Speaking in 2019, Bill Gates said that if he were beginning his career today he would “start an [artificial intelligence] company whose goal would be to teach computers how to read, so that they can absorb and understand all the written knowledge of the world.”
This is one of the key goals that nference has been pursuing for biomedical knowledge since it was founded in 2013. Headquartered in Cambridge, Massachusetts, nference has grown rapidly in the past 2 years, tripling its workforce to more than 150 scientists and engineers with advanced degrees from world-leading biomedical and computer science institutions, including the Massachusetts Institute of Technology and Harvard Medical School. Today nference has offices in Bangalore, India; Toronto, Canada; and Rochester, Minnesota.
nference operates at the convergence of three growing trends: the explosion of biological knowledge in the ‘multi-omics’ era, the coming of age of electronic health records and new developments in deep-learning neural networks. nference is uniquely positioned to generate new insights into health care by occupying the sweet spot that exists at the intersection of basic biology, clinical care and computer science.
Unlocking biomedical knowledge
The ever-increasing growth of biological knowledge from genomics, single-cell RNA sequencing, proteomics, metabolomics and all the other strands of the multi-omics era are yielding deep insights into disease processes and pathology. Making the best use of this enormous quantity of data has, however, been hampered by the fact that these data sets often sit in distinct silos, and the expert know-how needed to leverage insights from multi-omics data resides in a few specialized labs.
At the same time, a vast amount of valuable but untapped biomedical knowledge is encoded in electronic health records. A small proportion of this knowledge is represented by structured data, such as the International Classification of Disease (ICD) codes, which provide important insights into the clinical status of patients. A drawback of these structured data is that they use, by necessity, a very constrained and inflexible vocabulary that is frequently unable to capture crucial details about the context and specific details of a patient’s journey in the healthcare system. In many ways, trying to capture the complexity of a patient’s clinical experience with such structured data is akin to trying to describe the rich details of someone’s biography in a spreadsheet with a highly confined choice of words and clichés that have been generalized for the whole population.
Alongside the structured data that reside in electronic health records sits a much greater volume of unstructured information in the form of physician notes. This information, written by physicians to be read by other physicians, contains fine-grained contextual and patient-specific details about health and disease over time, and comprises up to 90% of veritable biomedical information in the electronic health records. Up until now, this rich resource has been largely untapped, except when individual physicians have consulted the notes in the course of their clinical care for patients.
Extracting and curating valuable health data
nference is seeking to revolutionize the value of electronic health records and turn this unstructured data into knowledge that can be used widely by the biomedical and health-care communities. To achieve this goal, nference is using deep-learning neural networks to extract and curate insights from the wealth of unstructured or semi-structured data currently sitting silent in electronic health records. This is a golden age of computer science, and artificial intelligence (AI) driven by machine learning and neural networks is set to seep into every aspect of our personal and professional lives, from self-driving cars, smart homes and facial recognition to AI-driven financial services, AI-regulated energy sectors and, in nference’s vision, health care.
The knowledge extracted from unstructured health records is valuable by itself, but gains even more worth when married to insights and inferences that emerge from the machine-learning analysis of other forms of unstructured data. These include the output of multi-omics efforts, details of clinical-trial protocols, imaging data such as radiology, as well as more than 100 million biomedical documents from diverse sources such as PubMed, clinical trial records, US Securities and Exchange Commission (SEC) filings, grants, preprints, patents, company websites and the broader media. These sources can be additionally buttressed by numerous structured databases, such as lab tests, vitals and the US Food and Drug Administration (FDA) adverse event reporting system (Fig. 1).
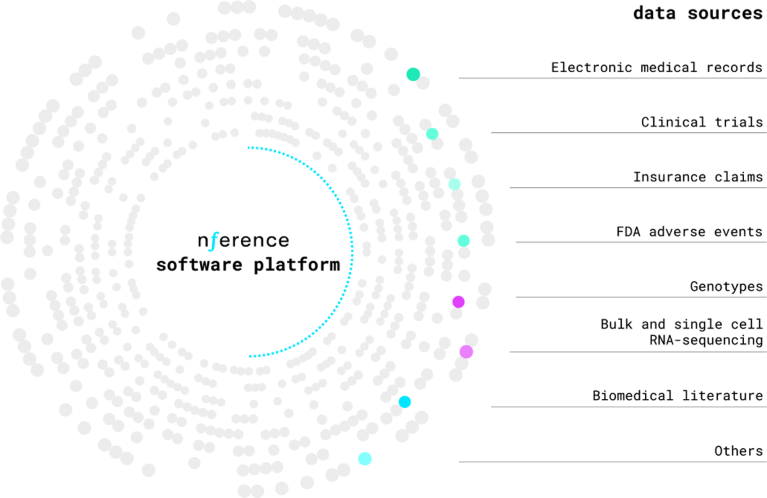
Fig. 1 | Pieces of the platform. A wide variety of data sources contribute to the nference technology platform. The machine-learning analysis derived from the collection of these sources helps to unlock and extract the knowledge and value from unstructured health records.
Although machine learning and neural networks are central to the nference technology platform, the result is not AI as some understand it. Instead, nference dubs their approach ‘augmented intelligence’, an alternative conceptualization of AI that focuses on its assistive role and emphasizes the fact that cognitive technology is designed to enhance human intelligence rather than replace it. The notion of augmented intelligence reinforces the role that expert human intelligence plays, particularly the curiosity that drives many salient research questions when developing thoughtful machine-learning and deep-learning models.
Such augmented intelligence, nference believes, will help rapidly pressure-test hypotheses to weed out the vast majority of false positives and false negatives in putative relationships via intense triangulation across diverse data sets. This distinctive approach, which blends the best of human scientists’ training and wisdom with the ongoing renaissance in deep-learning and unsupervised neural networks, has the potential to aid all aspects of health care, including drug discovery, clinical research, clinical-trial operations, life cycle management and clinical care.
Partnering with the Mayo Clinic
In a major step forward toward fulfilling the company’s vision, nference recently received $60 million in Series B financing that included a significant strategic investment from the Mayo Clinic. The strategic partnership with Mayo Clinic was established to transform health care by applying the distinctive nference technologies to making nearly 150 years’ worth of Mayo’s proprietary knowledge bases computable and actionable for researchers, drug hunters, physicians and patients.
Mayo has digitized more than 9 million complete electronic health records containing huge amounts of unstructured knowledge, with nearly 25 million pathology slides in their archives and patient-derived biospecimens. These resources provide another crucial link for connecting multi-omics data and deep pathological inferences to the context-rich, real-world, de-identified clinical trajectories of patients. nference is already de-identifying and gearing up to analyze the structured data residing in the de-identified health records, while simultaneously innovating technologies that overcome the scientific challenges that have prevented other companies from augmenting the human curation of rich unstructured knowledge through machine intelligence.
The strategic partnership between Mayo and nference constitutes Mayo’s Clinical Data Analytics Platform (CDAP) initiative, which has been established with Google as a cloud provider to house the de-identified data securely. The Mayo CDAP initiative features a distinctive federated architecture that has the potential to dramatically improve biomedical research and health-care delivery by bringing sophisticated digital technologies and augmented intelligence models from nference and its partners into the secure cloud framework.
In the current era of almost universal use of social media, privacy issues have rightfully emerged as a major concern among citizens and regulators. nference puts patient privacy first in all its efforts. In the CDAP initiative, de-identified patient data reside fully within Mayo’s span of control and do not leave their secure cloud framework. In addition to ensuring that even the anonymized patient data do not get into the hands of others, this secure federated learning model brings machine intelligence to bear where the data truly belong—the care provider’s infrastructure.
Taking advantage of the explosion in biomedical data presents great challenges (Fig. 2), but the payoffs for stakeholders across the entire spectrum of health care are enormous. For clinicians, augmented intelligence through machine learning could dra-matically improve patient care. Today, physicians can draw on the clinical insights of a small number of colleagues with whom they interact in their day-to-day work or in collaborations that draw on a limited number of manually de-identified patient health records. With ground-breaking automated de-identification technology from nference, combined with the augmented curation and triangulation technologies that nference has developed, physicians and practitioners in the near future will be able to draw on the collective wisdom of entire institutions such as the Mayo Clinic. Patients will be much more likely to receive the best standard of care, and the most appropriate therapies for their personalized medical needs.
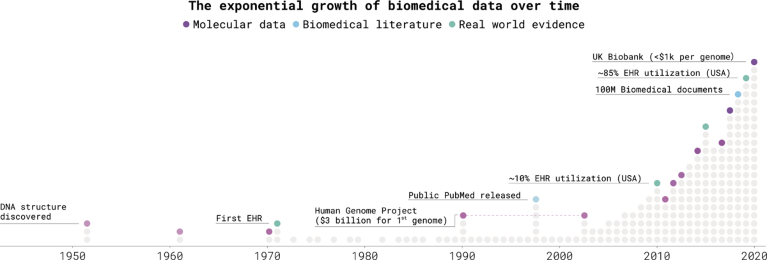
Fig. 2 | Timeline charting the growth of biomedical data. Since the discovery of DNA in the early 1950s to the sequencing of the human genome in the 1990s, the quantity of global biomedical data has rapidly grown.
Opportunities for biopharma
For the biopharmaceutical sector, the opportunities created by augmented intelligence applied to the unstructured data created by multi-omics and many other diverse data sources are similarly profound. The impact will be felt at every stage of the R&D chain, from identifying new drug targets and the design of preclinical studies that predict drug efficacy and safety, as well as translational medicine for patient segmentation based on biomarkers, to the design of clinical-trial protocols that reduce protocol complexity and amplify appropriate patient recruitment as part of clinical-trial operations. But the impact of data-science solutions does not stop there, and will contribute to the entire life cycle management of drugs, informing strategies for post-marketing surveillance and label expansions, decisions about drug repurposing for addressing unmet clinical need and business development strategies based on intense market segmentation and competitive landscaping.
nference believes that the convergence of the three strands of multi-omics, electronic health records and computer science, in this era of exponential knowledge growth across public and proprietary domains, is the wave of the future. “The explosion of digital biomedical information has the power to revolutionize drug development and health-care delivery. We believe that a holistic, machine-learning platform that synthesizes deep biological knowledge with insights drawn from large cohorts of fully de-identified health records is key to creating new life-saving therapies and the most effective clinical care solutions,” said Venky Soundararajan, co-founder and CSO of nference.
nference is advancing new strategic collaborations with biopharmaceutical companies operating at all stages, from early R&D to clinical development. nference is also fostering deep strategic partnerships with leading academic medical centers to help them de-identify and synthesize the vast stores of clinical knowledge that currently remain largely unviable for biopharma research and clinical care at a meaningful scale that drives significant patient benefit.