Abstract
Whole genome sequencing (WGS) can elucidate Mycobacterium tuberculosis (Mtb) transmission patterns but more data is needed to guide its use in high-burden settings. In a household-based TB transmissibility study in Peru, we identified a large MIRU-VNTR Mtb cluster (148 isolates) with a range of resistance phenotypes, and studied host and bacterial factors contributing to its spread. WGS was performed on 61 of the 148 isolates. We compared transmission link inference using epidemiological or genomic data and estimated the dates of emergence of the cluster and antimicrobial drug resistance (DR) acquisition events by generating a time-calibrated phylogeny. Using a set of 12,032 public Mtb genomes, we determined bacterial factors characterizing this cluster and under positive selection in other Mtb lineages. Four of the 61 isolates were distantly related and the remaining 57 isolates diverged ca. 1968 (95%HPD: 1945–1985). Isoniazid resistance arose once and rifampin resistance emerged subsequently at least three times. Emergence of other DR types occurred as recently as within the last year of sampling. We identified five cluster-defining SNPs potentially contributing to transmissibility. In conclusion, clusters (as defined by MIRU-VNTR typing) may be circulating for decades in a high-burden setting. WGS allows for an enhanced understanding of transmission, drug resistance, and bacterial fitness factors.
Similar content being viewed by others
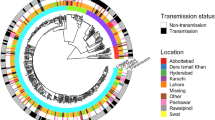
Introduction
Tuberculosis (TB) remains among the top ten causes of deaths globally, with 10.4 million new cases in 2016 alone1. Peru remains a high burden country for multidrug-resistant (MDR) TB with 117 TB cases reported per 100,000 population in 2016 and approximately 9% being MDR or rifampicin-resistant (RR)1. Molecular methods have been instrumental in identifying outbreaks, and single nucleotide polymorphism (SNPs) identified using whole genome sequencing (WGS) have a higher resolution in identifying transmission links compared to traditional genotyping methods such as spoligotyping or Mycobacterium interspersed repetitive unit-variable number tandem repeats (MIRU-VNTR)2,3,4,5,6,7,8,9,10,11,12. Yet we don’t yet fully understand how to use all the genetic information generated by WGS. By convention, as much as 10% of the genome is excluded2,3 and in some instances too little remaining variation is found to enable the resolution of transmission chains13. Resolving transmission events accurately is particularly challenging in high-burden settings where multiple source case suspects are common. In addition to guiding public health interventions including appropriate contact tracing, identifying the source case can inform patient care in some cases, such as in pediatric TB where the source case microbiological data can inform treatment14,15. Furthermore, in high-burden countries the term ‘outbreak’ may not apply as TB has been circulating continuously for decades16. Given the renewed emphasis on active case finding17 and the widespread adoption of WGS, an intensification of the latter in high-burden settings is needed.
Control efforts against TB have been undermined by the emergence and spread of drug resistant TB (DR-TB). Current evidence suggests that most cases of DR-TB are a result of transmission rather than de-novo evolution of the bacteria during treatment18,19,20. Factors known to contribute to DR-TB transmission include delays in diagnosis and treatment21, host factors (e.g. age, immune status22,23,24), as well as bacterial factors such as fitness and immunogenicity characteristics25,26,27. It is well recognized that Mycobacterium tuberculosis (MTB) strains with the same DR-conferring mutations have a range of fitness28,29,30. However, to date few molecular fitness determinants have been characterized and seldom in the context of high transmissibility31,32,33,34. Improved knowledge of such bacterial factors can inform efforts for transmission interruption by identifying targets for diagnosis, surveillance, and even potential therapeutics targeting fitness mechanisms. Here we use WGS data to examine the largest TB MIRU-VNTR cluster spanning pan-susceptible to MDR-TB isolates that was identified in 4,000 TB patients enrolled in a household transmissibility study. We examine both host data and TB genotypic data to understand the evolution of isolates within this cluster, infer the timing of emergence of antibiotic resistance, and identify genetic bacterial factors unique to this cluster that may have contributed to its success.
Materials and Methods
Study Design
A TB household transmissibility and treatment outcome study was performed in northern Lima, Peru from September 2009 to August 2012. The study procedures including patient enrollment and consent have been previously described35,36. Briefly, informed consent was obtained from participants or their parents or guardians. Patients were enrolled if they were diagnosed with pulmonary TB (PTB) at public health clinics and were followed through therapy. Their household contacts were also followed with tuberculin skin testing and monitored for development of TB for a period of 12 months. The following were collected at time of TB diagnosis: clinical signs and symptoms, sociodemographic characteristics (e.g. age, gender, occupation, household type), geographical coordinates of household and health center, co-morbidities (HIV status, diabetes mellitus, renal disorder), as well as alcohol, tobacco and drug use.
Approval was obtained from the Research Ethics Committee of the Peruvian National Institute of Health (Lima, Peru) and the Committee on Human Studies at Harvard Medical School (Boston, MA). All research was performed in accordance with relevant guidelines and regulations.
Culture, DST and genotyping
Lowenstein-Jensen (LJ) culture was performed from sputum specimens using standard NALC-NaOH decontamination. All sputum cultures positive for MTB complex subspecies tuberculosis were subjected to first-line drug susceptibility testing (DST) for isoniazid (critical concentration of 1 µg/mL and 0.2 µg/mL), rifampicin (40 µg/mL), streptomycin (4 µg/mL) and ethambutol (2 µg/mL) using the proportion method. Pyrazinamide susceptibility (100 µg/mL) was measured using the Wayne method37. Any resistant strains underwent further DST for second-line agents performed using the indirect proportion method on 7H11 agar as previously described38,39,40. DNA was extracted and genotyped by 24-loci MIRU-VNTR using standard methods41. A ‘cluster’ in the study was defined as a group of isolates having an identical MIRU-VNTR pattern.
Whole-genome sequencing (WGS) and variant calling
Sixty-three isolates collected between 2009 and 2012 were available for sequencing from the largest MIRU-VNTR cluster (n = 148) found in the study (Supplementary Table 3) based on the availability of culture for DNA extraction in 2013. DNA extraction, sequencing and read processing is described in the supplementary methods. Raw reads were processed and variants were called using a custom bioinformatics pipeline42 (supplementary methods).
Phylogenetic analysis
A multiple sequence alignment was generated as a concatenate of allelic states at all sites found to be variable using a custom script in Perl 5.10.142. We generated an alignment containing only substitutions and another with both substitutions and insertions and deletions (commonly referred to as ‘indels’). Variants in genes implicated in drug resistance43, transposases, and genes coding for proline-glutamate (PE) or proline-proline-glutamate (PPE)44 (commonly referred to as PE/PPEs) were excluded from phylogeny building by convention (e.g. due to high levels of recombination45), and also because drug resistance genes are under selective pressure and are expected to bias tree structure. A maximum likelihood phylogenetic tree was built for both alignments using RAxML 8.2.1146 as implemented in the R package ips47 (supplementary methods). Estimation of divergence times and timing of drug resistance acquisition was performed using BEAST 1.8.448,49 using an uncorrelated lognormal relaxed clock that allows for tree branches to evolve at different rates. The prior on the mean clock rate was assumed to be 0.5 SNP per genome per year based on published data3. We calculated the range of dates based on a 95% highest posterior density interval (HPDI) which indicates the smallest interval that includes 95% of the posterior probability distribution50. Please see supplementary methods for further details of the phylogenetic analysis.
To determine the number of times DR arose within the group of isolates, we examined the maximum clade credibility tree (where bifurcations denote internode branches supported by a posterior probability >0.5). We then counted the minimum number of paraphyletic switches from a sensitive to a resistant phenotype for each drug supported by bifurcating/high-confidence nodes.
SNPs occurring within a high-transmission cluster were further evaluated against other TB lineages using a large TB WGS database containing 12,032 strains curated from the literature through NCBI and the Reseq TB initiative51 (Supplementary Data 1). To determine SNPs with phylogenetic convergence, mutations in the high-transmission cluster that were present in more than 5% of strains in at least two other main lineages (i.e. lineages 1, 2 and 3) in addition to lineage 4 were identified as likely being under positive selection. As some of the hit SNPs occurred in repetitive regions, we confirmed their accuracy by remapping simulated reads from different reference genomes and using Pacific Biosciences (PacBio) long-read sequences (supplementary methods).
Other data analysis
Variation in PE/PPE regions and indels between closely related strains was confirmed via visualization and checked for false positives due to copy number variants (supplementary methods). To study host-related factors that may be associated with transmission, the propensity to propagate (PTP) method was used as previously described52.
Results
Patient and isolate characteristics
A large cluster of 148 isolates, collected from 147 patients, with identical MIRU-VNTR pattern was identified. The majority of the patients in this cluster were male, HIV-negative and had multidrug resistant (MDR) TB (Table 1). About one-half were smear-positive (51.4%) and one-third used alcohol (35.8%) or other intoxicating substances (27.9%). From this cluster, 63 isolates were available for sequencing (supplementary methods). Two patients had isolates that did not meet our sequencing quality criteria and were subsequently excluded. Of those patients with high quality sequence data (n = 61) a higher majority were male with MDR-TB but were otherwise comparable to the superset of 148 (Table 1). Sequencing data revealed that 58 isolates belonged to the Latin America-Mediterranean LAM-4.3.3 sublineage, and three isolates were more distant and belonged to the sublineages X -4.1.1, T-4.8, and LAM-4.3.2. In the LAM-4.3.3 group, we found 371 SNPs and 81 indels in total. Of these, 24 substitutions and one indel occurred in DR conferring regions, and 42 substitutions and 23 indels in PE/PPE genes. With the exclusion of DR conferring regions, the average pairwise SNP difference between isolates was 21.69 (range: 0–84) and 22.7 (range: 1–100), excluding and including the PE/PPE regions, respectively.
The geographic distribution of strains based on household coordinates, colored by resistance pattern is shown in Fig. 1. Comparison between genetic and geographic distance did not support that the cluster spread in a single geographic direction, even when three most distant strains were excluded (P = 0.2, Supplementary Fig. 1).
Geographic location of strains with resistance pattern. (A) All 148 strains with identical MIRU-VNTR patterns, (B) 61 strains that were sequenced. Maps generated using the plot() function as implemented in R package OutbreakTools70. MDR: multidrug-resistant (resistant to both isoniazid and rifampin).
The SNP-based phylogenetic tree (Fig. 2, Supplementary Fig. 2) demonstrated that the most genetically homogeneous group consisted of 57 isolates. These formed two main clades, where the first contained isolates that were pan-susceptible or streptomycin mono-resistant, and the second consisted of INH mono-resistant or MDR isolates. Using a prior on the mean clock rate of 0.5 SNP per genome per year3 (supplementary methods), the origin of the 61 isolates was estimated around the middle of the 14th century (1336 CE; 95%HPD 855–1680). The LAM-4.3 cluster of 58 isolates diverged ca. 1923 (95%HPD 1856–1967) and innermost cluster of 57 isolates diverged ca. 1968 (95%HPD 1945–1985).
Bayesian maximum clade credibility phylogenetic tree created via BEAST (using single nucleotide polymorphisms) of 61 strains with nodes in increasing order of age. Numbers at nodes are posterior means of node ages (years ago). Node ages <15 years are not shown for clarity. Bars represent 95%HPD interval for node age. Color of tip represents drug susceptibility - Green: pan-susceptible, Dark Red: Resistant only to Isoniazid or Rifampicin, Dark Green: Resistant to a drug other than Isoniazid or Rifampicin, Red: multi-drug resistant.
Epidemiological vs. genotypic data
We compared the use of genetic and epidemiological data for transmission inference. PE/PPE region differences and indels between closely related pairs were visualized in Integrated Genome Viewer and confirmed to be high confidence (supplementary methods). Sequencing data was available for three of seven household contact pairs in the cluster of 147 patients. Only one household link (index/parent M23 – contact/child M12) was consistent with a recent transmission event on the tree and by genetic distance (SNP difference = 1). When high-confidence PE/PPE regions were included, the genetic distance between this pair increased to 3 variants. With the further addition of high confidence indels the pairwise distance increased to 4, in the predicted 5 year interval (Supplementary Data 2). No variation was observed in this pair in DR-related loci. The other two isolate pairs from household contacts (index/parent M02 – contact/child M01, index/child M45 – contact/parent M58) were 17 and 421 SNPs apart, respectively. Isolates M01 and M02 were genetically closer to other isolates on the tree (M01-M28 6 SNPs apart and M02-M42-M11-M37 all within 4 SNPs of each other). A pair of isolates collected two months apart from a host who had not been on treatment (MDR strains M06 and M10) was found to have no SNP differences outside of PE/PPE regions. In PE/PPE regions, we found 5 high-quality SNPs; similarly, 2 indels were observed in other regions.
Looking at genetic evidence alone for recent transmission using a distance cutoff of ≤5 SNPs (excluding DR and PE/PPE regions)3, 139 links among 38 patients were identified (Fig. 3). Other than one pair (index/parent M23 and contact/child M12), none of these belonged to the same household. With the addition of high confidence indels and PE/PPE SNPs we used a cutoff of ≤12 variants: 5 SNPs plus 7 PE/PPE and indel variants based on the two serial isolates available from the same patient described above. Using this added variation and the cutoff of ≤12, there were 104 links among 38 patients, i.e. 25% fewer links than when these variants were excluded. Phylogenetic trees built by including indel variation also had notable differences within the cluster of 57 isolates (Supplementary Fig. 3).
Inferred transmission network based on genomic distance. Shown is a network graph depicting strains that were less than or equal to 5 single nucleotide polymorphisms (SNPs) apart (with the exclusion of PE/PPE regions and regions coding for drug resistance). Each vertex represents a strain or group of strains, edges are colored to denote number of SNP differences between the connected vertices. IG = Identical Group – consists of strains that had zero SNP difference, IG1 = M09, M14, M01; IG2 = M06, M10; IG3 = M04, M07; IG4 = M18, M28. Legend shows number of different SNPs and corresponding color of the edges. Plot generated using R package igraph.
Of the 375 isolates sequenced from a TB outbreak in London13, 325 (86.67%) met our quality criteria and were further examined. Using a genetic distance cut-off of ≤5 SNPs (excluding DR and PE/PPE regions), 309 of these isolates diversified into one large interconnected cluster consisting of 31,776 links. Among 38 serial isolates that were collected from 19 patients from that outbreak, the largest difference between a pair with the inclusion of indels and PE/PPE regions was also found to be 7 SNPs. When applying this as the threshold for identifying a genetic link, the interconnected cluster was reduced to 294 strains with 28,230 links, i.e. 11% fewer links than when PE/PPE variants and indels were excluded.
Host factors
We measured host infectiousness in the cluster using the ‘propensity to propagate’ (PTP) method52 and identified five patients as having the highest possible score (PTP > 4). This was related to patients being younger (20–29 years old) males with smear positive PTB and a history of substance use (Supplementary Fig. 4). Three of these were identified to be within the network of patients with genetically close MDR isolates (Fig. 3). The mean cluster PTP was also high at 1.699.
Drug resistance
First and second-line drug resistance was acquired several times within the core cluster of 57 isolates (Table 2, Supplementary Fig. 5, and Supplementary Table 1).
First line drugs
Isoniazid (INH) resistance was acquired at least twice, and in both times with a katG S315T mutation, ca. 1968 (95%HPD: 1945–1985) and ca. 1972 (95%HPD 1952–1985). Rifampicin resistance (RR) was acquired within the large INH-resistant clade at least twice ca. 1980 (95%HPD: 1964–1990, rpoB D435V) and ca. 1986 (95%HPD: 1973–1996, most frequent variant rpoB S450L). Pyrazinamide (PZA) resistance followed RR and was acquired at least 4 times, the most frequent mutation was pncA Q10R acquired ca. 1980 (95%HPD: 1964–1990), the most recent PZA resistance acquisition event was predicted to be within the last year of isolation (95%HPD: 2006–2012). Ethambutol resistance was acquired at least twice within the MDR clade and contemporaneous with RR acquisition in both cases. The mutation Y319S was the most common embB mutation observed.
Second line drugs
Of the 25 strains tested for ciprofloxacin, one MDR isolate (M43) acquired resistance ca. 1996 (95%HPD: 1979–2009). Similarly, only one (M30, which was also resistant to capreomycin) of the 41 isolates tested for kanamycin acquired resistance ca. 2009 (95%HPD: 2006–2012). We were not able to identify any mutations in gyr or rrs to explain resistance to these two drugs. For capreomycin, seven of forty tested isolates were resistant and carried the tlyA G232D mutation estimated to have been acquired ca. 2005 (95%HPD: 2001–2008).
Resistance and Bacterial Fitness
As there were several isolates measured to be resistant by the culture-based method that did not harbor any known resistance mutations, e.g. for EMB and PZA, we attempted a phylogeny-based genome wide association within the group of 61 isolates to identify new mutations associated with resistance (Supplementary Table 2). In addition to identifying the known mutations that confer resistance to isoniazid and rifampicin, we found an association between EMB resistance and a mutation (3778221AG) in the intergenic region between spoU and PE-PGRS51 genes (20 bp from spoU end and 347 bp before PE-PGRS51 start), corresponding to the acquisition of EMB resistance ca. 1980 (95%HPD: 1964-1990) shown in Supplementary Fig. 5.
We identified 175 mutations that were unique to the core cluster of 57 isolates and were absent from the four more distantly related isolates. Hypothesizing that a subset of these mutations may have contributed to transmissibility of this cluster, we measured which are under positive selection by looking at 12,032 other MTB isolates. Five mutations met our criteria for positive selection i.e. were found to have a frequency of >5% in at least three other TB lineages (lineage 1, 2, 3, and non-LAM-4) (Fig. 4). Of the five, two occurred in genes with known function, esxV which is an ESAT-6 like secreted protein and cobD, a cobalamin biosynthesis protein. As three of the five genes are known to contain repetitive regions, the accuracy of the convergent mutation calls was verified by simulating and remapping Illumina reads carrying the variant, and using PacBio long read sequences that was available for one strain (supplementary methods).
Discussion
In this detailed analysis of a MIRU-VNTR cluster with variable degrees of drug resistance from a high prevalence setting, we show that traditional genotyping methods have a significantly lower resolution in identifying transmission clusters as compared to WGS, particularly when variation in PE/PPE regions, indels are incorporated into the analysis. Additionally, we found complex evolutionary patterns within an otherwise identical MIRU cluster and identified the interplay of host and epidemiological factors contributing to transmission potential of a cluster.
The higher resolution gained by WGS is consistent with prior reports2,3,53,54,55, but the maximum genetic distance we find between the clustered isolates is larger than previously seen and we further estimate the group of LAM-4.3.3 sequenced isolates to have been circulating for over 8 decades in our study community. Previous studies performing WGS of MIRU-VNTR clusters in low prevalence settings have noted shorter genetic distance between isolates2,3,13, and in one case the distances were insufficient to reliably and consistently inform contact tracing interventions13. It is possible, that certain features of our selected cluster have led to the observation of such high levels of diversity. First, our cluster spans the spectrum of pan-susceptible to resistant against seven drugs. Second, epidemiological links were known for only three pairs of patients in the cluster. Third, our isolates all belong to lineage 4, a lineage that has been noted to be the most phenotypically and genotypically diverse of the TB lineages56. However, the proportion of diversity that could be linked directly to drug resistance was low. A parsimonious explanation of the high degree of observed genomic diversity is that the rate of MIRU-VNTR pattern evolution is on average slow and on the order of decades. Despite this, MIRU-VNTR likely offers sufficient resolution in low prevalence settings as most TB cases there tend to be imported2,3.
The genes in the PE and PPE families constitute about 10% of the TB genome and have been grouped together based on the proline-glutamate (PE) and proline-proline-glutamate (PPE) signature motifs but members of this family are scattered throughout the genome and have diverse functions57,58. Because they carry a high GC content and contain repetitive areas they have been typically excluded from analysis of sequencing data59. However, recent advances in sequencing technology allow for longer read lengths and increased throughput, which when combined with more accurate bioinformatics pipelines, makes it possible to call variants in a proportion of these genes with high confidence. We identified several high quality indels and variation in the PE/PPE regions in our dataset. The commonly used cutoff of ≤5 SNPs to infer transmission does not take into account the different evolutionary rate of these regions that may be driven by intra-genomic recombination or other mechanisms. In our study, when identifying closely related strains with the inclusion of high confidence PE/PPE regions, the number of possible links between strains decreased. An accepted standard that accounts for variation in these regions would allow for improved resolution of transmission events. Although, comparison of ancestral relationships in the phylogenetic trees with and without the inclusion of indels did not show significant differences, there were notable differences within the closely related cluster, highlighting that similarity measures that rely on SNPs alone could be misleading. Inclusion of indels and PE/PPE regions in the estimation of divergence dates is limited by our current lack of knowledge regarding their evolutionary rates or clock-like behavior. Prior studies support that at least a proportion of them accumulate variation in a manner consistent with lineage44 and coupled with our observation that these regions account for an appreciable proportion of variation seen between closely related isolates, including PE/PPE variants is likely to inform transmission inference.
We identified many genomic links using the SNP distance threshold of ≤5 criterion3 that were not discovered within household contact investigation, providing evidence that household contact investigation is not sufficient to identify and treat secondary TB cases as transmission can occur anywhere in the community60,61. Additionally, 2 of 3 case pairs that belonged to the same household were found to have large genetic distances making it more likely that transmission occurred outside the household. Although the dataset used was relatively small, these findings add to the current limited literature on the topic60,62,63. Overall our study highlights the utility of WGS in resolving transmission links particularly in high burden settings where several transmission chains may occur simultaneously. WGS of a well characterized cluster through MIRU-VNTR led to identification of several sub-clusters with further granularity achieved from addition of variants in regions that are routinely excluded from these analyses. With decreasing cost of WGS, sequencing data could be integrated with epidemiological investigation in lieu of traditional fingerprinting methods to identify transmission clusters and for reconstruction of contact networks, particularly given the increasing emphasis on active case finding for TB elimination17.
Our phylogenetic dating procedures support the conclusion that the acquisition of MDR is not recent in Lima, and that MDR cases, given the observed phylogenetic structure, are mostly related to transmission. This finding is consistent with other studies carried out in other countries, e.g. South Africa18. Within the MDR subcluster, ethambutol and pyrazinamide resistance was acquired and transmitted. This finding is similar to that of a study in Uganda64, where pyrazinamide resistance typically arose in MDR strains with several different causative mutations. This highlights the importance of testing for pyrazinamide resistance in order to determine benefit of its use in MDR-TB treatment regimens65.
Phenotypic resistance could not be explained by genotype in a few isolates including in our study. To this effect, we undertook a GWAS procedure to identify drug resistant phenotype-genotype associations. We identified the intergenic SNP 3778221AG 30 bp downstream of the putative tRNA/rRNA methylase gene spoU to be significantly associated with ethambutol resistance. Although a causative mechanism for how this variant modulates EMB susceptibility or fitness is not clear, this finding is supported further by a recent large genome-wide association study of 1452 MTB isolates66.
The cluster under study was the largest such cluster observed in the Lima household transmission study. Its transmission success was likely due to both bacterial and host factors. We quantified the host predilection to transmit TB with the PTP measure and found the cluster to have a higher score than the median PTP measure reported by a study in Netherlands52. Our study had five patients with a particularly high PTP above the highest reported value of 3·952, potentially contributing to transmission in the population we studied. A few prior studies have characterized bacterial genetic factors that contributed to increased transmissibility24,67,68. We add to this literature by identifying five cluster defining SNPs to be under positive selection in a large TB genomic dataset. One of these SNPs (esxV S23L) is a member of the ESAT-6 family of secreted proteins, some of which have been shown to be involved in host-pathogen interactions and may thus have contributed to increased transmissibility68,69.
Our study had several limitations. Contact tracing was done within household contacts and hence epidemiological links in the community were possibly missed. Sequencing a subset of isolates from the cluster may have led to missed links along the transmission chain. It may also have led to an underestimation of the diversity. However, the sampled subset demonstrated a substantial amount of diversity, more than would be expected within a cluster with identical MIRU pattern2,3. We also cannot exclude that the 2 outer most isolates were mis-assigned the reported MIRU pattern and because of this we focused on the isolates confirmed to be of the same lineage by in silico spoligotyping and the WGS SNP barcode. Finally, it is important to note that our dating estimates are heavily reliant on the molecular clock rate that has been previously reported in the literature.
In summary, our findings add to the evidence challenging the traditional interpretation of a MIRU-VNTR cluster as indicating recent transmission and suggest that the benefits of WGS over MIRU-VNTR may be even more prominent in high prevalence settings when TB transmission has been ongoing without interruption, especially when high confidence PE/PPE and indel genetic variants are included. WGS can also provide insights into biology of MTB to improve our understanding of DR, transmission and host-pathogen interaction.
Data Availability
Mycobacterium tuberculosis genome data were deposited in the NCBI BioProject database (ID: PRJNA343736 and PRJNA526078). Individual accession numbers for genomes analyzed in this study are given in Supplementary Data 3.
References
World Health Organization Global tuberculosis report 2017. Geneva, Switzerland (2017).
Gardy, J. L. et al. Whole-genome sequencing and social-network analysis of a tuberculosis outbreak. N. Engl. J. Med. 364, 730–739 (2011).
Walker, T. M. et al. Whole-genome sequencing to delineate Mycobacterium tuberculosis outbreaks: a retrospective observational study. Lancet Infect. Dis. 13, 137–146 (2013).
Roetzer, A. et al. Whole Genome Sequencing versus Traditional Genotyping for Investigation of a Mycobacterium tuberculosis Outbreak: A Longitudinal Molecular Epidemiological Study. PLOS Med. 10, e1001387 (2013).
Kohl, T. A. et al. Whole-genome-based Mycobacterium tuberculosis surveillance: a standardized, portable, and expandable approach. J. Clin. Microbiol. 52, 2479–2486 (2014).
Gurjav, U. et al. Whole Genome Sequencing Demonstrates Limited Transmission within Identified Mycobacterium tuberculosis Clusters in New South Wales, Australia. PloS One. 11, e0163612 (2016).
Outhred, A. C. et al. Identifying Likely Transmission Pathways within a 10-Year Community Outbreak of Tuberculosis by High-Depth Whole Genome Sequencing. PloS One. 11, e0150550 (2016).
Bryant, J. M. et al. Whole-genome sequencing to establish relapse or re-infection with Mycobacterium tuberculosis: a retrospective observational study. Lancet Respir. Med. 1, 786–792 (2013).
Luo, T. et al. Whole-genome sequencing to detect recent transmission of Mycobacterium tuberculosis in settings with a high burden of tuberculosis. Tuberc. Edinb. Scotl. 94, 434–440 (2014).
Guerra-Assunção, J. A. et al. Large-scale whole genome sequencing of M. tuberculosis provides insights into transmission in a high prevalence area. eLife. 4 (2015).
Clark, T. G. et al. Elucidating emergence and transmission of multidrug-resistant tuberculosis in treatment experienced patients by whole genome sequencing. PloS One. 8, e83012 (2013).
Ali, A. et al. Whole genome sequencing based characterization of extensively drug-resistant Mycobacterium tuberculosis isolates from Pakistan. PloS One. 10, e0117771 (2015).
Casali, N. et al. Whole Genome Sequence Analysis of a Large Isoniazid-Resistant Tuberculosis Outbreak in London: A Retrospective Observational Study. PLOS Med. 13, e1002137 (2016).
Management of drug-resistant TB in children in Guidance for National Tuberculosis Programmes on the Management of Tuberculosis in Children (World Health Organization, 2014).
Devi, N. P. P. G. & Swaminathan, S. Drug-Resistant Tuberculosis: Pediatric Guidelines. Curr. Infect. Dis. Rep. 15, 356–363 (2013).
Eldholm, V. et al. Four decades of transmission of a multidrug-resistant Mycobacterium tuberculosis outbreak strain. Nat. Commun. 6, 7119 (2015).
Bloom, B. R. A Neglected Epidemic. N. Engl. J. Med. 378, 291–293 (2018).
Shah, N. S. et al. Transmission of Extensively Drug-Resistant Tuberculosis in South Africa. N. Engl. J. Med. 376, 243–253 (2017).
Dowdy, D. W., Theron, G., Tornheim, J. A. & Kendall, E. A. Drug-resistant tuberculosis in 2017: at a crossroads. Lancet Respir. Med. 5, 241–242 (2017).
Dheda, K. et al. Outcomes, infectiousness, and transmission dynamics of patients with extensively drug-resistant tuberculosis and home-discharged patients with programmatically incurable tuberculosis: a prospective cohort study. Lancet Respir. Med. 5, 269–281 (2017).
WHO policy recommendations: C. The effect of delay in starting treatment on treatment outcomes for patients with drug-resistant TB in WHO Treatment Guidelines for Drug-Resistant Tuberculosis, 2016 Update (World Health Organization, 2016).
Schaaf, H. S. et al. Transmission of multidrug-resistant tuberculosis. Pediatr. Infect. Dis. J. 19, 695–699 (2000).
Borgdorff, M. W. et al. Progress towards tuberculosis elimination: secular trend, immigration and transmission. Eur. Respir. J. 36, 339–347 (2010).
Li, W.-B. et al. Factors associated with primary transmission of multidrug-resistant tuberculosis compared with healthy controls in Henan Province, China. Infect. Dis. Poverty. 4, 14 (2015).
Albanna, A. S. & Menzies, D. Drug-resistant tuberculosis: what are the treatment options? Drugs. 71, 815–825 (2011).
Verhagen, L. M. et al. Mycobacterial factors relevant for transmission of tuberculosis. J. Infect. Dis. 203, 1249–1255 (2011).
Nebenzahl-Guimaraes, H. et al. Transmissible Mycobacterium tuberculosis Strains Share Genetic Markers and Immune Phenotypes. Am. J. Respir. Crit. Care Med. 195, 1519–1527 (2017).
Luciani, F., Sisson, S. A., Jiang, H., Francis, A. R. & Tanaka, M. M. The epidemiological fitness cost of drug resistance in Mycobacterium tuberculosis. Proc. Natl. Acad. Sci. 106, 14711–14715 (2009).
Salvatore, P. P. et al. Fitness Costs of Drug Resistance Mutations in Multidrug-Resistant Mycobacterium tuberculosis: A Household-Based Case-Control Study. J. Infect. Dis. 213, 149–155 (2016).
Morcillo, N. S. et al. Fitness of drug resistant Mycobacterium tuberculosis and the impact on the transmission among household contacts. Tuberc. Edinb. Scotl. 94, 672–677 (2014).
Comas, I. et al. Whole-genome sequencing of rifampicin-resistant Mycobacterium tuberculosis strains identifies compensatory mutations in RNA polymerase genes. Nat. Genet. 44, 106–110 (2012).
Farhat, M. R. et al. Genomic Analysis Identifies Targets of Convergent Positive Selection in Drug Resistant Mycobacterium tuberculosis. Nat. Genet. 45, 1183–1189 (2013).
Merker, M. et al. Compensatory evolution drives multidrug-resistant tuberculosis in Central Asia. eLife. 7, e38200 (2018).
Casali, N. et al. Evolution and transmission of drug-resistant tuberculosis in a Russian population. Nature Genetics 46(3), 279–286 (2014).
Huang, C.-C. et al. The Effect of HIV-Related Immunosuppression on the Risk of Tuberculosis Transmission to Household Contacts. Clin. Infect. Dis. Off. Publ. Infect. Dis. Soc. Am. 58, 765–774 (2014).
Velásquez, G. E. et al. Pyrazinamide Resistance Assays and Two-Month Sputum Culture Status in Patients with Multidrug-Resistant Tuberculosis. Antimicrob. Agents Chemother. 60, 6766–6773 (2016).
Calderón, R. I. et al. Prevalence of pyrazinamide resistance and Wayne assay performance analysis in a tuberculosis cohort in Lima, Peru. Int. J. Tuberc. Lung Dis. Off. J. Int. Union Tuberc. Lung Dis. 21, 894–901 (2017).
Kent, P. T. & Kubica, G. P. Public Health Mycobacteriology: A Guide for the Level III Laboratory. (1995).
Canetti, G., Rist, N. & Grosset, J. Measurement of sensitivity of the tuberculous bacillus to antibacillary drugs by the method of proportions. Methodology, resistance criteria, results and interpretation. Rev. Tuberc. Pneumol. (Paris). 27, 217–272 (1963).
Wayne, L. G. Simple pyrazinamidase and urease tests for routine identification of mycobacteria. Am. Rev. Respir. Dis. 109, 147–151 (1974).
Supply, P. et al. Proposal for Standardization of Optimized Mycobacterial Interspersed Repetitive Unit-Variable-Number Tandem Repeat Typing of Mycobacterium tuberculosis. J. Clin. Microbiol. 44, 4498–4510 (2006).
A wrapper pipe for variant calling and genome assembly for M.tuberculosis: github.com/farhat-lab/megapipe. (Farhat Laboratory, 2018).
Sandgren, A. et al. Tuberculosis Drug Resistance Mutation Database. PLoS Med. 6 (2009).
Phelan, J. E. et al. Recombination in pe/ppe genes contributes to genetic variation in Mycobacterium tuberculosis lineages. BMC Genomics. 17, 151 (2016).
Liu, X., Gutacker, M. M., Musser, J. M. & Fu, Y.-X. Evidence for recombination in Mycobacterium tuberculosis. J. Bacteriol. 188, 8169–8177 (2006).
Stamatakis, A. RAxML version 8: a tool for phylogenetic analysis and post-analysis of large phylogenies. Bioinformatics. 30, 1312–1313 (2014).
Heibl, C. PHYLOCH: R language tree plotting tools and interfaces to diverse phylogenetic software packages. (2008).
Drummond, A. J. & Rambaut, A. BEAST: Bayesian evolutionary analysis by sampling trees. BMC Evol. Biol. 7, 214 (2007).
Drummond, A. J., Suchard, M. A., Xie, D. & Rambaut, A. Bayesian Phylogenetics with BEAUti and the BEAST 1.7. Mol. Biol. Evol. 29, 1969–1973 (2012).
Drummond, A. J. & Bouckaert, R. R. Bayesian Evolutionary Analysis with BEAST. (Cambridge University Press, 2015).
Starks, A. M. et al. Collaborative Effort for a Centralized Worldwide Tuberculosis Relational Sequencing Data Platform. Clin. Infect. Dis. Off. Publ. Infect. Dis. Soc. Am. 61Suppl 3, S141–146 (2015).
Nebenzahl-Guimaraes, H., Borgdorff, M. W., Murray, M. B. & Van Soolingen, D. A novel approach - the propensity to propagate (PTP) method for controlling for host factors in studying the transmission of Mycobacterium tuberculosis. PloS One. 9, e97816 (2014).
Tyler, A. D. et al. Application of whole genome sequence analysis to the study of Mycobacterium tuberculosis in Nunavut, Canada. PloS One. 12, e0185656 (2017).
Fiebig, L. et al. A joint cross-border investigation of a cluster of multidrug-resistant tuberculosis in Austria, Romania and Germany in 2014 using classic, genotyping and whole genome sequencing methods: lessons learnt. Euro Surveill. Bull. Eur. Sur Mal. Transm. Eur. Commun. Dis. Bull. 22 (2017).
Norheim, G. et al. Tuberculosis Outbreak in an Educational Institution in Norway. J. Clin. Microbiol. 55, 1327–1333 (2017).
Stucki, D. et al. Mycobacterium tuberculosis lineage 4 comprises globally distributed and geographically restricted sublineages. Nat. Genet. 48, 1535–1543 (2016).
Musser, J. M., Amin, A. & Ramaswamy, S. Negligible Genetic Diversity of Mycobacterium tuberculosis Host Immune System Protein Targets: Evidence of Limited Selective Pressure. Genetics. 155, 7–16 (2000).
Copin, R. et al. Sequence Diversity in the pe_pgrs Genes of Mycobacterium tuberculosis Is Independent of Human T Cell Recognition. mBio. 5, e00960–13 (2014).
Galagan, J. E. Genomic insights into tuberculosis. Nat. Rev. Genet. 15, 307–320 (2014).
Mathema, B. et al. Drivers of Tuberculosis Transmission. J. Infect. Dis. 216, S644–S653 (2017).
Auld, S. C. et al. XDR tuberculosis in South Africa: genomic evidence supporting transmission in communities. Eur. Respir. J. 1800246, https://doi.org/10.1183/13993003.00246-2018 (2018).
Kompala, T., Shenoi, S. V. & Friedland, G. Transmission of tuberculosis in resource-limited settings. Curr. HIV/AIDS Rep. 10, 264–272 (2013).
Middelkoop, K. et al. Transmission of Tuberculosis in a South African Community With a High Prevalence of HIV Infection. J. Infect. Dis. 211, 53–61 (2015).
Ssengooba, W. et al. Whole genome sequencing to complement tuberculosis drug resistance surveys in Uganda. Infect. Genet. Evol. J. Mol. Epidemiol. Evol. Genet. Infect. Dis. 40, 8–16 (2016).
Miotto, P., Cirillo, D. M. & Migliori, G. B. Drug Resistance inMycobacterium tuberculosis: Molecular Mechanisms Challenging Fluoroquinolones and Pyrazinamide Eff ectiveness. Chest. 147, 1135–1143 (2015).
Farhat, M. R. et al. Genome Wide Association Study of Mycobacterium Tuberculosis Reveals Multiple Novel Genes Associated with Large Increase in Drug Minimum Inhibitory Concentrations in A25. Tuberculosis Management: New Insights A1153–A1153, https://doi.org/10.1164/ajrccm-conference.2018.197.1_MeetingAbstracts.A1153 American Thoracic Society, (2018).
Nebenzahl-Guimaraes, H. et al. Transmissible Mycobacterium tuberculosis Strains Share Genetic Markers and Immune Phenotypes. Am. J. Respir. Crit. Care Med, https://doi.org/10.1164/rccm.201605-1042OC (2016).
Holt, K. E. et al. Frequent transmission of the Mycobacterium tuberculosis Beijing lineage and positive selection for the EsxW Beijing variant in Vietnam. Nat. Genet. 50, 849–856 (2018).
Pallen, M. J. The ESAT-6/WXG100 superfamily – and a new Gram-positive secretion system? Trends Microbiol. 10, 209–212 (2002).
The Hackout team. OutbreakTools: Basic Tools for the Analysis of Disease Outbreaks (2017).
Acknowledgements
We thank Megan Murray, the co-PI of the Peru Epi study for helpful input on the manuscript. We thank the patients and their families who contributed to this study. We also thank the Partners in Health healthcare personnel at participating health centers in Lima, Peru. We wish to thank Dr. Robert Husson for his invaluable feedback on an initial draft of the manuscript. The study was funded by the National Institutes of Health (Peru Epi study U19-AI076217 and K01-ES026835 to MRF) and The Welch Foundation (A-0015 to JS). The funding sources had no role in any aspect of the study, manuscript or decision to submit it for publication.
Author information
Authors and Affiliations
Contributions
Avika Dixit conducted the data analysis, drafted and revised the manuscript. All authors provided key edits to the manuscript. Additionally: Luca Freschi and Roger Vargas contributed to the data analysis. Roger Calderon performed the DNA extraction and DST testing. James Sacchettini conducted the sequencing of isolates included in the study. Francis Drobniewski shared genomic data and metadata from the London TB outbreak. Jerome T. Galea, Carmen Contreras, Rosa Yataco and Leonid Lecca helped conduct the household contact study in Peru. Zibiao Zhang managed the data from the household contact study in Peru Sergios-Orestis Kolokotronis verified the phylogenetic analysis. Barun Mathema contributed to study design and phylogenetic analysis. Maha Farhat conceptualized the study, supervised the data analysis, reviewed and edited the manuscript.
Corresponding author
Ethics declarations
Competing Interests
The authors declare no competing interests.
Additional information
Publisher’s note: Springer Nature remains neutral with regard to jurisdictional claims in published maps and institutional affiliations.
Supplementary information
Rights and permissions
Open Access This article is licensed under a Creative Commons Attribution 4.0 International License, which permits use, sharing, adaptation, distribution and reproduction in any medium or format, as long as you give appropriate credit to the original author(s) and the source, provide a link to the Creative Commons license, and indicate if changes were made. The images or other third party material in this article are included in the article’s Creative Commons license, unless indicated otherwise in a credit line to the material. If material is not included in the article’s Creative Commons license and your intended use is not permitted by statutory regulation or exceeds the permitted use, you will need to obtain permission directly from the copyright holder. To view a copy of this license, visit http://creativecommons.org/licenses/by/4.0/.
About this article
Cite this article
Dixit, A., Freschi, L., Vargas, R. et al. Whole genome sequencing identifies bacterial factors affecting transmission of multidrug-resistant tuberculosis in a high-prevalence setting. Sci Rep 9, 5602 (2019). https://doi.org/10.1038/s41598-019-41967-8
Received:
Accepted:
Published:
DOI: https://doi.org/10.1038/s41598-019-41967-8
This article is cited by
Comments
By submitting a comment you agree to abide by our Terms and Community Guidelines. If you find something abusive or that does not comply with our terms or guidelines please flag it as inappropriate.