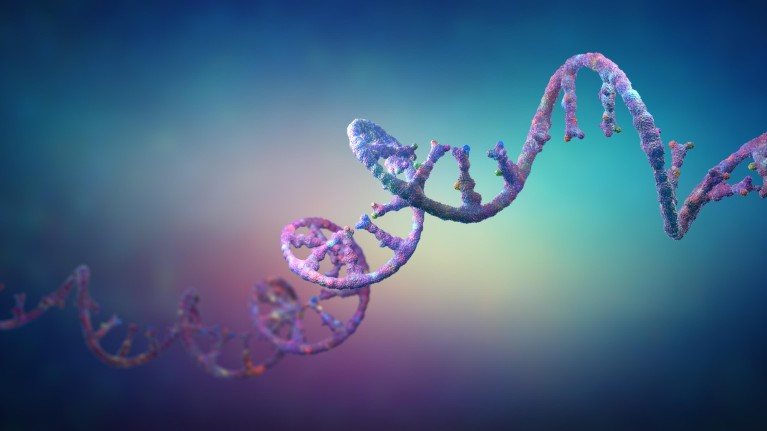
An efficient approach to finding and analysing the RNA species of interest, such as mRNA or relevant non-coding RNA, is to remove the abundant and unwanted species of RNA first.Credit: Christoph Burgstedt/Shutterstock
The transcriptome has been attracting a lot of attention of late. Using RNA sequencing (RNA-Seq), researchers probe the RNA in cells and tissues to understand underlying biological processes.
The transcriptome, however, is a busy place, and there are lots of different species of RNA. Messenger RNA (mRNA) codes for proteins, whereas non-coding RNA (ncRNA) serves other roles, many of which are unknown.
There is growing evidence that both small ncRNAs, such as microRNAs, and long ncRNAs are vital regulators of biological processes and could serve as biomarkers of disease and therapeutic targets.
Long ncRNAs, more than 200 nucleotides in length, can fold into a variety of structures to interact with DNA, RNA and proteins. Through various mechanisms, long ncRNAs are involved in the regulation of cell survival, growth and maintenance of stemness.
MicroRNAs also regulate gene expression, mainly by inducing the degradation of mRNA and repressing translation. Because miRNAs can be secreted into extracellular fluids and transported to target cells, they are thought to be important mediators of cell–cell communication. The most abundant ncRNA by far, however, is ribosomal RNA (rRNA), which binds with enzymes and proteins to make up ribosomes.
“rRNA contributes more than 80% of the total cellular RNA,” says Joanna Williams, a molecular neurobiologist working in the Brain Health Research Centre at the University of Otago in New Zealand. “If it is included in the sequencing protocol, most of the information returned will represent the rRNA.” Thus, removal of rRNA is a crucial step in streamlining the sequencing process and revealing the relevant data.
For efficient transcriptomic analysis, researchers can use one of several methods to home in on the RNA species of interest. Techniques involve either removing the unwanted RNA biotypes or enriching the target RNAs. An ideal approach would be fast, simple and accurate, and easily integrated with existing workflows.
“We need a method that can be flexibly adapted to sample type or aim of the project,” says Rastislav Horos, chief technology officer at Hummingbird Diagnostics, a company using RNA-Seq to develop microRNA-based diagnostics to guide the safe and effective use of therapeutics.
The challenge of rRNA removal
There are two commonly used methods to remove or work around rRNA. One approach involves enriching the polyadenylated (poly-A) RNA, which allows selection of mainly mRNAs. However, this approach doesn’t just exclude rRNAs (which don’t have a poly-A tail), but many other transcripts that are not polyadenylated, such as long ncRNAs, small regulatory ncRNAs, non-polyadenylated mRNAs, or mRNAs that have lost their poly-A tail due to degradation, as often happens in formalin-fixed paraffin-embedded (FFPE) samples. This approach limits the view of the transcriptome and results in an incomplete analysis.
The other method involves targeted depletion of rRNA, allowing analysis of a more varied population of RNA species. First-generation techniques removed rRNA by binding it to complementary DNA oligonucleotides, which are either coupled to magnetic beads or biotinylated sequence-specific probes that can be identified and removed; second-generation methods used ribonuclease H to digest the rRNA–DNA strands. Both methods are used as pre-treatments to cDNA synthesis.
Many targeted rRNA removal methods can be lengthy, inefficient and require multiple steps. Further, they often require relatively high amounts of starting material that could preclude their use when working with FFPE or other samples with degraded RNA. There is now a third approach, that Williams’s team uses.
The transcriptome in neuroscience
Williams and colleagues are exploring changes in brain regions affected by Alzheimer's disease. “Alzheimer's has long been considered to be caused by the accumulation of amyloid-ß peptide in the brain, based on observations from the rare individuals who have the inherited form of the disease where mutations increase the load of amyloid-ß in the brain,” she explains. Her team wants to understand the disease process in individuals without such mutations.
Nikita Potemkin, a researcher in Williams’ group, used a kit from QIAGEN when developing a method to simultaneously explore coding RNAs and regulatory ncRNAs in frozen brain samples1. “Removal of rRNA using FastSelect was extremely efficient, resulting in less than 1.5% rRNA content in the final library,” he says.
QIAGEN’s third-generation method removes cytoplasmic and mitochondrial rRNA from total RNA in one step, and in less than 14 minutes. The QIAseq FastSelect kits use complementary, highly modified locked-nucleic acid (LNA) oligonucleotides that tightly bind to rRNA, blocking cDNA synthesis, without the need to remove or degrade them (see 'The easy way to remove unwanted RNA').
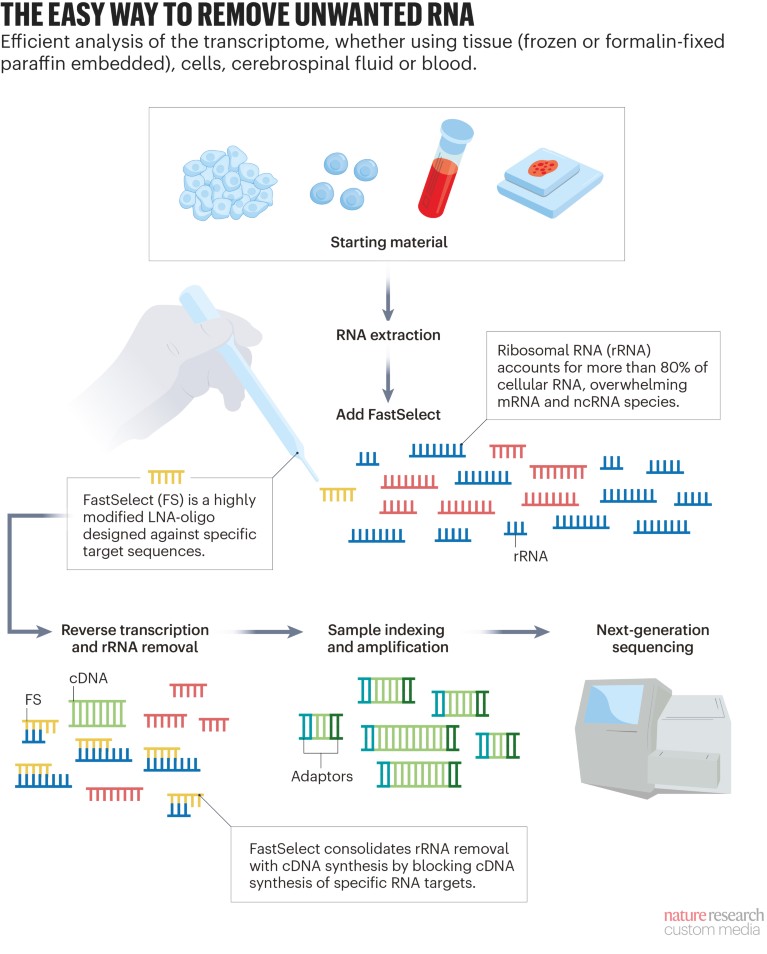
“The major advantages of using this kit are its seamless integration into existing library preparation protocols and the fact that it does not require any additional purification, precipitation or enrichment steps, thereby minimizing sample handling and sample loss,” Potemkin says.
Using Potemkin’s protocol, the researchers identified more than 15,000 unique transcripts in the human samples, including protein‑coding RNAs and many species of low-abundance ncRNAs. When they compared the RNAs from patients against healthy controls, they found differences in the expression of genes known to be associated with Alzheimer’s disease, as well as several differentially expressed microRNAs.
“We are currently exploring the biological relevance of our findings,” says Williams. “The changes we observe in RNA biotype profiles may reflect aspects of disease pathology and cognitive impairment.”
Customizable removal of unwanted RNAs
Peripheral whole blood is one of the most accessible tissues, making it ideally suited to the discovery of biomarkers of disease and response to treatment. Characterizing the blood transcriptome has its own challenges.
Erythrocyte-derived microRNAs can account for up to 50% of all sequencing reads from blood, obscuring RNA transcripts of interest. When carrying out RNA-Seq, it can be desirable to remove these microRNAs as well as rRNA.
Horos has been working with QIAGEN on a custom FastSelect reagent for whole blood samples. “By efficiently blocking unwanted microRNAs, we have widened the sequencing bandwidth to other, low abundant microRNAs,” he says, adding that this has not only accelerated discovery but also improved the economics of data generation. “Ultimately, a modular library of reagents ready to block any RNA that a researcher deems unimportant would be a great toolkit for every laboratory performing RNA sequencing.”
Using the custom reagent, Horos and colleagues discovered a 5-microRNA signature in blood that can predict survival following immunotherapy in advanced non-small cell lung cancer patients2. These findings will help determine which patients should be treated with immunotherapy alone and which will require additional chemotherapy3. “Being able to tailor therapeutic interventions based on blood-borne microRNAs could lead to marked improvements in patient outcomes,” he says.
Maximizing next-generation sequencing capacity demands ever faster and more-efficient solutions. FastSelect rRNA removal and custom design capability, along with QIAGEN’s RNA-Seq library prep and downstream bioinformatic analysis, help increase RNA sequencing sensitivity, save resources, and make sequencing more affordable.