“It’s natural that Korea became a medical data hub,” says Sungroh Yoon, leader of the Data Science & Artificial Intelligence Laboratory at Seoul National University.
South Korea, the world’s 11th largest economy and home to tech giants such as Samsung and LG Electronics, has a population of more than 51 million people, half of whom live in Seoul and surrounds.The huge number of people, with a culture of early technology adoption, combined with South Korea’s strong computing infrastructure and biological research strengths, make the country an obvious personalized medicine test-bed. But there are many obstacles to realizing such a goal.
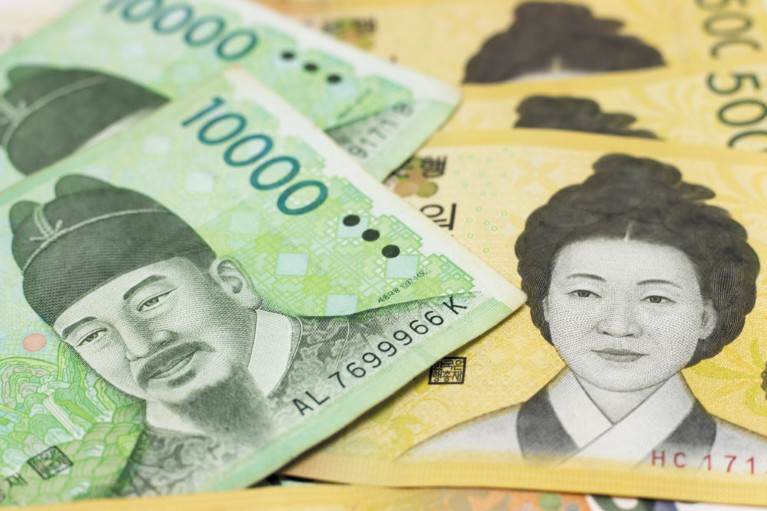
South Korea’s spending on R&D as a percentage of its GDP is the highest in the world.©dankuhs/iStock/Getty
A different approach
The promise of personalized medicine has emerged through advances in genetics. A mass of data has arisen through the falling costs of genome sequencing, with the potential to define precisely what treatment method will work for an individual.“With genetic information we will be able to tailor treatment plans individually,” explains Sun Kim, a professor at Seoul National University’s Department of Computer Science and Engineering.
The ability to treat patients without wasting time and money on superfluous tests will be particularly important for the South Korean economy in the coming decades: it is forecast that people over 65 will make up 41% of the population by 2060.
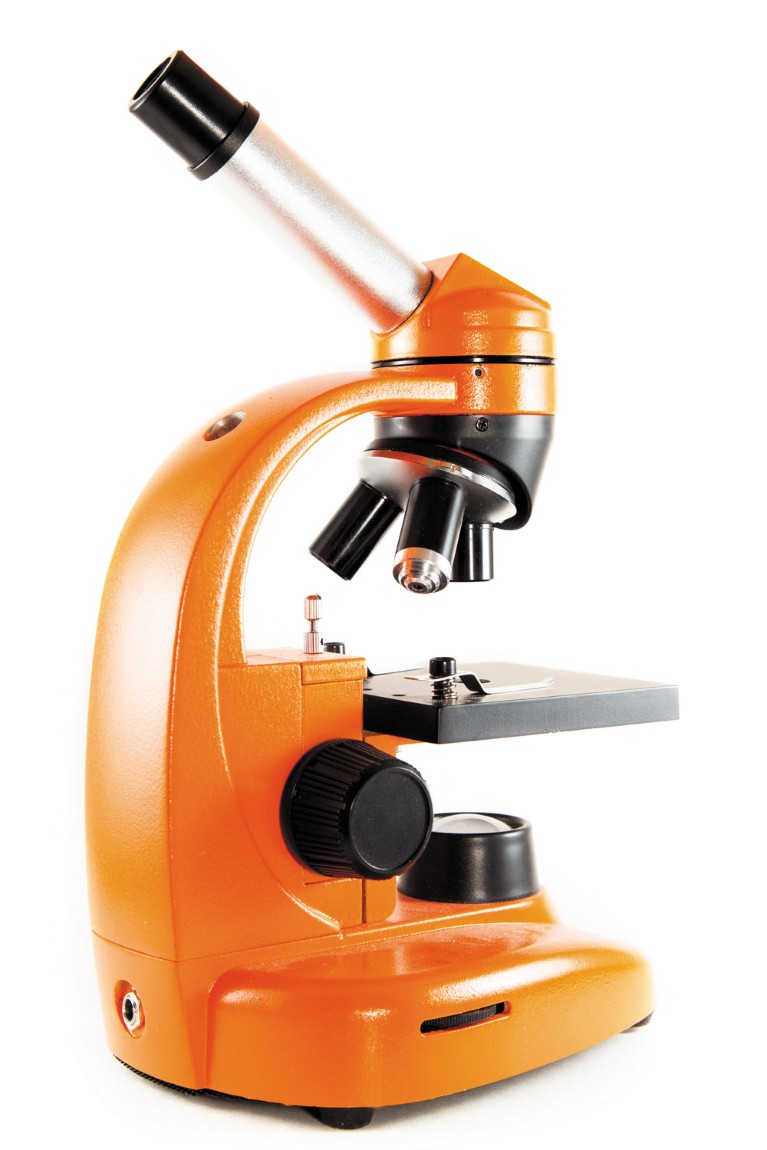
The global precision medicine market is predicted to reach US$85.5 billion by 2025.©Tatiana/iStock/Getty
A problem of many dimensions
Making use of all these data, however, is not straightforward. At the moment, the high-dimension, low-sample-size nature of the genetic data makes it a very complicated problem in terms of computer science.
“Dimensionally, genetic data is huge,” explains Kim. “Humans have 3.2 billion DNA characters. If you factor in mutations, there are well over 50 million dimensions, and epigenetics is even bigger. Gene expression and transcription — add another 20,000. So put it all together, we’re easily looking at 100-200 million dimensions.”
But the recent developments in big data and artificial intelligence (AI) offer a tantalizing possibility to manage such mind-boggling numbers, not only for personalized medicine, but also for drug development and genome editing.“We can’t deal with all these dimensions, so we use machine learning to reduce all these dimensions into a manageable space,” says Kim.
A community effort
The complexities of dealing with these data is why many bioinformatics researchers are making their codes freely available online. “Sharing the code is a community effort. We need to share and work together to mine the data,” explains Kim.
Jaewoo Kang, leader of the Database & Information Systems group at Korea University agrees and adds that by releasing codes, other researchers in the field are able to build on it and apply it to their own problems.
An example of the community spirit of the field at work is the annual DREAM challenge, initiated by IBM research in 2007. Every year, DREAM organizers set challenges that teams of researchers from around the globe try to answer. In 2018, teams were tasked with using public and private databases to find multi-targeting drug candidates. Kang’s team won the challenge using an AI-driven model — ReSimNet, a Siamese Neural Networks-based model — to predict the transcriptional response similarity of candidate drugs. Along with other high ranked entries, Kang’s candidates are now being verified experimentally.
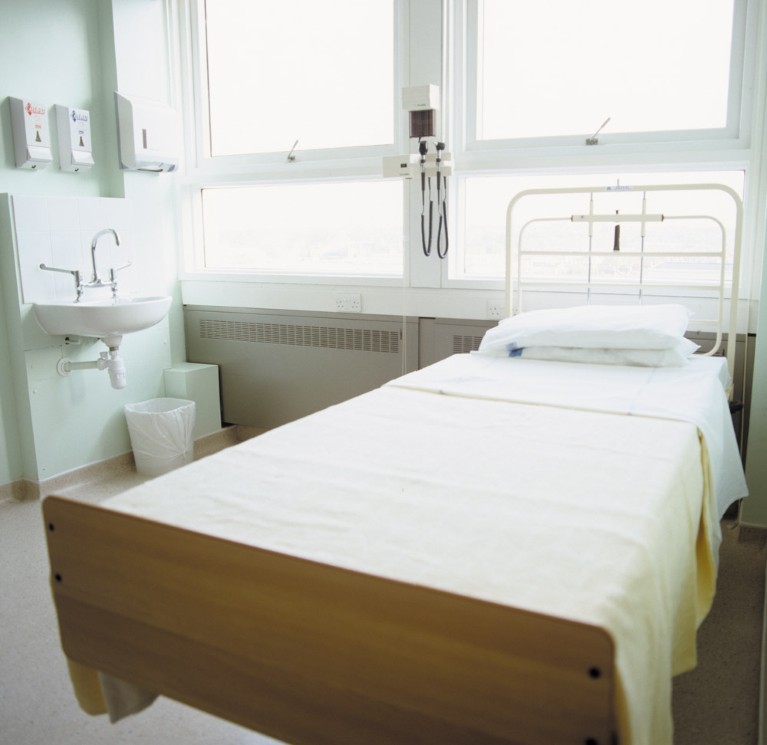
Just five hospitals account for 12.1% of the hospital beds in South Korea.Science Photo Library - Adam Gault/Brand X Pictures/Getty
A lack of data
Curiously, the other great challenge facing big-data-driven medicine is a lack of data. “The amount of genetic data is still not enough to train complex models. It’s hard to collect large-scale data sets from patients,” says Kang.Privacy concerns and ethical conundrums mean that not all data collected is available for analysis. Uniformity of data sets also poses a problem.For example, electronic medical record (EMR) data is often taken at irregular time intervals, such as when a patient visits a medical professional, and thus is very difficult to analyze. It is also quite common that EMRs from different individuals may have different fields or missing entries, which can make analysis very difficult.
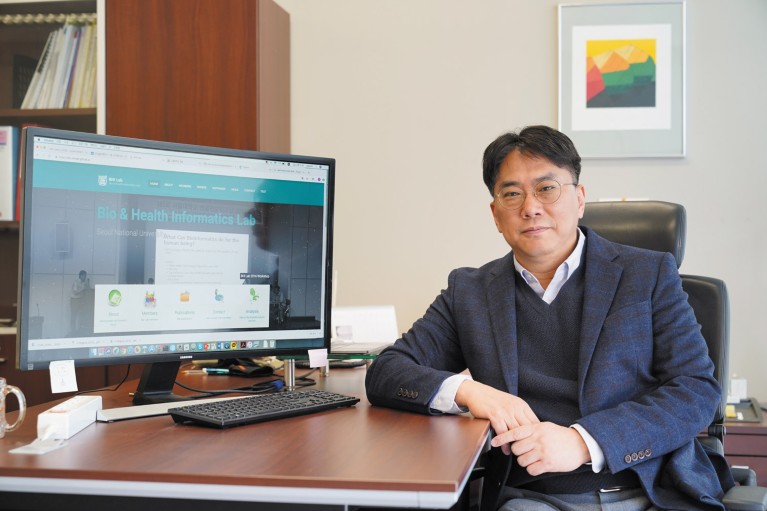
Sun Kim is the director of the Bioinformatics Institute at SEOUL NATIONAL UNIVERSITY.
Deep generative models, which can generate realistic samples from training data, are a recent advance in AI which could assist in bridging the gaps. “We are investigating deep generative methods to pre-process EMRs which often contain missing or incomplete fields,” says Yoon. He explains that by leveraging this technique, researchers can fill empty entries and perform EMR analyses more effectively, benefiting patients in a variety of situations.Integrating EMR data with genomic data will be extremely difficult. Expanding that to include daily mobile health data collected from wearables such as smart watches, becomes a task beyond mere human brains, so very high-level computer technology will be needed, “but that’s the exciting thing about working in this area,” says Kim.
Ultimately, Kim would like to be able to use machine learning to draw all of this genetic, epigenetic and medical record and health data together into a single metric space. “By doing so, we can help realize personalized medicine and may be able to understand diseases, such as metabolic syndrome, better.”