Abstract
The etiology and effect of age-related immune dysfunction in cancer is not completely understood. Here we show that limited priming of CD8+ T cells in the aged tumor microenvironment (TME) outweighs cell-intrinsic defects in limiting tumor control. Increased tumor growth in aging is associated with reduced CD8+ T cell infiltration and function. Transfer of T cells from young mice does not restore tumor control in aged mice owing to rapid induction of T cell dysfunction. Cell-extrinsic signals in the aged TME drive a tumor-infiltrating age-associated dysfunctional (TTAD) cell state that is functionally, transcriptionally and epigenetically distinct from canonical T cell exhaustion. Altered natural killer cell–dendritic cell–CD8+ T cell cross-talk in aged tumors impairs T cell priming by conventional type 1 dendritic cells and promotes TTAD cell formation. Aged mice are thereby unable to benefit from therapeutic tumor vaccination. Critically, myeloid-targeted therapy to reinvigorate conventional type 1 dendritic cells can improve tumor control and restore CD8+ T cell immunity in aging.
This is a preview of subscription content, access via your institution
Access options
Access Nature and 54 other Nature Portfolio journals
Get Nature+, our best-value online-access subscription
$29.99 / 30 days
cancel any time
Subscribe to this journal
Receive 12 print issues and online access
$209.00 per year
only $17.42 per issue
Buy this article
- Purchase on Springer Link
- Instant access to full article PDF
Prices may be subject to local taxes which are calculated during checkout






Similar content being viewed by others
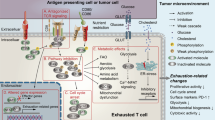
Data availability
Sequence data (scRNA-seq and ATAC-seq) have been deposited in the Gene Expression Omnibus under accession codes GSE255373 and GSE255230. All human scRNA-seq datasets analyzed in this study have been previously published and are publicly available (GSE179994, GSE115978 and E-MTAB-8107). The gene sets analyzed with GSEA are available through the Broad Institute Molecular Signatures Database. Source data are provided with this paper.
Code availability
No custom code beyond adaptation of existing software packages were used in this study. All code will be provided upon reasonable request by the corresponding author.
References
Berben, L., Floris, G., Wildiers, H. & Hatse, S. Cancer and aging: two tightly interconnected biological processes. Cancers 13, 1400 (2021).
Tsukamoto, H., Senju, S., Matsumura, K., Swain, S. L. & Nishimura, Y. IL-6-mediated environmental conditioning of defective TH1 differentiation dampens antitumour immune responses in old age. Nat. Commun. 6, 6702 (2015).
Sade-Feldman, M. et al. Defining T cell states associated with response to checkpoint immunotherapy in melanoma. Cell 175, 998–1013 (2018).
Schreiber, R. D., Old, L. J. & Smyth, M. J. Cancer immunoediting: integrating immunity’s roles in cancer suppression and promotion. Science 331, 1565–1570 (2011).
Goronzy, J. J., Fang, F., Cavanagh, M. M., Qi, Q. & Weyand, C. M. Naive T cell maintenance and function in human aging. J. Immunol. 194, 4073–4080 (2015).
Moskowitz, D. M. et al. Epigenomics of human CD8 T cell differentiation and aging. Sci. Immunol. 2, eaag0192 (2017).
Nikolich-Zugich, J. Ageing and life-long maintenance of T-cell subsets in the face of latent persistent infections. Nat. Rev. Immunol. 8, 512–522 (2008).
Moskophidis, D., Lechner, F., Pircher, H. & Zinkernagel, R. M. Virus persistence in acutely infected immunocompetent mice by exhaustion of antiviral cytotoxic effector T cells. Nature 362, 758–761 (1993).
Barber, D. L. et al. Restoring function in exhausted CD8 T cells during chronic viral infection. Nature 439, 682–687 (2006).
Blackburn, S. D. et al. Coregulation of CD8+ T cell exhaustion by multiple inhibitory receptors during chronic viral infection. Nat. Immunol. 10, 29–37 (2009).
Miller, B. C. et al. Subsets of exhausted CD8+ T cells differentially mediate tumor control and respond to checkpoint blockade. Nat. Immunol. 20, 326–336 (2019).
Zheng, L. et al. Pan-cancer single-cell landscape of tumor-infiltrating T cells. Science 374, abe6474 (2021).
Goronzy, J. J. & Weyand, C. M. Successful and maladaptive T cell aging. Immunity 46, 364–378 (2017).
Pereira, B. I. et al. Sestrins induce natural killer function in senescent-like CD8+ T cells. Nat. Immunol. 21, 684–694 (2020).
Palovics, R. et al. Molecular hallmarks of heterochronic parabiosis at single-cell resolution. Nature 603, 309–314 (2022).
Akbar, A. N. & Henson, S. M. Are senescence and exhaustion intertwined or unrelated processes that compromise immunity? Nat. Rev. Immunol. 11, 289–295 (2011).
Mogilenko, D. A. et al. Comprehensive profiling of an aging immune system reveals clonal GZMK+ CD8+ T cells as conserved hallmark of inflammaging. Immunity 54, 99–115 (2021).
Dutta, S. & Sengupta, P. Men and mice: relating their ages. Life Sci. 152, 244–248 (2016).
White, M. C. et al. Age and cancer risk: a potentially modifiable relationship. Am. J. Prev. Med. 46, S7–S15 (2014).
Scott, A. C. et al. TOX is a critical regulator of tumour-specific T cell differentiation. Nature 571, 270–274 (2019).
Pritykin, Y. et al. A unified atlas of CD8 T cell dysfunctional states in cancer and infection. Mol. Cell 81, 2477–2493 (2021).
Quinn, K. M. et al. Age-related decline in primary CD8+ T cell responses is associated with the development of senescence in virtual memory CD8+ T cells. Cell Rep. 23, 3512–3524 (2018).
Zhang, H. et al. Aging-associated HELIOS deficiency in naive CD4+ T cells alters chromatin remodeling and promotes effector cell responses. Nat. Immunol. 24, 96–109 (2023).
Parish, I. A. et al. The molecular signature of CD8+ T cells undergoing deletional tolerance. Blood 113, 4575–4585 (2009).
Zhang, H., Weyand, C. M. & Goronzy, J. J. Hallmarks of the aging T-cell system. FEBS J. 288, 7123–7142 (2021).
Cannarile, M. A. et al. Transcriptional regulator Id2 mediates CD8+ T cell immunity. Nat. Immunol. 7, 1317–1325 (2006).
Yang, C. Y. et al. The transcriptional regulators Id2 and Id3 control the formation of distinct memory CD8+ T cell subsets. Nat. Immunol. 12, 1221–1229 (2011).
Gautam, S. et al. The transcription factor c-Myb regulates CD8+ T cell stemness and antitumor immunity. Nat. Immunol. 20, 337–349 (2019).
Tsui, C. et al. MYB orchestrates T cell exhaustion and response to checkpoint inhibition. Nature 609, 354–360 (2022).
Wherry, E. J. et al. Molecular signature of CD8+ T cell exhaustion during chronic viral infection. Immunity 27, 670–684 (2007).
Saul, D. et al. A new gene set identifies senescent cells and predicts senescence-associated pathways across tissues. Nat. Commun. 13, 4827 (2022).
Yates, K. B. et al. Epigenetic scars of CD8+ T cell exhaustion persist after cure of chronic infection in humans. Nat. Immunol. 22, 1020–1029 (2021).
Whyte, W. A. et al. Master transcription factors and mediator establish super-enhancers at key cell identity genes. Cell 153, 307–319 (2013).
Vahedi, G. et al. Super-enhancers delineate disease-associated regulatory nodes in T cells. Nature 520, 558–562 (2015).
Shin, H. et al. A role for the transcriptional repressor Blimp-1 in CD8+ T cell exhaustion during chronic viral infection. Immunity 31, 309–320 (2009).
Wu, J. E. et al. In vitro modeling of CD8+ T cell exhaustion enables CRISPR screening to reveal a role for BHLHE40. Sci. Immunol. 8, eade3369 (2023).
Giordano, M. et al. Molecular profiling of CD8 T cells in autochthonous melanoma identifies Maf as driver of exhaustion. EMBO J. 34, 2042–2058 (2015).
Moore, M. J. et al. ZFP36 RNA-binding proteins restrain T cell activation and anti-viral immunity. eLife 7, e33057 (2018).
Petkau, G. et al. The timing of differentiation and potency of CD8 effector function is set by RNA binding proteins. Nat. Commun. 13, 2274 (2022).
Khan, K. et al. Unravelling structure, localization, and genetic crosstalk of KLF3 in human breast cancer. Biomed. Res. Int. 2020, 1354381 (2020).
Ilsley, M. D. et al. Kruppel-like factors compete for promoters and enhancers to fine-tune transcription. Nucleic Acids Res. 45, 6572–6588 (2017).
Shang, W. et al. Genome-wide CRISPR screen identifies FAM49B as a key regulator of actin dynamics and T cell activation. Proc. Natl Acad. Sci. USA 115, E4051–E4060 (2018).
Liu, B. et al. Temporal single-cell tracing reveals clonal revival and expansion of precursor exhausted T cells during anti-PD-1 therapy in lung cancer. Nat. Cancer 3, 108–121 (2022).
Qian, J. et al. A pan-cancer blueprint of the heterogeneous tumor microenvironment revealed by single-cell profiling. Cell Res. 30, 745–762 (2020).
Jerby-Arnon, L. et al. A cancer cell program promotes T cell exclusion and resistance to checkpoint blockade. Cell 175, 984–997 (2018).
Bonacina, F. et al. Myeloid apolipoprotein E controls dendritic cell antigen presentation and T cell activation. Nat. Commun. 9, 3083 (2018).
Hildner, K. et al. BATF3 deficiency reveals a critical role for CD8α+ dendritic cells in cytotoxic T cell immunity. Science 322, 1097–1100 (2008).
Schenkel, J. M. et al. Conventional type I dendritic cells maintain a reservoir of proliferative tumor-antigen specific TCF-1+ CD8+ T cells in tumor-draining lymph nodes. Immunity 54, 2338–2353 (2021).
Bottcher, J. P. et al. NK cells stimulate recruitment of cDC1 into the tumor microenvironment promoting cancer immune control. Cell 172, 1022–1037 (2018).
Dahling, S. et al. Type 1 conventional dendritic cells maintain and guide the differentiation of precursors of exhausted T cells in distinct cellular niches. Immunity 55, 656–670 (2022).
Vonderheide, R. H. CD40 agonist antibodies in cancer immunotherapy. Annu. Rev. Med. 71, 47–58 (2020).
Garris, C. S., Wong, J. L., Ravetch, J. V. & Knorr, D. A. Dendritic cell targeting with Fc-enhanced CD40 antibody agonists induces durable antitumor immunity in humanized mouse models of bladder cancer. Sci. Transl. Med. 13, eabd1346 (2021).
Sceneay, J. et al. Interferon signaling is diminished with age and is associated with immune checkpoint blockade efficacy in triple-negative breast cancer. Cancer Discov. 9, 1208–1227 (2019).
Al-Habsi, M. et al. Spermidine activates mitochondrial trifunctional protein and improves antitumor immunity in mice. Science 378, eabj3510 (2022).
Nakajima, Y., Chamoto, K., Oura, T. & Honjo, T. Critical role of the CD44lowCD62Llow CD8+ T cell subset in restoring antitumor immunity in aged mice. Proc. Natl Acad. Sci. USA 118, e2103730118 (2021).
Padron, A. et al. Age effects of distinct immune checkpoint blockade treatments in a mouse melanoma model. Exp. Gerontol. 105, 146–154 (2018).
Kaur, A. et al. sFRP2 in the aged microenvironment drives melanoma metastasis and therapy resistance. Nature 532, 250–254 (2016).
Fane, M. E. et al. Stromal changes in the aged lung induce an emergence from melanoma dormancy. Nature 606, 396–405 (2022).
Munshi, N. C. et al. Idecabtagene vicleucel in relapsed and refractory multiple myeloma. N. Engl. J. Med. 384, 705–716 (2021).
Rodriguez-Otero, P. et al. Ide-cel or standard regimens in relapsed and refractory multiple myeloma. N. Engl. J. Med. 388, 1002–1014 (2023).
Moller, S. H., Hsueh, P. C., Yu, Y. R., Zhang, L. & Ho, P. C. Metabolic programs tailor T cell immunity in viral infection, cancer, and aging. Cell Metab. 34, 378–395 (2022).
Yi, H. S. et al. T-cell senescence contributes to abnormal glucose homeostasis in humans and mice. Cell Death Dis. 10, 249 (2019).
Rojas, L. A. et al. Personalized RNA neoantigen vaccines stimulate T cells in pancreatic cancer. Nature 618, 144–150 (2023).
Nanishi, E., Levy, O. & Ozonoff, A. Waning effectiveness of SARS-CoV-2 mRNA vaccines in older adults: a rapid review. Hum. Vaccin. Immunother. 18, 2045857 (2022).
Jo, N. et al. Impaired CD4+ T cell response in older adults is associated with reduced immunogenicity and reactogenicity of mRNA COVID-19 vaccination. Nat. Aging 3, 82–92 (2023).
Kauffman, K. J. et al. Optimization of lipid nanoparticle formulations for mRNA delivery in vivo with fractional factorial and definitive screening designs. Nano Lett. 15, 7300–7306 (2015).
Das, R. et al. Hybrid LNP prime dendritic cells for nucleotide delivery. Adv. Sci. 10, e2303576 (2023).
Corces, M. R. et al. Lineage-specific and single-cell chromatin accessibility charts human hematopoiesis and leukemia evolution. Nat. Genet. 48, 1193–1203 (2016).
Orchard, P., Kyono, Y., Hensley, J., Kitzman, J. O. & Parker, S. C. J. Quantification, dynamic visualization, and validation of bias in ATAC-seq data with ataqv. Cell Syst. 10, 298–306 (2020).
Acknowledgements
We thank all members of the Sen laboratory for thoughtful scientific discussions. We thank the Massachusetts General Hospital Flow Cytometry Core and the Massachusetts General Hospital Animal Research Facility for their assistance. We are grateful for helpful advice from R. Das (Massachusetts General Hospital Center for Systems Biology) for some in vivo immunotherapy experiments. D.R.S. was supported by funding from the Melanoma Research Alliance, the V Foundation for Cancer Research and NIH DP2AI176139. A.C.Y.C. was supported by funding from NIH T32CA921643.
Author information
Authors and Affiliations
Contributions
A.C.Y.C. and D.R.S. conceived the study and designed the experiments. A.C.Y.C., S.J., D.M., C.Y., K.J., V.M., M.E.F., S.A.W., M.Z. and K.T. performed experiments and/or data analysis. C.S.G., T.R.M. and N.H. provided critical reagents and advice. A.C.Y.C., S.J., D.M. and D.R.S. wrote the paper. All authors reviewed and edited the paper. All authors are familiar with the Nature Portfolio Inclusion and Ethics guidelines.
Corresponding author
Ethics declarations
Competing interests
The authors declare no competing interests.
Peer review
Peer review information
Nature Immunology thanks Jorg Goronzy and the other, anonymous, reviewer(s) for their contribution to the peer review of this work. Primary Handling Editor: N. Bernard, in collaboration with the Nature Immunology team.
Additional information
Publisher’s note Springer Nature remains neutral with regard to jurisdictional claims in published maps and institutional affiliations.
Extended data
Extended Data Fig. 1 Phenotyping of CD8+ T cells in young vs. aged tumors.
Related to Fig. 1. (a) Representative flow cytometry plots (left) and frequencies of splenic CD8+ T cells that were either naive (PD-1-CD44−; right-top), activated (PD-1+CD44+; right-center), or antigen experienced (PD-1+Tim-3+; right-bottom) cells in mice that ranged in 5-85 weeks of age. (b) Representative flow cytometry gating strategy utilized to quantify tumor-infiltrating CD8+ T cells as well as tetramer (Tet+) specific, and BrdU+ CD8+ T cells in young and aged tumor-bearing mice. (c) Representative flow cytometry gating strategy used to measure lymphatic CD8+ T cells as well as tetramer (Tet+) specific CD8+ T cells in young and aged tumor-bearing mice. (d) ScRNA-seq UMAP projection of 5862 aged tumor-infiltrating CD8+ T cells defined in Fig. 1d. (e) Corresponding violin plots of scRNA-seq that denote RNA read counts (nFeature_RNA; left), and the percentage of mitochondrial genes per cell (% mitochondrial reads; right) for each cluster. (f) Hashtag of scRNA-seq profiles reveals distinct biological replicates, denoted by color, in young (left) and aged (right) tumor-bearing mice. (g) Expression of indicated genes (grey: low, purple: high). (h) Heatmap shows top cluster-defining genes in each CD8+ T cell cluster/subset identified in Fig. 1d. (i) Quantification of subsets within live tetramer (Tet+) antigen-experienced (CD44+ PD-1+) CD8+ T cells, classified based on the Tim-3 and Slamf6 expression in young (blue, n = 8) and aged (red, n = 4) tumor-bearing mice. (j) Geometric mean fluorescence intensity (MFI) of IL-2, IFN-γ, TNF, Gzmb, and BrdU in live antigen-experienced (CD44+ PD-1+) tumor-infiltrating CD8+ T cells from young (blue; n = 7) and aged (red; n = 4) tumor-bearing mice, separated by subsets. For panels a, i and j: Summary of at least two independent experiments. Mean ± s.d. For i and j, significance was calculated using a two-sided Student’s t-test. Asterisks used to indicate significance corresponds to the following: N.S. (not significant, P > 0.05), *P ≤ 0.05, **P ≤ 0.01, and ***P ≤ 0.001).
Extended Data Fig. 2 Transcriptional profiling of T cell-intrinsic defects in aging.
Related to Fig. 2. (a) Schematic of single and co-transfers OT-1 CD8+ T cells into young tumor bearing recipient mice and the corresponding representative flow cytometry gating strategy utilized to quantify endogenous and transferred OT-1 CD8+ T cells in young tumor bearing recipient mice. (b) Schematic of young and aged splenic OT-1 CD8+ T cell co-transfer into young recipient mice experiments. scRNA-seq UMAP projection of 7731 tumor-infiltrating OT-1 CD8+ T cells from co-transfer of young and aged splenic OT-1 CD8+ T cell into young recipient mice, colored by cluster. Expression of indicated genes (grey: low expression, purple: high expression). (c) Violin plots that denote RNA read counts (nFeature_RNA; top), total number of genes in each cell (nCount_RNA; top second), and the percentage of mitochondrial genes (% mitochondrial reads; top third) and ribosomal genes (% ribosomal reads; bottom) per cell. (d) Heatmap showing top cluster-defining genes in each CD8+ T cell cluster/subset identified in Fig. 2b. (e) Relative proportion of young (OT-1Y>Y, n = 5) and aged (OT-1A>Y, n = 5) CD8+ T cells within progenitor-like exhausted (TProg), terminally exhausted (TTerm), and dividing (TDivi) clusters. (f) Experimental design for in vivo transfer assay to study TProg formation and maintenance at early time points, such as days 7 and 9, between OT-1Y>Y (blue) and OT-1A>Y (red) in young B16-OVA tumor-bearing mice on days 7, 9, and 15. Proportion of TProg, TTran, and TTerm between OT-1Y>Y and OT-1A>Y on days 7, 9, and 15 (n = 9-10). (g) UMAP projection of scRNA-seq profiles from 6231 live splenic OT-1 CD8+ T cells from young and aged mice, colored by cluster (C). C1, young naive OT-1 CD8+ T cells (Young Spl TNaiv); C2, aged naive OT-1 CD8+ T cells (Aged Spl TNaiv); C3, aged memory-like OT-1 CD8+ T cells (Aged Spl TMem-like); C4, aged effector-like OT-1 CD8+ T cells (Aged Spl TEff-like); C5, young effector-like OT-1 CD8+ T cells (young Spl TEff-like); C6, aged exhausted-like OT-1 CD8+ T cells (Aged Spl TExh). Expression of indicated genes (grey: low expression, purple: high expression). i: Tcf7, ii: Pdcd1, iii: Ccr7, iv: Sell, v: Tox. (h) Heatmap showing top cluster-defining genes in each of splenic OT-1 CD8+ T cell cluster/subset identified in Extended Data Fig. 2e, with a specific focus of the top 7 genes in cluster 2 (C2). (i) UMAP projection of scRNA-seq profiles of young splenic OT-1 (Spl OT-1Y), aged splenic OT-1 PD-1- (Spl OT-1A), aged splenic OT-1 PD-1+ (Spl OT-1A PD-1+), and wildtype young splenic (WTY) CD8+ T cells. Frequencies of each sample within different cell clusters. Mean ± s.d. For e and f, significance was calculated using a two-sided Student’s t-test. Asterisks used to indicate significance corresponds to the following: N.S. (not significant, P > 0.05), *P ≤ 0.05, **P ≤ 0.01, and ***P ≤ 0.001).
Extended Data Fig. 3 Epigenetic profiling of aged T cell-intrinsic defects at baseline.
Related to Fig. 3. (a) Transcriptional start site (TSS) enrichment of chromatin accessibility profiles from aged and young pre-transfer splenic as well as tumor-infiltrating OT-1 CD8+ T cell subsets. (b) Representative ATAC-seq tracks of aged (yellow) and young (green) splenic naive OT-1 CD8+ T cells at the indicated gene loci. (c) Pathway enrichment in splenic young OT-1 CD8+ T cells (Spl OT-1Y, green) and splenic aged OT-1 CD8+ T cells (Spl OT-1A, yellow) chromatin accessible regions (ChARs). The FDR values (hypergeometric test) are presented as -log10(q-value).
Extended Data Fig. 4 Cell-extrinsic effects of aging on differentiation and function of CD8+ T cells.
Related to Fig. 4, part 1. (a) Experimental design and tumor growth curves for B16-OVA tumors in young (10w; blue; n = 17) and aged (68w; red; n = 16) recipient mice treated with young spleen OT-1 CD8+ T cells (Spl OT-1Y). (b) Frequency of transferred OT-1 CD8+ T cells isolated from young (10w, blue; n = 17) and aged (68w; red; n = 16) recipient B16-OVA tumor-bearing mice. (c) B16-OVA tumor volumes at day 15 from the cell-intrinsic model (left, young mice receiving either young or aged splenic OT-1), and cell-extrinsic model (right, young or aged mice received young spleen OT-1). (d) Experimental design for in vitro killing assay and percentage of cytotoxicity of sorted young (blue) and aged (red) OVA tetramer+ (Tet+) intratumoral CD8+ T cell subsets at 1:1 effector to target (E:T; OT-1: B16-OVA) ratio (n = 4-6). (e) Experimental design for in vivo transfer and T cell differentiation. (f) Schematic of young and aged intratumoral Tet+ CD8+ T cell subsets transfer into young recipient mice experiments. The corresponding representative flow cytometry gating strategy is utilized to quantify transferred Tet+ CD8+ T cell subsets in young tumor-bearing recipient mice. (g) Frequency of transferred OT-1 CD8+ T cells differentiated into different CD8+ T cell subsets (n = 4-5). Mean ± s.d. For b, d, and g, significance was calculated using a two-sided Student’s t-test. Asterisks used to indicate significance corresponds to the following: N.S. (not significant, P > 0.05), *P ≤ 0.05, **P ≤ 0.01, and ***P ≤ 0.001).
Extended Data Fig. 5 Epigenetic and transcriptional profiling of T cell-extrinsic defects in aged tumors.
Related to Fig. 4, part 2. (a) ScRNA-seq UMAP projection of 4263 tumor-infiltrating OT-1 CD8+ T cells from transfer of young splenic OT-1 CD8+ T cell into young or aged recipient tumor-bearing mice, colored by cluster. Expression of indicated genes (grey: low, purple: high). (b) Heatmap showing top cluster-defining genes in each CD8+ T cell cluster/subset identified in Fig. 4e. (c) Violin plots that denote RNA read counts (nFeature_RNA; top), total number of genes in each cell (nCount_RNA; top second), and the percentage of mitochondrial genes (% mitochondrial reads; bottom) per cell, related to Fig. 4e, f. (d) Volcano plot of differentially expressed genes between transferred young OT-1 (OT-1Y) subsets: progenitor-like exhausted (TProg; brown) and terminally exhausted (TTerm; blue) as well as the differentially expressed genes between TTerm (blue) and tumor-infiltrating age-associated dysfunctional (TTAD; red) OT-1 CD8+ T cells. (e) Heatmap showing Z-scored TF regulon activity within cell-type clusters identified by Seurat. (f) Principal component analysis (PCA) projection and transcriptional start site (TSS) enrichment of chromatin accessibility profiles from tumor-infiltrating CD8+ T cell subsets in young and aged tumor-bearing mice. (g) Violin plots that denote RNA read counts (nFeature_RNA; top), total number of genes in each cell (nCount_RNA; top second), related to Fig. 4j–l. (h) Heatmap showing top cluster-defining genes in each CD8+ T cell cluster/subset identified in Fig. 4j. (i) Volcano plot of differentially expressed genes between human tumor-infiltrating T cell subsets: TTerm vs. TTAD CD8+ T cells.
Extended Data Fig. 6 Phenotyping of immune cells in young vs. aged tumors.
Related to Fig. 5. (a) Representative flow cytometry gating strategy (top) utilized to quantify intratumoral CD45+ cells in young and aged tumor-bearing mice, including conventional CD4+ T cells, CD8+ T cells, natural killer (NK) cells, and subsets of dendritic cells (DCs), macrophages (Mac), Ly6C+ monocytes (Mono), and conventional dendritic cells type1 (cDC1s) and 2 (cDC2s) within DCs. Representative flow cytometry gating strategy (bottom) utilized to lymphatic CD8+ T cells, tetramer (Tet+) specific CD8+ T cells, subsets of dendritic cells (DCs), and cDC1/cDC2 within DCs in young and aged tumor-bearing mice. (b) Heatmap shows top cluster-defining genes in each cell cluster/subset identified in Fig. 5c. (c) Volcano plot of differentially expressed genes between intratumoral T, NK, and myeloid cells from young (blue) and aged (red) tumor-bearing mice. (d) All the significant ligand-receptor interactions between NK, cDC1, and CD8+ T cells in young (blue) and aged (red) tumor-bearing mice. The dot color and size represent the calculated communication probability and p-values. (e) Percentage of intratumoral CD8+ T cells and OVA tetramer+ (Tet+) intratumoral CD8+ T cells from B16-OVA tumor-bearing mice with different ages, from 10w, 24w, 44w, up to 68w old (n = 6-8). (f) Percentage of intratumoral CD8+ T cell subsets (such as TTerm and TTAD) from B16-OVA tumor-bearing mice with different ages, from 10w, 24w, 44w, up to 68w old (n = 6-8). Mean ± s.d. For b, d, e, f, and g, significance was calculated using a two-sided Student’s t-test. Asterisks used to indicate significance corresponds to the following: N.S. (not significant, P > 0.05), *P ≤ 0.05, **P ≤ 0.01, and ***P ≤ 0.001).
Extended Data Fig. 7 Transcriptional profiling of CD8+ T cells after myeloid-targeted immunotherapy.
Related to Fig. 6. (a) ScRNA-seq UMAP projection of 3454 tumor-infiltrating CD8+ T cells defined in Fig. 6h. (b) Expression of indicated genes (grey: low, purple: high). (c) Corresponding violin plots of scRNA-seq that denote RNA read counts (nFeature_RNA; up), and the percentage of mitochondrial genes per cell (% mitochondrial reads; down) for each cluster. (d) Heatmap shows top cluster-defining genes in each CD8+ T cell cluster/subset identified in Fig. 6h. (e) ScRNA-seq UMAP projection of different tumor-infiltrating CD8+ T cell cluster proportion from untreated young/aged, and young/aged treated with CD40 agonist antibody mice. (f) Quantification of naive (TNaiv), transitory exhausted (TTran), IFN-reponse (TIFN), dividing (TDivi), and Lars2+ (TLars2+) subsets based on scRNA with individual mouse hashtags (n = 5). (g) Volcano plot of differentially expressed genes between transferred terminally exhausted (TTerm; brown) and different subsets, including tumor-infiltrating age-associated dysfunctional (TTAD; red), progenitor-like exhausted (TProg; golden yellow), memory-like (TMem-like), and effector-like (TEff-like) subsets. (h) Dot plot of different expression levels of critical surface markers and transcriptional regulators among each CD8+ T cell cluster. Mean ± s.d. For f, significance was calculated using a two-sided Student’s t-test. Asterisks used to indicate significance corresponds to the following: N.S. (not significant, P > 0.05), *P ≤ 0.05, **P ≤ 0.01, and ***P ≤ 0.001).
Supplementary information
Supplementary Information
Supplementary Tables 1–20.
Supplementary Tables 1–20
Supplementary Table 1. Gene expression by cluster in scRNA-seq data from Fig. 1d. Each row is a gene; columns include P value, log2 fold change, percentage of cells with non-zero transcript, Bonferroni correction of observed differences and cluster identity. Supplementary Table 2. Gene expression by cluster in scRNA-seq data from Fig. 2b. Each row is a gene; columns include P value, log2 fold change, percentage of cells with non-zero transcript, Bonferroni correction of observed differences and cluster identity. Supplementary Table 3. Differential gene expression between aged and young OT-1 TTerm cells in scRNA-seq data from Fig. 2d. Each row is a gene; columns include P value, log2 fold change, percentage of cells with non-zero transcript, Benjamini–Hochberg and FDR correction of observed differences. Supplementary Table 4. Gene expression by cluster in scRNA-seq data from Fig. 2h. Each row is a gene; columns include P value, log2 fold change, percentage of cells with non-zero transcript, Bonferroni correction of observed differences and cluster identity. Supplementary Table 5. Differential gene expression between aged and young splenic TNaiv cells in scRNA-seq data from Fig. 2i. Each row is a gene; columns include P value, log2 fold change, percentage of cells with non-zero transcript, Benjamini–Hochberg and FDR correction of observed differences. Supplementary Table 6. GSEA of differential open chromatin regions between aged and young OT-1 TTerm cells from Fig. 3c. Each row is a gene set; columns include FDR q value, hypergeometric fold enrichment, –log10 (qvalue) and direction of significant enrichment. Supplementary Table 7. GSEA of differential open chromatin regions between aged and young splenic TNaiv cells from Extended Data Fig. 3c. Each row is a gene set; columns include FDR q value, hypergeometric fold enrichment, –log10 (q value) and direction of significant enrichment. Supplementary Table 8. Gene expression by cluster in scRNA-seq data from Fig. 4e. Each row is a gene; columns include P value, log2 fold change, percentage of cells with non-zero transcript, Bonferroni correction of observed differences and cluster identity. Supplementary Table 9. Differential gene expression between denoted clusters in scRNA-seq data from Extended Data Fig. 5d. Each row is a gene; columns include P value, log2 fold change, percentage of cells with non-zero transcript, Benjamini–Hochberg and FDR correction of observed differences. Supplementary Table 10. Summary of genes assigned to differential open chromatin regions between endogenous TTerm and TTAD cells from aged mice in Fig. 4i. Supplementary Table 11. Summary of publicly available samples used for the human pan-cancer scRNA-seq analysis from Fig. 4j–l. Each row is an individual; columns include dataset, cancer type, participant ID, sex, age, age group, stage, TNM classification, pathological subtype, molecular status, sample type, scRNA-seq ID and treatment. Supplementary Table 12. Gene expression by cluster in scRNA-seq data from Fig. 4j. Each row is a gene; columns include P value, log2 fold change, percentage of cells with non-zero transcript, Bonferroni correction of observed differences and cluster identity. Supplementary Table 13. Differential gene expression between human TTAD and TTerm cells in scRNA-seq data from Extended Data Fig. 5i. Each row is a gene; columns include P value, log2 fold change, percentage of cells with non-zero transcript, Benjamini–Hochberg and FDR correction of observed differences. Supplementary Table 14. Gene expression by cluster in scRNA-seq data from Extended Data Fig. 6b. Each row is a gene; columns include P value, log2 fold change, percentage of cells with non-zero transcript, Bonferroni correction of observed differences and cluster identity. Supplementary Table 15. Differential gene expression between aged and young T cells, NK cells and myeloid cells in scRNA-seq data from Extended Data Fig. 6c. Each row is a gene; columns include P value, log2 fold change, percentage of cells with non-zero transcript, Benjamini–Hochberg and FDR correction of observed differences. Supplementary Table 16. Differential gene expression between aged and young DCs in scRNA-seq data from Fig. 5d. Each row is a gene; columns include P value, log2 fold change, percentage of cells with non-zero transcript, Benjamini–Hochberg and FDR correction of observed differences. Supplementary Table 17. Differentially enriched interactions between aged and young DCs, NK cells and CD8+ T cells in scRNA-seq data from Extended Data Fig. 6d. Each row is a receptor–ligand interaction; columns include sender–receiver pairing, interaction type, enrichment in young and aged and delta of enrichment. Supplementary Table 18. Gene expression by cluster in scRNA-seq data from Fig. 6h. Each row is a gene; columns include P value, log2 fold change, percentage of cells with non-zero transcript, Bonferroni correction of observed differences and cluster identity. Supplementary Table 19. Differential gene expression between denoted clusters in scRNA-seq data from Extended Data Fig. 7g. Each row is a gene; columns include P value, log2 fold change, percentage of cells with non-zero transcript, Benjamini–Hochberg and FDR correction of observed differences. Supplementary Table 20. Flow cytometry antibodies. All antibodies used in this study, listing target protein, conjugated fluorophore, antibody clone, manufacturer and catalog number.
Source data
Source Data Figs. 1–6 and Extended Data Figs. 1, 2, 4, 6 and 7
Statistical source data.
Rights and permissions
Springer Nature or its licensor (e.g. a society or other partner) holds exclusive rights to this article under a publishing agreement with the author(s) or other rightsholder(s); author self-archiving of the accepted manuscript version of this article is solely governed by the terms of such publishing agreement and applicable law.
About this article
Cite this article
Chen, A.C.Y., Jaiswal, S., Martinez, D. et al. The aged tumor microenvironment limits T cell control of cancer. Nat Immunol (2024). https://doi.org/10.1038/s41590-024-01828-7
Received:
Accepted:
Published:
DOI: https://doi.org/10.1038/s41590-024-01828-7