Abstract
Temperature is an important determinant of malaria transmission. Recent work has shown that mosquito and parasite biology are influenced not only by average temperature, but also by the extent of the daily temperature variation. Here we examine how parasite development within the mosquito (Extrinsic Incubation Period) is expected to vary over time and space depending on the diurnal temperature range and baseline mean temperature in Kenya and across Africa. Our results show that under cool conditions, the typical approach of using mean monthly temperatures alone to characterize the transmission environment will underestimate parasite development. In contrast, under warmer conditions, the use of mean temperatures will overestimate development. Qualitatively similar patterns hold using both outdoor and indoor temperatures. These findings have important implications for defining malaria risk. Furthermore, understanding the influence of daily temperature dynamics could provide new insights into ectotherm ecology both now and in response to future climate change.
Similar content being viewed by others
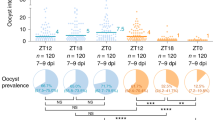
Introduction
Climate plays an important role in the dynamics and distribution of malaria1,2,3,4,5,6,7. Although rainfall is critical in providing suitable habitats for mosquitoes to breed3,8,9, temperature is a key driver of several of the essential mosquito and parasite life history traits that combine to determine transmission intensity, including mosquito development rate, biting rate and development rate and survival of the parasite within the mosquito (see Ref. 10). Accordingly, a number of studies have used environmental temperature (sometimes together with rainfall and/or humidity) to develop maps representing spatial and/or temporal variation in malaria transmission risk (e.g. Refs. 3,5,11,12,13).
A common feature of such studies is the characterization of environmental conditions using relatively coarse aggregate measures such as mean monthly temperatures. Recently, however, Paaijmans et al.14,15 demonstrated that malaria mosquito and parasite biology are influenced not only by average temperature, but also by the extent of the temperature variation that occurs throughout the day (see also Lambrechts, et al.16 for analogous effects with dengue). The effects, which appear to derive at least in part from non-linear rate summation (Jensen's inequality – see Refs. 17,18), lead to different influences of daily temperature dynamics depending on where the baseline mean temperature sits on the thermal performance curve of a particular trait. In general, daily fluctuation around cooler temperatures acts to speed up rate processes relative to the mean, fluctuation around warmer temperatures acts to slow them down and fluctuation around intermediate temperatures tends to have little net effect14,15. Thus, while use of mean temperatures might be appropriate under certain conditions, we expect in general that use of mean temperatures will either over or underestimate individual traits and hence, composite metrics of transmission intensity such as vectorial capacity or the basic reproductive rate (R0).
Here we examine how parasite development is expected to vary over time and space depending on the extent of diurnal temperature range and baseline mean temperature. We focus on parasite development as defined by the Extrinsic Incubation Period, or EIP, which describes the length of time it takes for a parasite to complete development within the mosquito from initial acquisition via an infected blood meal to the point at which it can be transmitted to another host via a further blood meal. The EIP is one of the most influential parameters determining malaria transmission intensity19,20. In addition, the EIP is known to be very sensitive to temperature and to vary non-linearly such that small changes in temperature can have potentially large effects on transmission1,15,21. We begin by examining four sites in Kenya using detailed temperature records to compare estimates of EIP based on measures of mean temperature vs. the hourly fluctuating temperatures experienced in the field. Utilizing these site-specific insights, we then scale up to explore the influence of daily temperature variation on EIP across the malaria transmission range within Kenya and then Africa. Consistent with expectations, we find contrasting effects of daily temperature dynamics across time and space, with both increases and decreases in parasite development relative to standard predictions based on mean temperatures. Determining where and when these different conditions apply could improve mechanistic understanding of malaria risk. More broadly, given that many aspects of ectotherm life history and fitness are characterized by non-linear thermal performance curves, we suggest that daily temperature dynamics could have marked effects on many species, affecting understanding of both current ecology and the expected responses to future climate change.
Results
Effects of environmental temperature on parasite development
We estimated the extrinsic incubation period of Plasmodium falciparum using long-term temperature data from four sites in Kenya: Kisumu, Garissa, Kitale and Kericho. Malaria transmission varies in both seasonality and intensity across these locations (e.g. see Refs. 11,12,22,23,24,25,26,27 and Table 1) with highest malaria incidence in Kisumu, followed by Kericho and Kitale24. Although Garissa has acute seasonal malaria transmission, parasite prevalence is low (<1% during the dry season)23. Even though malaria at these sites is variable, the sites were selected primarily to illustrate the contrasting thermal environments that can exist across relatively small spatial scales within a country. The sites are located at similar latitudes but vary in altitude (see Table 1) leading to marked differences in mean temperatures and diurnal temperature ranges (see Supplementary Fig. S1 online). Based on rate summation effects (discussed above), we expect these temperature differences to have divergent effects on parasite development (Figure 1). In Figure 1, the red line captures the full range of temperatures (minimum and maximum temperatures) at the field locations. Yellow circles represent the mean monthly temperature at each of the sites. Mean temperatures are coolest for Kericho (18°C) and Kitale (19°C), hottest for Garissa (29°C) and intermediate for Kisumu (24°C).
Predicted thermal performance of malaria parasite development within the mosquito15 in relation to the temperatures experienced at each of four study locations in Kenya, Africa.
The effects of temperature on EIP were estimated using the thermodynamic parasite development model of Paaijmans et al.15. This model describes the rate of Plasmodium falciparum development across the parasite's operative temperature range (15.4 – 35.0°C – see Figure 1, Supplementary Fig. S1 online). EIP was estimated for each site using three measures of temperature derived from the climate data (see equations 1–7 in Table 2): (i) mean monthly temperature, (ii) mean daily temperature and (iii) hourly temperatures (designated ‘monthly’, ‘daily’ and ‘hourly’ henceforth).
EIPs estimated using monthly, daily and hourly temperatures for each of the four sites are shown in Figure 2. For the warmest site (Garissa) the estimates of EIP based on mean monthly temperature and mean daily temperature were similar to one another, ranging from around 9–10.5 days (RMSE = 0.71). These EIPs were significantly shorter than the EIPs predicted using hourly temperatures, where parasite development was predicted to take between roughly 12–14 days (RMSEDaily vs Hourly = 2.41; RMSEMonthly vs Hourly = 2.81; H(2) = 827.8, P < 0.001).
Estimated mean (±95% C.I.) Extrinsic Incubation Period (EIP) (days) of P. falciparum for four locations in Kenya, calculated using monthly, daily or hourly temperatures.
Details of temperature time series given in Table 1.
Comparable patterns were also observed for the other ‘warm’ site, Kisumu. EIPs based on mean monthly temperature or mean daily temperatures were again similar, ranging from around 12.5–16.5 days across the year (RMSE = 0.57). The EIPs based on hourly temperatures were significantly longer, ranging from 15–18 days (RMSEDaily vs Hourly = 1.44; RMSEMonthly vs Hourly = 1.76; H(2) = 269.5, P < 0.001). Thus, in the warmer transmission environments, estimates of EIP increase as the resolution of the temperature data becomes more fine-scale. What we consider the ‘true’ EIP (i.e. based on the hour to hour fluctuations actually experienced by mosquitoes in the field) is longer than predicted from standard measures of environmental temperature such as daily or monthly means.
More marked but opposite patterns were revealed for the two ‘cool’ transmission sites. For Kericho, mean monthly and daily temperatures predicted similar parasite development (RMSE = 12.37), although because of the cooler temperatures, estimated EIPs were longer and more variable ranging from around 40–100 days (the temperature-growth relationship is strongly linear at lower temperatures so small changes in temperature have a greater effect on EIP). With hourly temperature data, estimated EIPs were greatly reduced ranging from 30–40 days (RMSEDaily vs Hourly = 22.8; RMSEMonthly vs Hourly = 28.46; H(2) = 152.4, P < 0.001).
For Kitale, EIPs based on mean monthly temperature or mean daily temperatures were again similar (23–50 days across the year, RMSE = 3.77). The EIPs based on hourly temperatures were significantly shorter, ranging from 23–35 days (RMSEDaily vs Hourly = 7.33; RMSEMonthly vs Hourly = 7.81; H(2) = 67.3, P < 0.001). Thus, in contrast to Kisumu and Garissa, in the cooler transmission environments, estimates of EIP decrease as the temporal resolution of the temperature data increases and the ‘true’ EIP is substantially shorter than predicted from daily or monthly means.
Country- and continent-wide implications
The preceding analysis indicates that estimates of parasite development rate differ depending on the temporal resolution of temperature data used (Figure 2). To estimate ‘true’ EIP we need to capture the influence of daily temperature fluctuations using hourly temperature data. Our four study sites were selected, in part, because of the availability of suitable temperature data. However, hourly temperature data do not exist for all locations in Kenya or across Africa. Accordingly, we approximated the average diurnal cycle for different locations by fitting a modified sine model28 through mean monthly minimum and maximum temperature series based on 10 years of data (WorldClim by Hijmans, et al.29), to generate estimated hourly temperatures representative of a given month. We then used these monthly average diurnal cycle data to drive the thermodynamic model of EIP. Although, this method does not replicate precise diurnal temperature curves for each day, it does provide a measure of average diurnal fluctuation to compare to the single value of mean monthly temperature used in most other studies.
When we compared the monthly average diurnal cycle predictions of EIP with the monthly means of the ‘true’ EIPs derived from the hourly temperatures (averaged for each month) for the four locations in Kenya (Figure 3) we found that for three of the sites there were no significant differences between these different measures of EIP (Kisumu: U = 68292.0, z = −0.31, P = 0.75. Kericho: U = 6506.0, z = −1.29, P = 0.12. Kitale: U = 32335.0, z = −1.43, P = 0.15). There was a significant difference between the two approaches at the warmest site, Garissa (U = 49841.0, z = −4.17, P < 0.001). For this site the EIP estimates generated from the hourly temperatures were longer than those generated from the monthly max-min model. However, although statistically significant, the differences were extremely small (RMSE = 0.45 days). EIP from the hourly temperatures ranged from 10.2–14.4 days with a mean (±95% C.I.) of 11.9 ± 0.18 days, while EIP from the monthly diurnal model ranged from 10.2–14.1 days with a mean of 11.7 ± 0.19 days. Such small differences are unlikely to have any biological relevance. Thus, use of mean monthly maximum and minimum temperatures to generate an average diurnal temperature profile yields robust estimates of the average of the ‘true’ EIPs exhibited across a month.
Comparison of mean (±95% C.I.) Extrinsic Incubation Periods (EIP) for four sites in Kenya calculated using a diurnal temperature cycle model based on monthly maximum and minimum temperatures (‘Monthly’ EIPs - black symbols) or the hourly temperatures for the equivalent months (Hourly EIPs - yellow symbols).
In Figure 4 we present maps of EIP for Kenya using one example month per quarter to represent variation across the year. We compare EIPs estimated using mean monthly temperatures (4A), with EIPs estimated using hourly temperatures from the average monthly diurnal temperature variation models (4B). Map 4C illustrates the percent change between A and B, with positive values (blue) indicating when B > A and negative values (brown) when B < A. These maps support the patterns described above, whereby mean temperatures overestimate parasite development rate under warm conditions, provide a good approximation of growth under intermediate conditions and underestimate development under cool conditions (Figure 4). For Kenya the most striking effects are in the highland areas to the west and north of Nairobi, where daily fluctuations in temperature suggest more extensive and more rapid (up to 100%) development than predicted using monthly mean temperatures. Constraining the EIP estimates to times where monthly rainfall >80 mm (this is a relatively conservative measure to describe the minimum rainfall required to support mosquito breeding – see methods) reduces the spatial distribution of potential malaria transmission but on the whole, does not lessen the relative differences between monthly and hourly predictions.
Maps illustrating number of days for malaria to become transmittable (EIP) across Kenya.
Map A illustrates the number of days taken to complete EIP using mean monthly temperatures. Map B illustrates the number of days taken to complete EIP using hourly temperatures based on an average diurnal cycle for the month. Map C illustrates the percent change between A and B. Positive values (blue) show when EIP values for B are greater than EIP values for A and negative values (brown) show when EIP values for B are less than EIP values for A. Hatched areas indicate where sufficient rainfall (> 80 mm) has fallen to support mosquito breeding.
Scaling up further, we see considerable spatial and temporal variation in parasite development across Africa (Figure 5). As above, map 5A illustrates EIPs based on mean monthly temperatures. Map 5B illustrates EIPs based on an average diurnal cycle for the month and map 5C, the percent difference between 5A and 5B. Within large parts of central Africa in and around the equator, the estimates of EIP are similar between approaches indicating that mean monthly temperature is appropriate for characterizing the transmission environment. However, there are also many areas within the current malaria transmission range where mean monthly temperatures under- or over- estimate EIP, sometimes by 100% or more. Again, adding the rainfall filter for mosquito breeding reduces the spatial distribution of potential malaria transmission but large differences between the monthly and hourly predictions remain. Given the sensitivity of malaria transmission to EIP (or more precisely the length of the EIP relative to daily mosquito survival13), such errors have important implications for understanding the spatial and temporal variation in malaria dynamics. For example, assuming a daily survival probability of adult mosquitoes of 0.865, a 5 day increase in EIP from a baseline value of 10 days would reduce the proportion of mosquitoes living long enough to transmit malaria from 0.221 to 0.104, which, assuming all else to be equal, would approximate a halving in transmission potential. Conversely, if conditions are cooler and the true EIP is predicted to be 30 days rather than 45 days, the proportion of mosquitoes living long enough to transmit malaria would increase from 0.001 to 0.011, representing an order of magnitude increase in transmission potential.
Maps illustrating number of days for malaria to become transmittable across Africa within the defined malaria transmission zone utilizing outdoor temperature.
Maps A, B and C as in Figure 4.
Thus far we have considered the influence of outdoor temperatures. This approach follows nearly every other malaria-temperature study published to date so is consistent with the ‘industry standard’. However, adult mosquitoes can rest indoors (endophily) as well as outdoors (exophily), although there appears considerable variation between and within species (see later discussion). Data on the relationship between indoor and outdoor temperatures are extremely scant but to enable a preliminary investigation we apply simple regressions to predict indoor maximum and minimum temperatures based on published data from 8 locations in East Africa30. We use these regressions to generate maps of EIP corrected for indoor temperatures (Figure 6). We find that the general buffering nature of indoor environments reduces daily temperature variation and increases mean temperatures. Accordingly, the differences between predictions of EIP based on mean temperatures vs. hourly temperatures are reduced compared to the outdoor situation. However, the broad patterns are qualitatively similar (compare map 5C with 6C) and daily temperature variation still leads to percent differences in EIP of –100% to +77% relative to the monthly means. The differences are reduced slightly with the addition of the rainfall filter but still range between −50% to +50%.
Maps illustrating number of days for malaria to become transmittable across Africa within the defined malaria transmission zone using indoor temperature.
Maps A, B and C as in Figure 4.
Discussion
Our aim in the current study was to explore how different measures of environmental temperature influence estimates of the EIP. The current temperature-dependent models for the EIP of P. falciparum1,15,31 draw heavily on limited empirical data from studies conducted on a Eurasian mosquito vector in the early part of last century1,19. EIPs from field infections have rarely been compared to model outputs. Given the significance of EIP in determining transmission intensity and how widely the standard degree-day model of Detinova1 has been applied, it is surprising how little work has been done to validate this relationship and to quantify whether, for example, the temperature-dependence of P. falciparum (or P. vivax) is consistent across different mosquito species and/or parasite clones.
These issues notwithstanding, we find that estimates of EIP depend strongly on the temporal resolution of the temperature data used to drive the parasite development model. Under cool conditions (and especially with large diurnal temperature ranges) the use of standard metrics such as mean monthly temperatures will tend to underestimate parasite development. This effect is nicely illustrated for Kericho in the Kenyan highlands where EIPs predicted using mean daily or monthly temperatures frequently exceed the maximum predicted lifespan of the mosquito vectors (56 days, see Ref. 3), including during the known peak malaria season (March-July). In contrast, under warmer conditions, use of mean temperatures alone will over-estimate parasite development. Thus, in areas of the Sahel and also Garissa, our warmest site in Kenya, we predict the true EIP to be longer than predicted using mean temperatures. At intermediate temperatures, such as in the thermally stable lowland environments around the equator, mean temperatures appear to provide a good approximation of the thermal environment. Kisumu in western Kenya is representative of this situation, with very small differences between the different estimates of EIP.
The EIP is only one parameter determining transmission intensity and so the results presented here are not intended to provide quantitative measures of malaria transmission risk. In different areas malaria might be constrained by many factors including low humidity32, lack of water for breeding sites32,33 or by human activities34, irrespective of EIP. Indeed, when we add a constraint for the minimum rainfall necessary for mosquito breeding, we find that the spatial extent of potential malaria transmission in any one period is reduced. However, the importance of daily temperature variation remains. Other studies have considered a broader set of both biological and environmental parameters to determine malaria transmission intensity3,11,12,13. This research includes some influential malaria mapping work to inform contemporary public health strategies12,35,36. Yet no studies have explored the influence of daily temperature variation in the way we consider here. Use of simple degree-day models that consider only the linear parts of a thermal performance curve will tend not to capture the full effects of daily temperature dynamics (e.g. see Refs. 7,11).
Individual mosquito species tend to exhibit different extents of endophily vs. exophily and while there are some generalizations, resting behaviour appears to be relatively plastic30. Indeed, some studies report no significant tendency for repeated endophily or exophily for even the same individual mosquito37,38. Moreover, there is evidence that current vector control tools such as indoor residual insecticide sprays and insecticide treated nets are selecting for outdoor biting and resting39,40,41. Thus, characterizing the effects of outdoor temperatures is clearly relevant to malaria transmission ecology. That said, indoor resting is also important yet virtually no studies have considered indoor temperatures (see Refs. 30,42 for exceptions). The data on indoor temperatures are extremely limited and the conditions themselves are expected to vary considerably depending on house design, construction materials, housing density, adjacent vegetation cover and altitude30,42. Given the limited nature of the data, the regressions we apply to predict indoor temperatures based on outdoors should be treated with some caution. Nonetheless, our analysis suggests that while indoor environments are relatively thermally buffered, daily temperature variation still matters.
More broadly, our analysis of EIP illustrates the effects of temperature on a life history trait characterized by a non-linear, asymmetric thermodynamic curve. Several of the other mosquito traits that contribute directly or indirectly to malaria transmission intensity (e.g. fecundity, duration of gonotrophic cycle, larval development rate), follow this general pattern3,4,43. Such curves have also been widely used to describe the thermal performance of other taxa, considering both individual life history traits and composite fitness metrics such as intrinsic rate of increase (r) and basic reproductive numbers (Ro)44,45,46,47. Thermal performance curves tend to be derived from constant temperature experiments conducted under controlled laboratory conditions (e.g. see the majority of species listed in46). As with the EIP, integrating the effects of daily variation around mean temperatures will likely alter estimates of performance over both time and space; we suggest that the qualitative patterns we have revealed will apply to multiple traits across diverse systems.
Numerous studies provide evidence that short-term environmental variation has the potential to affect life history traits and fitness above and beyond the effects of mean temperatures alone (e.g. Refs. 14,16,18,48,49,50,51,52). Yet other studies highlight the importance of temperature fluctuations for acclimation and adaptation53,54 and in the evolution of thermal optima55,56. However, in spite of this research, the vast majority of ecological studies examining temperature-dependent effects consider mean temperatures alone. Incorporating the effects of daily temperature variation could improve mechanistic understanding of ectotherm ecology and provide new insights into likely impacts of anthropogenic climate change on pest and disease risk20,50,57, species range expansions and contractions58,59 and biodiversity loss53,60.
Methods
Environmental characteristics
Daily minimum and maximum temperature data for each of the four sites in Kenya were obtained from the Global Surface Summary of the Day Database (Version 7), National Climate Data Center website (http://www.ncdc.noaa.gov/) (GSOD NOAA). Consecutive years with the least number of missing days were selected to represent each station (Table 1). Missing temperature values were infilled using temperature averages based on the 15 days preceding and 15 days following the missing date across all the years in the data set. These long-term temperature data were used to estimate extrinsic incubation period of Plasmodium falciparum from four sites in Kenya: Kisumu, Garissa, Kitale and Kericho.
Quantifying the effects of environmental temperature on parasite development
The rate of parasite development across a range of temperatures was estimated by the model of Paaijmans et al.15. This model was used to estimate the effects of monthly, daily and hourly temperatures on EIP for each site (see equations 1–7 in Table 2). The model largely follows the widely used degree-day model for malaria parasite development of Detinova1 but allows for the effect of temperature fluctuations that extend beyond the linear part of the thermal performance curve. Where temperatures fell outside the operative range, development was set to zero. For each of these estimates, growth rates from the temperature-dependent model were accumulated using the respective temperature measures until they reached a value of 1, which defines the completion of the extrinsic incubation period. For the ‘hourly’ estimates we fitted a modified sine model28 to interpolate between the daily maximum and minimum temperatures and accumulated development rate at hourly intervals. An upper threshold for the EIP duration was set to 56 days, the upper limit of mosquito longevity used in a number of previous studies3,15. The data and models used to perform these assessments are summarized in Table 2. Thus, monthly EIP was calculated using a single mean monthly temperature value; daily EIP was calculated for each day of the year using the daily mean temperature; hourly EIP was calculated for each hour of each day of the year using the hourly mean temperature.
Initial comparisons between EIP estimates at the different temporal scales were made by calculating the root mean square error. The root mean squared error (RMSE)-- the square root of the variance of the difference plus the square of the mean (Eq. 11, Table 2)-- is an absolute indication of the difference between measurement scales. The lower the RMSE value, the less difference in EIP and the larger value, the greater the difference. In addition we tested our results to see if differences in EIP were significant. EIP measurement variance was not homogenous and could not be transformed to meet Gaussian assumptions, hence non-parametric tests were used. A Kruskal-Wallis test using Monte Carlo methods was used for comparing the monthly, daily and hourly estimates for each site. Subsequently tests between measurement levels were conducted using Mann-Whitney tests again using the Monte Carlo method. Because of the danger of inflating the Type 1 error rate, a Bonferroni correction was applied and all effects are reported at the 0.0167 level of significance for these post hoc tests.
Mapping EIP across Africa
To conduct the broad-scale spatial analyses we integrated the parasite development model into a Geographic Information System (GIS) (equations in Table 2). Minimum and maximum mean monthly temperature surfaces were created using thinplate smoothing spline interpolation method and obtained from WorldClim, version 1.4 (release 3) (http://www.worldclim.org29). These surfaces were imported into ESRITM ArcGIS ArcView 10 and integrated with the Paaijmans et al.15 model to create monthly and hourly EIP maps for Africa. Monthly estimates were created using equation 1 (Table 2) and hourly estimates were made using equation 4–7 (Table 2).
Numerous malaria-modeling studies have utilized WorldClim data (e.g. Refs. 6,7,47). As partial validation of these data, we compared the GSOD NOAA monthly temperature values (estimated from the daily temperature data values) with the WorldClim monthly values used to generate the temperature surfaces for each of the four sites in Kenya. This analysis revealed Root Mean Square Errors ranging from 0.33–1.98 for all paired comparisons of minimum, maximum and mean monthly temperature for each site. Root Mean Square Errors were smallest for mean monthly temperature (0.33 (Kitale), 0.47 (Kisumu) and 0.51 (Garissa and Kericho)) with greater differences occurring between sites for minimum temperatures (0.44 (Garissa), 1.0 (Kisumu), 1.97 (Kericho) and 1.98 (Kitale)) and maximum temperature (0.68 (Kisumu), 1.15 (Kericho), 1.36 (Garissa) and 1.59 (Kitale)).
Indoor temperature estimates were determined using the regression equations (Eq. 8, 9 and 10) in Table 2 that capture the relationship between indoor and outdoor temperatures at different elevations30. These regressions were used to convert the outdoor temperature surfaces to matching estimates of indoor temperatures. We then applied appropriate equations from Table 2 to generate estimates of EIP based on indoor monthly mean temperatures and the average daily temperature range.
Limits of Plasmodium falciparum malaria transmission zones were obtained from the 2007 malaria map of Africa from the malaria atlas project (http://www.map.ox.ac.uk/data12). An image of the map was brought into ArcGIS ArcView 10, geo-referenced and the malaria transmission limits were digitized. The limits were used to constrain our mapped outputs to the existing malaria transmission boundaries. We add a further possible filter on transmission by highlighting (hatched shading) areas that received greater than 80 mm of rainfall on average during the time period shown. This level of rainfall has been used elsewhere to indicate conditions suitable for mosquito breeding (see Ref. 3), although it ignores the influence of permanent water bodies, dams, wells, irrigated areas, water storage containers etc. that can sustain mosquito populations under reduced rainfall conditions61. Monthly rainfall data were obtained from WorldClim.
References
Detinova, T. S. Age-grouping methods in Diptera of medical importance with special reference to some vectors of malaria. Monograph Series. World Health Organization 47, 13–191 (1962).
Noden, B. H., Kent, M. D. & Beier, J. C. The impact of variations in temperature on early Plasmodium falciparum development in Anopheles stephensi. Parasitology 111, 539–545 (1995).
Craig, M. H., Snow, R. W. & le Sueur, D. A climate-based distribution model of malaria transmission in sub-Saharan Africa. Parasitol Today 15, 105–111 (1999).
Bayoh, M. N. & Lindsay, S. W. Effect of temperature on the development of the aquatic stages of Anopheles gambiae sensu stricto (Diptera: Culicidae). Bulletin of Entomological Research 93, 375–381 (2003).
Kiszewski, A. et al. A global index representing the stability of malaria transmission. Am J Trop Med Hyg 70, 486–498 (2004).
Guerra, C. A. et al. The limits and intensity of Plasmodium falciparum transmission: Implications for malaria control and elimination worldwide. Plos Medicine 5, 300–311 (2008).
Gething, P. W. et al. Modelling the global constraints of temperature on transmission of Plasmodium falciparum and P. vivax. Parasite & Vector 4 (2011).
Grover-Kopec, E. et al. An online operational rainfall-monitoring resource for epidemic malaria early warning systems in Africa. Malaria J 4 (2005).
Thomson, M. C., Mason, S. J., Phindela, T. & Connor, S. J. Use of rainfall and sea surface temperature monitoring for malaria early warning in Botswana. Am J Trop Med Hyg 73, 214–221 (2005).
Mordecai, E. A. et al. Optimal temperature for malaria transmission is dramatically lower than previously predicted. Ecology letters 16, 22–30 (2013).
Gething, P. W. et al. A new world malaria map: Plasmodium falciparum endemicity in 2010. Malaria J 10, 378 (2011).
Hay, S. I. et al. A world malaria map: Plasmodium falciparum endemicity in 2007. PLOS Medicine 6 (2009).
Lindsay, S. W. & Martens, W. J. M. Malaria in the African highlands: past, present and future. Bull WHO 76, 33–45 (1998).
Paaijmans, K. P. et al. Influence of climate on malaria transmission depends on daily temperature variation. P Natl Acad Sci USA 107, 15135–15139 (2010).
Paaijmans, K. P., Read, A. F. & Thomas, M. B. Understanding the link between malaria risk and climate. P Natl Acad Sci USA 106, 13844–13849 (2009).
Lambrechts, L. et al. Impact of daily temperature fluctuations on dengue virus transmission by Aedes aegypti. P Natl Acad Sci USA 108, 7460–7465 (2011).
Ruel, J. J. & Ayres, M. P. Jensen's inequality predicts effects of environmental variation. Trends Ecol Evol 14, 361–366 (1999).
Martin, T. L. & Huey, R. B. Why "suboptimal" is optimal: Jensen's inequality and ectotherm thermal preferences. Am. Nat. 171, E102–118 (2008).
Macdonald, G. The epidemiology and control of Malaria. (Oxford University Press, 1957).
Rogers, D. J. & Randolph, S. E. in Advances in Parasitology, Vol 62: Global Mapping of Infectious Diseases: Methods, Examples and Emerging Applications. Vol. 62, Advances in Parasitology (eds Hay, S. I., Graham, A. & Rogers, D. J.) 345–381 (2006).
Smith, D. L. et al. Ross, Macdonald and a theory for the dynamics and control of mosquito-transmitted pathogens. PLoS pathog 8 (2012).
Githeko, A. K. et al. Some observations on the biting behavior of Anopheles gambiae s.s., Anopheles arabiensis and Anopheles funestus and their implications for malaria control. Exp Parasitol 82, 306–315 (1996).
Lawford, H. et al. Adherence to prescribed artemisinin-based combination therapy in Garissa and Bunyala districts, Kenya. Malaria J 10, 281(2011).
Okiro, E. et al. Malaria paediatric hospitalization between 1999 and 2008 across Kenya. BMC Med 18, (2009).
Noor, A. M. et al. The risks of malaria infection in Kenya in 2009. BMC Inf Dis 9, (2009).
Shanks, G. D., Hay, S. I., Omumbo, J. A. & Snow, R. W. Malaria in Kenya's western highlands. Emerg Infect Dis 11, 1425–1432 (2005).
Snow, R. W. et al. The coverage and impact of malaria intervention in Kenya 2007–2009. Division of Malaria Control, Ministry of Public Health and Sanitation, December 2009 (2009) <http://www.map.ox.ac.uk/client_media/publications/DOMC_ME_report_2007-09_281109.pdf>. Accessed Dec 18th 2011.
Parton, W. J. & Logan, J. A. A model for diurnal-variation in soil and air-temperature. Agr Meteorol 23, 205–216 (1981).
Hijmans, R. J., Cameron, S. E., Parra, J. L., Jones, P. G. & Jarvis, A. Very high resolution interpolated climate surfaces for global land areas. Int J Climatol 25 (2005).
Paaijmans, K. P. & Thomas, M. B. The influence of mosquito resting behaviour and associated microclimate for malaria risk. Malaria J 10, 183 (2011).
Ikemoto, T. Tropical malaria does not mean hot environments. J. Med. Entomol. 45, 963–969 (2008).
Gray, E. M. & Bradley, T. J. Physiology of desiccation resistance in Anopheles gambiae and Anopheles arabiensis. Am J Trop Med Hyg 73, 553–559 (2005).
Shililu, J. I. et al. Development and survival of Anopheles gambiae eggs in drying soil: influence of the rate of drying, egg age and soil type. J Am Mosquit Contr 20, 243–247 (2004).
Gething, P. W., Patil, A. P. & Hay, S. I. Quantifying aggregated uncertainty in Plasmodium falciparum malaria prevalence and populations at risk via efficient space-time geostatistical joint simulation. PLoS Comp Biol 6, e1000724 (2010).
Smith, D. L., Smith, T. A. & Hay, S. I. in In Shrinking the Malaria Map: a Prospectus on Malaria Elimination (ed Feachem, R. G. A., Phillips, A. A. and Targett, G. A. on behalf of theMalaria Elimination Group) 108–126. (The Global Health Group, University of California, San Francisco: San Francisco, U.S.A., 2009).
White, M. T. et al. Modelling the impact of vector control interventions on Anopheles gambiae population dynamics. Parasit Vectors 4, 153 (2011).
Githeko, A. K., Service, M. W., Mbogo, C. M. & Atieli, F. K. Resting behaviour, ecology and genetics of malaria vectors in large scale agricultural areas of Western Kenya. Parassitologia 38, 481–489 (1996).
Lines, J. D., Lyimo, E. O. & Curtis, C. F. Mixing of indoor-resting and outdoor-resting adults of Anopheles gambiae Giles S. L. and Anopheles funestus Giles (Diptera, Culicidae) in Coastal Tanzania. Bulletin of Entomological Research 76, 171–178 (1986).
Reddy, M. R. et al. Outdoor host seeking behaviour of Anopheles gambiae mosquitoes following initiation of malaria vector control on Bioko Island, Equatorial Guinea. Malaria J 10, 184 (2011).
Stevenson, J. et al. Novel vectors of malaria parasites in the Western highlands of Kenya. Emerg Infect Dis 18, 1547–1549 (2012).
Bayoh, M. N. et al. Anopheles gambiae: historical population decline associated with regional distribution of insecticide-treated bed nets in western Nyanza Province, Kenya. Malaria J 9, 62 (2010).
Alonso, D., Bouma, M. J. & Pascual, M. Epidemic malaria and warmer temperatures in recent decades in an East African highland. Proc R Soc B 278, 1661–1669 (2011).
Lardeux, F. J., Tejerina, R. H., Quispe, V. & Chavez, T. K. A physiological time analysis of the duration of the gonotrophic cycle of Anopheles pseudopunctipennis and its implications for malaria transmission in Bolivia. Malaria J 7 (2008).
Briere, J. F., Pracros, P., Le Roux, A. Y. & Pierre, J. S. A novel rate model of temperature-dependent development for arthropods. Environ. Entomol. 28, 22–29 (1999).
Clusella-Trullas, S., Blackburn, T. M. & Chown, S. L. Climatic predictors of temperature performance curve parameters in ectotherms imply complex responses to climate change. Am. Nat. 177, 738–751(2011).
Deutsch, C. A. et al. Impacts of climate warming on terrestrial ectotherms across latitude. P Natl Acad Sci USA 105, 6668–6672 (2008).
Parham, P. E. & Michael, E. Modelling climate change and malaria transmission. Adv Exp Med Biol 673, 184–199 (2010).
Duncan, A. B., Fellous, S. & Kaltz, O. Temporal variation in temperature determines disease spread and maintenance in Paramecium microcosm populations. Proc R Soc B 278, 3412–3420 (2011).
Klass, J. I., Blanford, S. & Thomas, M. B. Development of a model for evaluating the effects of environmental temperature and thermal behaviour on biological control of locusts and grasshoppers using pathogens. Agr Forest Entomol 9, 189–199 (2007).
Rohr, J. R. et al. Frontiers in climate change-disease research. Trends Ecol Evo 26, 270–277 (2011).
Liu, S. S., Zhang, G. M. & Zhu, J. Influence of temperature-variations on rate of development in insects - analysis of case-studies from entomological literature. Ann Entomol Soc Am 88, 107–119 (1995).
Bozinovic, F. et al. The mean and variance of environmental temperature interact to determine physiological tolerance and fitness. Physiol Biochem Zool 84, 543–552 (2011).
Dillon, M. E., Wang, G., Garrity, P. A. & Huey, R. B. Thermal preference in Drosophila. J Therm Biol 34, 109–119 (2009).
Rohr, J. R. & Raffel, T. R. Linking global climate and temperature variability to widespread amphibian declines putatively caused by disease. P Natl Acad Sci USA 107, 8269–8274 (2010).
Asbury, D. A. & Angilletta, M. J. Thermodynamic eEffects on the evolution of performance curves. Am. Nat. 176, E40–E49 (2010).
Frazier, M. R., Huey, R. B. & Berrigan, D. Thermodynamics constrains the evolution of insect population growth rates: "Warmer is better". Am Nat 168, 512–520 (2006).
Dangles, O., Carpio, C., Barragan, A. R., Zeddam, J. L. & Silvain, J. F. Temperature as a key driver of ecological sorting among invasive pest species in the tropical Andes. Ecol Appl 18, 1795–1809 (2008).
Chen, I. C., Hill, J. K., Ohlemuller, R., Roy, D. B. & Thomas, C. D. Rapid range shifts of species associated with high levels of climate warming. Science 333, 1024–1026 (2011).
Pateman, R. M., Hill, J. K., Roy, D. B., Fox, R. & Thomas, C. D. Temperature-dependent alterations in host use drive rapid range expansion in a butterfly. Science 336, 1028–1030 (2012).
Thomas, C. D. et al. Extinction risk from climate change. Med Vet Entomol 427, 145–148 (2004).
Walker, K. & Lynch, M. Contributions of Anopheles larval control to malaria suppression in tropical Africa: review of achievements and potential. Med Vet Entomol 21, 2–21 (2007).
Acknowledgements
This work was supported by the NSF-EID program (no. EF-0914384).
Author information
Authors and Affiliations
Contributions
Conceived and designed paper: M.B.T., K.P.P., R.G.C., M.E.M., S.B., J.I.B. Data Analysis: J.I.B., S.B., K.V.S. Wrote the paper: M.B.T. and J.I.B., with additional input from S.B., K.V.S. All authors commented on and approved the final version of the manuscript.
Ethics declarations
Competing interests
The authors declare no competing financial interests.
Electronic supplementary material
Supplementary Information
Supplementary Info
Rights and permissions
This work is licensed under a Creative Commons Attribution-NonCommercial-NoDerivs 3.0 Unported License. To view a copy of this license, visit http://creativecommons.org/licenses/by-nc-nd/3.0/
About this article
Cite this article
Blanford, J., Blanford, S., Crane, R. et al. Implications of temperature variation for malaria parasite development across Africa. Sci Rep 3, 1300 (2013). https://doi.org/10.1038/srep01300
Received:
Accepted:
Published:
DOI: https://doi.org/10.1038/srep01300
This article is cited by
-
Impact of climate change on temperature variations and extrinsic incubation period of malaria parasites in Chennai, India: implications for its disease transmission potential
Parasites & Vectors (2024)
-
Malaria, climate variability, and interventions: modelling transmission dynamics
Scientific Reports (2023)
-
Implications of Climate Change and Anopheles stephensi Liston in Africa: Knowledge Gaps and Lessons from History
Current Tropical Medicine Reports (2023)
-
Effect of fluralaner on the biology, survival, and reproductive fitness of the neotropical malaria vector Anopheles aquasalis
Malaria Journal (2023)
-
Plasmodium vivax: the potential obstacles it presents to malaria elimination and eradication
Tropical Diseases, Travel Medicine and Vaccines (2022)
Comments
By submitting a comment you agree to abide by our Terms and Community Guidelines. If you find something abusive or that does not comply with our terms or guidelines please flag it as inappropriate.