Abstract
The objective of this study was to determine whether proteomic profiling in serum samples can be utilized in identifying and differentiating mood disorders. A consecutive sample of patients with a confirmed diagnosis of unipolar (UP n=52) or bipolar depression (BP-I n=46, BP-II n=49) and controls (n=141) were recruited. A 7.5-ml blood sample was drawn for proteomic multiplex profiling of 320 proteins utilizing the Myriad RBM Discovery Multi-Analyte Profiling platform. After correcting for multiple testing and adjusting for covariates, growth differentiation factor 15 (GDF-15), hemopexin (HPX), hepsin (HPN), matrix metalloproteinase-7 (MMP-7), retinol-binding protein 4 (RBP-4) and transthyretin (TTR) all showed statistically significant differences among groups. In a series of three post hoc analyses correcting for multiple testing, MMP-7 was significantly different in mood disorder (BP-I+BP-II+UP) vs controls, MMP-7, GDF-15, HPN were significantly different in bipolar cases (BP-I+BP-II) vs controls, and GDF-15, HPX, HPN, RBP-4 and TTR proteins were all significantly different in BP-I vs controls. Good diagnostic accuracy (ROC-AUC⩾0.8) was obtained most notably for GDF-15, RBP-4 and TTR when comparing BP-I vs controls. While based on a small sample not adjusted for medication state, this discovery sample with a conservative method of correction suggests feasibility in using proteomic panels to assist in identifying and distinguishing mood disorders, in particular bipolar I disorder. Replication studies for confirmation, consideration of state vs trait serial assays to delineate proteomic expression of bipolar depression vs previous mania, and utility studies to assess proteomic expression profiling as an advanced decision making tool or companion diagnostic are encouraged.
Similar content being viewed by others
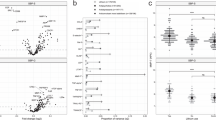
Introduction
Psychiatric diagnoses are still based on criteria that focus on behavioral observation and symptom endorsement without corresponding biological validation. This contrasts with other fields of medicine, where diagnosis and treatment are often based not only on a sound clinical examination, but also biological tests based on validated biomarkers. Biological markers, or biomarkers, are quantitative measurements that provide information about biological processes, a disease state or about response to treatment (Food and Drug Administration (FDA)ās Biomarkers Research Group definition).1 There is increasing interest in developing feasibility studies for biomarker identification in mood disorders.2
Initial studies in schizophrenia, bipolar disorder and major depressive disorder have highlighted the potential utility of multiplex biomarker development. These studies were primarily non-hypothesis driven, based on an established immune mediator and cytokine quantification platforms, and were predominantly compared with a healthy control population. While there has been initial validation, replication and development of classification decision rules in a series of studies in schizophrenia,3, 4, 5, 6 the majority of studies have not been comparative within mood disorders and have not corrected for multiple testing and adjusted for covariates, thus limiting their replication potential and overall generalizability.7, 8, 9, 10, 11, 12 This study was conducted with Myriad RBM Human Multi-Analyte Profiling (MAP) platform to assess the feasibility of MAP in distinguishing (vs healthy controls) and differentiating subgroups of mood disorder patients.
Materials and methods
This study was approved by the Mayo Clinic Institutional IRB (IRB number: 10-005352, principal investigator: Mark A. Frye). All participants provided written informed consent prior to enrollment, evaluation and biomarker blood draw.
Subjects
A consecutive sample of treatment-seeking adult (age 18ā65) depressed patients from 9 May 2011 to 14 April 2014 were recruited from the Mayo Clinic Depression Center (Figure 1). Additional inclusion criteria included: diagnoses of major or bipolar I/II depression were confirmed by DSM IV TR Structured Clinical Diagnostic Interview (SCID).13 Exclusion criteria included: inability to provide written informed consent, other Axis I or II diagnoses that by clinical judgement were the main reason for seeking treatment, current substance use disorder determined by drug screen (except nicotine and caffeine), unipolar (UP) patients with first degree relative with bipolar disorders, acute unstable medical illness, inflammatory disease (that is, rheumatological, autoimmune), chronic pain, chronic use of non-steroidal or any anti-inflammatory drugs, systemic corticosteroids within the past 4 weeks, monoclonal antibody therapy within the past 3 months, acute infection or chronic infection requiring non-topical anti-infective agent, history of cancer with chemotherapy or radiation in the past year, and pregnant or lactating women.
Non-mood controls age 18ā65 with no evidence of acute unstable medical illness, current or historic psychiatric diagnosis or first degree relative with psychiatric diagnosis were recruited from the community through newspaper, flyer, brochure and web-based advertisement (n=141).
Protocol for drug screening
A urine drug screen was performed by study personnel using a One Step Multi-Drug Urine Test Panel kit (W.H.P.M., Irwindale, CA, USA) to screen for any current illicit substances after participants signed the study consent form. If illicit substances were detected in mood subjects, they were excluded from study unless the substance was medically prescribed (that is, benzodiazepines (Table 1) and stimulants (n=1)). If illicit substances were detected in healthy controls or patients, they were excluded from study (n=1). If a participant was currently an inpatient and completed a drug screen during their inpatient stay, the results of that urinalysis were used instead of requiring the participant to complete a second drug screen.
Clinical assessment
Clinical assessments completed at the time of blood draw included: Inventory For Depressive Symptoms (IDS),14 the Young Mania Rating Scale (YMRS),15 Patient Health Questionnaire (PHQ-9),16 Generalized Anxiety Disorder 7-item scale (GAD-7)17 and Alcohol Use Disorders Identification Test (AUDIT).18 Current medications were recorded at the time of blood draw from the patientās electronic medical record and/or from the patient directly. In addition to current urine toxicology evaluation, additional substance use was quantified specifically lifetime alcohol use (self-report of never, occasional, regular and abuse), current cigarette smoking (yes/no) and lifetime history of illicit drug abuse (yes/no).
Multi-analyte profiling
Myriad RBM is a CLIA-certified biomarker testing laboratory based in Austin, TX, USA. Their DiscoveryMAP is a quantitative multiplexed immunoassay service product, based on Luminex xMAP technology platform (for more information about the development of this platform see Supplementary online-text).19, 20 The current platform, based initially on pharmaceutical and biotechnological opportunities, focused on immune mediator and cytokine quantification for drug development has continued to expand and now includes 320 proteins (see Supplementary eTable 1).21, 22, 23 These same inflammatory and immune mediated biomarkers are increasingly recognized in the underlying neurobiology of mood disorders.7, 8, 9, 10, 11, 12
Using standard phlebotomy techniques and established laboratory protocol, the samples (~7.5āml) were collected into serum-separating tubes. No adverse events were reported. Fasting vs nonāfasting status and collection time (0600ā1730 hours) was recorded on each sample. The time from blood draw to blood sample freeze was less than 1āh for all samples. All specimens collected at Mayo Clinic were packaged and sent overnight on dry ice to Myriad RBM. Shipped samples contained no direct identifiers and Myriad RBM was blind to plate identification and case vs control status.
About 288 serum samples (141 controls, 52 unipolar, 49 bipolar II, 46 bipolar I) were randomized to 4 plates (72 wells Ć 4). There was no difference in plate by group allocation and age of sample (from collection to analysis, R Kaldate, personal communication). Proteins (n=320) were measured in 250āμl serum samples using the DiscoveryMAP multiplexed immunoassays in the CLIA-certified laboratory at Myriad RBM. Assays were calibrated using duplicate eight-point standard curves and raw intensity measurements were converted to absolute protein concentrations using proprietary software. Assay performance was verified using quality control samples at low, medium and high levels for each analyte. All standard and quality control samples were analyzed in a complex matrix to match the sample background. All study protocols are in compliance with Standards for Reporting of Diagnostic Accuracy (STARD) initiative.24
Statistical analysis
The relationships between clinical/demographic variables and diagnosis of mood disorder (cases vs controls) were modeled first using logistic regression models, testing the relationship of individual variables using likelihood ratio tests. Multinomial regression was used to model the relationship of the clinical/demographic variables with the mood disorder diagnoses (BP-I, BP-II and UP), and likelihood ratio tests were used to evaluate statistical significance.
For proteins with measurements below the Lower Limit of Quantification (LLOQ), to test for a relationship between protein levels and the clinical/demographic factors listed in (Supplementary eTable 2 in the Supplement), values less than the LLOQ were set to LLOQ/2. Analyte levels ⩾90% (n=48) below the LLOQ were excluded from analysis, leaving 272 proteins included in the study. There were also a few values above the upper limit of detection and they were set to the upper limit of detection. Since this imputation led to a distribution with a point mass at LLOQ/2 for some of the proteins, non-parametric tests were used to test for association between protein levels and covariates. Wilcoxon tests were used for categorical predictors, while Spearman correlation was used for continuous predictors, with an exact test approximation based on Monte Carlo simulation.
A number of the clinical/demographic variables were associated with levels of certain proteins (Supplementary eTable 2 in the Supplement). Therefore, when evaluating the association of diagnosis with protein levels, models were adjusted for current smoking status, history of illicit drug use, age, body mass index and years of education by including these variables as covariates. While not different in case vs control or within case analyses, we also adjusted for gender, lifetime alcohol use and fasting status because of their potential association with proteins. To model the relationships between diagnosis and proteins, first multinomial logistic regression was used to evaluate whether protein levels differ among the four diagnosis categories (BP-I vs BP-II vs UP vs control). Rather than imputing protein values below the LLOQ, the effect of the protein was modeled using two parameters corresponding to: (1) an indicator of a protein value being below the LLOQ, and (2) the actual protein value if the protein value was above the LLOQ, or zero otherwise (that is, the interaction of I*protein level, where I denotes the indicator variable for protein level>LLOQ). Sensitivity analyses were conducted imputing protein values less than the LLOQ using LLOQ/2, with similar results (data not shown). P-values were corrected for multiple testing using the Bonferroni method, thus P=0.05/272=1.84eā04 was considered statistically significant.
A series of post hoc pairwise comparisons between particular diagnoses (for example, BP-I+BP-II+UP vs controls, BP-I+BP-II vs controls, BP-I vs controls) were used to identify specific differences between diagnostic groups that contributed to significant results in the multinomial analyses of the four groups. For the pairwise comparisons, logistic regression was used to model probability of the two diagnoses using individual proteins as predictors, adjusting for the same covariates as in the multinomial analyses. To address multiple testing in these post hoc comparisons, the Bonferroni method was used, by further correcting the experiment-wise control of type I error corrected for 272 proteins (that is, 1.84eā04). Because only particular pairs of diagnoses were compared, three comparisons were accounted for in this multiple testing correction (P=1.84eā04/3=6.13eā05). While this was a pilot study, we have 80% power to detect odds ratios for predicting: mood disorders vs controls, bipolar disease vs controls and bipolar I disease vs controls of 1.72, 1.84 and 2.18 per s.d. increase in protein level assuming a type I error rate of 1.84Eā04 (that is, the Bonferonni-corrected 5% error rate). While medication status was not considered in the primary analysis due to a high degree of confounding with diagnosis, a visual inspection of protein level differences was conducted by medication status (yes vs no for antipsychotics, lithium, sedatives/hypnotics, antidepressants and antiepileptic mood stabilizers) for each protein that was significantly different by diagnostic group.
To evaluate the predictive performance of the models, the area under the curve (AUC) of the receiver operating characteristic (ROC) curve was calculated from the logistic regression models based on the C-statistic. All analyses were completed using R v3.1.1 (Vienna, Austria); multinomial models were fit using 'nnet' R package v7.3-8 (New York, NY, USA), and C-statistic calculated using the 'rms' R package v4.2-1 (Nashville, TN, USA).
Results
As shown in (Table 1), cases had significantly higher age, body mass index, current smoking and history of illicit drug use, as well as less years of education than controls. For all the clinical assessment comparisons, there were significant differences between cases and controls, but no difference between cases in moderate symptoms of depression, anxiety and alcohol screen; there was, however, a higher score on the YMRS, implying mix depressive symptoms in bipolar II patients.
Of the 272 proteins measured and analyzed from 288 samples (141 controls, 52 Unipolar, 49 Bipolar II, 46 Bipolar I), 73 showed nominally significant difference among the 4 compared groups at an uncorrected significance level (P<0.05. see bold font in Supplementary Table 1); 6 of those proteins showed statistically significant differences after Bonferroni correction (P<0.05/272=1.84eā04) (growth differentiation factor 15 (GDF-15), hemopexin (HPX), hepsin (HPN), matrix metalloproteinase-7 (MMP-7), retinol-binding protein 4 (RBP-4) and transthyretin (TTR)). Figure 2 shows the values of those 6 proteins by diagnosis, demonstrating that the protein levels were higher in BP-I vs all other compared groups and controls. As presented in Table 2, a series of three post hoc analyses were performed identifying greater statistical differences in the bipolar I vs control analyses. GDF-15, RBP-4 and TTR were good predictors of BP-I with ROC-AUC of 0.81, while HPX and HPN were fair predictors of BP-I with ROC-AUC of 0.74 and 0.78, respectively. The significant protein means and s.d. in each group, as well as fold changes between compared groups is demonstrated in Table 3.
Comparison of proteins levels among groups (BP-I= 46, BP-II=49, UP=52, controls=141). All six proteins levels were higher in BP-I vs all other compared groups and controls. Five proteins were significantly different in BP-I vs controls GDF-15 P=0.0278, HPX P=0.0221, HPN P=0.0156, RBP-4 P=0.0001 and TTR P=0.0012. BP, bipolar depression; GDF-15, growth differentiation factor 15; HPN, hepsin; HPX, hemopexin; MMP-7, matrix metalloproteinase-7; RBP-4, retinol-binding protein 4; TTR, transthyretin.
For the six proteins significantly associated with mood disorders, values of proteins by diagnosis and medication groups (antipsychotics, lithium, antiepileptic mood stabilizers, antidepressants, sedatives/hypnotics) are presented (Supplementary eFigure 1 in the Supplement). These comparisons suggest that the effect may be driven by the diagnostic group, rather than medication. Although some differences appear to be further augmented by certain medications, differences between BP-I cases and controls (not on medication) appear to still be present for patients that are currently not taking these medications.
Discussion
To our knowledge, this is the first study to assess the feasibility of high throughput multiplexed immunoassay technology (that is 272 proteins) for studying mood disorders. Taking advantage of an established platform based initially on immune mediator and cytokine quantification, increasingly recognized in the underlying neurobiology of mood disorders,4, 5 we have identified six proteins, all expressed in brain,25, 26 that distinguish mood disorder patients, particularly bipolar I patients from healthy controls. After adjusting for several possible confounders, 73 proteins differed among the 4 studied groups at an uncorrected significance level (see Supplementary Table 2), with GDF-15, HPX, HPN, MMP-7, RBP-4 and TTR showing statistically significant differences after conservative Bonferroni correction. Furthermore, GDF-15, RBP-4 and TTR were good predictors of BP-I with ROC-AUC>0.8. Although these discovery results need to be replicated in independent samples, this study demonstrates feasibility of a multiplex blood-based testing for bipolar disorder. This investigation has both identified new proteins possibly implicated in mood disorder and further refines our previous understanding of specific proteins in mood disorder.
GDF-15 plays a role as a growth factor as well as immune modulator, and has been implicated in cognitive decline.27, 28 HPX is a type II acute phase reactant glycoprotein and functions to bind heme, facilitate antioxidation and maintain iron homeostasis.29 HPX increases in preclinical early life stress models,30 and has been shown previously to be significantly elevated in bipolar and schizophrenic patients in comparison to controls.31 HPN is a type II membrane serine protease and might play a role in blood coagulation.32 To our knowledge, HPN association with mood disorders is reported here for the first time. MMP-7 is a metalloproteinase and its functions include breaking down of extracellular matrix, degrading proteoglycans, fibronectin, elastin and casein.33 MMP-7 cleaves synaptosomal-associated protein of 25 kDa (SNAP-25), an intraneuronal protein that is important for neurotransmitter release.34 This gene (rs6039769 variant) was also reported to be associated with early onset bipolar disorder.35
RBP-4 is a mainly expressed in liver with a primary function to transport retinol (vitamin A) from the liver to peripheral tissues.36 Vitamin A is essential for the brain to facilitate learning, memory and cognition37 and Rbp4ā/ā knockout mice have cortical and hippocampal (CA3) neuronal loss and gliosis.38 Although a vitamin A bipolar disorder hypothesis has not been studied before, an earlier study reported low maternal vitamin A and an association with schizophrenia among exposed offsprings.39 There is one prior study that reported decreased RBP-4 is unipolar depressed patients; there was no bipolar comparison group.40
TTR is a homotetrameric protein synthesized by the liver, retinal pigment epithelium and choroid plexus.41 Present in cerebrospinal fluid (CSF), TTR transports tetraiodothyronine (T4) across bloodābrain barrier.42 TTR locus, 18q12, has been implicated in bipolar disorder in a Danish pedigree of bipolar patients.43 Chronic administration of lithium to rats caused a 16-fold decrease in TTR,44 which differs from our data that suggest lithium-treated patients had an increase in TTR. Interestingly, neuropeptide Y has been found to be increased in both TTR knockout rats,45 and after prolonged lithium administration.46 Several studies have reported reduced CSF TTR in depressed patients,47, 48, 49 but this has not been confirmed in other investigations.50 In a recent clinical trial, it is been shown that bipolar depressed female patients treated with high dose levothyroxine (mean dose 325āμg) had a significant reduction in depressive symptoms in comparison to adjunctive placebo; our data could explain the therapeutic mechanism-of-action (that is, greater ability to transport more T4 via TTR to brain).51 However, TTR knockout mouse models are associated with reduced depressive-like behavior, which may be related to increased noradrenergic modulation in limbic forebrain.52 Interestingly, RBP-4 transports vitamin A by forming a complex with TTR (RBP-4/TTR). Taken together, RBP-4/TTR complex, thyroxine and vitamin A are all present in the CSF and participate in brain maturation and, cognitive, acquisition of memory and behavioral activities and may be implicated in mood disorders.50
Although this is the first attempt to study both bipolar I/II and unipolar depression, other studies have utilized MAP of specific mood disorders. Schwarz et al.,4 reported 20 proteins that were differentially expressed in pre-symptomatic BP subjects (n=110) vs controls. However, this study by Schwarz and colleagues did not correct for multiple tastings in the analysis and was not assessing symptomatic (i.e., depressed) patients. Domenici et al.,3 reported that insulin and MMP-9 were significantly higher in patients with major depressive disorder (n=245), vs controls. Although the P-value threshold was corrected for multiple testing, current mood state or symptom severity of the cases were not reported. Herberth et al.53 comparing euthymic BP patients (n=32) vs controls, identified three proteins (chemokine C-C motif ligand 2, endothelin-1, macrophage migration inhibitory factor) that showed statistically significant difference after correcting for multiple testing. While Stelzhammer et al.,54 performed a similar study on antidepressant drug-naĆÆve unipolar patients (n=38) and identified 11 and 2 differentially expressed proteins utilizing multi-analyte profiling platform and liquid chromatography-mass spectrometry, respectively,48 the results were not corrected for multiple testing. The top results from both aforementioned studies did not match with our top findings.53 Haenisch et al.,55 reported 26 proteins, including MMP-7, which were differentially expressed in bipolar patients (n=17) vs controls. Similar to our data, the majority of these patients were BP-I with current or recent depression. Taken together, these previous studies either did not consider mood state in phenotyping, would likely not retain statistical significance after accounting for multiple testing correction or did not have access to this large of a proteomic platform.
The strengths of this study include a single recruitment site for depression treatment-seeking patients and rigorous exclusion criteria to eliminate non-specific inflammatory contributions from systemic illness and anti-inflammatory/biotic drug therapy. While there was no evidence of different level of symptom severity of anxiety, depression, alcohol use, between case groups, we did not conduct a protein level analysis with index episode duration, previous treatment trials nor SCID lifetime assessment of psychiatric diagnoses. The lifetime assessment of manic illness burden (that is, episodes), history of psychosis or age of illness onset may have provided greater understanding of the striking BP-I proteomic expression. The multiple correction testing was conservative. Furthermore, the more conservative adjustment for multiple covariates not commonly done in cross-sectional biomarker mood studies (fasting status, years education, lifetime drug abuse history and lifetime alcohol use) may have reduced power. Nonetheless, even after adjustment for these variables, this discovery has identified a number of proteins that differ significantly in patients with mood disorders, in particular bipolar I disorder.
One limitation of this study relates to the cross-sectional design without serial measurement. A second measurement when euthymic could address mood state-dependent proteomic expression, and clarify whether the proteomic expression is representative of bipolar depression, or trait or burden of previous mania/ hypomania-associated brain changes.56, 57 Another limitation of this study relates to the lack of statistical analyses, evaluating the effects of medication use on the observed protein level differences between diagnostic groups. This was not formally tested because of the lack of drug-naĆÆve patients, and small sample size. Our exploratory descriptive data (Supplementary eFigure 1 in the supplements) suggest that medication status was not the primary driver of protein expression; however, this was not rigorously assessed. While disease, drug or an interaction between the two can change analyte or proteomic expression, none of the identified proteins have been identified, to our knowledge, in a drug mechanism-of-action or pharmacokinetic/pharmacodynamically mediated therapeutic drug response. The lack of association with thyroid hormone replacement, which was more prevalent in our BP-I vs BP-II and UP patient groups, and TTR has been confirmed.58, 59
While patients in the population are rarely treatment naĆÆve, conducting similar studies in first index episode major depression where bipolar disorder is in the differential diagnosis has merit. Results of such studies could have significant clinical implications, aiding clinicians in selecting unimodal antidepressants vs FDA-approved bipolar depression treatments.60 Additional biomarker clinical verification (that is, replication of proteins distinguishing mood disorder subtypes) and utility studies (that is, proteomic-based clinical outcome or decision tool) are encouraged. A proteomic-based differential diagnosis as an advanced decision making tool or companion diagnostic to guide evidence-based algorithms for mood stabilizer vs unimodal antidepressant therapy would have great clinical impact.
In conclusion, the results of this feasibility study support the possibility of developing a diagnostic test using the discovered biomarkers, which need to be validated, to help facilitate accurate diagnosis and rapid treatment initiation with improved clinical outcomes. Further functional studies of the identified proteins will increase our understanding of the pathophysiology of mood disorders, which may lead to the discovery of novel pharmacological targets.
References
Biomarkers Definitions Working Group. Biomarkers and surrogate endpoints: preferred definitions and conceptual framework. Clin Pharmacol Ther 2001; 69: 89ā95.
Frey BN, Andreazza AC, Houenou J, Jamain S, Goldstein BI, Frye MA et al. Biomarkers in bipolar disorder: a positional paper from the international society for bipolar disorders biomarkers task force. Aust NZ J Psychiatry 2013; 47: 321ā332.
Domenici E, Wille DR, Tozzi F, Prokopenko I, Miller S, McKeown A et al. Plasma protein biomarkers for depression and schizophrenia by multi analyte profiling of case-control collections. PLoS One 2010; 5: e9166.
Schwarz E, Izmailov R, Spain M, Barnes A, Mapes JP, Guest PC et al. Validation of a blood-based laboratory test to aid in the confirmation of a diagnosis of schizophrenia. Biomark Insights 2010; 5: 39ā47.
Vapnik VN . An overview of statistical learning theory. IEEE Trans Neural Netw 1999; 10: 988ā999.
Schwarz E, Guest PC, Rahmoune H, Harris LW, Wang L, Leweke FM et al. Identification of a biological signature for schizophrenia in serum. Mol Psychiatry 2012; 17: 494ā502.
Khandaker GM, Pearson RM, Zammit S, Lewis G, Jones PB . Association of serum interleukin 6 and C-reactive protein in childhood with depression and psychosis in young adult life: a population-based longitudinal study. JAMA Psychiatry 2014; 71: 1121ā1128.
Setiawan E, Wilson AA, Mizrahi R, Rusjan PM, Miler L, Rajkowska G et al. Role of translocator protein density, a marker of neuroinflammation, in the brain during major depressive episodes. JAMA Psychiatry 2015; 72: 268ā275.
Raison CL, Miller AH . Role of inflammation in depression: implications for phenomenology, pathophysiology and treatment. Mod Trends Pharmacopsychiatri 2013; 28: 33ā48.
Raison CL, Rutherford RE, Woolwine BJ, Shuo C, Schettler P, Drake DF et al. A randomized controlled trial of the tumor necrosis factor antagonist infliximab for treatment-resistant depression: the role of baseline inflammatory biomarkers. JAMA Psychiatry 2013; 70: 31ā41.
Walker AJ, Kim Y, Price JB, Kale RP, McGillivray JA, Berk M et al. Stress, inflammation, and cellular vulnerability during early stages of affective disorders: biomarker strategies and opportunities for prevention and intervention. Front Psychiatry 2014; 5: 34.
Li H, Hong W, Zhang C, Wu Z, Wang Z, Yuan C et al. IL-23 and TGF-beta1 levels as potential predictive biomarkers in treatment of bipolar I disorder with acute manic episode. J Affect Disord 2015; 174: 361ā366.
First MB, Spitzer RL, Gibbon M, Gibbon W, Janet BW . Structured Clinical Interview for DSM-IV-TR Axis I Disorders, Research Version, Patient Edition (SCID-I/P). Biometrics Research, New York State Psychiatric Institute: New York, NY, USA, 2002.
Rush AJ, Gullion CM, Basco MR, Jarrett RB, Trivedi MH . The Inventory of Depressive Symptomatology (IDS): psychometric properties. Psychol Med 1996; 26: 477ā486.
Young RC, Biggs JT, Ziegler VE, Meyer DA . A rating scale for mania: reliability, validity and sensitivity. Br J Psychiatry 1978; 133: 429ā435.
Spitzer RL, Kroenke K, Williams JB . Validation and utility of a self-report version of PRIME-MD: the PHQ primary care study. Primary care evaluation of mental disorders. patient health questionnaire. JAMA 1999; 282: 1737ā1744.
Spitzer RL, Kroenke K, Williams JB, Lowe B . A brief measure for assessing generalized anxiety disorder: the GAD-7. Arch Intern Med 2006; 166: 1092ā1097.
Saunders JB, Aasland OG, Babor TF, de la Fuente JR, Grant M . Development of the alcohol use disorders identification test (AUDIT): WHO collaborative project on early detection of persons with harmful alcohol consumptionāII. Addiction 1993; 88: 791ā804.
Krishhan VV, Khan IH, Luciw PA . Multiplexed microbead immunoassays by flow cytometry for molecular profiling: Basic concepts and proteomics applications. Crit Rev Biotechnol 2009; 29: 29ā43.
Chowdhury F, Williams A, Johnson P . Validation and comparison of two multiplex technologies, luminex and mesoscale discovery, for human cytokine profiling. J Immunol Methods 2009; 340: 55ā64.
Statland J, Donlin-Smith CM, Tapscott SJ, van der Maarel S, Tawil R . Multiplex screen of serum biomarkers in facioscapulohumeral muscular dystrophy. J Neuromuscul Dis 2014; 1: 181ā190.
Llano DA, Devanarayan V, Simon AJ . Evaluation of plasma proteomic data for Alzheimer disease state classification and for the prediction of progression from mild cognitive impairment to Alzheimer disease. Alzheimer Dis Assoc Disord 2013; 27: 233ā243.
Walcott BP, Patel AP, Stapleton CJ, Trivedi RA, Young AM, Ogilvy CS . Multiplexed protein profiling after aneurysmal subarachnoid hemorrhage: characterization of differential expression patterns in cerebral vasospasm. J Clin Neurosci 2014; 21: 2135ā2139.
Bossuyt PM, Reitsma JB, Bruns DE, Gatsonis CA, Glasziou PP, Irwig LM et al. Towards complete and accurate reporting of studies of diagnostic accuracy: the STARD initiative. Standards for reporting of diagnostic accuracy. Clin Chem 2003; 49: 1ā6.
Karolchik D, Barber GP, Casper J, Clawson H, Cline MS, Diekhans M et al. The UCSC genome browser database: 2014 update. Nucleic Acids Res 2014; 42: D764āD770.
Su AI, Wiltshire T, Batalov S, Lapp H, Ching KA, Block D et al. A gene atlas of the mouse and human protein-encoding transcriptomes. Proc Natl Acad Sci USA 2004; 101: 6062ā6067.
Liu HT, Wang HC, Tao L, Li CX, Li F, Zhang YY et al. Stress-induced growth-differentiation factor 15 plays an intriguing role in cardiovascular diseases. Chin Med J (Engl) 2013; 126: 1350ā1354.
Fuchs T, Trollor JN, Crawford J, Brown DA, Baune BT, Samaras K et al. Macrophage inhibitory cytokine-1 is associated with cognitive impairment and predicts cognitive declineāthe Sydney Memory and Aging Study. Aging Cell 2013; 12: 882ā889.
Delanghe JR, Langlois MR . Hemopexin: a review of biological aspects and the role in laboratory medicine. Clin Chim Acta 2001; 312: 13ā23.
Maestre JR, Mateo M, Sanchez P . [Bacteremia after periodontal procedures]. Rev Esp Quimioter 2008; 21: 153ā156.
Maes M, Delange J, Ranjan R, Meltzer HY, Desnyder R, Cooremans W et al. Acute phase proteins in schizophrenia, mania and major depression: modulation by psychotropic drugs. Psychiatry Res 1997; 66: 1ā11.
Wu Q, Parry G . Hepsin and prostate cancer. Front Biosci 2007; 12: 5052ā5059.
Ii M, Yamamoto H, Adachi Y, Maruyama Y, Shinomura Y . Role of matrix metalloproteinase-7 (matrilysin) in human cancer invasion, apoptosis, growth, and angiogenesis. Exp Biol Med (Maywood) 2006; 231: 20ā27.
Szklarczyk A, Oyler G, McKay R, Gerfen C, Conant K . Cleavage of neuronal synaptosomal-associated protein of 25 kDa by exogenous matrix metalloproteinase-7. J Neurochem 2007; 102: 1256ā1263.
Etain B, Dumaine A, Mathieu F, Chevalier F, Henry C, Kahn JP et al. A SNAP25 promoter variant is associated with early-onset bipolar disorder and a high expression level in brain. Mol Psychiatry 2010; 15: 748ā755.
Kotnik P, Fischer-Posovszky P, Wabitsch M . RBP4: a controversial adipokine. Eur J Endocrinol 2011; 165: 703ā711.
Goodman AB . Retinoid receptors, transporters, and metabolizers as therapeutic targets in late onset Alzheimer disease. J Cell Physiol 2006; 209: 598ā603.
Buxbaum JN, Roberts AJ, Adame A, Masliah E . Silencing of murine transthyretin and retinol binding protein genes has distinct and shared behavioral and neuropathologic effects. Neuroscience 2014; 275: 352ā364.
Bao Y, Ibram G, Blaner WS, Quesenberry CP, Shen L, McKeague IW et al. Low maternal retinol as a risk factor for schizophrenia in adult offspring. Schizophr Res 2012; 137: 159ā165.
Maes M, Scharpe S, Van Grootel L, Uyttenbroeck W, Cooreman W, Cosyns P et al. Higher alpha 1-antitrypsin, haptoglobin, ceruloplasmin and lower retinol binding protein plasma levels during depression: further evidence for the existence of an inflammatory response during that illness. J Affect Disord 1992; 24: 183ā192.
Raghu P, Sivakumar B . Interactions amongst plasma retinol-binding protein, transthyretin and their ligands: implications in vitamin A homeostasis and transthyretin amyloidosis. Biochim Biophys Acta 2004; 1703: 1ā9.
Prapunpoj P, Leelawatwattana L . Evolutionary changes to transthyretin: structure-function relationships. FEBS J 2009; 276: 5330ā5341.
Ewald H, Mors O, Koed K, Eiberg H, Kruse TA . Susceptibility loci for bipolar affective disorder on chromosome 18? A review and a study of Danish families. Psychiatr Genet 1997; 7: 1ā12.
Pulford DJ, Adams F, Henry B, Mallinson DJ, Reid IC, Stewart CA . Chronic lithium administration down regulates transthyretin mRNA expression in rat choroid plexus. Neuropsychiatr Dis Treat 2006; 2: 549ā555.
Nunes AF, Saraiva MJ, Sousa MM . Transthyretin knockouts are a new mouse model for increased neuropeptide Y. FASEB J 2006; 20: 166ā168.
Zachrisson O, Mathe AA, Stenfors C, Lindefors N . Region-specific effects of chronic lithium administration on neuropeptide Y and somatostatin mRNA expression in the rat brain. Neurosci Lett 1995; 194: 89ā92.
Hatterer JA, Herbert J, Hidaka C, Roose SP, Gorman JM . CSF transthyretin in patients with depression. Am J Psychiatry 1993; 150: 813ā815.
Sullivan GM, Hatterer JA, Herbert J, Chen X, Roose SP, Attia E et al. Low levels of transthyretin in the CSF of depressed patients. Am J Psychiatry 1999; 156: 710ā715.
Sullivan GM, Mann JJ, Oquendo MA, Lo ES, Cooper TB, Gorman JM . Low cerebrospinal fluid transthyretin levels in depression: correlations with suicidal ideation and low serotonin function. Biol Psychiatry 2006; 60: 500ā506.
Fleming CE, Nunes AF, Sousa MM . Transthyretin: more than meets the eye. Prog Neurobiol 2009; 89: 266ā276.
Stamm TJ, Lewitzka U, Sauer C, Pilhatsch M, Smolka MN, Koeberle U et al. Supraphysiologic doses of levothyroxine as adjunctive therapy in bipolar depression: a randomized, double-blind, placebo-controlled study. J Clin Psychiatry 2014; 75: 162ā168.
Sousa JC, Grandela C, Fernandez-Ruiz J, de Miguel R, de Sousa L, Magalhaes AI et al. Transthyretin is involved in depression-like behaviour and exploratory activity. J Neurochem 2004; 88: 1052ā1058.
Herberth M, Koethe D, Levin Y, Schwarz E, Krzyszton ND, Schoeffmann S et al. Peripheral profiling analysis for bipolar disorder reveals markers associated with reduced cell survival. Proteomics 2011; 11: 94ā105.
Stelzhammer V, Haenisch F, Chan MK, Cooper JD, Steiner J, Steeb H et al. Proteomic changes in serum of first onset, antidepressant drug-naive major depression patients. Int J Neuropsychopharmacol 2014; 17: 1599ā1608.
Haenisch F, Alsaif M, Guest PC, Rahmoune H, Dickerson F, Yolken R et al. Multiplex immunoassay analysis of plasma shows prominent upregulation of growth factor activity pathways linked to GSK3beta signaling in bipolar patients. J Affect Disord 2014; 156: 139ā143.
Frye MA, Thomas MA, Yue K, Binesh N, Davanzo P, Ventura J et al. Reduced concentrations of N-acetylaspartate (NAA) and the NAA-creatine ratio in the basal ganglia in bipolar disorder: a study using 3-Tesla proton magnetic resonance spectroscopy. Psychiatry Res 2007; 154: 259ā265.
Altshuler LL . Bipolar disorder: are repeated episodes associated with neuroanatomic and cognitive changes? Biol Psychiatry 1993; 33: 563ā565.
Franklyn JA, King S, Ahlquist JA, Sheppard MC . Effect of hypothyroidism and thyroid hormone treatment of the rat on hepatic Spot 14 and thyroxine binding prealbumin mRNAs. Acta Endocrinol (Copenh) 1989; 121: 383ā388.
Blay P, Nilsson C, Owman C, Aldred A, Schreiber G . Transthyretin expression in the rat brain: effect of thyroid functional state and role in thyroxine transport. Brain Res 1993; 632: 114ā120.
Frye MA . Clinical practice. Bipolar disorderāa focus on depression. N Engl J Med 2011; 364: 51ā59.
Acknowledgements
We thank study participants and Sheri Sutor for processing samples for this investigation. Data access, responsibility and analysis: MAF had full access to all the data in the study and takes responsibility for the integrity of the data and the accuracy of the data analysis. All data analyses were completed by Mayo Clinic.
Author information
Authors and Affiliations
Corresponding author
Ethics declarations
Competing interests
Funding for the study was provided by Myriad RBM. All statistical analyses were completed by Mayo Clinic. MAF has received previous grant support from Assurex Health, Myriad, Pfizer, the National Institute of Mental Health (RO1 MH079261), the National Institute of Alcohol Abuse and Alcoholism (P20AA017830) and Mayo Foundation that did not support the work reported in this manuscript; has been a consultant to Janssen Global Services, Mitsubishi Tanabe Pharma Corporation, Myriad, Sunovion and Teva Pharmaceuticals; has received CME/Travel Support/presentation from CME Outfitters and Sunovian. The remaining authors have no conflict of interest.
Additional information
Supplementary Information accompanies the paper on the Translational Psychiatry website
Supplementary information
Rights and permissions
This work is licensed under a Creative Commons Attribution 4.0 International License. The images or other third party material in this article are included in the articleās Creative Commons license, unless indicated otherwise in the credit line; if the material is not included under the Creative Commons license, users will need to obtain permission from the license holder to reproduce the material. To view a copy of this license, visit http://creativecommons.org/licenses/by/4.0/
About this article
Cite this article
Frye, M., Nassan, M., Jenkins, G. et al. Feasibility of investigating differential proteomic expression in depression: implications for biomarker development in mood disorders. Transl Psychiatry 5, e689 (2015). https://doi.org/10.1038/tp.2015.185
Received:
Revised:
Accepted:
Published:
Issue Date:
DOI: https://doi.org/10.1038/tp.2015.185
This article is cited by
-
Altered circulating GDF-15 level predicts sex hormone imbalance in males with major depressive disorder
BMC Psychiatry (2023)
-
High mobility group box 1 and a network of other biomolecules influence fatigue in patients with Crohnās disease
Molecular Medicine (2023)
-
Classifying and clustering mood disorder patients using smartphone data from a feasibility study
npj Digital Medicine (2023)
-
Alterations in blood proteins in the prodromal stage of bipolar II disorders
Scientific Reports (2022)
-
Peripheral biomarkers to predict the diagnosis of bipolar disorder from major depressive disorder in adolescents
European Archives of Psychiatry and Clinical Neuroscience (2022)