer 3: 685–694.
Henderson SR, Guiliano D, Presneau N, McLean S, Frow R, Vujovic S et al. (2005). A molecular map of mesenchymal tumors. Genome Biol 6: R76.
Hernando E, Charytonowicz E, Dudas ME, Menendez S, Matushansky I, Mills J et al. (2007). The AKT-mTOR pathway plays a critical role in the development of leiomyosarcomas. Nat Med 13: 748–753.
Jun HJ, Lee J, Lim DH, Park JO, Ahn G, Seo SW et al. (2009). Expression of MET in alveolar soft part sarcoma. Med Oncol (e-pub ahead of print 27 May 2009; PMID: 19472090).
Kapp AV, Tibshirani R . (2007). Are clusters found in one dataset present in another dataset? Biostatistics 8: 9–31.
Kattan MW, Leung DH, Brennan MF . (2002). Postoperative nomogram for 12-year sarcoma-specific death. J Clin Oncol 20: 791–796.
Kochetkova M, McKenzie OL, Bais AJ, Martin JM, Secker GA, Seshadri R et al. (2002). CBFA2T3 (MTG16) is a putative breast tumor suppressor gene from the breast cancer loss of heterozygosity region at 16q24.3. Cancer Res 62: 4599–4604.
Lakhani SR, Ashworth A . (2001). Microarray and histopathological analysis of tumours: the future and the past? Nat Rev Cancer 1: 151–157.
Landis-Piwowar KR, Milacic V, Dou QP . (2008). Relationship between the methylation status of dietary flavonoids and their growth-inhibitory and apoptosis-inducing activities in human cancer cells. J Cell Biochem 105: 514–523.
Lapointe J, Li C, Higgins JP, van de Rijn M, Bair E, Montgomery K et al. (2004). Gene expression profiling identifies clinically relevant subtypes of prostate cancer. Proc Natl Acad Sci USA 101: 811–816.
Larramendy ML, Gentile M, Soloneski S, Knuutila S, Bohling T . (2008). Does comparative genomic hybridization reveal distinct differences in DNA copy number sequence patterns between leiomyosarcoma and malignant fibrous histiocytoma? Cancer Genet Cytogenet 187: 1–11.
Lee CH, Espinosa I, Vrijaldenhoven S, Subramanian S, Montgomery KD, Zhu S et al. (2008). Prognostic significance of macrophage infiltration in leiomyosarcomas. Clin Cancer Res 14: 1423–1430.
Lee YF, John M, Falconer A, Edwards S, Clark J, Flohr P et al. (2004). A gene expression signature associated with metastatic outcome in human leiomyosarcomas. Cancer Res 64: 7201–7204.
Lensch MW, Tischkowitz M, Christianson TA, Reifsteck CA, Speckhart SA, Jakobs PM et al. (2003). Acquired FANCA dysfunction and cytogenetic instability in adult acute myelogenous leukemia. Blood 102: 7–16.
Li L, Ren CH, Tahir SA, Ren C, Thompson TC . (2003). Caveolin-1 maintains activated Akt in prostate cancer cells through scaffolding domain binding site interactions with and inhibition of serine/threonine protein phosphatases PP1 and PP2A. Mol Cell Biol 23: 9389–9404.
Meza-Zepeda LA, Kresse SH, Barragan-Polania AH, Bjerkehagen B, Ohnstad HO, Namlos HM et al. (2006). Array comparative genomic hybridization reveals distinct DNA copy number differences between gastrointestinal stromal tumors and leiomyosarcomas. Cancer Res 66: 8984–8993.
Nakayama R, Nemoto T, Takahashi H, Ohta T, Kawai A, Seki K et al. (2007). Gene expression analysis of soft tissue sarcomas: characterization and reclassification of malignant fibrous histiocytoma. Mod Pathol 20: 749–759.
Nielsen TO, West RB, Linn SC, Alter O, Knowling MA, O’Connell JX et al. (2002). Molecular characterisation of soft tissue tumours: a gene expression study. Lancet 359: 1301–1307.
Ottone T, Hasan SK, Montefusco E, Curzi P, Mays AN, Chessa L et al. (2009). Identification of a potential ‘hotspot’ DNA region in the RUNX1 gene targeted by mitoxantrone in therapy-related acute myeloid leukemia with t(16;21) translocation. Genes Chromosomes Cancer 48: 213–221.
Perot G, Derre J, Coindre JM, Tirode F, Lucchesi C, Mariani O et al. (2009). Strong smooth muscle differentiation is dependent on myocardin gene amplification in most human retroperitoneal leiomyosarcomas. Cancer Res 69: 2269–2278.
Pervaiz N, Colterjohn N, Farrokhyar F, Tozer R, Figueredo A, Ghert M . (2008). A systematic meta-analysis of randomized controlled trials of adjuvant chemotherapy for localized resectable soft-tissue sarcoma. Cancer 113: 573–581.
Potti A, Dressman HK, Bild A, Riedel RF, Chan G, Sayer R et al. (2006). Genomic signatures to guide the use of chemotherapeutics. Nat Med 12: 1294–1300.
Quade BJ, Wang TY, Sornberger K, Dal Cin P, Mutter GL, Morton CC . (2004). Molecular pathogenesis of uterine smooth muscle tumors from transcriptional profiling. Genes Chromosomes Cancer 40: 97–108.
Ramaswamy S, Ross KN, Lander ES, Golub TR . (2003). A molecular signature of metastasis in primary solid tumors. Nat Genet 33: 49–54.
Ren B, Yu YP, Jing L, Liu L, Michalopoulos GK, Luo JH et al. (2003). Gene expression analysis of human soft tissue leiomyosarcomas. Hum Pathol 34: 549–558.
Rong S, Jeffers M, Resau JH, Tsarfaty I, Oskarsson M, Vande Woude GF . (1993). Met expression and sarcoma tumorigenicity. Cancer Res 53: 5355–5360.
Sarcoma Meta-analysis Collaboration (2000). Adjuvant chemotherapy for localised resectable soft tissue sarcoma in adults. Cochrane Database Syst Rev. Art. No. CD001419.
Seale P, Bjork B, Yang W, Kajimura S, Chin S, Kuang S et al. (2008). PRDM16 controls a brown fat/skeletal muscle switch. Nature 454: 961–967.
Segal NH, Pavlidis P, Antonescu CR, Maki RG, Noble WS, DeSantis D et al. (2003). Classification and subtype prediction of adult soft tissue sarcoma by functional genomics. Am J Pathol 163: 691–700.
Shmulevich I, Hunt K, El-Naggar A, Taylor E, Ramdas L, Laborde P et al. (2002). Tumor specific gene expression profiles in human leiomyosarcoma: an evaluation of intratumor heterogeneity. Cancer 94: 2069–2075.
Sidransky D . (2002). Emerging molecular markers of cancer. Nat Rev Cancer 2: 210–219.
Skubitz KM, Skubitz AP . (2003). Differential gene expression in leiomyosarcoma. Cancer 98: 1029–1038.
Sorlie T, Perou CM, Tibshirani R, Aas T, Geisler S, Johnsen H et al. (2001). Gene expression patterns of breast carcinomas distinguish tumor subclasses with clinical implications. Proc Natl Acad Sci USA 98: 10869–10874.
Sotiriou C, Piccart MJ . (2007). Taking gene-expression profiling to the clinic: when will molecular signatures become relevant to patient care? Nat Rev Cancer 7: 545–553.
Tibshirani R, Hastie T, Narasimhan B, Chu G . (2002). Diagnosis of multiple cancer types by shrunken centroids of gene expression. Proc Natl Acad Sci USA 99: 6567–6572.
Tibshirani R, Wang P . (2008). Spatial smoothing and hot spot detection for CGH data using the fused lasso. Biostatistics 9: 18–29.
Toro JR, Travis LB, Wu HJ, Zhu K, Fletcher CD, Devesa SS . (2006). Incidence patterns of soft tissue sarcomas, regardless of primary site, in the surveillance, epidemiology and end results program, 1978–2001: An analysis of 26 758 cases. Int J Cancer 119: 2922–2930.
Tusher VG, Tibshirani R, Chu G . (2001). Significance analysis of microarrays applied to the ionizing radiation response. Proc Natl Acad Sci USA 98: 5116–5121.
Wang W . (2007). Emergence of a DNA-damage response network consisting of Fanconi anaemia and BRCA proteins. Nat Rev Genet 8: 735–748.
Weigelt B, Hu Z, He X, Livasy C, Carey LA, Ewend MG et al. (2005). Molecular portraits and 70-gene prognosis signature are preserved throughout the metastatic process of breast cancer. Cancer Res 65: 9155–9158.
Weigelt B, van’t Veer LJ . (2004). Hard-wired genotype in metastatic breast cancer. Cell Cycle 3: 756–757.
Weiss SW, Goldblum JR . (2008). Enzinger and Weiss's Soft Tissue Tumors, 5th edn. Mosby/Elsevier: Philadelphia.
Zou H, Hastie T, Tibshirani R . (2006). Sparse principal component analysis. J Comput Graphical Stat 15: 265–286.
Acknowledgements
Grant support came from NIH grant CA112270, the National Leiomyosarcoma Foundation and the Leiomyosarcoma Direct Research Foundation. The authors dedicate this paper to the memory of Suzanne Kurtz, LMS patient and founder of LMSdr. This work was supported by NIH grant CA112270, the National Leiomyosarcoma Foundation and the Leiomyosarcoma Direct Research Foundation.
Author information
Authors and Affiliations
Corresponding author
Additional information
This material was presented in part at the 2009 United States and Canadian Academy of Pathology Meeting in Boston, MA, USA.
Supplementary Information accompanies the paper on the Oncogene website (http://www.nature.com/onc)
Supplementary information
Rights and permissions
About this article
Cite this article
Beck, A., Lee, CH., Witten, D. et al. Discovery of molecular subtypes in leiomyosarcoma through integrative molecular profiling. Oncogene 29, 845–854 (2010). https://doi.org/10.1038/onc.2009.381
Received:
Revised:
Accepted:
Published:
Issue Date:
DOI: https://doi.org/10.1038/onc.2009.381