Abstract
Background/Objectives:
High consumption of meat has been linked with the risk for obesity and chronic diseases. This could partly be explained by the association between meat and lower-quality diet. We studied whether high intake of red and processed meat was associated with lower-quality dietary habits, assessed against selected nutrients, other food groups and total diet. Moreover, we studied whether meat consumption was associated with obesity, after adjustment for all identified associations between meat and food consumption.
Subjects/Methods:
The nationally representative cross-sectional study population consisted of 2190 Finnish men and 2530 women, aged 25–74 years. Food consumption over the previous 12 months was assessed using a validated 131-item Food Frequency Questionnaire. Associations between nutrients, foods, a modified Baltic Sea Diet Score and meat consumption (quintile classification) were analysed using linear regression. The models were adjusted for age and energy intake and additionally for education, physical activity and smoking.
Results:
High consumption of red and processed meat was inversely associated with fruits, whole grain and nuts, and positively with potatoes, oil and coffee in both sexes. Results separately for the two types of meat were essentially similar. In a linear regression analysis, high consumption of meat was positively associated with body mass index in both men and women, even when using a model adjusted for all foods with a significant association with meat consumption in both sexes identified in this study.
Conclusions:
The association between meat consumption and a lower-quality diet may complicate studies on meat and health.
This is a preview of subscription content, access via your institution
Access options
Subscribe to this journal
Receive 12 print issues and online access
$259.00 per year
only $21.58 per issue
Buy this article
- Purchase on Springer Link
- Instant access to full article PDF
Prices may be subject to local taxes which are calculated during checkout
Similar content being viewed by others
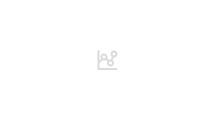
References
Kearney J . Food consumption trends and drivers. Philos Trans R Soc Lond B Biol Sci 2010; 365: 2793–2807.
Aston LM, Smith JN, Powles JW . Impact of a reduced red and processed meat dietary pattern on disease risks and greenhouse gas emissions in the UK: a modelling study. BMJ Open 2012; 2: e001072.
Micha R, Michas G, Mozaffarian D . Unprocessed red and processed meats and risk of coronary artery disease and type 2 diabetes—an updated review of the evidence. Curr Atheroscler Rep 2012; 14: 515–524.
Rohrmann S, Overvad K, Bueno-de-Mesquita HB, Jakobsen MU, Egeberg R, Tjønneland A et al. Meat consumption and mortality—results from the European Prospective Investigation into Cancer and Nutrition. BMC Med 2013; 11: 63.
Chan DSM, Lau R, Aune D, Vieira R, Greenwood DC, Kampman E et al. Red and processed meat and colorectal cancer incidence: meta-analysis of prospective studies. PLoS One 2011; 6: e20456.
Larsson SC, Wolk A . Red and processed meat consumption and risk of pancreatic cancer: meta-analysis of prospective studies. Br J Cancer 2012; 106: 603–607.
Männistö S, Kontto J, Kataja-Tuomola M, Albanes D, Virtamo J . High processed meat consumption is a risk factor of type 2 diabetes in the Alpha-Tocopherol, Beta-Carotene Cancer Prevention study. Br J Nutr 2010; 103: 1817–1822.
Fretts AM, Howard BV, McKnight B, Duncan GE, Beresford SAA, Mete M et al. Associations of processed meat and unprocessed red meat intake with incident diabetes: the Strong Heart Family Study. Am J Clin Nutr 2012; 95: 752–758.
Brennan IM, Luscombe-Marsh ND, Seimon RV, Otto B, Horowitz M, Wishart JM et al. Effects of fat, protein, and carbohydrate and protein load on appetite, plasma cholecystokinin, peptide YY, and ghrelin, and energy intake in lean and obese men. Am J Physiol Gastrointest Liver Physiol 2012; 303: G129–G140.
Fogelholm M, Anderssen S, Gunnarsdottir I, Lahti-Koski M . Dietary macronutrients and food consumption as determinants of long-term weight change in adult populations: a systematic literature review. Food Nutr Res 2012; 56; doi:10.3402/fnr.v56i0.19103.
Vergnaud A-C, Norat T, Romaguera D, Mouw T, May AM, Travier N et al. Meat consumption and prospective weight change in participants of the EPIC-PANACEA study. Am J Clin Nutr 2010; 92: 398–407.
Kappeler R, Eichholzer M, Rohrmann S . Meat consumption and diet quality and mortality in NHANES III. Eur J Clin Nutr 2013; 67: 598–606.
Pan A, Sun Q, Bernstein AM, Schulze MB, Manson JE, Willett WC et al. Red meat consumption and risk of type 2 diabetes: 3 cohorts of US adults and an updated meta-analysis. Am J Clin Nutr 2011; 94: 1088–1096.
Vartiainen E, Laatikainen T, Peltonen M, Juolevi A, Männistö S, Sundvall J et al. Thirty-five-year trends in cardiovascular risk factors in Finland. Int J Epidemiol 2010; 39: 504–518.
Männistö S, Virtanen M, Mikkonen T, Pietinen P . Reproducibility and validity of a food frequency questionnaire in a case-control study on breast cancer. J Clin Epidemiol 1996; 49: 401–409.
Kaartinen NE, Tapanainen H, Valsta LM, Similä ME, Reinivuo H, Korhonen T et al. Relative validity of a FFQ in measuring carbohydrate fractions, dietary glycaemic index and load: exploring the effects of subject characteristics. Br J Nutr 2012; 107: 1367–1375.
Reinivuo H, Hirvonen T, Ovaskainen M-L, Korhonen T, Valsta LM . Dietary survey methodology of FINDIET 2007 with a risk assessment perspective. Public Health Nutr 2010; 13: 915–919.
Meltzer HM, Brantsaeter AL, Ydersbond TA, Alexander J, Haugen M . Methodological challenges when monitoring the diet of pregnant women in a large study: experiences from the Norwegian Mother and Child Cohort Study (MoBa). Matern Child Nutr 2008; 4: 14–27.
Kanerva N, Kaartinen NE, Schwab U, Lahti-Koski M, Männistö S . Adherence to the Baltic Sea diet consumed in the Nordic countries is associated with lower abdominal obesity. Br J Nutr 2012; 10: 1–9.
Tolonen H, Koponen P, Aromaa A . Recommendations for the Health Examination Surveys in Europe. The National Public Health Institute: Helsinki, Finland, 2008.
R Development Core Team R: A language and environment for statistical computing (Internet). (cited 3 February 2013). Available from: www.R-project.org.
Willett W, Stampfer MJ . Total energy intake: implications for epidemiologic analyses. Am J Epidemiol 1986; 124: 17–27.
FAO/WHO/UNU. Report of a joint expert consultation. Energy and Protein Requirements. World Health Organization: Geneva, Switzerland, 1985.
Goldberg GR, Black AE, Jebb SA, Cole TJ, Murgatroyd PR, Coward WA et al. Critical evaluation of energy intake data using fundamental principles of energy physiology: 1. Derivation of cut-off limits to identify under-recording. Eur J Clin Nutr 1991; 45: 569–581.
Black AE . Critical evaluation of energy intake using the Goldberg cut-off for energy intake:basal metabolic rate. A practical guide to its calculation, use and limitations. Int J Obes Relat Metab Disord 2000; 24: 1119–1130.
Fung TT, Schulze M, Manson JE, Willett WC, Hu FB . Dietary patterns, meat intake, and therisk of type 2 diabetes in women. Arch Intern Med 2004; 164: 2235–2240.
Mikkilä V, Räsänen L, Raitakari OT, Pietinen P, Viikari J . Consistent dietary patterns identified from childhood to adulthood: the cardiovascular risk in Young Finns Study. Br J Nutr 2005; 93: 923–931.
Rosell M, Appleby P, Spencer E, Key T . Weight gain over 5 years in 21,966 meat-eating, fish-eating, vegetarian, and vegan men and women in EPIC-Oxford. Int J Obes (Lond) 2006; 30: 1389–1396.
Sánchez-Villegas A, Bes-Rastrollo M, Martínez-González MA, Serra-Majem L . Adherence to a Mediterranean dietary pattern and weight gain in a follow-up study: the SUN cohort. Int J Obes (Lond) 2006; 30: 350–358.
Halkjaer J, Tjønneland A, Overvad K, Sørenson TIA . Dietary predictors of 5-year changes in waist circumference. J Am Diet Assoc 2009; 109: 1356–1366.
Mozaffarian D, Hao T, Rimm EB, Willett WC, Hu FB . Changes in diet and lifestyle and long-term weight gain in women and men. N Engl J Med 2011; 364: 2392–2404.
Romaguera D, Ängquist L, Du H, Jakobsen MU, Forouhi NG, Halkjær J et al. Food composition of the diet in relation to changes in waist circumference adjusted for body mass index. PLoS One 2011; 6: e23384.
Acknowledgements
The study was supported by the Academy of Finland (grants 136895 and 263836).
Author Contributions
MF and SM designed research; NK analyzed data; all the authors wrote the paper; and MF had primary responsibility for the final content.
Author information
Authors and Affiliations
Corresponding author
Ethics declarations
Competing interests
The authors declare no conflict of interest.
Additional information
Supplementary Information accompanies this paper on European Journal of Clinical Nutrition website
Rights and permissions
About this article
Cite this article
Fogelholm, M., Kanerva, N. & Männistö, S. Association between red and processed meat consumption and chronic diseases: the confounding role of other dietary factors. Eur J Clin Nutr 69, 1060–1065 (2015). https://doi.org/10.1038/ejcn.2015.63
Received:
Revised:
Accepted:
Published:
Issue Date:
DOI: https://doi.org/10.1038/ejcn.2015.63
This article is cited by
-
The nature of protein intake as a discriminating factor of diet sustainability: a multi-criteria approach
Scientific Reports (2023)
-
Red meat consumption and all-cause and cardiovascular mortality: results from the UK Biobank study
European Journal of Nutrition (2022)
-
Default Vegetarianism and Veganism
Journal of Agricultural and Environmental Ethics (2021)
-
Climate change: a natural streamliner towards entomophagy?
International Journal of Tropical Insect Science (2021)
-
Therapy to Obese Type 2 Diabetes Mellitus: How Far Will We Go Down the Wrong Road?
Chinese Journal of Integrative Medicine (2020)