Abstract
The aetiology of suicidal behaviour is complex, and knowledge about its neurobiological mechanisms is limited. Neuroimaging methods provide a noninvasive approach to explore the neural correlates of suicide vulnerability in vivo. The ENIGMA-MDD Working Group is an international collaboration evaluating neuroimaging and clinical data from thousands of individuals collected by research groups from around the world. Here we present analyses in a subset sample (n=3097) for whom suicidality data were available. Prevalence of suicidal symptoms among major depressive disorder (MDD) cases ranged between 29 and 69% across cohorts. We compared mean subcortical grey matter volumes, lateral ventricle volumes and total intracranial volume (ICV) in MDD patients with suicidal symptoms (N=451) vs healthy controls (N=1996) or MDD patients with no suicidal symptoms (N=650). MDD patients reporting suicidal plans or attempts showed a smaller ICV (P=4.12 × 10−3) or a 2.87% smaller volume compared with controls (Cohen’s d=−0.284). In addition, we observed a nonsignificant trend in which MDD cases with suicidal symptoms had smaller subcortical volumes and larger ventricular volumes compared with controls. Finally, no significant differences (P=0.28–0.97) were found between MDD patients with and those without suicidal symptoms for any of the brain volume measures. This is by far the largest neuroimaging meta-analysis of suicidal behaviour in MDD to date. Our results did not replicate previous reports of association between subcortical brain structure and suicidality and highlight the need for collecting better-powered imaging samples and using improved suicidality assessment instruments.
Similar content being viewed by others
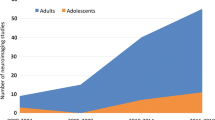
Introduction
Suicide has become a major public health issue for societies around the world. According to the World Health Organisation, more than 800 000 people die from suicide each year,1 and for every completed suicide, there are between 10 and 20 attempted suicides.2, 3, 4 It is also of concern that reported population rates have increased by almost 60% between the 1960s and 2012, with the largest increases seen in developing countries.3
The aetiology of suicidal behaviour is complex, and our knowledge about its neurobiological mechanisms is limited.5 The stress vulnerability model for suicidal behaviour5 suggests that a combination of individual genetic vulnerability and exposure to environmental stressors is what triggers suicidal behaviour. The current state of knowledge indicates that up to 38-55% of risk variance may be explained by genetic factors.6 Other known risk factors for suicidal behaviour include drug misuse, mood state and a range of cultural, family and social situations. However, the main contributing vulnerability for suicidal behaviour is having a history of self-harm and suicide attempts or having a psychiatric disorder.7, 8 In fact, up to 90% of suicide victims may have a (often untreated) psychiatric disorder,8, 9 with major depressive disorder (MDD) affecting between 50 and 70% of all victims.9, 10, 11, 12, 13, 14
Typically, depressive episodes involve symptoms such as hopelessness, negative affect and recurrent thoughts about death and suicide.15 Twin, family and adoption studies have demonstrated a significant genetic overlap between suicidal ideation and depression,16 while also recognizing that a proportion of the heritable component of suicidal behaviour is independent from other psychiatric disorders.17, 18 Remarkably, this is now reflected in the newest version of the Diagnostic and Statistical Manual of Mental Disorders (DSM-V),15 which has introduced suicidal behaviour disorder as a diagnosis on its own, as compared with previous versions in which it was only considered as a symptom of a range of disorders. In addition, stressful life events (abuse/assault, interpersonal conflict or loss, or employment difficulties) also increase the likelihoods for both depression and suicidal behaviour.19, 20 Yet, despite multiple shared genetic and environmental risk contributors, only a small proportion of individuals with MDD who are confronted with acute stressors will display any suicidal symptoms.
Although suicide is a preventable cause of death, predicting and preventing suicidal behaviour has proven to be challenging. At present, individual suicidality risk is assessed on the basis of social, demographic and clinical factors, which despite being highly sensitive, have low specificity.21 Several suicide-risk questionnaires and scales have been proposed, achieving varying degrees of predictive accuracy.22 One important consideration is that it is sometimes difficult to obtain sufficient information from patients who may not always be willing or able to share subjective experiences with clinicians. Thus, the field has started to focus on the underlying neuropsychological and neurobiological mechanisms of suicidal behaviour. Ultimately, an improved understanding of the neurobiology of suicidal vulnerability may facilitate the development of more effective suicide-prevention strategies.23
Magnetic resonance imaging of the brain provides a noninvasive method by which to explore the neural correlates of suicidal behaviour in vivo. Early structural magnetic resonance imaging studies of suicidal behaviour in MDD showed promising results, revealing smaller grey matter volumes of the orbitofrontal cortex and larger amygdala volume in suicidal patients compared with controls and nonsuicidal patients.24 Several literature reviews of neuroimaging and suicidal behaviour have been published recently.23, 25, 26, 27 Studies in the literature suggest potential associations between suicidality and brain regions such as the orbitofrontal and anterior cingulate cortex.26, 28 However, such neuroanatomical patterns might not be consistent across individuals that display suicidal behaviour but suffer from different psychiatric disorders.29, 30 A possible explanation could be that different pathways can lead to increased suicide vulnerability in different psychiatric disorders, or that different components of suicidal behaviour such as impulsivity, hopelessness, cognitive rigidity or ideation are managed through different brain regions. Empirical evidence indicates that suicidal behaviour is dissimilar in patients with different psychiatric disorders.31 However, no formal comparison has been conducted to date.
Together with the anterior cingulate cortex and orbitofrontal cortex, subcortical regions of the striatum may be particularly relevant for suicidal behaviour vulnerability in a depressed state as compared with other states.26 Some MDD studies have reported increases in amygdala volume24 or reductions in caudate and pallidum volume in patients with a history of suicide attempts.32 However, as pointed out by others,26, 33 published studies in the field are few, and rely upon small sample sizes, which means limited statistical power and higher risk of false positive/negative associations. To overcome these limitations, increasing statistical power and reliability, and advancing our understanding of the neural correlates of suicidal vulnerability in MDD, we initiated a project within the Major Depression Working Group of the ENIGMA consortium,34 an international collaboration evaluating neuroimaging and clinical data from a growing number of research samples from around the world. Our aim is to identify neuroimaging markers that robustly discriminate MDD patients from healthy controls, using individual participant data-based meta-analysis and incorporating standardized image processing and statistical analysis protocols.
Previously, we performed a meta-analysis of subcortical volumes35 and confirmed a reduction in hippocampal volume in recurrent MDD patients relative to controls.35 Here we present a series of analyses in a subset of that sample for which suicidality data were available. We compare mean grey matter volumes of seven subcortical structures, lateral ventricle volumes and total ICV in MDD patients with suicidal symptoms vs either healthy controls or MDD patients with no suicidal symptoms. Based on reports from previous studies, we hypothesized that volumetric differences would be observed between suicidal and nonsuicidal groups. This is by far the largest neuroimaging meta-analysis of suicidal behaviour in MDD to date, and establishes a new platform within the ENIGMA consortium for open collaboration among researchers interested in finding neuroimaging markers for suicidality.
Materials and methods
Samples
At the time of data freeze for this study, the ENIGMA-MDD working group included 20 samples with both neuroimaging and clinical data from healthy controls and individuals who meet criteria for major depressive disorder.35 A subset of seven of these cohorts were included in this secondary project. Detailed demographics for each contributing site are listed in Table 1. Diagnostic instruments used to determine MDD status, specific items on suicidality and exclusion criteria for enrolment in each sample are given in Supplementary Tables 1 and 2. In total, we analysed data from 3097 participants including 1101 cases with MDD (451 of whom exhibited suicidal ideation or behaviour) and 1996 healthy controls. All participating sites obtained approval from local institutional review boards and ethics committees, and all study participants provided written, informed consent at their local institution.
Image processing and analysis
Structural T1-weighted magnetic resonance imaging brain scans were acquired at each site and analysed locally using the fully automated and validated segmentation software FreeSurfer36 (freely available at http://surfer.nmr.mgh.harvard.edu/). Image acquisition parameters and software descriptions for each sample are given in Supplementary Table 3. The segmentations of seven subcortical grey matter regions (nucleus accumbens, amygdala, caudate nucleus, hippocampus, pallidum, putamen, thalamus), the lateral ventricles and total ICV were visually inspected for accuracy following standardized protocols designed to facilitate harmonized image analysis across multiple sites (http://enigma.ini.usc.edu/protocols/imaging-protocols/). Segmentations deemed improper were excluded from the analysis. Histogram plots were generated for each region to examine the distribution of each volumetric variable. Outlier measures (defined as >2.698 standard deviations away from the mean) were all visually verified for accuracy, and excluded only if they were not properly segmented.
Suicide nomenclature
Suicide, the act of intentionally terminating one’s life, is commonly regarded as the extreme of a continuum, which also includes suicidal ideation, planning and attempt.37 In this study, we were interested in exploring the subcortical neuroanatomical correlates of the following nonfatal suicidal manifestations in MDD: suicidal ideation, defined as thinking about suicide or taking one’s life, but without making any specific plan or acting upon those thoughts; suicidal planning, or the systematic formulation of a programme of action that has the potential to lead to a suicide attempt. And, finally, a suicidal attempt, defined as any self-initiated action aimed at terminating one’s life, regardless of the method or degree of its consequences.
Considering that the number of suicidal attempters in the studies included in this meta-analysis was too small to allow for any cross-group comparison, it was decided to group the MDD cases with suicide attempt with those MDD cases with suicide planning into a common category called suicidal behaviour. Similarly, a broader category called suicidal symptoms was created to include all MDD cases with any type of suicidal symptom (that is, ideation or behaviour). It was not possible to ascertain intent to die due to lack of information. See Supplementary Table 2 for a description of how these different definitions of suicide were measured in each individual site.
Meta-analyses
Within-group differences for MDD patients with or without suicidal symptoms and healthy controls were analysed for each sample using multiple linear regression models with total ICV or mean bilateral volume [(volume of left hemisphere+volume of right hemisphere)/2] for each region of interest as outcome measures and a binary indicator of status as a predictor of interest.
The following groups were defined: healthy controls (CTL), MDD cases with suicidal ideation (MDD-SI), MDD cases with suicidal behaviour (that is, planning or attempt; MDD-SB), MDD cases with any suicidal symptoms (that is, ideation, planning or attempt; MDD-SS) and MDD cases with no suicidal symptoms (MDD-NSS). Four sets of cross-group analyses were conducted, three of which were aimed at detecting differences between MDD groups with suicidality and healthy controls, and one more aimed at detecting differences in MDD patients with suicidal symptoms vs those without: (i) MDD-SI (1) vs CTL (0); (ii) MDD-SB (1) vs CTL (0); (iii) MDD-SS (1) vs CTL (0); and (iv) MDD-SS (1) vs MDD-NSS (0). All models controlled for age, sex and, if applicable, total ICV effects. Additional covariates were included whenever necessary to control for scanner differences. We chose to perform these cross-group comparisons for three reasons. First, considering the unprecedented large sample size and that the number of studies in the literature investigating ideation is limited,38 this was an exceptional opportunity to investigate any potential correlates. Second, the degree of overlap between the mechanisms that lead to ideation and those that activate and control behaviour is not well established. We hoped that by analysing these groups both independently and together, we could potentially gain insights about their overlap. And third, any potential biomarker for suicidality in MDD should ideally enable us to differentiate between MDD-SS and MDD-NSS, rather than just between MDD-SS and CTL.
Effect size estimates were calculated using Cohen’s d metric computed from the t-statistic of the status indicator variable from the regression models. Uncorrected P-values are reported in this manuscript. To estimate P-value thresholds that account for multiple testing, we performed matrix spectral decomposition39 on a correlation matrix of the nine phenotypic variables (ICV-adjusted mean subcortical and ventricular volumes, and ICV) obtained from a sample of unrelated individuals from the QTIM sample (N=467). Given that common variance related to ICV had been removed, the effective number of independent variables was 8.532. Thus, the P-value threshold required to keep Type I error rate at 5% adjusted for the number of independent variables being tested was 5.86 × 10−3. Furthermore, after considering the four types of cross-group comparisons that were conducted, the required significance threshold at the meta-analysis level was P<1.46 × 10−3.
Finally, we performed an additional set of analyses to investigate any potential influence of antidepressant (AD) use on our results. The only information recorded consistently across studies was whether participants were on AD treatment at the time of scanning (the proportion of MDD patients on AD treatment in each sample is shown in Table 1). Details about previous AD use history, duration, type or dosage of treatment were not recorded and therefore the analyses only considered AD use (yes/no) at the time of scanning, which was incorporated as a covariate in the model. The results of these analyses are shown in Supplementary Table 4.
Results
Prevalence of suicidal behaviour
The prevalence of suicidal symptoms among all MDD cases was 40% (451 individuals). Within-cohort prevalence among MDD cases ranged between 29 and 69%. Of those reporting any suicidal symptom, a total of 153 participants reported having at least planned to take their life, and only 14 of them having actually attempted to take their lives. Given the small number of participants who had attempted suicide, it was decided to group together those individuals who reported either having made a suicide plan or an attempt in a group labelled as MDD with suicidal behaviour (MDD-SB; see the 'Materials and methods' section for details).
Group differences in subcortical volumes
MDD suicidal ideation vs healthy controls
We meta-analysed and compared differences across subcortical, ventricular and intracranial volumes in MDD patients with suicidal ideation (N=298) vs healthy controls (N=1996; Table 2). Adjusted Cohen’s d values suggested small reductions in subcortical and intracranial volumes and a slight increase in ventricular volume. No measure displayed statistically significant differences between MDD-SI and CTL groups at the study significance threshold (P<0.00146).
MDD suicidal behaviour (planning/attempt) vs healthy controls
We meta-analysed and compared differences across subcortical, ventricular and intracranial volumes in five cohorts (the CODE and DepOx samples were excluded from the meta-analysis due to the low number of MDD patients reporting suicide planning/attempt: 3 and 0, respectively). We compared brain volumes in MDD patients with suicidal behaviour (planning/attempt, N=150) vs the healthy controls (N=1893; Table 3). A similar trend was observed, with reductions in intracranial and subcortical volumes and an increase in ventricular volume. In fact, an ICV reduction in MDD patients who reported having made suicidal plans or attempts was nearly significant at the study-wide level (P=4.12 × 10−3), with a Cohen’s d=−0.284, or a 2.87% smaller ICV compared to the CTL group.
MDD suicidal symptoms (ideation/planning/attempt) vs healthy controls
Given that similar trends were observed in both MDD-SI vs CTL and MDD-SB vs CTL analyses, we grouped together the MDD-SI and MDD-SB groups, into a group we labelled as MDD with suicidal symptoms (MDD-SS; N=451), and compared them vs healthy controls (N=1996; Table 4). We consistently observed reductions across subcortical and intracranial volumes and an increase in ventricular volume in MDD-SS individuals. Thalamic volume showed nominal significance (P=0.041), and the amygdala showed a nonsignificant trend (P=0.087), but these P-values did not survive correction for multiple testing.
To further investigate whether the pattern we observed consistently in MDD patients with suicidal symptoms vs healthy controls was due to structural brain differences linked to suicidality rather than just MDD, we compared MDD-NSS vs CTL. Although we did not observe a consistent pattern between MDD-NSS and CTLs in this study (results shown in Supplementary Table 5), the pattern is in fact consistent with our previous MDD meta-analysis, which did not separate individuals by suicidality.
MDD suicidal symptoms (ideation/planning/attempt) vs MDD with no suicidal symptoms
Last, we compared subcortical, ventricular and intracranial volumes in MDD-SS (N=451), vs MDD cases reporting no suicidal symptoms (MDD-NSS; N=650; Table 5). The largest difference was observed in the amygdala (Cohen’s d=0.081), equivalent to a 1.09% difference in volume. However, this was not significant (P=0.28), which indicates that it is not possible to reject the null hypotheses, which states that mean volumes between MDD-SS and MDD-NSS are not significantly different.
Antidepressant effects
To investigate the effects of AD use, we performed an additional set of analyses including AD use (yes/no) at the time of scanning as a covariate. The results are shown in Supplementary Table 4. Although the AD-adjusted results suggest a possible significant difference in thalamus volume between MDD-SS and healthy controls, no significant difference was observed between MDD-SS and MDD-NSS.
Power analysis
With 1910 healthy controls and 292 MDD-SI cases, we could detect lateral ventricle volume differences as small as Cohen’s d=0.119 (95% confidence interval=−0.020, 0.258) for mean lateral ventricles at a P=0.09 significance level. A post hoc analysis estimated the observed (two-tailed hypothesis) power of this analysis at 0.347 at a study-wide significance threshold of P=0.001465, and a power of 0.796 at the nominal significance threshold of P=0.05. Also, an a priori sample size calculation indicated that to achieve a power of 0.8 to detect a Cohen’s d=0.119 at P=0.001465, the study would need a minimum sample of 2290 individuals per group in a two-tailed hypothesis analysis.
Similarly, based on the final meta-analysed effect sizes found in our comparison between MDD-SS vs healthy controls, we could detect a Cohen’s d=−0.121 (95% confidence interval=−0.238, −0.005) at P=0.042, with 443 MDD-SS cases and 1892 healthy controls. The observed (two-tailed hypothesis) power was estimated at 0.396 at the study-wide significance threshold (P=0.001465), and at 0.831 for nominal significance (P=0.05). To achieve a 0.8 power at study-wide significance, 2215 individuals per group would be needed. Last, considering that the largest difference between MDD-SS and MDD-NSS was a Cohen’s d=0.081, we estimate that a sample consisting of 2394 individuals per group would be needed to achieve 0.8 power to detect a significant difference at P=0.05.
Discussion
Although earlier studies suggested potential associations between suicidality and subcortical brain regions such as the amygdala,24 caudate32, 40 or pallidum,32 a recent study in a larger sample (N=274) of patients with mood disorder failed to replicate these findings.33 To the best of our knowledge, we conducted the first neuroimaging study that uses an international coordinated meta-analytic approach to investigate potential neurobiological markers for suicidality. We analysed individual participant neuroimaging and clinical data of suicidal behaviour in MDD in the largest sample to date (N=3097), and participants encompassed diverse degrees of disease severity, scanner acquisitions and geographies. The meta-analytic approach achieves increased statistical power by combining data from different sites using standardised image processing, segmentation and data analysis protocols.
We analysed mean ventricular and subcortical grey matter volumes and total ICV in MDD patients with and without suicidal symptoms and healthy controls. Our results found no robust association of suicidal behaviour with any of the nine neuroimaging traits under investigation at the study-wide significance threshold (P<1.46 × 10−3). Observed effect sizes were relatively small, with the strongest effect observed for ICV between MD-SS and healthy controls (2.83% difference in volume). Power analyses indicated that given the observed Cohen’s d values, the current study is underpowered and that considerably larger samples will be needed (N=4788 to achieve 0.8 power to detect a significant mean difference at nominal threshold level P=0.05). This suggests that many of the previous reports of higher effect sizes in studies with small sample sizes (even cohorts as small as 7,32 10 (ref. 24) or 15 (ref. 40) individuals) may be false positives, or due to very specific characteristics of those samples. Therefore, we hope that our results discourage positive outcome bias in the literature.
If the real effects of suicidality on subcortical brain volumes are indeed small, an important question will be whether these effects are clinically relevant. We believe that it is not possible to assess the value of an effect based just on its effect size. Brain alterations with a small effect size might still have clinical relevance if they are associated with treatment response, prediction of clinical outcomes or if they help identify novel disease mechanisms. Future studies will need to confirm the relevance of these findings, and more research is needed to uncover the neuroanatomical correlates of suicidality.
Although not statistically significant, MDD-SI and MDD-SB patient groups displayed smaller intracranial and subcortical volumes and larger ventricular volumes compared with controls. These findings are consistent with our results from our previous ENIGMA-MDD study35 on a larger sample, which did not take their suicidality symptoms into account, and with the MDD-SS vs MDD-NSS analyses, where we observed no significant differences (P=0.28–0.97). Therefore, we cannot determine whether the observed pattern is associated with suicidality or whether it is a part of the existing neuroanatomical differences between MDD patients and healthy controls.
When controlling for AD use at the time of scanning, we observed a significant difference in thalamic volume between MDD-SS and healthy controls (P=0.006). However, this was not observed between MDD-SS and MDD-NSS (P=0.841). One lesson from our previous subcortical analyses of the larger ENIGMA-MDD working group was that AD users tend to show more widespread structural effects than non-antidepressant users.35 This might be because AD users tend to be the most severe/chronic or recurrent patients in the sample, and thus the results are likely to be confounded by severity. In the present study, no data were available on medication history, or duration, type and dosage of the treatment that patients were receiving at the time of scanning, and therefore it is not possible to reliably estimate the actual effects of antidepressants with our current experimental design. This issue should be investigated in future intervention studies that compare pre- and post-AD treatment to draw conclusions on the impact of AD use on suicidality and subcortical brain structure in MDD.
This study also presents some limitations. For instance, the samples in the meta-analysis displayed low prevalence of suicidal attempts and the effects of suicidality might not be detectable, either because they are only noticeable in individuals who are at very high risk or because the neural correlates of suicidality tend to become acute only during certain periods of time and then reduce quickly, unlike depression, which is less responsive. Also, given the highly heterogeneous nature of both MDD and suicidality, it is possible that there is more than one pathway that increases suicide vulnerability and therefore it will be important to gather more details about the frequency, severity or type of suicidal thoughts and behaviour that patients experience. Further, while the suicidality items used by contributing sites are routinely used to assess suicidality symptoms in major depression, future studies should consider the use of more robust and comprehensive suicidality assessment instruments that gather information on the cognitive, behavioural and affective processes of suicidality, such as rumination, impulsivity or negative affect.41
In addition, despite our quality control, standardization and harmonization efforts, which required significant work by all contributing sites, we recognize that the studies in this meta-analysis do still display methodological differences across sites. The criteria, scanners, acquisition parameters and suicidality assessment instruments were not strictly the same across groups. To test whether methodological differences explained a significant proportion of the variance in effect sizes across sites in the meta-analyses, we performed a series of meta-regressions, where each moderator variable (scanner field strength, voxel-size, FreeSurfer version used for image processing, temporality of the suicidality item and percentage of patients acutely depressed at the time of scanning) was separately included as a fixed-effect predictor in the model. The significance threshold for each moderator hypothesis was determined by false discovery rate procedure at q=0.05. Our results (shown in Supplementary Tables 6–11) showed that sample characteristics did not significantly moderate effect size estimates of brain volumes in any of the cross-group comparisons undertaken in this study. Nonetheless, our results should be confirmed in future large scale studies with improved and harmonized assessment of suicidality.
Given the considerable human and societal costs of suicide and suicidal behaviour, many countries have developed suicide-prevention programmes in recent years. However, the field faces several major challenges and there is an urgent need for biomarkers to aid in the prediction and prevention of suicidal behaviour. Neuroimaging methods provide a great opportunity to understand the structural and functional differences in the brains of individuals with psychiatric disorders and suicidal symptoms. However, as demonstrated here, major efforts might be needed to discover such biomarkers.
Future efforts could focus around longitudinal studies, which can provide insights about suicidality in the context of disease trajectories, and scanning of subjects before and after drug treatment and behavioural interventions to provide insights into disease mechanisms and treatment response. Finally, while this meta-analysis was restricted to subcortical grey matter volumes, ventricular and intracranial volumes, future studies should also try to replicate previously reported associations of cortical phenotypes with suicidality. In fact, future efforts within the ENIGMA-MDD working group will include coordinating a large meta-analysis of cortical phenotypes to identify potential biomarkers for suicidality in MDD, and we invite groups from around the world to join our efforts.
References
Bertolote JM, Fleischmann A, De Leo D, Bolhari J, Botega N, De Silva D et al. Suicide attempts, plans, and ideation in culturally diverse sites: the WHO SUPRE-MISS community survey. Psychol Med 2005; 35: 1457–1465.
Chang B, Gitlin D, Patel R . The depressed patient and suicidal patient in the emergency department: evidence-based management and treatment strategies. Emerg Med Pract 2011; 13: 1–23.
Hawton K, van Heeringen K . Suicide. Lancet 2009; 373: 1372–1381.
Lesage A, Moubarac JC, Desrochers L, Turecki G . Interdisciplinary dialogue on the etiology and prevention of suicide in Quebec. Sante Ment Que 2012; 37: 25–30.
van Heeringen K . Stress-diathesis model of suicidal behavior. In: Dwivedi Y (ed). The Neurobiological Basis of Suicide. CRC Press: Boca Raton, FL, USA, 2012.
Brent DA, Melhem N . Familial transmission of suicidal behavior. Psychiatr Clin North Am 2008; 31: 157–177.
Hawton K, Saunders KE, O'Connor RC . Self-harm and suicide in adolescents. Lancet 2012; 379: 2373–2382.
Qin P . The impact of psychiatric illness on suicide: differences by diagnosis of disorders and by sex and age of subjects. J Psychiatr Res 2011; 45: 1445–1452.
Rihmer Z . Suicide risk in mood disorders. Curr Opin Psychiatry 2007; 20: 17–22.
Richards D . Prevalence and clinical course of depression: a review. Clin Psychol Rev 2011; 31: 1117–1125.
Nock MK, Borges G, Bromet EJ, Cha CB, Kessler RC, Lee S . Suicide and suicidal behavior. Epidemiol Rev 2008; 30: 133–154.
Jacobson CM, Gould M . The epidemiology and phenomenology of non-suicidal self-injurious behavior among adolescents: a critical review of the literature. Arch Suicide Res 2007; 11: 129–147.
Lofthouse N, Muehlenkamp JJ, Adler R . Nonsuicidal self-injury and co-occurrence. In: Nixon MK, Heath NL (eds). Self-Injury in Youth: The Essential Guide to Assessment and Intervention. Routledge: New York, NY, USA, 2009, pp 59–78.
Fliege H, Lee J-R, Grimm A, Klapp BF . Risk factors and correlates of deliberate self-harm behavior: a systematic review. J Psychosom Res 2009; 66: 477–493.
American Psychiatric Association Diagnostic and Statistical Manual of Mental Disorders: DSM-5. American Psychiatric Association: Washington, DC, 2013.
Linker J, Gillespie NA, Maes H, Eaves L, Silberg JL . Suicidal ideation, depression, and conduct disorder in a sample of adolescent and young adult twins. Suicide Life Threat Behav 2012; 42: 426–436.
Roy A, Segal NL . Suicidal behavior in twins: a replication. J Affect Disord 2001; 66: 71–74.
Brent DA, Bridge J, Johnson BA, Connolly J . Suicidal behavior runs in families. A controlled family study of adolescent suicide victims. Arch Gen Psychiatry 1996; 53: 1145–1152.
Haw C, Hawton K . Life problems and deliberate self-harm: associations with gender, age, suicidal intent and psychiatric and personality disorder. J Affect Disord 2008; 109: 139–148.
Kendler KS, Karkowski LM, Prescott CA . Causal relationship between stressful life events and the onset of major depression. Am J Psychiatry 1999; 156: 837–841.
Pokorny AD . Prediction of suicide in psychiatric patients. Report of a prospective study. Arch Gen Psychiatry 1983; 40: 249–257.
Batterham PJ, Ftanou M, Pirkis J, Brewer JL, Mackinnon AJ, Beautrais A et al. A systematic review and evaluation of measures for suicidal ideation and behaviors in population-based research. Psychol Assess 2015; 27: 501–512.
van Heeringen C, Bijttebier S, Godfrin K . Suicidal brains: a review of functional and structural brain studies in association with suicidal behaviour. Neurosci Biobehav Rev 2011; 35: 688–698.
Monkul ES, Hatch JP, Nicoletti MA, Spence S, Brambilla P, Lacerda AL et al. Fronto-limbic brain structures in suicidal and non-suicidal female patients with major depressive disorder. Mol Psychiatry 2007; 12: 360–366.
Desmyter S, van Heeringen C, Audenaert K . Structural and functional neuroimaging studies of the suicidal brain. Prog Neuropsychopharmacol Biol Psychiatry 2011; 35: 796–808.
Zhang H, Chen Z, Jia Z, Gong Q . Dysfunction of neural circuitry in depressive patients with suicidal behaviors: a review of structural and functional neuroimaging studies. Prog Neuropsychopharmacol Biol Psychiatry 2014; 53: 61–66.
Martin PC, Zimmer TJ, Pan LA . Magnetic resonance imaging markers of suicide attempt and suicide risk in adolescents. CNS Spectr 2015; 20: 355–358.
Jollant F, Lawrence NS, Giampietro V, Brammer MJ, Fullana MA, Drapier D et al. Orbitofrontal cortex response to angry faces in men with histories of suicide attempts. Am J Psychiatry 2008; 165: 740–748.
Kim CD, Seguin M, Therrien N, Riopel G, Chawky N, Lesage AD et al. Familial aggregation of suicidal behavior: a family study of male suicide completers from the general population. Am J Psychiatry 2005; 162: 1017–1019.
Turecki G . Suicidal behavior: is there a genetic predisposition? Bipolar Disord 2001; 3: 335–349.
Nakagawa M, Kawanishi C, Yamada T, Sugiura K, Iwamoto Y, Sato R et al. Comparison of characteristics of suicide attempters with schizophrenia spectrum disorders and those with mood disorders in Japan. Psychiatry Res 2011; 188: 78–82.
Vang FJ, Ryding E, Traskman-Bendz L, van Westen D, Lindstrom MB . Size of basal ganglia in suicide attempters, and its association with temperament and serotonin transporter density. Psychiatry Res 2010; 183: 177–179.
Gifuni AJ, Ding Y, Olie E, Lawrence N, Cyprien F, Le Bars E et al. Subcortical nuclei volumes in suicidal behavior: nucleus accumbens may modulate the lethality of acts. Brain Imaging Behav 2015; 10: 96–104.
Thompson PM, Stein JL, Medland SE, Hibar DP, Vasquez AA, Renteria ME et al. The ENIGMA Consortium: large-scale collaborative analyses of neuroimaging and genetic data. Brain Imaging Behav 2014; 8: 153–182.
Schmaal L, Veltman DJ, van Erp TGM, Sämann PG, Frodl T, Jahanshad N et al. Subcortical brain alterations in major depressive disorder: findings from the enigma major depressive disorder working group. Mol Psychiatry 2015; 21: 806–812.
Fischl B, Salat DH, Busa E, Albert M, Dieterich M, Haselgrove C et al. Whole brain segmentation: automated labeling of neuroanatomical structures in the human brain. Neuron 2002; 33: 341–355.
Silverman MM . The language of suicidology. Suicide Life Threat Behav 2006; 36: 519–532.
Cox Lippard ET, Johnston JA, Blumberg HP . Neurobiological risk factors for suicide: insights from brain imaging. Am J Prev Med 2014; 47 (3 Suppl 2): S152–S162.
Nyholt DR . A simple correction for multiple testing for single-nucleotide polymorphisms in linkage disequilibrium with each other. Am J Hum Genet 2004; 74: 765–769.
Wagner G, Koch K, Schachtzabel C, Schultz CC, Sauer H, Schlosser RG . Structural brain alterations in patients with major depressive disorder and high risk for suicide: evidence for a distinct neurobiological entity? Neuroimage 2011; 54: 1607–1614.
Harris KM, Syu JJ, Lello OD, Chew YL, Willcox CH, Ho RH . The ABC's of suicide risk assessment: applying a tripartite approach to individual evaluations. PLoS ONE 2015; 10: e0127442.
Acknowledgements
The ENIGMA-Major Depressive Disorder working group gratefully acknowledges support from the NIH BD2K award, U54EB020403. MER, NGM, HC and IBH received support from the Australian National Health and Medical Research Council (NHMRC) Centre for Research Excellence on Suicide Prevention (CRESP) [GNT1042580]. QTIM: Australian National Health and Medical Research Council (Project Grants No. 496682 and 1009064 to MJW and Fellowship No. 464914 to IBH), US National Institute of Child Health and Human Development (RO1HD050735 to MJW) and US National Institute on Drug Abuse (R00DA023549 to NAG). BC-D is supported by a University of Queensland International PhD scholarship. NESDA: The infrastructure for the NESDA study (www.nesda.nl) is funded through the Geestkracht programme of the Netherlands Organisation for Health Research and Development (Zon-Mw, grant number 10-000-1002) and is supported by participating universities (VU University Medical Center, GGZ inGeest, Arkin, Leiden University Medical Center, GGZ Rivierduinen, University Medical Center Groningen) and mental health care organizations, see www.nesda.nl. LS is supported by The Netherlands Brain Foundation Grant number F2014(1)-24 and the Neuroscience Campus Amsterdam Scientific Excellence grant (IPB-SE-15-PSYCH-Schmaal). CODE: The CODE cohort was collected from studies funded by Lundbeck and the German Research Foundation (WA 1539/4-1, SCHN 1205/3-1). ES is supported by the Grant of the Deutsche Forschungsgemeinschaft/German Research Association (SCHR443/11-1). SHIP: The Study of Health in Pomerania (SHIP) is supported by the German Federal Ministry of Education and Research (grants 01ZZ9603, 01ZZ0103 and 01ZZ0403) the Ministry of Cultural Affairs as well as the Social Ministry of the Federal State of Mecklenburg-West Pomerania. magnetic resonance imaging scans were supported by Siemens Healthcare, Erlangen, Germany. SHIP-LEGEND was supported by the German Research Foundation (GR1912/5-1). CK received fees for an educational programme from Esparma/Aristo Pharma, Lilly, Servier and MagVenture, as well as travel support and speakers honoraria from Lundbeck and Servier. TGMvE consulted for Roche Pharmaceuticals in 2013–2014.
Author contributions
MER and LS were involved in the protocol design, quality testing and meta-analysis. All authors were involved in the data collection, processing, analysis and funding. All authors contributed in the edits and approved the content of this manuscript.
Author information
Authors and Affiliations
Consortia
Corresponding author
Ethics declarations
Competing interests
The authors declare no conflict of interest.
Additional information
Supplementary Information accompanies the paper on the Translational Psychiatry website
Supplementary information
Rights and permissions
This work is licensed under a Creative Commons Attribution-NonCommercial-ShareAlike 4.0 International License. The images or other third party material in this article are included in the article’s Creative Commons license, unless indicated otherwise in the credit line; if the material is not included under the Creative Commons license, users will need to obtain permission from the license holder to reproduce the material. To view a copy of this license, visit http://creativecommons.org/licenses/by-nc-sa/4.0/
About this article
Cite this article
Rentería, M., Schmaal, L., Hibar, D. et al. Subcortical brain structure and suicidal behaviour in major depressive disorder: a meta-analysis from the ENIGMA-MDD working group. Transl Psychiatry 7, e1116 (2017). https://doi.org/10.1038/tp.2017.84
Received:
Revised:
Accepted:
Published:
Issue Date:
DOI: https://doi.org/10.1038/tp.2017.84
This article is cited by
-
Neurophysiological stratification of major depressive disorder by distinct trajectories
Nature Mental Health (2023)
-
History of suicide attempt associated with amygdala and hippocampus changes among individuals with schizophrenia
European Archives of Psychiatry and Clinical Neuroscience (2023)
-
Connectome architecture modulates the gray matter atrophy in major depression disorder patients with diverse suicidal ideations
Brain Imaging and Behavior (2023)
-
Age- and gender-related dispersion of brain networks across the lifespan
GeroScience (2023)
-
Connectomics-based resting-state functional network alterations predict suicidality in major depressive disorder
Translational Psychiatry (2023)