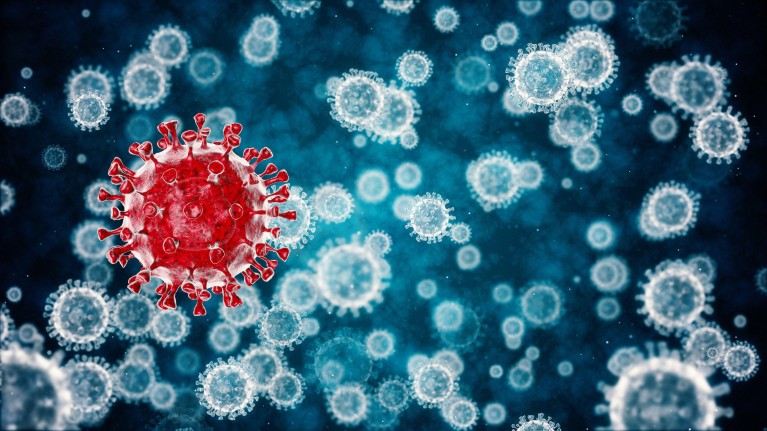
Researchers at UAEU have created an AI-enabled system that selects the best model for predicting pandemic infection patterns.STANCA SANDA / Alamy Stock Photo
The COVID-19 pandemic has taken a serious toll across the world not only due to the numbers of deaths, but also the associated pressures on healthcare systems. Moreover, governmental measures such as lockdowns have had their own impacts on factors such as mental health and employment.
Modelling the spread of COVID-19 across different countries is critical in helping governments tackle future outbreaks as efficiently as possible, but current infection prediction models have limited accuracy. Now, an international team led by scientists at UAEU has developed an automated, real-time Artificial Intelligence (AI)-enabled system for accurate prediction of COVID-19 infection growth, given a country’s past infection trends.
“For governments and healthcare organizations to plan and manage resources efficiently during pandemics, we need an AI-enabled system that predicts infection growth with accuracy and ideally without requiring human intervention, in order to make the process as efficient as possible,” says founding Director of Intelligent Distributed Computing and Systems Laboratory at UAEU Leila Ismail, who led the project.
The researchers used World Health Organization databases for COVID-19 to build and train their system, which is unique in the way it tailors its output. Rather than using just one prediction model, it hosts a selection of existing prediction models that have been adopted for COVID-19. The AI selection process identifies the type of trend—for example, linear or exponential—in a country’s data and chooses the most appropriate model to use for future predictions. The system then trains the model using that country’s current dataset, and produces predictions for the most likely scenario in that place.
“Given the difference in geographical characteristics and social behaviors of different countries, one model cannot be applied to all nations,” says Ismail. “Because our system tunes into each specific scenario, it also captures and identifies temporal trends, including the changes due to seasonality, and other introduced events such as lockdowns and vaccine rollouts.”
Noting how well the team’s AI-enabled system performed and improved on predictions of infection trends, Ismail is confident it can be employed globally. “We have learned from our research that machine learning models should be data-driven, and they can only be used effectively to control a pandemic and its consequences if they are tailored to infection data trends,” says Ismail.
UAEU’s system provides valuable insights into infection curves, and should allow policymakers to enhance, ease, or lift restrictions on daily life with more confidence. Improved infection prediction could also contribute to smart healthcare planning and provision.