Abstract
Ventilator-associated pneumonia (VAP) is one of the commonest hospital-acquired infections associated with high mortality. VAP pathogenesis is closely linked to organisms colonizing the endotracheal tube (ETT) such as Staphylococcus epidermidis and Pseudomonas aeruginosa, the former a common commensal with pathogenic potential and the latter a known VAP pathogen. However, recent gut microbiome studies show that pathogens rarely function alone. Hence, we determined the ETT microbial consortium co-colonizing with S. epidermidis or P. aeruginosa to understand its importance in the development of VAP and for patient prognosis. Using bacterial 16S rRNA and fungal ITS-II sequencing on ETT biomass showing presence of P. aeruginosa and/or S. epidermidis on culture, we found that presence of P. aeruginosa correlated inversely with patient survival and with bacterial species diversity. A decision tree, using 16S rRNA and patient parameters, to predict patient survival was generated. Patients with a relative abundance of Pseudomonadaceae <4.6% and of Staphylococcaceae <70.8% had the highest chance of survival. When Pseudomonadaceae were >4.6%, age of patient <66.5 years was the most important predictor of patient survival. These data indicate that the composition of the ETT microbiome correlates with patient prognosis, and presence of P. aeruginosa is an important predictor of patient outcome.
Similar content being viewed by others
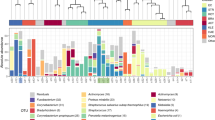
Introduction
Assisted ventilation is performed through an endotracheal tube (ETT), that is readily colonized by bacteria within 24 hours after intubation1,2. These microorganisms, migrating along the ETT cuff and inside the lumen of the ETT, form a so called biofilm, together with a network of secretions. Biofilm formation is indeed facilitated by the presence of nutrient-rich patient material which accumulates in the lower part of the ETT3.
Ventilator associated pneumonia (VAP) occurs in approximately 9–27% of all mechanical ventilated patients after at least 48 h of ventilation and is associated with high mortality and morbidity4,5. The exact mechanisms leading to the development of VAP are not understood, although a strong link exists with the microbial consortium on the ETT1,6,7. Endotracheal secretions often mirror the microbial consortium present in the ETT, at least at the resolution achieved by culture methods8,9. For this reason it is believed that micro-aspiration of detached pieces of biofilm containing harmful organisms might cause infection in the lower respiratory tract, and are believed to significantly contribute to the occurrence of (VAP)1. Regular screening of endotracheal secretions facilitates early diagnosis of the microorganisms linked to VAP and has been shown to impact on patient treatment and survival10.
Pseudomonas aeruginosa is one of the most common causes of VAP followed by Gram positive organisms like Staphylococcus aureus5. In some cases, however, no clear pathogen can be detected by conventional culture methods11, while in other cases a significant growth of oro-pharyngeal or cutaneous commensals including Staphylococcus epidermidis can be observed, suggesting that these organisms are not as harmless as frequently believed12. Recently, the presence of oro-pharyngeal microorganisms was demonstrated on the ETTs of mechanically ventilated patients6 and in respiratory samples11, however, the presence of any of these organisms on the ETT did not always lead to VAP with these bacteria7,12, indicating a contribution from host factors and the general health status of the patient. In fact, an important role for the entire bacterial consortium on the ETT in the development of VAP has been suggested6 and involves interspecies interactions that provide nutrient sources or produce growth inhibiting molecules13,14. While the presence of specific bacteria leading to dysbiosis has been shown for diseases like periodontitis and cystic fibrosis15,16, there is a lack of knowledge of such relationships within ETT biofilms and their potential role in the development of VAP.
Next generation sequencing technology is a powerful molecular tool to detect a wide variety of species, including the unculturable fraction.
By screening a large collection of ETTs obtained from mechanically ventilated patients at the Antwerp University Hospital over a three-year period, we selected 39 ETTs that showed positive culture results for P. aeruginosa, a major VAP pathogen, and S. epidermidis, the most frequently cultured bacterium in this study. We aimed (i) to study organism and species diversity in ETT biofilms using a culture independent metagenomic approach by bacterial 16S rRNA and fungal internal transcribed spacer (ITS) amplicon sequencing, (ii) to determine the core microbiomes on the ETTs which were culture positive for the key organisms P. aeruginosa and S. epidermidis and (iii) to link these findings with patient parameters in an attempt to identify markers of disease or of patient outcome.
Results
Abundance of ETT biomass does not correlate with VAP aetiology or patient prognosis
To study if the ETT biomass quantity or composition correlate to the development of VAP or to patient prognosis, we prospectively collected 203 ETTs from mechanically ventilated patients admitted to the intensive care unit (ICU) during a 3-year period, from April 2011 until December 2013, at the University hospital Antwerp, Belgium. Of these 203 patients, 44 progressed to pneumonia (VAP). Previous studies have shown that P. aeruginosa is a common VAP pathogen associated with high mortality and morbidity5 whereas S. epidermidis is the most frequently isolated bacterium in this study (51/203 ETT) that under specific circumstances can become a nosocomial pathogen12. P. aeruginosa was cultured from 36/203 ETT and was the second most common bacterium (Fig. 1A). To further study co-carriage with P. aeruginosa or S. epidermidis, ETTs from 39 patients showing presence of P. aeruginosa (n = 13), S. epidermidis (n = 21), or both (n = 5) on culture were selected for further analysis. Fifteen of these 39 patients progressed to pneumonia (VAP). In the ETTs showing presence of P. aeruginosa, this organism was also identified as the aetiology of the 4 VAP cases in broncho-alveolar lavage (BAL) cultures of these patients. However, in ETTs showing presence of S. epidermidis, other organism(s) were identified as the aetiological agent of VAP (9/11) or as a non-aureus Staphylococcus (1/11) or remained unidentified (1/11). Summary of the data from all 39 patients is shown in Table 1 and details on patients progressing to VAP in Supplementary Table S1.
Biomass was visually scored under a 5x microscope as present or absent and validated by histology (Fig. 2). H&E staining revealed a typical laminar patterning in the ETT biomass associated with small clusters of bacteria or yeast hyphae. We also found many lacunae in the structures that harboured human cells. Similar structures have been previously observed by Inglis et al.17 who performed light and confocal scanning laser microscopy on ETT biofilms. Light and fluorescence microscopy allow the preservation of the ETT biofilm structures, in contrast to scanning electron microscopy used in most studies2,9,18 were the original structure is lost.
Histology of typical ETT biofilms.
H&E staining revealed the presence of candida hyphae, white arrows (a); bacterial clusters, white arrows (b); and a clearly layered structure, black arrows (c); lacunae that harbored human cells are shown in the Gram-stained slices (d and e, black arrows); PAS stain also showed the layered structure (black arrows) and many cavities (f).
In our study, the presence of visible biomass in the ETT lumen did not correlate with development of VAP (P = 0.104) or patient survival (P = 0.100), indicating that the amount of ETT biofilm is not a major effector of VAP or patient prognosis. During mechanical ventilation, endotracheal secretions are removed on a regular basis with a suction catheter to prevent biofilm formation and VAP19. Furthermore, the ETT biofilm mainly consists of patient material in addition to bacteria and the exact amount of secretions produced is patient dependent17. These findings suggest that the presence of biomass as such is not necessarily bad for the patient prognosis and composition might play a role as well.
In addition, the APACHE II scores also did not significantly differ among the patients that developed VAP or not (P = 0.943) or survived or not (P = 0.196), indicating that the physiological condition was the same at the time of intubation. This result is confirmed by k-means clustering and logistic regression analysis which showed no correlation between patient survival and the reason of admission to the ICU. These data raised the question what other effectors, like the microbial consortium on the ETT or other patient related parameters are likely to play a role in VAP aetiology and patient prognosis.
A two-step cluster analysis reveals three natural clusters with patient survival and development of VAP as the two main cluster predictors
To investigate patient-related parameters, the 39 patients were subdivided by a two-step cluster analysis, which facilitates the discovery of natural clusters of individuals with similar characteristics within a larger population20. The 39 patients were divided into three clusters with patient survival upon extubation (predictor importance 1) and development of VAP (predictor importance 0.55) being the most important cluster predictors (Table 2). All patients in clusters 2 and 3 (n = 16 and 12, respectively) survived whereas all in cluster 1 (n = 11) died. Details of the patients are shown in Supplementary Table S1. Clusters 2 and 3 are differentiated by the development of VAP; 13 of the 16 patients (81.2%) in cluster 2 and none of the 12 in cluster 3 progressed to VAP. On the other hand, only 2 patients from cluster 1 (18.2%), wherein 100% mortality was observed, developed VAP. Remarkably, all patients in cluster 2, who showed the highest incidence of VAP, survived. Because the development of VAP is usually known to be associated with high mortality5, a combination of other factors might determine survival in this subset of patients. The third most important predictor of clustering was the presence of a visible biomass on the ETT upon extubation (predictor importance 0.25). All ETTs of cluster 3 and 9/11 (81.8%) ETTs of cluster 1 showed a visible biomass, while in only half of the ETTs of cluster 2 a biomass was visible.
As a fourth predictor, P. aeruginosa was more frequently isolated from ETTs in cluster 1 (7/11), while S. epidermidis was more frequent in cluster 2 (9/16 alone and 5/16 with P. aeruginosa) and cluster 3 (8/12) (predictor importance 0.175). Average APACHE II scores (predictor importance 0.170) were similar for cluster 1 (26.55) and cluster 2 (25.5) and lower for cluster 3 (18.50), where all patients survived and none developed VAP. A least significant difference (LSD) post-hoc analysis showed that survivors in cluster 2 were on average 10 years younger than the patients who died in cluster 1 (P = 0.043), while there was no significant difference in age compared to cluster 3.
The microbiome present on the ETTs correlates with patient prognosis
Next, we questioned whether the microbial composition of the ETT biomass might correlate to patient prognosis. Therefore, 16S rRNA analysis was performed to identify the ETT microbial consortium co-existing with P. aeruginosa or/and S. epidermidis and subjected these data together with the patient parameters to a multivariate analysis.
First, we generated a decision tree that identified a low relative abundance of family Pseudomonadaceae as the key predictor of patient survival (Fig. 3). A relative abundance of Pseudomonadaceae below 4.6% and of Staphylococcaceae below 70.8% correlated with a high chance of patient survival. When the relative abundance of Pseudomonadaceae was above 4.6%, patient age below 66.5 years also correlated with a higher chance of survival, confirming the importance of the organisms present on the ETTs and age of the patient (Fig. 3).
Second, we investigated whether other members of the ETT microbiome could be associated with patient survival using the online binomial tool LefSe dedicated for biomarker discovery21. This tool considers frequency as well as relative microbial abundance and performs a linear discriminant analysis (LDA) to calculate the effect size of each parameter21. An LDA score above 2 was considered significant. LefSe analysis showed that ETTs of patients who survived (n = 28) were more likely to harbour the phylum Actinobacteria (Fig. 4a, n = 11, LDA score of 4.8). Within this phylum, Actinomyces and Corynebacterium were more frequently identified in the ETTs of the surviving patients (18/28 and 15/28, respectively) compared to the patients that did not survive (1/11 and 4/11, respectively; LDA score >3.6). In addition, Bifidobacterium adolescentis was identified in 9/28 ETTs of the survivors while it was completely absent in the ETTs of the patients that died (LDA score >2.4). Furthermore, the ETTs of patients that died showed a close association with the genus Pseudomonas, including P. aeruginosa (8/11), P. fluorescens (3/11) and uncultured Pseudomonas spp. (6/11). Also noteworthy was the presence of Morganella morganii (4/11), Burkholderia cepacia (2/11), Proteus mirabilis (2/11), Marinomonas sp. MED121 (2/11), and Xylella fastidosa (2/11) on the ETT of the patients that died. While these were found in only a fraction of the ETTs from the non-surviving patients, their frequency and abundance was significant in this group compared to the ETTs of the survivors (Morganella morganii 2/28, others absent, LDA score >2).
Cladogram showing the association of distinct ETT microbial components (OTUs) with patient survival (a) or with the 3 groups of ETTs defined by culture (b). The inner circles represent the highest taxonomic level and the coloured bands contain the taxonomic levels which are significantly more associated with one group of patients (LDA score > 2). The cladogram was generated using the LefSe galaxy tool. A. Red: patients that did not survive upon extubation; Green: patients that survived upon extubation. B. Red: ETT with positive culture for P. aeruginosa; blue: ETT with positive culture for S. epidermidis; green: ETT with positive culture for both P. aeruginosa and S. epidermidis.
Distinct predominant microbiomes are associated with the presence of P. aeruginosa and S. epidermidis
After we identified significant associations between microbial composition and patient prognosis, we also questioned whether a microbial fingerprint might be linked to development of VAP. As the aetiology of VAP differed between the three groups of ETTs identified by culture (Supplementary Table S1), we aimed to identify the differential microbiomes of these three groups in correlation to VAP as well as a common ETT core microbiome.
Firstly, classification of all 16S sequences by alignment to the SILVA reference database showed a total of 8 different phyla that were associated with the ETTs analysed in this study. Within these phyla, 69 different families harbouring 354 different species were identified. The majority (61%) of the sequences belonged to three phyla: Proteobacteria (34%), Firmicutes (18%) and Actinobacteria (9%) as shown in Fig. 5a. Of note, 39% of the sequences remained unclassified when compared to existing reference sequences in the Greengenes, SILVA and RDP databases. An additional BLAST against the NCBI nr database revealed that the majority (89%) of these were of the Proteobacteria family.
Distribution of the relative abundance of the major phyla.
The phyla Proteobacteria and Firmicutes were the most abundant. BLAST analysis revealed that approximately 90% of the unclassified sequences also belonged to Proteobacteria (a). OTUs defined on the family level found to be associated with the 3 groups of ETTs. Families present in at least 50% of the ETTs in a certain group were assigned to the microbiome of that group. (b). Distribution of OTUs, defined at the phylum level, within the 3 groups of ETTs (c). Species diversity in the three groups of ETTs. Black squares: average number of species (d).
Secondly, we aimed to identify which families were present on the majority of ETTs across the three groups regardless of their relative abundance. Enterobacteriaceae and Phyllobacteriaceae could be identified on the majority of the ETTs (77% and 86%, respectively) (Fig. 5c). However, at the level of the genus and species, the Enterobacteriaceae family showed a large diversity. The genera Escherichia and Klebsiella were the most and second-most commonly identified with E. coli, E. albertii and K. pneumoniae as the main representatives. However, none of these species were present in more than 50% of all ETTs. In contrast to the high variability of the Enterobacteriaceae, the Phyllobacteriaceae had only one representative, Phyllobacterium myrsinacearum, which was present at low abundance in 86% of all ETTs. More details on the species distribution are given in Supplementary Table S2.
Thirdly, we identified the phylum distribution and species diversity in the three groups of ETTs (Fig. 5b). In the ETTs of the P. aeruginosa group, the phylum Proteobacteria was most abundant (98%), while Firmicutes and Actinobacteria (1% each) were present in a minority. In contrast, ETTs belonging to the S. epidermidis group showed a more even distribution over the three phyla: Proteobacteria (56%), Firmicutes (30%) and Actinobacteria (12%). In the third group, the majority of sequences were also assigned to the Proteobacteria (72%), followed by Actinobacteria (19%) and Firmicutes (9%). At lower taxonomic levels, ETTs belonging to the S. epidermidis group showed the maximum species diversity (290 species in total) and the highest average number of species per tube (35 species, 3357 reads). This was in contrast to the ETTs of the P. aeruginosa group that harboured on average 20 different species (3791 reads) per tube and in total 116 different species (P = 0.029) (Supplementary Table S3).
Lastly, we used the LefSe tool to discover distinct microbial signatures associated with each of the three ETT groups (Fig. 4b). In the P. aeruginosa group, only bacteria belonging to the family Pseudomonadaceae were identified and these included various Pseudomonas spp. These data provide in vivo evidence for previous laboratory based studies describing the production of antibacterial factors and inhibition of competitive colonizers (non-pseudomonads) both on co-cultures and in a biofilm phenotype by P. aeruginosa22,23,24,25,26,27. In the S. epidermidis group, Corynebacteriaceae were identified in 17/21 (80%) of the ETTs (Fig. 5c). Since the VAP aetiology was variable in this group, differences in the ETT microbial signatures of patients developing VAP or not were sought. Interestingly, presence of Klebsiella pneumoniae (7/9 VAP, 2/12 non-VAP) and Serratia marcescens (8/9 VAP, absent in non-VAP) among the S. epidermidis group that developed VAP was highly significant (LDA > 4). Finally, in the group of ETTs containing both P. aeruginosa and S. epidermidis (n = 5), Lactobacillaceae (4 ETTs) and Coriobacteriaceae (3 ETTs) were more prevalent (Figs 4b and 5c). The family of the Lactobacillaceae showed mainly Lactobacillus but sequences could not be identified to the species level. Atopobium parvulum was the main representative of the Coriobacteriaceae. We can conclude that the microbial composition of the ETTs consists of two parts: a common (up to family level) microbiome shared by all ETTs and a clearly distinct microbiome at least for the ETTs harbouring P. aeruginosa or S. epidermidis. Importantly, our finding that presence of P. aeruginosa (and lower species diversity) correlates strongly with worse patient outcome underlines the importance of detecting its presence as a marker of patient prognosis.
Candida spp. are the most common fungi in the ETT microbiome
During culture of the ETTs, Candida albicans and Candida glabrata were the most and third most common organisms isolated, with an incidence of 81/203 and 37/203 ETTs, respectively (Fig. 1A,B). In the selection of ETTs used for this study, Candida spp. were present in 17/39 tubes, of which 11 also showed the presence of S. epidermidis, 4 of P. aeruginosa and 2 of both. ITS sequencing identified 55 different fungal species, of which the majority (70%) belonged to the phylum Ascomycota and a smaller proportion (27%) to Basidiomycota. All 17 samples harboured at least one and in most cases two of the following Candida species: C. albicans (11 ETTs), C. glabrata (13 ETTs) or C. tropicalis (14 ETTs). Additional Candida species identified include C. catenulata (8 ETTs), C. dubliniensis (2 ETTs) and C. parapsilosis (1 ETT) (Supplementary Table S3).
Furthermore, LefSe analysis assigned the family Onygenaceae to the group of ETTs from VAP patients with an LDA score of 2.5, however, this association was only present at order and family level, probably due to the low number of VAP patients in this subgroup (4 ETTs). Of note, relative abundance of strict and facultative anaerobes was significantly higher in the ETTs harbouring Candida spp. compared to the ETTs without Candida (54% versus 43% relative abundance, respectively; strict anaerobes 13% versus 5%; facultative anaerobes 41% versus 38%, respectively) (Z-test, P < 0.001). These differences between the two groups were primarily due to relative proportions rather than species composition except for the genus Prevotella, which was found to be significantly associated with presence of Candida by LefSe analysis (LDA < 2). Prior studies have shown that fungi are important for maintaining oral microbiome homeostasis but their role in the ETT biofilm remains unclear28,29. Remarkably, in our samples, Candida spp. were more frequent in the ETTs harbouring S. epidermidis and were only rarely co-isolated with P. aeruginosa (Fig. 1B). These results support earlier findings that P. aeruginosa and C. albicans share an antagonistic relationship30 whereas Staphylococci and C. albicans are thriving well together31.
Discussion
This study, to our knowledge, is the largest to date analysing the ETT biofilm in ventilated patients. Instead of a random molecular profiling of the bacterial community in the ETT, we opted to study the accessory microbiome co-colonizing with P. aeruginosa or S. epidermidis, the former is one of the most common causes of VAP and the latter one of the most common commensals with a known pathogenic potential. Both are the most common bacteria cultured from the ETTs in this study. Our aim was to decipher consistent associations between presence of P. aeruginosa or S. epidermidis and other organisms in the ETT biofilm in correlation with multiple patient parameters. Finally, this study provides a proof-of-concept of utilization of 16S microbiome data and patient parameters to generate a prognostic algorithm to predict patient survival.
We found that P. aeruginosa was consistently present in patients that did not survive upon extubation, suggesting that it might be an indicator of a worse patient outcome. Multivariate analysis of 16S data and patient parameters showed that relative abundance of Pseudomonadaceae was the most important predictor of patient survival followed by age and relative abundance of Staphylococcaceae. Our identified best-case scenario for patient survival was an abundance of Pseudomonadaceae below 4.6% and of Staphylococcaceae below 70.8%. When the relative abundance of the Pseudomonadaceae was above 4.6%, a patient age <66.5 years became the most important predictor of patient survival.
Although this algorithm is based on ETT microbiome data and represents a later stage of microbial colonization, previous research has shown consistent correlations between organisms isolated from ETTs and earlier stage respiratory samples like endotracheal aspirates and BALs8,18. In the present study, for patients who developed VAP due to P. aeruginosa (BAL cultures and clinical diagnosis), we were also able to identify the pathogen in the ETT of all patients using cultures and 16S analysis. Therefore this algorithm would be a useful addition to current ICU protocols in hospitals performing routine endotracheal aspirate sentinel cultures as well as for clinical trials pre-enriching for patients colonized with Pseudomonas aeruginosa and likely to develop VAP.
In addition to P. aeruginosa, the microbiome of patients who did not survive harboured other potentially pathogenic bacterial genera such as Morganella, Proteus, Burkholderia etc (Fig. 4a). LefSe analysis showed that not all genera were consistently present in all patients who died, however, there was a clear difference with the microbiome of surviving patients. The ETT microbiome of the latter group of patients tended to harbour the phylum Actinobacteria, which include Bifidobacteria, commonly used as probiotics, and non-diptheriae Corynebacteriaceae. Interestingly, a recent study analysing the benefits of probiotic Bifidobacterium longum administration in patients undergoing surgery, showed that an increase in relative abundance of Actinobacteria in the gut correlated inversely with blood inflammatory parameters32. These data support our findings that surviving patients tend to harbour a ‘healthy’ microbiome. Whether microbial shifts or dysbiosis in the endotracheal microbiome are the cause or the consequence of a worse patient outcome remains to be investigated and would require sequential sampling of the endotracheal microbiota in order to obtain better insight on the community dynamics.
Loss of microbial diversity has been previously identified as a consistent marker of a diseased microbiome33,34. In our study, while the incidence of VAP was indeed lower in the S. epidermidis colonized group, the patients who did develop VAP in this group did not show any loss of microbial diversity compared to others in this group (p = 0.464). In fact, we found a significant loss of microbial diversity to be associated with P. aeruginosa colonization. This competitive exclusion is a hallmark of P. aeruginosa colonization and has also been previously observed in cystic fibrosis26. These data are also supported by in vitro studies demonstrating the predominant nature of P. aeruginosa and its inhibition of other potential pathogens or colonizers13,22,25,26. Of note, unlike the P. aeruginosa colonized group, the high microbial diversity in the S. epidermidis colonized group was also reflected in the concurrent difficulty in identifying a clear VAP pathogen in BAL cultures. While the possibility exists that the causative pathogen is a nonculturable (in the culture conditions utilized) organism, VAP might also be caused by a microbial consortium without a predominant pathogen14. Finally, lack of a clear VAP pathogen in BAL might also be due to the fact that microbiology laboratories conventionally report only the predominant organism (in most cases a Staphylococcus in the S. epidermidis group), while a low abundance pathogen might be missed on culture. In the S. epidermidis group, we found a consistent co-presence of K. pneumoniae and S. marcescens, present in relatively low numbers (median: 10.5 and 5 reads respectively) in patients who developed VAP whereas both were absent in the patients that did not develop VAP. Of note, ETT cultures did not grow K. pneumoniae and S. marcescens and their presence was only detected upon 16S sequencing. Since the ETT reflects the end-stage microbiome, the potential role of these correlations remain to be validated in vitro and in earlier stage patient samples.
Finally, Candida spp., which are known nosocomial pathogens, were more often isolated from ETTs of the S. epidermidis group (13/17). These results corroborate prior studies demonstrating the antifungal activity of P. aeruginosa31,35,36, and the frequent co-isolation of S. epidermidis and C. albicans in oral polymicrobial infections13,31,37. Fungi are also part of the healthy oral microbiota, supporting the growth of oral anaerobes and increasing the resilience of the consortium28,38. Indeed, we also found a remarkably higher relative abundance, but not differing species, of anaerobes in ETTs harbouring Candida versus those that did not.
In conclusion, this study represents one of the first instances/attempts of utilization of the patient microbiome and clinical parameters to develop a prediction model of patient prognosis. The next steps would involve analysis of sequential respiratory samples in a larger cohort of intubated patients to understand the endotracheal biofilm dynamics and to develop models based on presence or relative abundances of early microbial markers that are predictive of patient prognosis and of the risk of developing VAP.
Materials and Methods
Study design and sample collection
ETTs from 203 mechanically ventilated patients admitted to the intensive care unit (ICU) were collected prospectively during 2011–2013 at the Antwerp University Hospital in accordance with relevant regulations and guidelines. Inclusion criteria were minimum 48 hours of ventilation, non-pregnant and age above 18 years. This study was approved by the ethical commission of the Antwerp University Hospital (12/12/112) and informed consent to use these data for research purpose was obtained. The lower 15 cm of the collected ETTs was processed immediately. ETTs were divided into 5 parts, A–E (Supplementary Figure S1). Parts A and E were cultured by gently tapping the ETT pieces on Colombia blood agar and Sabouraud’s dextrose agar. Visually distinct colonies were identified using MALDI-TOF (Bruker Daltonics, Bremen, Germany). Thirty-nine ETTs that yielded P. aeruginosa (n = 13) or S. epidermidis (n = 21) or both (n = 5) were studied further. From each of the 39 ETTs, parts below the cuff (D, E and E1) were utilized for sequencing (D and E) and for microscopy (E1) (Supplementary Figure S1).
Microscopic analysis
Part E1 of ETT was fixed in 4% paraformaldehyde for 24 h. When a biomass was visible on the internal lumen of the fixed E1 slices, the mass was removed by scalpel and prepared for standard paraffin embedding as done previously39. Histology was performed on 5 μm thick sections stained with Gram, haematoxylin and eosin (H&E), and Periodic acid Schiff (PAS) stains. All light microscopy images were captured on a Zeiss lab.A1 (Heidelberg, Germany) microscope equipped with a UC30 colour camera (Olympus, Antwerp, Belgium).
DNA extraction and multiplexed pyrosequencing
Adherent material on parts D and E of the ETT was removed by sonication and vigorous vortexing (5 min. each). Samples were centrifuged (5 min. at 17949× g, 5430 R centrifuge, Eppendorf, Rotselaar, Belgium) and reduced to a volume of approximately 0.5 ml. DNA was extracted using the Masterpure complete DNA and RNA purification kit (Epicentre, Leusden, The Netherlands) as recommended with the following modification: 1 μl Ready-lyse lysozyme solution (Epicentre, Leusden, The Netherlands) was added to 150 μl sample followed by incubation at 37 °C for 30 minutes prior to the extraction. Primers V345_341F (CCT ACG GGR SGC AGC AG) and V345_909R (TTT CAG YCT TGC GRC CGT AC) were used for 16S amplification of the V3–V5 region and primers (ITS-F3): GCATCGATGAAGAACGCAGC and (ITS-R4): TCCTCCGCTTATTGATATGC for amplifying the fungal ITS-II region using multiplexed Roche 454 pyrosequencing40,41,42. Samples were purified by bead-beating prior to amplification. Purified and quantified PCR products were pooled in equimolar amounts and uni-directional sequencing on ½ run was performed on 454 GS FLX Sequencer (Roche, Basel, Switzerland) using Titanium FLX reagents, resulting in 5000 reads with a 400–600 bp read length on average per sample (Microsynth, Balgach, Switzerland). Sequence analysis was performed using the online MetaGenome Rapid Annotation using Subsystem Technology (MG-RAST)43.
Sequence analysis
Sequences were sorted based on their multiplex identifier tag and the template-specific primers were trimmed. Sequences shorter than 200 bp were eliminated, and others were aligned against the SILVA database (release 115) using the mothur tool44,45. A second-round quality filtering eliminated non-aligned reads with <95% alignment and chimera sequences were removed using the UCHIME46 tool implemented in mothur. The taxonomic classification was performed using mothur based on a Bayesian method47 with K-mer size of 8bp and 1000 replicates. An additional parallel analysis was performed using the online analysis server MetaGenome Rapid Annotation using Subsystem Technology (MG-RAST)43. After pre-processing of the raw reads with default parameters, the 16S sequences were processed similar as described above. Processed reads were searched against the SILVA database using BLAT48 for rRNA identification with 97% identity to cluster the sequences, where after the longest sequence of each cluster was used as a representative. BLAT similarity search with default parameters was performed against the M5rna database with the max e-value cut-off set to 1 × 105, the min% identity cut-off to 97% and a minimum alignment length cut-off of 15.
Analysis of microbial composition of the ETT biofilms
ETTs were grouped into those showing presence of P. aeruginosa, S. epidermidis or both organisms by culture and their microbial composition was analysed to the family level. Presence/absence of a family in each of the three groups was determined and assigned to the microbiome of a certain group with at a cut-off value of present in 50% of the ETT from a certain group. Differences in relative abundance on all taxonomic levels between the three ETT groups were identified by the binomial disclaim tool linear discriminant analysis effect size (LefSe)21. First, LefSe determines features (organisms, clades, operational taxonomic units, genes, or functions) most likely to explain differences between groups by coupling standard tests for statistical significance with additional tests encoding biological consistency and effect relevance. Second, linear discriminant analysis (LDA) is used to rank the features differing between the groups based on their effect sizes21. All taxonomic levels showing an LDA score above 2 were considered significant. Statistical significance was set at 0.05 and the strategy for multi-class analysis was set all against one.
Definitions
VAP was identified according to the classical definition as a bacterial pneumonia present in patients with mechanical ventilation for at least 48 hours combined with a new infiltrate on the chest X-ray, signs of infection and detection of a bacterial causative agent4,49. The severity of illness in the ICU was scored according to the validated Apache II score39.
Statistical analyses
Statistical analyses were performed in IBM SPSS statistics v2.0. Normality was tested using the Shapiro-Wilk test for normality. One-way ANOVA was used for normally distributed data and the Mann-Whitney U test for not normally distributed data. Two-step cluster analysis was performed to discover natural clusters in the dataset using the Schwarz’s Bayesian Criterion (BIC) and outlier treatment was applied using default parameters. Statistical differences between each variable in the clusters were determined using one-way ANOVA for continuous data and χ2 for categorical data in a post-hoc least significant difference (LSD) analysis. Significance level was 0.05 in all statistical analyses. Logistic regression and K-means clustering of the 16S and patient data were performed in Matlab R2015a (MathWorks, Eindhoven, The Netherlands) and R platform v0.99.47 (RStudio, Boston, USA), respectively. Results of the two-step cluster analysis were confirmed by the k-means clustering algorithm on the R platform.
Additional Information
Accession codes: All sequencing data generated for this project were deposited to European Nucleotide Archive (ENA) under study accession number PRJEB16308.
How to cite this article: Hotterbeekx, A. et al. The endotracheal tube microbiome associated with Pseudomonas aeruginosa or Staphylococcus epidermidis. Sci. Rep. 6, 36507; doi: 10.1038/srep36507 (2016).
Publisher’s note: Springer Nature remains neutral with regard to jurisdictional claims in published maps and institutional affiliations.
References
Pneumatikos, I. A., Dragoumanis, C. K. & Bouros, D. E. Ventilator-associated pneumonia or endotracheal tube-associated pneumonia? An approach to the pathogenesis and preventive strategies emphasizing the importance of endotracheal tube. Anesthesiology 110, 673–680, doi: 10.1097/ALN.0b013e31819868e0 (2009).
Perkins, S. D., Woeltje, K. F. & Angenent, L. T. Endotracheal tube biofilm inoculation of oral flora and subsequent colonization of opportunistic pathogens. Int J Med Microbiol 300, 503–511, doi: 10.1016/j.ijmm.2010.02.005 (2010).
Raghavendran, K., Mylotte, J. M. & Scannapieco, F. A. Nursing home-associated pneumonia, hospital-acquired pneumonia and ventilator-associated pneumonia: the contribution of dental biofilms and periodontal inflammation. Periodontology 2000 44, 164–177, doi: 10.1111/j.1600-0757.2006.00206.x (2007).
American Thoracic, S. & Infectious Diseases Society of, A. Guidelines for the management of adults with hospital-acquired, ventilator-associated, and healthcare-associated pneumonia. Am J Respir Crit Care Med 171, 388–416, doi: 10.1164/rccm.200405-644ST (2005).
Chastre, J. & Fagon, J. Y. Ventilator-associated pneumonia. Am.J.Respir.Crit Care Med. 165, 867–903, doi: 10.1164/ajrccm.165.7.2105078 (2002).
Cairns, S. et al. Molecular analysis of microbial communities in endotracheal tube biofilms. PLoS.One. 6, e14759, doi: 10.1371/journal.pone.0014759 (2011).
Safdar, N., Crnich, C. J. & Maki, D. G. The pathogenesis of ventilator-associated pneumonia: its relevance to developing effective strategies for prevention. Respiratory Care 50, 725–739 (2005).
Adair, C. G. et al. Implications of endotracheal tube biofilm for ventilator-associated pneumonia. Intensive Care Med 25, 1072–1076, doi: 90251072.134 (1999).
Feldman, C. et al. The presence and sequence of endotracheal tube colonization in patients undergoing mechanical ventilation. Eur Respir J 13, 546–551 (1999).
Wiener-Kronish, J. P. & Dorr, H. I. Ventilator-associated pneumonia: problems with diagnosis and therapy. Best Pract Res Clin Anaesthesiol 22, 437–449 (2008).
Huebinger, R. M. et al. Examination with next-generation sequencing technology of the bacterial microbiota in bronchoalveolar lavage samples after traumatic injury. Surg. Infect. (Larchmt.) 14, 275–282, doi: 10.1089/sur.2012.095 (2013).
Lambotte, O. et al. The significance of distal bronchial samples with commensals in ventilator-associated pneumonia - Colonizer or pathogen? Chest 122, 1389–1399, doi: 10.1378/chest.122.4.1389 (2002).
Bousbia, S., Raoult, D. & La, S. B. Pneumonia pathogen detection and microbial interactions in polymicrobial episodes. Future.Microbiol. 8, 633–660, doi: 10.2217/fmb.13.26 (2013).
Bousbia, S. et al. Repertoire of intensive care unit pneumonia microbiota. PLoS One 7, e32486, doi: 10.1371/journal.pone.0032486 (2012).
Lynch, S. V. & Bruce, K. D. The cystic fibrosis airway microbiome. Cold Spring Harb.Perspect.Med . 3, a009738, doi: 10.1101/cshperspect.a009738 (2013).
Hajishengallis, G., Darveau, R. P. & Curtis, M. A. The keystone-pathogen hypothesis. Nat Rev Microbiol 10, 717–725, doi: 10.1038/nrmicro2873 (2012).
Inglis, T. J., Lim, T. M., Ng, M. L., Tang, E. K. & Hui, K. P. Structural features of tracheal tube biofilm formed during prolonged mechanical ventilation. Chest 108, 1049–1052 (1995).
Gil-Perotin, S. et al. Implications of endotracheal tube biofilm in ventilator-associated pneumonia response: a state of concept. Crit Care 16, R93, doi: 10.1186/cc11357 (2012).
Damas, P. et al. Prevention of ventilator-associated pneumonia and ventilator-associated conditions: a randomized controlled trial with subglottic secretion suctioning. Crit Care Med 43, 22–30, doi: 10.1097/CCM.0000000000000674 (2015).
Mooi, E. & Sarstedt, M. A Concise Guide to Market Research . 237–284 (Springer Berlin Heidelberg, 2011).
Segata, N. et al. Metagenomic biomarker discovery and explanation. Genome Biol 12, R60, doi: 10.1186/gb-2011-12-6-r60 (2011).
Qin, Z., Yang, L., Qu, D., Molin, S. & Tolker-Nielsen, T. Pseudomonas aeruginosa extracellular products inhibit staphylococcal growth, and disrupt established biofilms produced by Staphylococcus epidermidis. Microbiology 155, 2148–2156, doi: 10.1099/mic.0.028001-0 (2009).
Haba, E. et al. Physicochemical characterization and antimicrobial properties of rhamnolipids produced by Pseudomonas aeruginosa. Biotechnology and bioengineering 81, 316–322, doi: 10.1002/bit.10474 (2003).
Kessler, E., Safrin, M., Olson, J. C. & Ohman, D. E. Secreted LasA of Pseudomonas aeruginosa is a staphylolytic protease. The Journal of biological chemistry 268, 7503–7508 (1993).
Machan, Z. A. et al. Interaction between Pseudomonas aeruginosa and Staphylococcus aureus: description of an anti-staphylococcal substance. Journal of medical microbiology 34, 213–217, doi: 10.1099/00222615-34-4-213 (1991).
Costello, A., Reen, F. J., O’Gara, F., Callaghan, M. & McClean, S. Inhibition of co-colonizing cystic fibrosis-associated pathogens by Pseudomonas aeruginosa and Burkholderia multivorans. Microbiology 160, 1474–1487, doi: 10.1099/mic.0.074203-0 (2014).
Voggu, L. et al. Microevolution of cytochrome bd oxidase in Staphylococci and its implication in resistance to respiratory toxins released by Pseudomonas. Journal of bacteriology 188, 8079–8086, doi: 10.1128/JB.00858-06 (2006).
Krom, B. P., Kidwai, S. & Ten Cate, J. M. Candida and other fungal species: forgotten players of healthy oral microbiota. J Dent Res 93, 445–451, doi: 10.1177/0022034514521814 (2014).
Zaura, E., Nicu, E. A., Krom, B. P. & Keijser, B. J. Acquiring and maintaining a normal oral microbiome: current perspective. Front Cell Infect Microbiol 4, 85, doi: 10.3389/fcimb.2014.00085 (2014).
Gibson, J., Sood, A. & Hogan, D. A. Pseudomonas aeruginosa-Candida albicans interactions: localization and fungal toxicity of a phenazine derivative. Appl Environ Microbiol 75, 504–513, doi: 10.1128/AEM.01037-08 (2009).
Shirtliff, M. E., Peters, B. M. & Jabra-Rizk, M. A. Cross-kingdom interactions: Candida albicans and bacteria. FEMS microbiology letters 299, 1–8, doi: 10.1111/j.1574-6968.2009.01668.x (2009).
Mizuta, M. et al. Perioperative supplementation with bifidobacteria improves postoperative nutritional recovery, inflammatory response, and fecal microbiota in patients undergoing colorectal surgery: a prospective, randomized clinical trial. Biosci Microbiota Food Health 35, 77–87, doi: 10.12938/bmfh.2015-017 (2016).
Shankar, J. et al. Looking beyond respiratory cultures: Microbiome-cytokine signatures of bacterial pneumonia and tracheobronchitis in lung transplant recipients. Am J Transplant, doi: 10.1111/ajt.13676 (2015).
Flight, W. G. et al. Rapid Detection of Emerging Pathogens and Loss of Microbial Diversity Associated with Severe Lung Disease in Cystic Fibrosis. Journal of Clinical Microbiology 53, 2022–2029, doi: 10.1128/jcm.00432-15 (2015).
Mear, J. B. et al. Candida albicans and Pseudomonas aeruginosa interactions: more than an opportunistic criminal association? Medecine et maladies infectieuses 43, 146–151, doi: 10.1016/j.medmal.2013.02.005 (2013).
Morales, D. K. et al. Control of Candida albicans metabolism and biofilm formation by Pseudomonas aeruginosa phenazines. mBio 4, e00526-00512, doi: 10.1128/mBio.00526-12 (2013).
Pammi, M., Liang, R., Hicks, J., Mistretta, T. A. & Versalovic, J. Biofilm extracellular DNA enhances mixed species biofilms of Staphylococcus epidermidis and Candida albicans. BMC. Microbiol. 13, 257, doi: 10.1186/1471-2180-13-257 (2013).
Janus, M. M. et al. In vitro phenotypic differentiation towards commensal and pathogenic oral biofilms. Biofouling 31, 503–510, doi: 10.1080/08927014.2015.1067887 (2015).
Knaus, W. A., Draper, E. A., Wagner, D. P. & Zimmerman, J. E. APACHE II: a severity of disease classification system. Crit Care Med 13, 818–829 (1985).
Hansen, M. C., Tolker-Nielsen, T., Givskov, M. & Molin, S. Biased 16S rDNA PCR amplification caused by interference from DNA flanking the template region. Fems Microbiology Ecology 26, 141–149, doi: 10.1016/S0168-6496(98)00031-2 (1998).
Brunk, C. F. & Eis, N. Quantitative measure of small-subunit rRNA gene sequences of the kingdom korarchaeota. Appl Environ Microbiol 64, 5064–5066 (1998).
White, T., Burns, T., Lee, S. & Taylor, J. In PCR Protocols: A Guide to Methods and Applications (ed Academic Press Inc.) Ch. 38 (1990).
Meyer, F. et al. The metagenomics RAST server - a public resource for the automatic phylogenetic and functional analysis of metagenomes. BMC. Bioinformatics. 9, 386, doi: 10.1186/1471-2105-9-386 (2008).
Quast, C. et al. The SILVA ribosomal RNA gene database project: improved data processing and web-based tools. Nucleic Acids Res . 41, D590–D596, doi: 10.1093/nar/gks1219 (2013).
Schloss, P. D. et al. Introducing mothur: open-source, platform-independent, community-supported software for describing and comparing microbial communities. Appl. Environ. Microbiol. 75, 7537–7541, doi: 10.1128/AEM.01541-09 (2009).
Edgar, R. C., Haas, B. J., Clemente, J. C., Quince, C. & Knight, R. UCHIME improves sensitivity and speed of chimera detection. Bioinformatics 27, 2194–2200, doi: 10.1093/bioinformatics/btr381 (2011).
Wang, Q., Garrity, G. M., Tiedje, J. M. & Cole, J. R. Naive Bayesian classifier for rapid assignment of rRNA sequences into the new bacterial taxonomy. Appl Environ Microb 73, 5261–5267, doi: 10.1128/Aem.00062-07 (2007).
Kent, W. J. BLAT–the BLAST-like alignment tool. Genome Res . 12, 656–664, doi: 10.1101/gr.229202 (2002).
Jorens, P. G. Sticking to an Old Definition of Ventilator-Associated Pneumonia Is Not Old-Fashioned. Respir Care 61, 390–392, doi: 10.4187/respcare.04736 (2016).
Acknowledgements
We would like to thank the nursing staff of the Critical Care unit of the Antwerp University Hospital, and especially the research nurse Evi Van Den Broeck for rigorous sample and data collection and Arie Weeren for help with the multivariate analysis of the patient parameters. This work, AH, and PM (50%) were supported by funding from Research Foundation Flanders (FWO-F, G.0513.12) and University of Antwerp Research funds (BOF-GOA 41/FA020000/2/5139). BBX is supported by University of Antwerp Research Funds (BOF-DOCPRO 2012-27450). KB is supported by the Agency for Innovation by Science & technology IWT, grant #111664).
Author information
Authors and Affiliations
Contributions
S.M.K., S.K.S. and H.G. designed the study. A.H., P.M. and K.B. performed the experimental work. B.B.X. and A.H. performed bioinformatics analysis. P.G.J., T.S., M.I. and C.L. collected patient samples and clinical data. S.M.K., S.K.S., A.H., B.B.X. and K.B. analysed and interpreted the data. S.M.K., S.K.S. and A.H. wrote the manuscript. All authors read and revised the manuscript.
Ethics declarations
Competing interests
The authors declare no competing financial interests.
Electronic supplementary material
Rights and permissions
This work is licensed under a Creative Commons Attribution 4.0 International License. The images or other third party material in this article are included in the article’s Creative Commons license, unless indicated otherwise in the credit line; if the material is not included under the Creative Commons license, users will need to obtain permission from the license holder to reproduce the material. To view a copy of this license, visit http://creativecommons.org/licenses/by/4.0/
About this article
Cite this article
Hotterbeekx, A., Xavier, B., Bielen, K. et al. The endotracheal tube microbiome associated with Pseudomonas aeruginosa or Staphylococcus epidermidis. Sci Rep 6, 36507 (2016). https://doi.org/10.1038/srep36507
Received:
Accepted:
Published:
DOI: https://doi.org/10.1038/srep36507
This article is cited by
-
Logistic Normal Multinomial Factor Analyzers for Clustering Microbiome Data
Journal of Classification (2023)
-
Endotracheal tube microbiome in hospitalized patients defined largely by hospital environment
Respiratory Research (2022)
-
Strain-specific interspecies interactions between co-isolated pairs of Staphylococcus aureus and Pseudomonas aeruginosa from patients with tracheobronchitis or bronchial colonization
Scientific Reports (2022)
-
Third generation cephalosporins and piperacillin/tazobactam have distinct impacts on the microbiota of critically ill patients
Scientific Reports (2021)
-
Bidirectional alterations in antibiotics susceptibility in Staphylococcus aureus—Pseudomonas aeruginosa dual-species biofilm
Scientific Reports (2020)
Comments
By submitting a comment you agree to abide by our Terms and Community Guidelines. If you find something abusive or that does not comply with our terms or guidelines please flag it as inappropriate.