Abstract
Feed efficiency (FE) is an important economic indicator in pig production. Improving the FE of commercial pigs is an important strategy for minimizing pig production costs and providing sustainability to the pig industry. In this study, nontargeted LC–MS metabolomics was performed on the contents of the three intestine segments (ileum, cecum and colon) of high-FE and low-FE pigs to explore the effects of small-molecule metabolites in pig intestine on pig FE. A total of 225 Duroc × (Landrace × Yorkshire) pigs in the 30–100 kg stage were sorted based on FE, and 20 pigs with extreme phenotypes were selected, with 10 in each group. A total of 749 metabolites were identified, of which 15, 38 and 11 differed between high-FE and low-FE pigs in ileum, cecum and colon, respectively. These candidate biomarkers mainly comprised lipids and organic acids, which could partially explain the FE difference between the two groups. Among the identified differential metabolites, the lipids are mainly involved in combatting inflammation and oxidation in the ileum and cecum and in bile acid metabolism and vitamin D absorption in the cecum. A difference in organic acids was mainly observed in the hindgut, which is involved in the metabolism of amino acids and fatty acids. This comprehensive study provides new insight into the biochemical mechanisms associated with pig FE.
Similar content being viewed by others
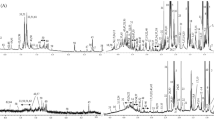
Introduction
Feed costs are a major component of pig production costs1. Feed efficiency (FE), an important economic trait in pig production, is often indirectly measured as either the feed conversion ratio (FCR) or the residual feed intake (RFI)2,3. Low FE can cause the excessive release of nutrients, such as phosphorus and nitrogen, into the environment, causing environmental pollution4. In addition, low-FE pigs consume more feed than high-FE individuals before reaching the market weight standard and thus reduce the income and efficiency of pig producers. Therefore, improving FE is an essential way to reduce pig production costs and provide sustainability to the pig industry.
The FE of pigs is affected by many factors, including pig genetics, disease, production management, and intestinal microorganisms5,6,7. In addition, gut microbiota affect host metabolism and health through the production of metabolites8. Our previous studies have shown that the microbiota composition and functions in different intestinal locations of Duroc × (Landrace × Yorkshire) (DLY) pigs affect intestinal physiological function, and many microbes were identified that can potentially affect pig FE9. In this study, we further investigated the correlation between the intestinal contents of metabolites and FE in different intestinal regions through nontargeted metabolome detection.
The microbiota, microbial metabolites, secretions of the host gastrointestinal tract, and exogenous nutritional molecules constitute a complex and dynamic intestinal environment10. Untargeted and targeted mass spectrometry-based metabolomics enables the analysis of large numbers of small-molecule metabolites in biological samples. Compared with nuclear magnetic resonance (NMR), mass spectrometry (MS) is more sensitive and can measure higher numbers of molecules in samples11. Gas chromatography-mass spectrometry (GC–MS) can obtain better separation of metabolites than the liquid chromatography-mass spectrometry (LC–MS) when the derivatization information of metabolic species is provided before chemical analysis. In general, no derivatization is performed in LC–MS metabolic profiling, but it can be applied for more targeted analysis or to increase selectivity or sensitivity12. In this study, we used nontargeted LC–MS to identify the composition of metabolites in different intestinal locations and explore the metabolites potentially associated with pig FE.
Result
Phenotypic variables
The twenty selected pigs were evaluated for feeding efficiency and growth traits. There were significant differences in AFI, FCR and RFI between the high-FE group and the low-FE group (P < 0.05). The average AMBW and ADG of the HFE group were higher than those of the LFE group, but the differences were not significant (P = 0.12 and 0.17, respectively) (Table 1).
Metabolome profile
To identify the small-molecule metabolites related to FE variation in pigs, we selected ten gilts with high FE and ten with low FE for metabolomic analysis of intestinal contents (Fig. 1). After quality control and annotation, a total of 749 metabolites were detected in samples from the three different intestinal locations and analyzed. Principal coordinate analysis (PCoA) analysis revealed strong separation of metabolites among the three locations, suggesting that the metabolic profile differs among these three intestinal segments. However, there was no clear separation of the samples between the high- and low-FE groups in any of the three locations (Fig. 2).
Analysis of differential metabolites
To identify the differential metabolites in each location between the two groups, initial screening of the metabolites was performed using ANOVA (P < 0.1)13. After the initial screening, 31, 84 and 25 metabolites in the three locations were selected. These metabolites were further screened according to VIP value from the OPLS-DA model (VIP > 1), yielding 15, 38 and 11 differential metabolites between the two groups for the three intestine locations (Fig. 3). These metabolites were classified into 9 major categories and more than 30 subcategories (Fig. 4).
Metabolic pathway analysis
Metabolic pathway analysis of all the differential metabolites in each intestine location was performed. In cecum, pathway analysis identified 3 pathways that were enriched (tryptophan metabolism, steroid biosynthesis and purine metabolism), but none were significantly enriched. There were no enriched pathways in the ileum and colon (see Supplementary Fig.S1).
Lipids and organic acids
We focused on the major categories of metabolites that likely contribute to feed efficiency in pigs, such as lipids and organic acids (Table 2). Lipids were identified in all intestinal locations, with prenol lipids, steroids and steroid derivatives composing the majority. In ileum and cecum, prenol lipids occurred at higher concentrations in the high-FE group. Steroids and steroid derivatives were found at higher concentrations in cecum but lower concentrations in colon in the high-FE group than in the low-FE group.
The organic acids mainly comprised amino acids, peptides, and analogues in the ileum and cecum. These metabolites tended to occur at higher concentrations in the high-FE group. Three organic acids in colon occurred at higher levels in the high-FE group: a carboxylic acid, a hydroxy acid and a keto acid.
Discussion
Global profiling of intestinal contents can provide insight into metabolic factors associated with feed efficiency6,14. Our study performed nontargeted metabolomics on content samples from three intestinal locations of pigs grouped into two feed-efficiency groups to identify potential FE-related intestinal metabolites. The metabolic profiles significantly differed among the different parts of the intestine. Metabolomics testing using the contents of a single part of the intestine cannot comprehensively reveal the host's intestinal metabolic activity. We identified a total of 785 metabolites in the three intestinal locations, of which 31, 84 and 25 were differentially accumulated in ileum, cecum and colon, respectively, between the two groups. As potential biomarkers of interest, we focused on lipids and organic acids, which have been considered to be related to FE in pigs in previous studies15,16,17.
The lipids identified in ileum and cecum included a variety of triterpenoid metabolites that occurred at higher concentrations in the high-FE group (liquoric acid, soyasapogenol C, and ganoderenic acid C in ileum and ginsenoside Rf, isothankunic acid, melilotoside A, and methyl glycyrrhetate in cecum). Triterpenoids are important natural products of plant origin that exhibit a wide range of biological activities, such as protecting the integrity of the intestinal barrier, regulating intestinal microbiota and inhibiting inflammation in the gastrointestinal tract18,19,20,21. The high levels of these metabolites in the high-FE group may partially explain the difference in FE between the groups.
In addition, several bile acids and provitamin D were identified in the cecum. Bile acids facilitate the absorption and metabolism of dietary lipids and fat-soluble vitamins22. Vitamin D (VD), as a fat-soluble prohormone steroid, improves calcium absorption, mediates the immune response and tempers inflammation in the intestine23. Previous studies have found that oral probiotics can increase VD levels in plasma24. In our study, the high-FE pigs had higher levels of secondary bile acids (nutriacholic acid) and provitamin D (7-dehydrocholesterol and 22,23-dihydroergosterol), which suggests a potentially increased conversion rate of bile acids from the primary to the secondary form and potentially increased efficiency of VD metabolism and utilization in high-FE pigs.
The organic acids in cecum with higher levels in the high-FE pigs were mainly amino acids and their derivatives. Cecal bacteria can use these amino acids for the synthesis of microbial proteins25. Among these organic acids, molecules related to the central inhibitory gamma-aminobutyric acid (GABA) systems were of particular interest (D-lysine, D-pipecolic acid and 5-aminopentanoic acid). Previous studies have shown that GABA has physiological functions, such as promoting animal feed intake and combatting stress20,26. Pipecolic acid and 5-aminopentanoic acid can be produced endogenously and through intestinal bacterial catabolism of lysine. Pipecolic acid is considered a neurotransmitter and plays a role in the central inhibitory GABA systems27. 5-aminovalerate, as a methylene homologue of GABA, functions as a weak GABA agonist28. Thus, our results suggest that the observed differences in these amino acids between groups contributed to the group differences in feeding efficiency.
Hydroxy fatty acids and oxo fatty acids are intermediates in intestinal microbial fatty acid metabolism29. Their higher colon concentrations in the high-FE pigs suggest that fatty acid biosynthesis and metabolism in colon might affect the feed efficiency of pigs.
Conclusion
The aim of this work was to detect a molecular signature of the feed efficiency of DLY commercial pigs by performing untargeted metabolomics. This work provides comprehensive information regarding the metabolites in pig intestines. The molecular signature reveals the lipids and organic acids in intestine as important metabolites for feed efficiency. The identified differential lipids are mainly involved in combatting inflammation and oxidation in the ileum and cecum and in bile acid metabolism and vitamin D absorption in the cecum. The differences in organic acids were observed mainly in the hindgut, which is involved the metabolism of amino acids and fatty acids.
Methods
Ethics statement
All experimental procedures followed the ARRIVE guidelines (http://www.nc3rs.org.uk/arrive-guidelines). The 225 female DLY pigs in this study were all provided by Guangdong Wen's Foodstuffs Group Co., Ltd. (Yunfu, China). The care and use of animals in this study were approved and conducted according to standards established by the Animal Care and Use Committee (ACUC) of the South China Agriculture University (SCAU) (Guangzhou, China) (approval number SCAU#0032). The intestinal contents were sampled at the same intestinal locations in each pig that had been selected for slaughter. Briefly, the luminal contents of the ileum and colon were collected separately from the middle section, and the cecum contents were collected at the distal end of the cecum. All samples were collected within 30 min after slaughter and immediately placed in liquid nitrogen. The samples were returned to the laboratory and stored at − 80 °C for subsequent analysis.
Phenotypic data collection
The feed intake and weight gain of all experimental pigs were recorded by an Osborne Feed Intake Recording Equipment (FIRE) Pig Performance Testing System (Osborne, KS, United States) in this study. These data were recorded when pig body weight (BW) was between 30 and 100 kg. The FCR value of each pig was calculated after the measurements of feed intake and weight gain were completed. RFI value was calculated by the method reported by Cai et al.30, which considers the midtest metabolic BW (MBW), average daily gain (ADG) and back fat (BF). The MBW was calculated as [(BW at on-test + BW at off-test)/2]0.75. Then, the FE performance of 225 animals was ranked according to FCR value, and 50 pigs with extreme FE were assigned two groups (25 highest FCR and 25 lowest FCR) and 10 pigs were randomly selected from each group to be slaughtered. The specific phenotypes of these 20 pigs are given in the Supplementary Table S1. Subsequently, the contents at three gut locations (ileum, cecum and colon) were collected from each pig. All samples were immediately stored in liquid nitrogen, transferred to the laboratory and stored at − 80 °C until LC–MS analysis. The R base package was used to detect differences in phenotypic traits between the two groups. The Shapiro–Wilk test was performed to examine the normality of the data. The Student t-test was applied for intergroup comparisons of normally distributed variables, and the Wilcoxon test was used for nonparametric variables. The results were considered significant at P-value < 0.05.
LC–MS analysis
A total of 50 mg of each intestinal content sample was accurately weighed, and the metabolites were extracted using a 400 µL methanol: water (4:1, v/v) solution. The mixture was allowed to settle at − 20 °C and treated by high throughput tissue crusher Wonbio-96c (Shanghai Wanbo Biotechnology Co., Ltd) at 50 Hz for 6 min, followed by vortexing for 30 s and ultrasound at 40 kHz for 30 min at 5 °C. The samples were placed at − 20 °C for 30 min to allow the proteins to precipitate. After centrifugation at 13,000g at 4 °C for 15 min, the supernatant was carefully transferred to sample vials with 20 μL of 2-chloro-l-phenylalanine (0.3 mg/mL) for LC–MS/MS analysis. The Ultra Performance Liquid Chromatography (UPLC) system was coupled to a quadrupole-time-of-flight mass spectrometer (Triple TOFTM 5600+, AB Sciex, USA) equipped with an electrospray ionization (ESI) source operating in positive and negative mode. To monitor the stability of the analysis, quality control was prepared by two different methods. One is to extract and mix the same volume of each sample, the other is to set internal standard (2-chloro-l-phenylalanine). In the process of instrument analysis, a mixed sample was inserted every 8–10 samples. A relative standard deviation (RSD) of Internal standard < 30%, which represents the stability and repeatability of the system.
Data processing and metabolite annotation
Peak detection and alignment of the raw data were performed with Progenesis QI 2.3 (Nonlinear Dynamics, Waters, USA), which generated a data matrix that included retention time (RT), mass-to-charge ratio (m/z) and peak intensity. The features detected in at least 50% of the samples were retained. After filtering out the low-coverage features, missing value was imputed according to the value in other samples by using the k-nearest neighbor (KNN) approach with the R DMwR package. Metabolic features with a relative standard deviation (RSD) of QC > 30% were discarded. The metabolic features value were normalized with a log10 transformation to better approximate a normal distribution. Mass spectra of the metabolic features were identified using the accurate mass, MS/MS fragment spectra and isotope ratio difference with searching in reliable biochemical databases such as the Human metabolome database (HMDB) (http://www.hmdb.ca/)31. Concretely, the mass tolerance between the measured m/z values and the exact mass of the components of interest was ± 10 ppm. For metabolites having MS/MS confirmation, only the ones with MS/MS fragments score above 30 were considered as confidently identified. Metabolome analysis data have been deposited to the Metabolights public repository under accession number MTBLS2512.
Statistical analysis
Principal coordinate analysis (PCoA) was carried out using the vegan package of R. For the initial screening of features, an analysis of variance (ANOVA) was performed using the R base package. The metabolites that were significantly different between the two groups were selected (P < 0.1). Then, we screened these metabolites according to a threshold variable importance in projection (VIP) value (VIP > 1) from the orthogonal partial least-square discriminant analysis (OPLS-DA) model. OPLS-DA was performed using the R package ropls32. The OPLS-DA model quality was assessed using goodness of fit (R2) and goodness of prediction (Q2) in cross-validation via a permutation test with 200 permutations. Student's t-test was performed to assess the significance of differences in the abundance of metabolites (P = 0.05). Metabolite pathway analysis (MetPA) of all metabolites selected for each intestine location was performed with MetaboAnalyst 4.0 (www.metaboanalyst.ca)33. The metabolic pathways with P-value < 0.05 were considered significantly enriched.
References
Patience, J. F., Rossoni-Serão, M. C. & Gutiérrez, N. A. A review of feed efficiency in swine: biology and application. J. Anim. Sci. Biotechnol. 6, 33 (2015).
Case, L. A., Wood, B. J. & Miller, S. P. The genetic parameters of feed efficiency and its component traits in the turkey (Meleagris gallopavo). Genet. Sel. Evol. 44, 2 (2012).
Koch, R. M., Swiger, L. A., Chambers, D. & Gregory, K. E. Efficiency of feed use in beef cattle. J. Anim. Sci. 22, 486–494 (1963).
Zhang, X. et al. Novel transgenic pigs with enhanced growth and reduced environmental impact. Elife 7, e34286 (2018).
Quan, J. et al. Metagenomic characterization of intestinal regions in pigs with contrasting feed efficiency. Front. Microbiol. 11, 32 (2020).
Carmelo, V. A. O., Banerjee, P., da Silva Diniz, W. J. & Kadarmideen, H. N. Metabolomic networks and pathways associated with feed efficiency and related-traits in Duroc and Landrace pigs. Sci. Rep. 10, 1–14 (2020).
Siegerstetter, S.-C. et al. Feed restriction modulates the fecal microbiota composition, nutrient retention, and feed efficiency in chickens divergent in residual feed intake. Front. Microbiol. 9, 2698 (2018).
Nagpal, R. & Yadav, H. Bacterial translocation from the gut to the distant organs: an overview. Ann. Nutr. Metab. 71, 11–16 (2017).
Quan, J. et al. A global comparison of the microbiome compositions of three gut locations in commercial pigs with extreme feed conversion ratios. Sci. Rep. 8, 4536. https://doi.org/10.1038/s41598-018-22692-0 (2018).
Wakita, Y. et al. Analysis of free radical production capacity in mouse faeces and its possible application in evaluating the intestinal environment: a pilot study. Sci. Rep. 9, 1–9 (2019).
Liu, X. & Locasale, J. W. Metabolomics: a primer. Trends Biochem. Sci. 42, 274–284. https://doi.org/10.1016/j.tibs.2017.01.004 (2017).
Dunn, W. B., Broadhurst, D. I., Atherton, H. J., Goodacre, R. & Griffin, J. L. Systems level studies of mammalian metabolomes: the roles of mass spectrometry and nuclear magnetic resonance spectroscopy. Chem. Soc. Rev. 40, 387–426 (2011).
Zou, S. et al. Identification of key taste components in loquat using widely targeted metabolomics. Food Chem. 323, 126822 (2020).
Artegoitia, V. M., Foote, A. P., Lewis, R. M. & Freetly, H. C. Rumen fluid metabolomics analysis associated with feed efficiency on crossbred steers. Sci. Rep. 7, 1–14 (2017).
Ramayo-Caldas, Y. et al. Integrative approach using liver and duodenum RNA-Seq data identifies candidate genes and pathways associated with feed efficiency in pigs. Sci. Rep. 8, 1–11 (2018).
Schiavon, S. et al. Effects of feed allowance and indispensable amino acid reduction on feed intake, growth performance and carcass characteristics of growing pigs. PLoS ONE 13, e0195645 (2018).
Thacker, P., Campbell, G. & GrootWassink, J. The effect of organic acids and enzyme supplementation on the performance of pigs fed barley-based diets. Can. J. Anim. Sci. 72, 395–402 (1992).
Vibha, J., Choudhary, K., Singh, M., Rathore, M. & Shekhawat, N. A study on pharmacokinetics and therapeutic efficacy of Glycyrrhiza glabra: a miracle medicinal herb. Bot. Res. Int. 2, 157–163 (2009).
Radhika, S., Senthilkumar, R. & Arumugam, P. A review on ethnic florae with antihyperglycemic efficacy. Int. J. Herbal Med. 1, 55–62 (2013).
Hu, R. et al. Extracts of Ganoderma lucidum attenuate lipid metabolism and modulate gut microbiota in high-fat diet fed rats. J. Funct. Foods 46, 403–412 (2018).
Guang, C., Chen, J., Sang, S. & Cheng, S. Biological functionality of soyasaponins and soyasapogenols. J. Agric. Food Chem. 62, 8247–8255 (2014).
Chiang, J. Y. Bile acids: regulation of synthesis. J. Lipid Res. 50, 1955–1966 (2009).
Braithwaite, V. S. et al. The effect of vitamin D supplementation on hepcidin, iron status, and inflammation in pregnant women in the United Kingdom. Nutrients 11, 190 (2019).
Jones, M. L., Martoni, C. J. & Prakash, S. Oral supplementation with probiotic L. reuteri NCIMB 30242 increases mean circulating 25-hydroxyvitamin D: a post hoc analysis of a randomized controlled trial. J. Clin. Endocrinol. Metab. 98, 2944–2951 (2013).
Macfarlane, G., Cummings, J. & Allison, C. Protein degradation by human intestinal bacteria. Microbiology 132, 1647–1656 (1986).
Chand, N., Muhammad, S., Khan, R. U., Alhidary, I. A. & ur Rehman, Z. Ameliorative effect of synthetic γ-aminobutyric acid (GABA) on performance traits, antioxidant status and immune response in broiler exposed to cyclic heat stress. Environ. Sci. Pollut. Res. 23, 23930–23935 (2016).
Gutiérrez, M. C. & Delgado-Coello, B. A. Influence of pipecolic acid on the release and uptake of [3 H] GABA from brain slices of mouse cerebral cortex. Neurochem. Res. 14, 405–408 (1989).
Callery, P. S. & Geelhaar, L. A. 1-Piperideine as an in vivo precursor of the γ-aminobutyric acid homologue 5-aminopentanoic acid. J. Neurochem. 45, 946–948 (1985).
Nanthirudjanar, T. et al. Gut microbial fatty acid metabolites reduce triacylglycerol levels in hepatocytes. Lipids 50, 1093–1102 (2015).
Cai, W., Casey, D. & Dekkers, J. Selection response and genetic parameters for residual feed intake in Yorkshire swine. J. Anim. Sci. 86, 287–298 (2008).
Wishart, D. S. et al. HMDB 4.0: the human metabolome database for 2018. Nucleic Acids Res. 46, D608–D617 (2018).
Thévenot, E. A., Roux, A., Xu, Y., Ezan, E. & Junot, C. Analysis of the human adult urinary metabolome variations with age, body mass index, and gender by implementing a comprehensive workflow for univariate and OPLS statistical analyses. J. Proteome Res. 14, 3322–3335 (2015).
Chong, J. et al. MetaboAnalyst 4.0: towards more transparent and integrative metabolomics analysis. Nucleic Acids Res. 46, W486–W494 (2018).
Acknowledgements
Thanks to Guangdong Wen's Foodstuffs Group for providing laboratory animals and phenotype records.
Funding
This study was financially supported by the Guangdong YangFan Innovative and Entrepreneurial Research Team Program (2016YT03H062), the Natural Science Foundation of Guangdong Province (2018B030315007 and 2018B030313011).
Author information
Authors and Affiliations
Contributions
J.Y. and Z.W. conceived and designed the experiments. Y.Y., J.W., J.Q., R.D., M.Y., X.W. collected the samples and recorded the phenotypes. R.D. calculated the FCR and RFI of experimental pigs. Y.Y. and J.W. data analyzed. Y.Y., J.Y., and Z.W. wrote and revised the manuscript. All authors reviewed and approved the manuscript.
Corresponding authors
Ethics declarations
Competing interests
The authors declare no competing interests.
Additional information
Publisher's note
Springer Nature remains neutral with regard to jurisdictional claims in published maps and institutional affiliations.
Supplementary Information
Rights and permissions
Open Access This article is licensed under a Creative Commons Attribution 4.0 International License, which permits use, sharing, adaptation, distribution and reproduction in any medium or format, as long as you give appropriate credit to the original author(s) and the source, provide a link to the Creative Commons licence, and indicate if changes were made. The images or other third party material in this article are included in the article's Creative Commons licence, unless indicated otherwise in a credit line to the material. If material is not included in the article's Creative Commons licence and your intended use is not permitted by statutory regulation or exceeds the permitted use, you will need to obtain permission directly from the copyright holder. To view a copy of this licence, visit http://creativecommons.org/licenses/by/4.0/.
About this article
Cite this article
Ye, Y., Wu, J., Quan, J. et al. Lipids and organic acids in three gut locations affect feed efficiency of commercial pigs as revealed by LC–MS-based metabolomics. Sci Rep 11, 7746 (2021). https://doi.org/10.1038/s41598-021-87322-8
Received:
Accepted:
Published:
DOI: https://doi.org/10.1038/s41598-021-87322-8
This article is cited by
Comments
By submitting a comment you agree to abide by our Terms and Community Guidelines. If you find something abusive or that does not comply with our terms or guidelines please flag it as inappropriate.