Insurance statistics show a sharp rise in thefts of catalytic converters, devices fitted to vehicles to change pollutants in exhaust gases to less toxic gases, as supply-chain issues have boosted the price of rare metals such as rhodium, platinum and palladium in the muffler-sized devices. Rhodium, for example, now trades at more than US$10,000 per ounce, roughly five times its price before the COVID-19 pandemic.
“One solution to the problem of rare metals would be to use more-abundant materials and significantly reduce rare elements in vehicle components such as magnets and catalysts,” says Tetsuya Shoji, a project head of the Materials Informatics and Digital Transformation Wavebase project of Toyota Motor Corporation in Japan. “We could reduce their cost, stabilize global purchasing, and even simultaneously improve their performance.”
But strategy needs to be nimble in the face of rapidly changing conditions, as the changes imposed by the pandemic have demonstrated. “Since targets might fluctuate in the future, our strategy is to use digital tools to consider all directions,” says Shoji.
And it’s not just catalysts that stand to benefit from adopting such a material informatics approach to material design. Other vehicle components, including batteries, power semiconductors and magnets, will also gain from this technology. “We’re using material informatics to transform materials research and development,” says Shoji.
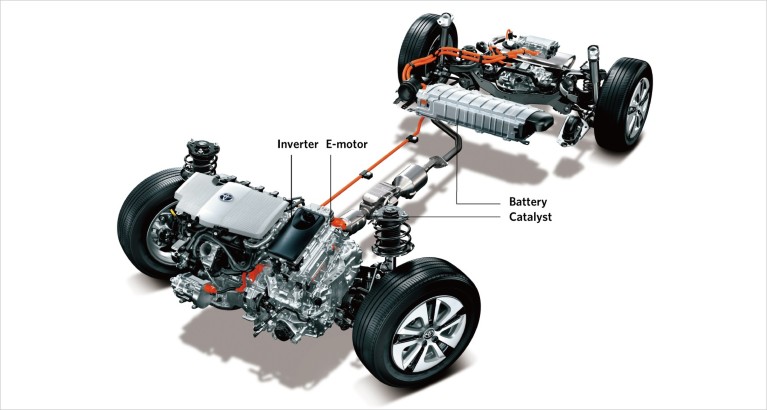
Toyota engineers are targeting various materials in automobiles. In particular, three-way catalytic converters have been stripping exhaust of dangerous pollutants for half a century and will continue to be a key pollution-reduction technology going into the future.
Catching the digital wave
To address this challenge, Shoji and his colleagues have been developing a data-analysis cloud service that can decipher the complexities of developing advanced materials for automobiles. Known as the Wavebase project1, the promising new algorithms are now being deployed beyond Toyota to boost Japanese industry as a whole. The system employs non-coding tools for analysing all material data acquired during experiments.
“People have been modelling material information for years, and ideally all this data should be gathered into a single database,” says Shoji. “That’s what we are trying to build, to realize this kind of information integration.”
The team uses various types of radiation beams including X-rays and neutrons to investigate the atomic arrangement and higher level structure. “X-ray diffraction and spectral measurements include various information — the diffraction data reveals the atomic structure, while scattering signals at low angles reveal nanostructure. Both kinds of information are contained in one spectrum,” says Shoji.
Even for relatively small data sets containing 20 to 30 X-ray diffraction and X-ray absorption spectra, the Wavebase system allows researchers to extract certain features — a material’s porosity or magnetism, for example. It does this by employing dimensionality reduction — mathematical techniques that transform large sets of variables into smaller groups with related features. This gives Wavebase the ability to quantify data that is inherently difficult to quantify, such as spectra of magnets and carbon, and then using it to inform machine learning.
“Many researchers just use their eyes to extract features from electron microscopy images,” notes Shoji. “But these images are static and it takes a lot of skill to correctly interpret nanostructures in them. We’re trying to use the scattering principle to automatically extract nano-features, and then use microscopy to support these predictions.”
Designed with an intuitive drag-and-drop interface, the Wavebase system enables teams to automatically build databases and visualize the results of the feature-extracting algorithms as diagrams ranging from experimental data to completely virtually generated data.
Catalytic conundrums
To demonstrate the power of materials informatics technology, the team has applied it to catalytic converters2, which reduce pollution emission from exhaust gas. A key aspect of Toyota’s three-way catalyst system is its oxygen storage capacity (OSC) materials.
This is the kind of complex challenge that materials informatics algorithms are ideally suited to address. Experimentalists and computational material scientists at Toyota and Toyota Central R&D Labs teamed up to search for new candidates for OSC materials. They synthesised 60 kinds of metal oxides with various crystal structures and then measured each crystal’s OSC response at different temperatures. After collecting the experimental results in a database, the team generated a calibration curve that relates OSC performance to crystallographic structure and components related the electronic state. Finally, they created a prediction model using artificial-intelligence algorithms and applied this model to other materials from a database. They successfully predicted an entirely new OSC material copper niobium oxide. Toyota researchers are using materials informatics to broaden the usage of catalysts. For example, they are predicting catalyst performance using small, powder-based samples instead of scaled-up catalytic converters for vehicles.
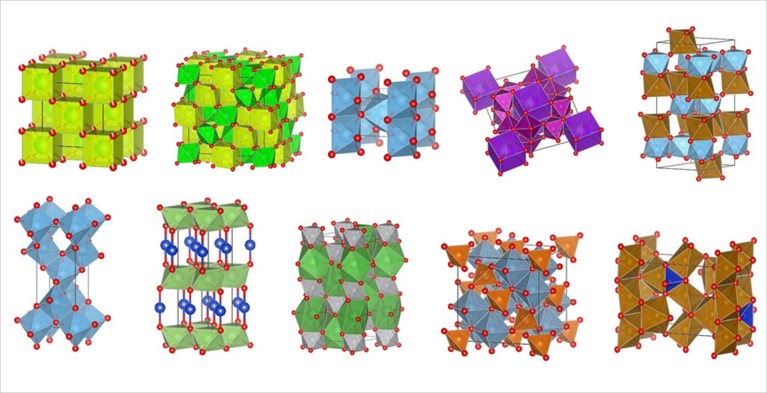
The atomic structures of ten doped catalytic materials. As there are hundreds of thousands of possible structures, Toyota has created a materials informatics analytics platform called Wavebase to analyse massive data.
Uncovering hidden material principles
The ability to visualize the latent space generated by hidden material features extracted by AI, which map various new crystals, has been useful for identifying materials with potential multipurpose capabilities.
“Battery and catalyst materials sometimes have very similar structures, especially in the way they store and transport ions,” says Hirohito Hirata, general manager of Toyota’s Advanced Material Engineering Division. “When we see overlapping points on these maps, we know that there’s commonality between their functions.”
The team is currently developing a platform that will allow anyone to utilize material informatics, and they are expanding so that it can be applied to other vehicle components, such as magnets and batteries. Material data generated by research in Toyota’s Advanced Material Engineering Division are analysed and collected by the Wavebase system. By using Wavebase as a data-sharing platform, Toyota and partners in Japan and overseas can share data with collaborators, accelerating research in a data-driven way.
In the future, the platform could be used at synchrotron-radiation facilities, such as SPring-8 in Japan, to enable on-site analysis of huge, high-dimensional data. This could drastically alter experimental design and decision making in material development due to faster acquisition of data3 and material insights. Amassing a large variety of data may allow Wavebase to extract key, underlying principles of materials.
The Wavebase system is highly customizable. For example, Sumitomo Rubber Industries of Japan were able to drastically accelerate analysis of massive data generated in synchrotron experiments for tyre development by using Wavebase to analyse data obtained over years of research.
“We’re always looking to collaborate with people who recognize our level of technology,” says Shoji. “And the amount of statistical data we have just keeps growing4,5; we’ve already calculated more than two million data sets and also have strategy for utilizing them for future R&D scene.”