Abstract
Subcortical brain structures are integral to motion, consciousness, emotions and learning. We identified common genetic variation related to the volumes of the nucleus accumbens, amygdala, brainstem, caudate nucleus, globus pallidus, putamen and thalamus, using genome-wide association analyses in almost 40,000 individuals from CHARGE, ENIGMA and UK Biobank. We show that variability in subcortical volumes is heritable, and identify 48 significantly associated loci (40 novel at the time of analysis). Annotation of these loci by utilizing gene expression, methylation and neuropathological data identified 199 genes putatively implicated in neurodevelopment, synaptic signaling, axonal transport, apoptosis, inflammation/infection and susceptibility to neurological disorders. This set of genes is significantly enriched for Drosophila orthologs associated with neurodevelopmental phenotypes, suggesting evolutionarily conserved mechanisms. Our findings uncover novel biology and potential drug targets underlying brain development and disease.
This is a preview of subscription content, access via your institution
Access options
Access Nature and 54 other Nature Portfolio journals
Get Nature+, our best-value online-access subscription
$29.99 / 30 days
cancel any time
Subscribe to this journal
Receive 12 print issues and online access
$209.00 per year
only $17.42 per issue
Buy this article
- Purchase on Springer Link
- Instant access to full article PDF
Prices may be subject to local taxes which are calculated during checkout



Similar content being viewed by others
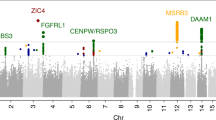
Data availability
The genome-wide summary statistics that support the findings of this study are available from the CHARGE dbGaP (accession code: phs000930) and ENIGMA (http://enigma.ini.usc.edu/research/download-enigma-gwas-results) websites.
References
Marsden, C. D. The mysterious motor function of the basal ganglia: the Robert Wartenberg Lecture. Neurology 32, 514–539 (1982).
Yin, H. H. & Knowlton, B. J. The role of the basal ganglia in habit formation. Nat. Rev. Neurosci. 7, 464–476 (2006).
McDonald, A. J. & Mott, D. D. Functional neuroanatomy of amygdalohippocampal interconnections and their role in learning and memory. J. Neurosci. Res. 95, 797–820 (2016).
Hikosaka, O., Kim, H. F., Yasuda, M. & Yamamoto, S. Basal ganglia circuits for reward value-guided behavior. Annu. Rev. Neurosci. 37, 289–306 (2014).
Salzman, C. D. & Fusi, S. Emotion, cognition, and mental state representation in amygdala and prefrontal cortex. Annu. Rev. Neurosci. 33, 173–202 (2010).
Floresco, S. B. The nucleus accumbens: an interface between cognition, emotion, and action. Annu. Rev. Psychol. 66, 25–52 (2015).
Fabbro, F., Aglioti, S. M., Bergamasco, M., Clarici, A. & Panksepp, J. Evolutionary aspects of self- and world consciousness in vertebrates. Front. Hum. Neurosci. 9, 157 (2015).
Alexander, G. E., DeLong, M. R. & Strick, P. L. Parallel organization of functionally segregated circuits linking basal ganglia and cortex. Annu. Rev. Neurosci. 9, 357–381 (1986).
Jahanshahi, M., Obeso, I., Rothwell, J. C. & Obeso, J. A. A fronto–striato–subthalamic–pallidal network for goal-directed and habitual inhibition. Nat. Rev. Neurosci. 16, 719–732 (2015).
Shepherd, G. M. Corticostriatal connectivity and its role in disease. Nat. Rev. Neurosci. 14, 278–291 (2013).
Stratmann, K. et al. Precortical phase of Alzheimer’s disease (AD)-related Tau cytoskeletal pathology. Brain Pathol. 26, 371–386 (2016).
Del Tredici, K., Rub, U., De Vos, R. A., Bohl, J. R. & Braak, H. Where does Parkinson disease pathology begin in the brain? J. Neuropathol. Exp. Neurol. 61, 413–426 (2002).
Hibar, D. P. et al. Common genetic variants influence human subcortical brain structures. Nature 520, 224–229 (2015).
Elliott, L. T. et al. Genome-wide association studies of brain imaging phenotypes in UK Biobank. Nature 562, 210–216 (2018).
Renteria, M. E. et al. Genetic architecture of subcortical brain regions: common and region-specific genetic contributions. Genes Brain Behav. 13, 821–830 (2014).
Clarke, L. et al. The 1000 Genomes Project: data management and community access. Nat. Methods 9, 459–462 (2012).
McCarthy, S. et al. A reference panel of 64,976 haplotypes for genotype imputation. Nat. Genet. 48, 1279–1283 (2016).
Willer, C. J., Li, Y. & Abecasis, G. R. METAL: fast and efficient meta-analysis of genomewide association scans. Bioinformatics 26, 2190–2191 (2010).
Pruim, R. J. et al. LocusZoom: regional visualization of genome-wide association scan results. Bioinformatics 26, 2336–2337 (2010).
Hibar, D. P. et al. Novel genetic loci associated with hippocampal volume. Nat. Commun. 8, 13624 (2017).
Adams, H. H. et al. Novel genetic loci underlying human intracranial volume identified through genome-wide association. Nat. Neurosci. 19, 1569–1582 (2016).
Verhaaren, B. F. et al. Multiethnic genome-wide association study of cerebral white matter hyperintensities on MRI. Circ. Cardiovasc. Genet. 8, 398–409 (2015).
Malik, R. et al. Multiancestry genome-wide association study of 520,000 subjects identifies 32 loci associated with stroke and stroke subtypes. Nat. Genet. 50, 524–537 (2018).
Yengo, L. et al. Meta-analysis of genome-wide association studies for height and body mass index in approximately 700000 individuals of European ancestry. Hum. Mol. Genet. 27, 3641–3649 (2018).
Davies, G. et al. Study of 300,486 individuals identifies 148 independent genetic loci influencing general cognitive function. Nat. Commun. 9, 2098 (2018).
Kunkle, B. W. et al. Genetic meta-analysis of diagnosed Alzheimer’s disease identifies new risk loci and implicates Aβ, tau, immunity and lipid processing. Nat. Genet. 51, 414–430 (2019).
Simon-Sanchez, J. et al. Genome-wide association study reveals genetic risk underlying Parkinson’s disease. Nat. Genet. 41, 1308–1312 (2009).
Bipolar Disorder and Schizophrenia Working Group of the Psychiatric Genomics Consortium. Genomic dissection of bipolar disorder and schizophrenia, including 28 subphenotypes. Cell 173, 1705–1715.e16 (2018).
Demontis, D. et al. Discovery of the first genome-wide significant risk loci for attention deficit/hyperactivity disorder. Nat. Genet. 51, 63–75 (2019).
Finucane, H. K. et al. Partitioning heritability by functional annotation using genome-wide association summary statistics. Nat. Genet. 47, 1228–1235 (2015).
Hnisz, D. et al. Super-enhancers in the control of cell identity and disease. Cell 155, 934–947 (2013).
Szklarczyk, D. et al. STRINGv10: protein–protein interaction networks, integrated over the tree of life. Nucleic Acids Res. 43, D447–D452 (2015).
Deans, M. R. et al. Control of neuronal morphology by the atypical cadherin Fat3. Neuron 71, 820–832 (2011).
Takahashi, K. et al. Expression of FOXP2 in the developing monkey forebrain: comparison with the expression of the genes FOXP1, PBX3, and MEIS2. J. Comp. Neurol. 509, 180–189 (2008).
Kjaer-Sorensen, K. et al. Pregnancy-associated plasma protein A (PAPP-A) modulates the early developmental rate in zebrafish independently of its proteolytic activity. J. Biol. Chem. 288, 9982–9992 (2013).
Bayes-Genis, A. et al. Pregnancy-associated plasma protein A as a marker of acute coronary syndromes. N. Engl. J. Med. 345, 1022–1029 (2001).
Funayama, A. et al. Serum pregnancy-associated plasma protein A in patients with heart failure. J. Card. Fail. 17, 819–826 (2011).
Desbuquois, B., Carre, N. & Burnol, A. F. Regulation of insulin and type 1 insulin-like growth factor signaling and action by the Grb10/14 and SH2B1/B2 adaptor proteins. FEBS J. 280, 794–816 (2013).
Li, J. et al. TXNDC5 contributes to rheumatoid arthritis by down-regulating IGFBP1 expression. Clin. Exp. Immunol. 192, 82–94 (2018).
Matulka, K. et al. PTP1B is an effector of activin signaling and regulates neural specification of embryonic stem cells. Cell Stem Cell 13, 706–719 (2013).
Krishnan, N. et al. PTP1B inhibition suggests a therapeutic strategy for Rett syndrome. J. Clin. Invest. 125, 3163–3177 (2015).
Sebastian-Serrano, A. et al. Tissue-nonspecific alkaline phosphatase regulates purinergic transmission in the central nervous system during development and disease. Comput. Struct. Biotechnol. J. 13, 95–100 (2015).
Diaz-Hernandez, M. et al. Tissue-nonspecific alkaline phosphatase promotes the neurotoxicity effect of extracellular tau. J. Biol. Chem. 285, 32539–32548 (2010).
Vardy, E. R., Kellett, K. A., Cocklin, S. L. & Hooper, N. M. Alkaline phosphatase is increased in both brain and plasma in Alzheimer’s disease. Neurodegener. Dis. 9, 31–37 (2012).
Kellett, K. A., Williams, J., Vardy, E. R., Smith, A. D. & Hooper, N. M. Plasma alkaline phosphatase is elevated in Alzheimer’s disease and inversely correlates with cognitive function. Int. J. Mol. Epidemiol. Genet. 2, 114–121 (2011).
Searles Quick, V. B., Davis, J. M., Olincy, A. & Sikela, J. M. DUF1220 copy number is associated with schizophrenia risk and severity: implications for understanding autism and schizophrenia as related diseases. Transl. Psychiatry 5, e697 (2015).
Hsu, S. C. et al. Mutations in SLC20A2 are a major cause of familial idiopathic basal ganglia calcification. Neurogenetics 14, 11–22 (2013).
Taglia, I., Bonifati, V., Mignarri, A., Dotti, M. T. & Federico, A. Primary familial brain calcification: update on molecular genetics. Neurol. Sci. 36, 787–794 (2015).
Figueiro-Silva, J. et al. Neuronal pentraxin 1 negatively regulates excitatory synapse density and synaptic plasticity. J. Neurosci. 35, 5504–5521 (2015).
Abad, M. A., Enguita, M., DeGregorio-Rocasolano, N., Ferrer, I. & Trullas, R. Neuronal pentraxin 1 contributes to the neuronal damage evoked by amyloid-β and is overexpressed in dystrophic neurites in Alzheimer’s brain. J. Neurosci. 26, 12735–12747 (2006).
Tobaben, S., Varoqueaux, F., Brose, N., Stahl, B. & Meyer, G. A brain-specific isoform of small glutamine-rich tetratricopeptide repeat-containing protein binds to Hsc70 and the cysteine string protein. J. Biol. Chem. 278, 38376–38383 (2003).
Fonte, V. et al. Interaction of intracellular β amyloid peptide with chaperone proteins. Proc. Natl Acad. Sci. USA 99, 9439–9444 (2002).
Mao, C. X. et al. Microtubule-severing protein katanin regulates neuromuscular junction development and dendritic elaboration in Drosophila. Development 141, 1064–1074 (2014).
Yu, W. et al. The microtubule-severing proteins spastin and katanin participate differently in the formation of axonal branches. Mol. Biol. Cell 19, 1485–1498 (2008).
Zhu, J., Shang, Y. & Zhang, M. Mechanistic basis of MAGUK-organized complexes in synaptic development and signalling. Nat. Rev. Neurosci. 17, 209–223 (2016).
Ingason, A. et al. Expression analysis in a rat psychosis model identifies novel candidate genes validated in a large case-control sample of schizophrenia. Transl. Psychiatry 5, e656 (2015).
Nithianantharajah, J. et al. Synaptic scaffold evolution generated components of vertebrate cognitive complexity. Nat. Neurosci. 16, 16–24 (2013).
Nalls, M. A. et al. Large-scale meta-analysis of genome-wide association data identifies six new risk loci for Parkinson’s disease. Nat. Genet. 46, 989–993 (2014).
Guan, J. J. et al. DRAM1 regulates apoptosis through increasing protein levels and lysosomal localization of BAX. Cell Death Dis. 6, e1624 (2015).
Yu, M., Jiang, Y., Feng, Q., Ouyang, Y. & Gan, J. DRAM1 protects neuroblastoma cells from oxygen-glucose deprivation/reperfusion-induced injury via autophagy. Int. J. Mol. Sci. 15, 19253–19264 (2014).
Scarpa, J. R. et al. Systems genetic analyses highlight a TGFβ-FOXO3 dependent striatal astrocyte network conserved across species and associated with stress, sleep, and Huntington’s disease. PLoS Genet. 12, e1006137 (2016).
Donlon, T. A. et al. FOXO3 longevity interactome on chromosome 6. Aging Cell 16, 1016–1025 (2017).
Sears, J. C. & Broihier, H. T. FoxO regulates microtubule dynamics and polarity to promote dendrite branching in Drosophila sensory neurons. Dev. Biol. 418, 40–54 (2016).
Peng, K. et al. Knockdown of FoxO3a induces increased neuronal apoptosis during embryonic development in zebrafish. Neurosci. Lett. 484, 98–103 (2010).
Santama, N., Er, C. P., Ong, L. L. & Yu, H. Distribution and functions of kinectin isoforms. J. Cell Sci. 117, 4537–4549 (2004).
Liu, X. A., Rizzo, V. & Puthanveettil, S. V. Pathologies of axonal transport in neurodegenerative diseases. Transl. Neurosci. 3, 355–372 (2012).
Consortium, E. et al. Genome-wide association analysis of genetic generalized epilepsies implicates susceptibility loci at 1q43, 2p16.1, 2q22.3 and 17q21.32. Hum. Mol. Genet. 21, 5359–5372 (2012).
Martins-de-Souza, D. et al. Proteomic analysis identifies dysfunction in cellular transport, energy, and protein metabolism in different brain regions of atypical frontotemporal lobar degeneration. J. Proteome Res. 11, 2533–2543 (2012).
Shulman, J. M. et al. Functional screening in Drosophila identifies Alzheimer’s disease susceptibility genes and implicates Tau-mediated mechanisms. Hum. Mol. Genet. 23, 870–877 (2014).
Friede, R. L. & Samorajski, T. Axon caliber related to neurofilaments and microtubules in sciatic nerve fibers of rats and mice. Anat. Rec. 167, 379–387 (1970).
Yuan, A., Rao, M. V., Veeranna & Nixon, R. A. Neurofilaments and neurofilament proteins in health and disease. Cold Spring Harb. Perspect. Biol. 9, a018309 (2017).
Bis, J. C. et al. Whole exome sequencing study identifies novel rare and common Alzheimer’s-associated variants involved in immune response and transcriptional regulation. Mol Psychiatry https://doi.org/10.1038/s41380-018-0112-7 (2018).
Marioni, R. E. et al. GWAS on family history of Alzheimer’s disease. Transl. Psychiatry 8, 99 (2018).
Psaty, B. M. et al. Cohorts for Heart and Aging Research in Genomic Epidemiology (CHARGE) Consortium: design of prospective meta-analyses of genome-wide association studies from 5 cohorts. Circ. Cardiovasc. Genet. 2, 73–80 (2009).
Thompson, P. M. et al. The ENIGMA Consortium: large-scale collaborative analyses of neuroimaging and genetic data. Brain Imaging Behav. 8, 153–182 (2014).
Sudlow, C. et al. UK Biobank: an open access resource for identifying the causes of a wide range of complex diseases of middle and old age. PLoS Med. 12, e1001779 (2015).
Tsao, C. W. & Vasan, R. S. Cohort Profile: the Framingham Heart Study (FHS): overview of milestones in cardiovascular epidemiology. Int. J. Epidemiol. 44, 1800–1813 (2015).
Schmidt, R. et al. Assessment of cerebrovascular risk profiles in healthy persons: definition of research goals and the Austrian Stroke Prevention Study (ASPS). Neuroepidemiology 13, 308–313 (1994).
Almasy, L. & Blangero, J. Multipoint quantitative-trait linkage analysis in general pedigrees. Am. J. Hum. Genet. 62, 1198–1211 (1998).
Yang, J., Lee, S. H., Goddard, M. E. & Visscher, P. M. GCTA: a tool for genome-wide complex trait analysis. Am. J. Hum. Genet. 88, 76–82 (2011).
Bulik-Sullivan, B. K. et al. LD score regression distinguishes confounding from polygenicity in genome-wide association studies. Nat. Genet. 47, 291–295 (2015).
Winkler, T. W. et al. Quality control and conduct of genome-wide association meta-analyses. Nat. Protoc. 9, 1192–1212 (2014).
Bennett, D. A., Yu, L. & De Jager, P. L. Building a pipeline to discover and validate novel therapeutic targets and lead compounds for Alzheimer’s disease. Biochem. Pharm. 88, 617–630 (2014).
Chan, G. et al. CD33 modulates TREM2: convergence of Alzheimer loci. Nat. Neurosci. 18, 1556–1558 (2015).
Johnson, W. E., Li, C. & Rabinovic, A. Adjusting batch effects in microarray expression data using empirical Bayes methods. Biostatistics 8, 118–127 (2007).
Roadmap Epigenomics Association et al. Integrative analysis of 111 reference human epigenomes. Nature 518, 317–330 (2015).
Eicher, J. D. et al. GRASPv2.0: an update on the Genome-Wide Repository of Associations between SNPs and phenotypes. Nucleic Acids Res. 43, D799–D804 (2015).
Zhang, X. et al. Synthesis of 53 tissue and cell line expression QTL datasets reveals master eQTLs. BMC Genomics 15, 532 (2014).
Zhang, W. et al. SCAN database: facilitating integrative analyses of cytosine modification and expression QTL. Database 2015, bav025 (2015).
Consortium, G. T. The Genotype-Tissue Expression (GTEx) project. Nat. Genet. 45, 580–585 (2013).
Veyrieras, J. B. et al. High-resolution mapping of expression-QTLs yields insight into human gene regulation. PLoS Genet. 4, e1000214 (2008).
Bennett, D. A. et al. Overview and findings from the rush Memory and Aging Project. Curr. Alzheimer Res. 9, 646–663 (2012).
Bennett, D. A., Schneider, J. A., Arvanitakis, Z. & Wilson, R. S. Overview and findings from the religious orders study. Curr. Alzheimer Res. 9, 628–645 (2012).
Replogle, J. M. et al. A TREM1 variant alters the accumulation of Alzheimer-related amyloid pathology. Ann. Neurol. 77, 469–477 (2015).
Barnes, L. L., Schneider, J. A., Boyle, P. A., Bienias, J. L. & Bennett, D. A. Memory complaints are related to Alzheimer disease pathology in older persons. Neurology 67, 1581–1585 (2006).
McKeith, I. G. et al. Consensus guidelines for the clinical and pathologic diagnosis of dementia with Lewy bodies (DLB): report of the Consortium on DLB International Workshop. Neurology 47, 1113–1124 (1996).
Schneider, J. A. et al. Substantia nigra tangles are related to gait impairment in older persons. Ann. Neurol. 59, 166–173 (2006).
Zheng, J. et al. LD Hub: a centralized database and web interface to perform LD score regression that maximizes the potential of summary level GWAS data for SNP heritability and genetic correlation analysis. Bioinformatics 33, 272–279 (2017).
Wangler, M. F., Hu, Y. & Shulman, J. M. Drosophila and genome-wide association studies: a review and resource for the functional dissection of human complex traits. Dis. Model Mech. 10, 77–88 (2017).
Hu, Y. et al. An integrative approach to ortholog prediction for disease-focused and other functional studies. BMC Bioinformatics 12, 357 (2011).
Marygold, S. J., Crosby, M. A., Goodman, J. L. & FlyBase, C. Using FlyBase, a database of Drosophila genes and genomes. Methods Mol. Biol. 1478, 1–31 (2016).
Acknowledgements
We thank all of the study participants for contributing to this research. Full acknowledgements and grant support details are provided in the Supplementary Note.
Author information
Authors and Affiliations
Contributions
C.L.S. drafted the manuscript with contributions from H.H.H.A., D.P.H., C.C.W., T.V.L., A.A.-V., S.Ehrlich., A.K.H., M.W.V., D.J., T.G.M.v.E., C.D.W., M.J.W., S.E.F., K.A.M., P.J.H., B.F., H.J.G., A.D.J., O.L.L., S.Debette, S.E.M., J.M.S., P.M.T., S.S. and M.A.I. M.S., N.J., L.R.Y., T.V.L., G.C., L.A., M.E.R., A.d.B., I.K., M.A., S.A., S.E., R.R.-S., A.K.H., H.J.J., A.Stevens., J.B., M.W.V., A.V.W., K.W., N.A., S.H., A.L.G., P.H.L., S.G., S.L.H., D.K., L.Schmaal, S.M.L., I.A., E.W., D.T.-G., J.C.I., L.N.V., R.B., F.C., D.J., O.C., U.K.H., B.S.A., C.-Y.C., A.A.A., M.P.B., A.F.M., S.K.M., P.A., A.J.Schork., D.C.M.L., T.Y.W., L.Shen, P.G.S., E.J.C.d.G., M.T., K.R.v.E., N.J.A.v.d.W., A.M.M., J.S.R., N.R., W.H., M.C.V.H., J.B.J.K., L.M.O.L., A.Hofman, G.H., M.E.B., S.R., J.-J.H., A.Simmons, N.H., P.R.S., T.W.M., P.Maillard, O.Gruber, N.A.G., J.E.S., H.Lemaître, B.M.-M., D.v.R., I.J.D., R.M.B., I.M., R.K., H.v.B., M.J.W., D.v.‘t.E., M.M.N., S.E.F., A.S.B., K.A.M., N.R.-S., D.J.H., H.J.G., C.M.v.D., J.M.W., C.DeCarli, P.L.D.J. and V.G. contributed to the preparation of data. C.L.S., H.H.H.A., D.P.H., M.J.K., J.L.S., M.S., M.Sargurupremraj, N.J., G.V.R., A.V.S., J.C.B., X.J., M.Luciano., E.H., A.Teumer, S.J.v.d.L., J.Y., L.R.Y., S.L., K.J.Y., G.C., M.E.R., N.J.A., H.J.J., A.V.W., S.H., N.M.S., S.G., D.T.G., J.S., C.-Y.C., L.M.O.L., Q.Y., A.Thalamuthu, I.O.F., D.v.‘t.E., C.Depondt and P.L.D.J. performed the statistical analyses. C.L.S., H.H.H.A., C.C.W., M.J.K., T.V.L., S.L., Y.H., K.J.Y., J.D.E., Q.Y. and A.D.J. carried out the downstream analyses. All authors reviewed the manuscript for intellectual content.
Corresponding authors
Ethics declarations
Competing interests
D.P.H. is currently an employee at Genentech. D.J. has received travel and speaker’s honoraria from Janssen–Cilag, as well as research funding from DFG. R.L.B. is a consultant for Pfizer and Roche. P.A. is a scientific adviser for Genoscreen. T.Y.W. is a consultant and advisory board member for Allergan, Bayer, Boehringer–Ingelheim, Genentech, Merck, Novartis, Oxurion (formerly ThromboGenics) and Roche, and is a co-founder of Plano and EyRiS. A.M.M. has received grant support from Eli Lilly, Janssen, Pfizer and the Sackler Trust. B.M.P. serves on the steering committee of the Yale Open Data Access Project funded by Johnson & Johnson. A.M.-L. is a member of the advisory board for the Lundbeck International Neuroscience Foundation and Brainsway, a member of the editorial board for the American Association for the Advancement of Science and Elsevier, a faculty member of the Lundbeck International Neuroscience Foundation and a consultant for Boehringer Ingelheim. W.J.N. is the founder and scientific lead of Quantib BV, in addition to being a shareholder. M.M.N. is a shareholder of Life & Brain, receives a salary from Life & Brain, has received support from Shire for attending conferences and has received financial remuneration from the Lundbeck Foundation, Robert Bosch Foundation and Deutsches Ärzteblatt for participation in scientific advisory boards. B.F. has received educational speaking fees from Shire and Medice. H.J.G. has received travel grants and speaker’s honoraria from Fresenius Medical Care, Neuraxpharm and Janssen–Cilag, as well as research funding from Fresenius Medical Care.
Additional information
Publisher’s note Springer Nature remains neutral with regard to jurisdictional claims in published maps and institutional affiliations.
Supplementary information
Supplementary Information
Supplementary Note and Figs. 1–4
Supplementary Tables
Supplementary Tables 1–21
Rights and permissions
About this article
Cite this article
Satizabal, C.L., Adams, H.H.H., Hibar, D.P. et al. Genetic architecture of subcortical brain structures in 38,851 individuals. Nat Genet 51, 1624–1636 (2019). https://doi.org/10.1038/s41588-019-0511-y
Received:
Accepted:
Published:
Issue Date:
DOI: https://doi.org/10.1038/s41588-019-0511-y
This article is cited by
-
Insights into the genetic architecture of cerebellar lobules derived from the UK Biobank
Scientific Reports (2024)
-
Genetic architecture of the structural connectome
Nature Communications (2024)
-
Proxy-analysis of the genetics of cognitive decline in Parkinson’s disease through polygenic scores
npj Parkinson's Disease (2024)
-
Unsupervised deep representation learning enables phenotype discovery for genetic association studies of brain imaging
Communications Biology (2024)
-
Unveiling Promising Neuroimaging Biomarkers for Schizophrenia Through Clinical and Genetic Perspectives
Neuroscience Bulletin (2024)